# Factor Expr [![status][ci_badge]][ci_page] [![pypi][pypi_badge]][pypi_page]
[ci_badge]: https://github.com/dovahcrow/factor-expr/workflows/ci/badge.svg
[ci_page]: https://github.com/dovahcrow/factor-expr/actions
[pypi_badge]: https://img.shields.io/pypi/v/factor-expr?color=green&style=flat-square
[pypi_page]: https://pypi.org/project/factor-expr/
<center>
<table>
<tr>
<th>Factor Expression</th>
<th></th>
<th>Historical Data</th>
<th></th>
<th>Factor Values</th>
</tr>
<tr>
<td>(TSLogReturn 30 :close)</td>
<td>+</td>
<td>2019-12-27~2020-01-14.pq</td>
<td>=</td>
<td>[0.01, 0.035, ...]</td>
</tr>
</table>
</center>
----------
Extreme fast factor expression & computation library for quantitative trading in Python.
On a server with an E7-4830 CPU (16 cores, 2000MHz),
computing 48 factors over a dataset with 24.5M rows x 683 columns (12GB) takes 150s.
Join [\[Discussions\]](https://github.com/dovahcrow/factor-expr/discussions) for Q&A and feature proposal!
## Features
* Express factors in [S-Expression](https://en.wikipedia.org/wiki/S-expression).
* Compute factors in parallel over multiple factors and multiple datasets.
## Usage
There are three steps to use this library.
1. Prepare the datasets into files. Currently, only the [Parquet](https://parquet.apache.org/) format is supported.
2. Define factors using [S-Expression](https://en.wikipedia.org/wiki/S-expression).
3. Run `replay` to compute the factors on the dataset.
### 1. Prepare the dataset
A dataset is a tabular format with float64 columns and arbitrary column names.
Each row in the dataset represents a tick, e.g. for a daily dataset, each row is one day.
For example, here is an OHLC candle dataset representing 2 ticks:
```python
df = pd.DataFrame({
"open": [3.1, 5.8],
"high": [8.8, 7.7],
"low": [1.1, 2.1],
"close": [4.4, 3.4]
})
```
You can use the following code to store the DataFrame into a Parquet file:
```python
df.to_parquet("data.pq")
```
### 2. Define your factors
`Factor Expr` uses the S-Expression to describe a factor.
For example, on a daily OHLC dataset, the 30 days log return on the column `close` is expressed as:
```python
from factor_expr import Factor
Factor("(TSLogReturn 30 :close)")
```
Note, in `Factor Expr`, column names are referred by the `:column-name` syntax.
### 3. Compute the factors on the prepared dataset
Following step 1 and 2, you can now compute the factors using the `replay` function:
```python
from factor_expr import Factor, replay
result = await replay(
["data.pq"],
[Factor("(TSLogReturn 30 :close)")]
)
```
The first parameter of `replay` is a list of dataset files and the second parameter is a list of Factors. This gives you the ability to compute multiple factors on multiple datasets.
Don't worry about the performance! `Factor Expr` allows you parallelize the computation over the factors as well as the datasets by setting `n_factor_jobs` and `n_data_jobs` in the `replay` function.
The returned result is a pandas DataFrame with factors as the column names and `time` as the index.
In case of multiple datasets are passed in, the results will be concatenated with the exact order of the datasets. This is useful if you have a scattered dataset. E.g. one file for each year.
For example, the code above will give you a DataFrame looks similar to this:
| index | (TSLogReturn 30 :close) |
| ----- | ----------------------- |
| 0 | 0.23 |
| ... | ... |
Check out the [docstring](#replay) of `replay` for more information!
## Installation
```bash
pip install factor-expr
```
## Supported Functions
Notations:
* `<const>` means a constant, e.g. `3`.
* `<expr>` means either a constant or an S-Expression or a column name, e.g. `3` or `(+ :close 3)` or `:open`.
Here's the full list of supported functions. If you didn't find one you need,
consider asking on [Discussions](https://github.com/dovahcrow/factor-expr/discussions) or creating a PR!
### Arithmetics
* Addition: `(+ <expr> <expr>)`
* Subtraction: `(- <expr> <expr>)`
