没有合适的资源?快使用搜索试试~ 我知道了~
传统的多变量分析(MVA)故障诊断方法通常要求分离的采样数据潜在变量必须服从正态分布,这通常很难满足实际的工业过程。 本文首先介绍了一种基于Q统计量的故障诊断方法。 它要求采样数据必须服从正态分布。 然后介绍一种基于信息增量矩阵(IIM)的故障诊断方法,该方法的采样数据不受正态分布的限制。 该方法主要由定义协方差矩阵,计算信息增量矩阵,信息增量均值和动态阈值等组成。 最后,给出了一个数值模拟的例子和一个田纳西州的伊斯曼过程的例子,以验证两种错误诊断方法,即Q统计量和IIM,在误报和漏报中的检测性能。 结果表明,在采样数据不服从正态分布的情况下,Q统计方法的检测性能较差,而基于IIM的故障诊断方法较好。
资源推荐
资源详情
资源评论
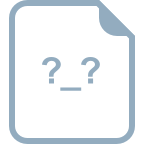
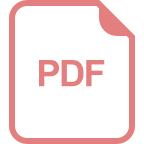
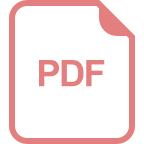
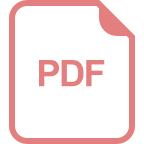
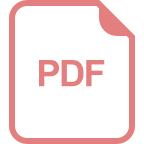
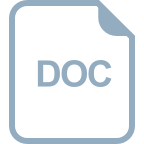
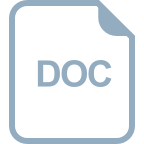
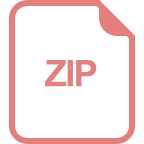
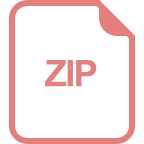
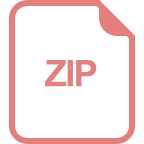
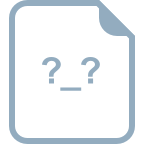
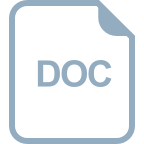
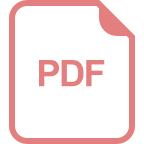
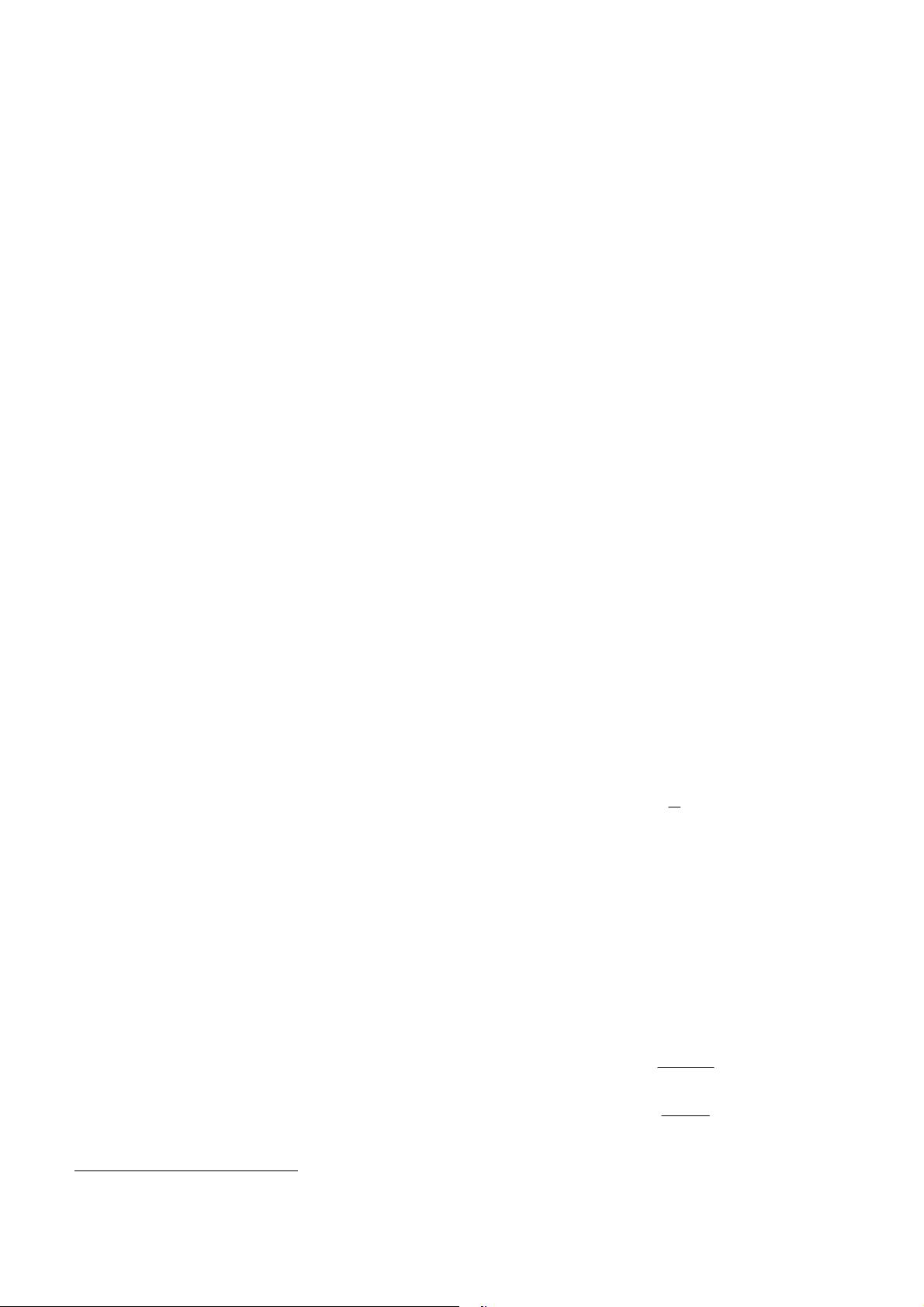
Comparison of Multivariate Analysis methods with Application to Fault
Diagnosis for non-Gaussian process
Xu Jiapeng, Wen Chenglin
Institute of Systems Science and Control Engineering, school of Automation,
Hangzhou Dianzi University, Hangzhou Zhejiang 310018, China
E-mail: imxjp@sina.com, E-mail: wencl@hdu.edu.cn
Abstract: Traditional fault diagnosis methods of multivariate analysis (MVA) usually require that sampling data of separated
latent variables must be subject to normal distribution, which is usually difficult to meet the actual industrial p rocesses. This
paper firstly introduces a method of fault diagnosis based on Q statistic. It requires that sampling data must be subject to normal
distribution. Then this paper introduces a method of fault diagnosis based on information incremental matrix (IIM), whose
sampling data h aven’t the limitation of n ormal distribution. The method is mainly composed of defining covariance matrix,
calculating information incremental matrix, information incremental mean and dynamic threshold, and so on. Finally, this paper
gives a example of numerical simulation and a example of Tennessee Eastman Process (TEP), to verify the detection
performance of two fault diagnosis methods, i.e., Q statistic and IIM, in false and missed alarm. The results show that Q statistic
method have poor detection performance in the case that sampling data are not subject to normal distribution, while the method
of fault diagnosis based on IIM is better.
Key words
fault d iagnosis;non-Gaussian process; Q statistic; information incremental matrix
1 Introduction
In industrial systems, many of the actual processes
contain both Gaussian and non-Gaussian process [1], so the