* Multiplication: `(* <expr> <expr>)`
* Division: `(/ <expr> <expr>)`
* Power: `(^ <const> <expr>)` - compute `<expr> ^ <const>`
* Negation: `(Neg <expr>)`
* Signed Power: `(SPow <const> <expr>)` - compute `sign(<expr>) * abs(<expr>) ^ <const>`
* Natural Logarithm after Absolute: `(LogAbs <expr>)`
* Sign: `(Sign <expr>)`
* Abs: `(Abs <expr>)`
### Logics
Any `<expr>` larger than 0 are treated as `true`.
* If: `(If <expr> <expr> <expr>)` - if the first `<expr>` is larger than 0, return the second `<expr>` otherwise return the third `<expr>`
* And: `(And <expr> <expr>)`
* Or: `(Or <expr> <expr>)`
* Less Than: `(< <expr> <expr>)`
* Less Than or Equal: `(<= <expr> <expr>)`
* Great Than: `(> <expr> <expr>)`
* Greate Than or Equal: `(>= <expr> <expr>)`
* Equal: `(== <expr> <expr>)`
* Not: `(! <expr>)`
### Window Functions
All the window functions take a window size as the first argument. The computation will be done on the look-back window with the size given in `<const>`.
* Sum of the window elements: `(TSSum <const> <expr>)`
* Mean of the window elements: `(TSMean <const> <expr>)`
* Min of the window elements: `(TSMin <const> <expr>)`
* Max of the window elements: `(TSMax <const> <expr>)`
* The index of the min of the window elements: `(TSArgMin <const> <expr>)`
* The index of the max of the window elements: `(TSArgMax <const> <expr>)`
* Stdev of the window elements: `(TSStd <const> <expr>)`
* Skew of the window elements: `(TSSkew <const> <expr>)`
* The rank (ascending) of the current element in the window: `(TSRank <const> <expr>)`
* The value `<const>` ticks back: `(Delay <const> <expr>)`
* The log return of the value `<const>` ticks back to current value: `(TSLogReturn <const> <expr>)`
* Rolling correlation between two series: `(TSCorrelation <const> <expr> <expr>)`
* Rolling quantile of a series: `(TSQuantile <const> <const> <expr>)`, e.g. `(TSQuantile 100 0.5 <expr>)` computes the median of a window sized 100.
#### Warm-up Period for Window Functions
Factors containing window functions require a warm-up period. For example, for
`(TSSum 10 :close)`, it will not generate data until the 10th tick is replayed.
In this case, `replay` will write `NaN` into the result during the warm-up period, until the factor starts to produce data.
This ensures the length of the factor output will be as same as the length of the input dataset. You can use the `trim`
parameter to let replay trim off the warm-up period before it returns.
## Factors Failed to Compute
`Factor Expr` guarantees that there will not be any `inf`, `-inf` or `NaN` appear in the result, except for the warm-up period. However, sometimes a factor can fail due to numerical issues. For example, `(Pow 3 (Pow 3 (Pow 3 :volume)))` might overflow and become `inf`, and `1 / inf` will become `NaN`. `Factor Expr` will detect these situations and mark these factors as failed. The failed factors will still be returned in the replay result, but the values in that column will be all `NaN`. You can easily remove these failed factors from the result by using `pd.DataFrame.dropna(axis=1, how="all")`.
## I Want to Have a Time Index for the Result
The `replay` function optionally accepts a `index_col` parameter.
If you want to set a column from the dataset as the index of the returned result, you can do the following:
```python
from factor_expr import Factor, replay
pd.DataFrame({
"time": [datetime(2021,4,23), datetime(2021,4,24)],
"open": [3.1, 5.8],
"high": [8.8, 7.7],
"low": [1.1, 2.1],
"close": [4.4, 3.4],
}).to_parquet("data.pq")
result = await replay(
["data.pq"],
[Factor("(TSLogReturn 30 :close)")],
index_col="time",
)
```
Note, accessing the `time` column from factor expressions will cause an error.
Factor expressions can only read `float64` columns.
## API
There are two components in `Factor Expr`, a `Factor` class and a `replay` function.
### Factor
The f
没有合适的资源?快使用搜索试试~ 我知道了~
用于 Python 量化交易的极速因子表达式和计算库。
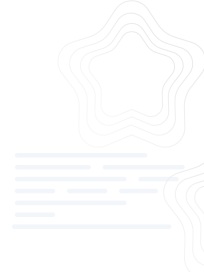
共44个文件
rs:23个
py:9个
yml:3个

需积分: 49 8 下载量 154 浏览量
2021-06-28
19:36:28
上传
评论
收藏 1.26MB ZIP 举报
温馨提示
因子表达式因子表达 历史数据 因子值 (TSLogReturn 30 :关闭) + 2019-12-27~2020-01-14.pq = [0.01, 0.035, ...] 用于 Python 量化交易的极速因子表达式和计算库。在配备 E7-4830 CPU(16 核,2000MHz)的服务器上,在 2450 万行 x 683 列 (12GB) 的数据集上计算 48 个因子需要 150 秒。加入[讨论]进行问答和功能提案!特征在S-Expression 中表达因子。在多个因子和多个数据集上并行计算因子。用法使用这个库需要三个步骤。将数据集准备成文件。目前,仅支持Parquet格式。使用S-Expression定义因子。运行replay以计算数据集上的因子。 1.准备数据集数据集是具有 float64 列和任意列名的表格格式。数据集中的每一行代表一个刻度,例如对于每日数据集,每一行代表一天。例如,这是一个 OHLC 蜡烛数据集,表示 2 个价格变动点:df=pd .DataFrame ({"open" : [3.1 ,5.8 ],"high" : [8.8 ,7.7 ],"low" :
资源推荐
资源详情
资源评论
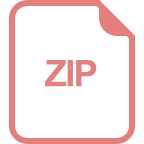
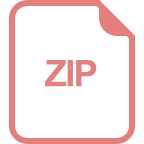
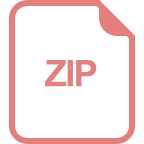
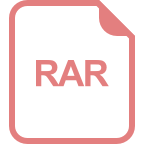
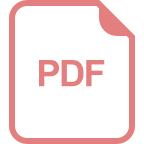
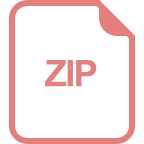
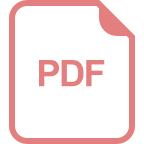
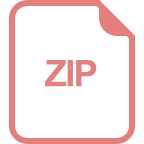
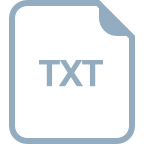
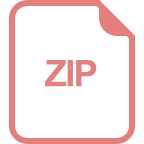
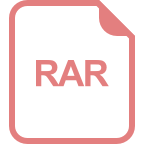
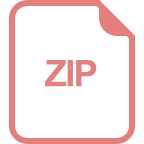
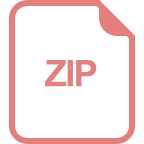
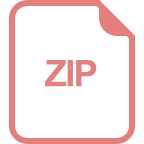
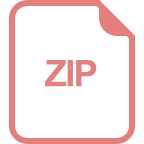
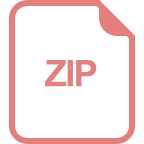
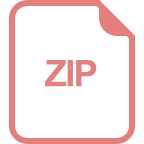
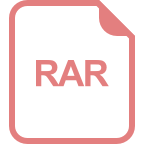
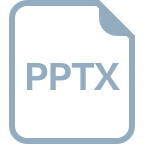
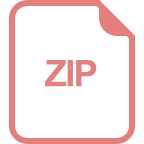
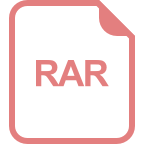
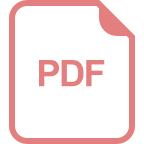
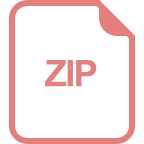
收起资源包目录



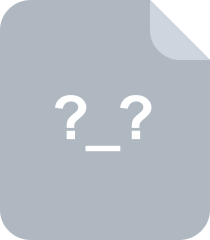
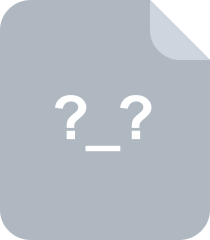

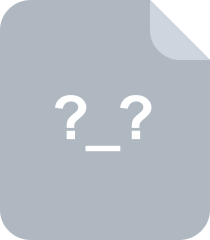
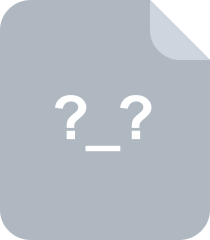
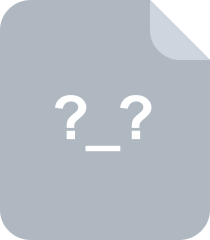

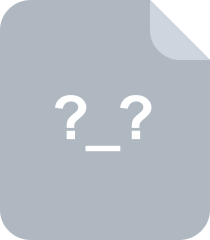

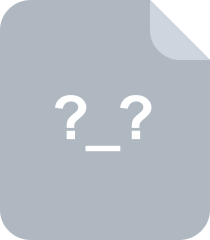
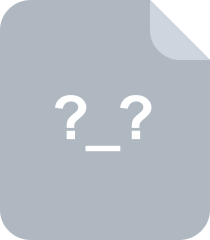
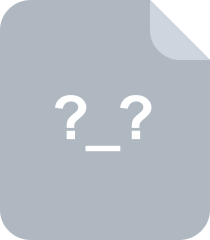
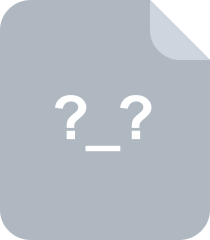
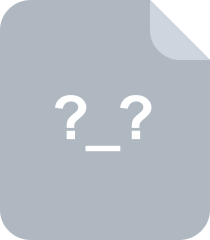
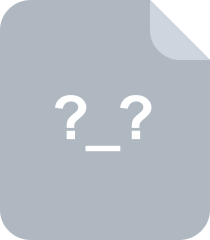
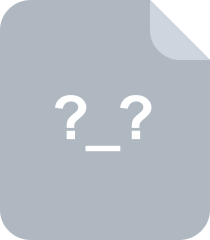
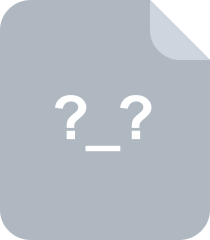
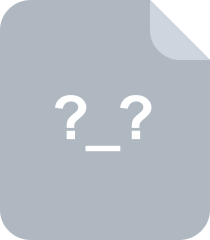
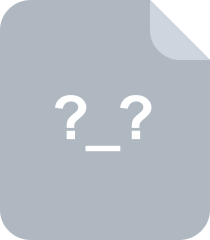
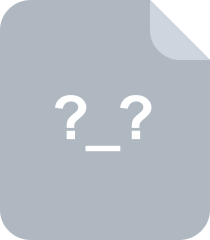
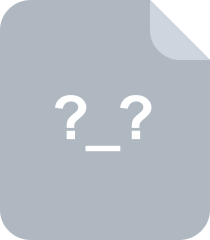
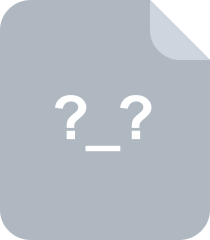
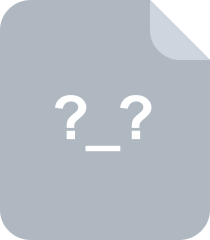
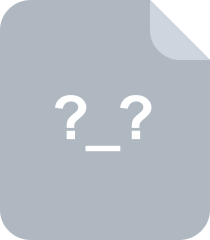
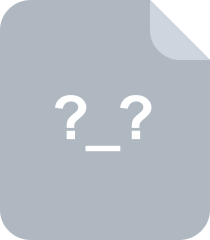
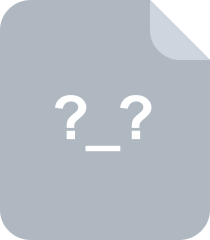
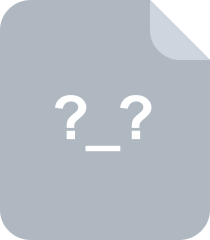
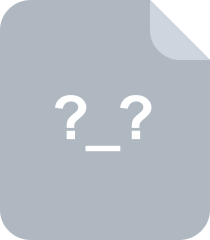
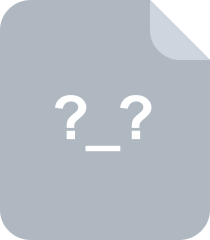


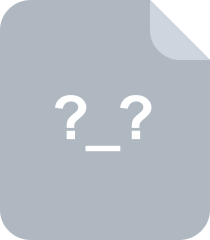
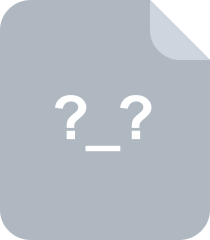
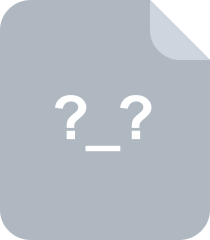

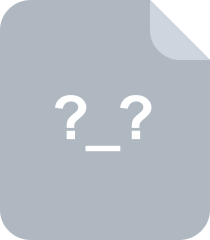

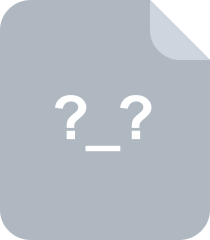
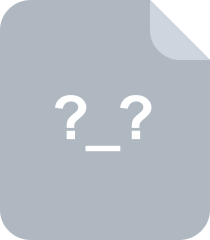



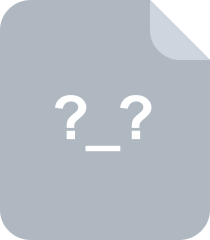
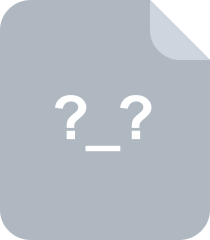
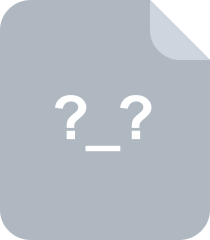
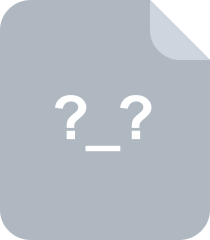
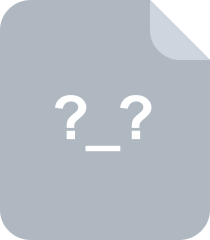
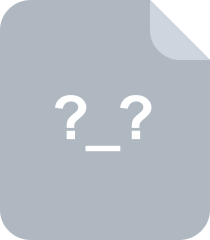
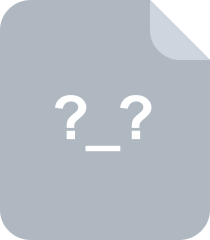
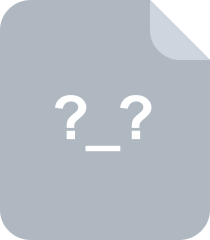
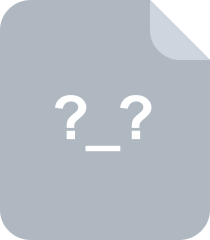

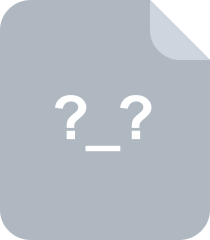
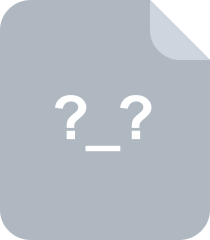

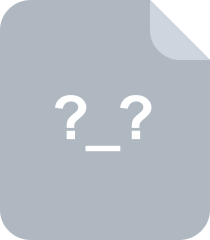
共 44 条
- 1
资源评论
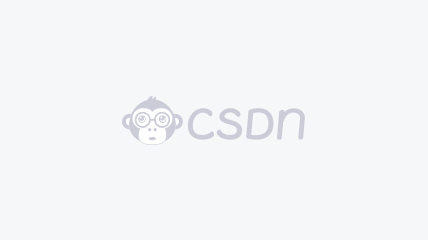

weixin_38748555
- 粉丝: 6
- 资源: 933
上传资源 快速赚钱
我的内容管理 展开
我的资源 快来上传第一个资源
我的收益
登录查看自己的收益我的积分 登录查看自己的积分
我的C币 登录后查看C币余额
我的收藏
我的下载
下载帮助

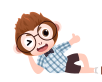
最新资源
- Python实现AVL树:自平衡二叉搜索树的构建与维护
- Python中的文本分析技术:从特征提取到模型应用
- 基于C++的Qt+mysql实现医院信息管理系统源码+数据库脚本(高分项目)
- NOI 全国青少年信息学奥林匹克竞赛(官网)-2024.11.05.pdf
- 【Unity抢劫和犯罪题材的低多边形3D资源包】POLYGON Heist - Low Poly 3D Art
- 网络安全是一个广泛的领域,涉及的知识和技能非常多样.docx
- 用Python实现,PySide构建GUI界面的“井字棋”游戏 具备学习功能(源码)
- 系统测试报告模板 测试目的、测试依据、测试准备、测试内容、测试结果及分析、总结
- 雷柏2.4G无线鼠标键盘对码软件V3.1
- Python基础入门-待办事项列表.pdf
资源上传下载、课程学习等过程中有任何疑问或建议,欢迎提出宝贵意见哦~我们会及时处理!
点击此处反馈


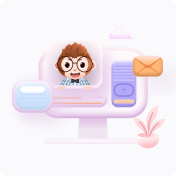
安全验证
文档复制为VIP权益,开通VIP直接复制