sampling data acquired from industrial systems are usually
not subject to normal distribution. Currently, most
data-driven fault diagnosis methods based on MVA require
that sampling data are subject to normal distribution, such as
Principal Component Analysis (PCA), Relative Principal
Component Analysis (RPCA) [2], Designated Component
Analysis (DCA) [3]. Ding introduced a fault diagnosis
method based on Q statistic in the literature [4], which is a
method of MVA like the PCA. The method firstly
establishes a mon itoring mod e l based on extensive historical
data, then it can determine whether the new observational
data deviate monitoring model, to judge whether the system
is faulty. This method requires that the sample data are
subject to normal distribution and it is only applicable to
invariant model. However, most of industrial processes are
slow time-variant [5].
Addressing the above problems, the literature [6, 7] gave
a fault diagnosis method based on IIM. This fault diagnosis
method neither has a limi tation that sampling data must be
subject to normal distribution, nor needs extensive historical
data to build model offline. In the case of IIM, the most
interesting side is that sampling data haven’t limi tation of
Gaussian distribution, whereas the method of Q statistic is
looking for the Gaussian distribution. Thus, IIM may reveal
more meaningful information in the non-Gaussian data than
Q statistic. The IIM method de fines and calculates the
covariance matrix based on current and previous time
sampling data, updating covariance matrix when new
observational data is gained. Then covariance matrix of the
current time subtract the covariance matrix of the previous
time, resulting in infor matio n incremental matrix. Finally,
calculating the information incremental mean and dynamic
*
This work is supported by National Natural Science Foundation (NNSF)
of China under Grant 61304109, 61203094, 61174112, 61433001.
threshold based on the infor mation incremental matrix, the
method can determine the system whether there is a failure.
2Faultdia
g
nosis method based on Q statistic
(Algorithm 1 [4])
Consider a process with m variables, let
11 1
22 2
12
11
11
mn
n
mm m
xx xn
xx xn
xx xn
!
#+
#+
(
#+
#+
#+
%,
XR
(1)
Where n is the number of samples. The mean vector of
n
X
is constructed as:
nnn
n
lXb
1
(2)
Where
&
n
n
Rl (
T
1,1,1
.
Q statistic is
T
nn
Q ))xb xb
(3)
Assuming the sampling data subject to normal distribution,
Box [7] proved that the Q distribution can be approximated
as
hgQ
2
~
(4)
Where
)(
2
h
is the chi-squared distribution with h degrees
of freedom and
S
Q
h
)(2
2
(5)
)(2
g
Q
S
(6)
Where
)()()))(((
222
QQQQS
))
.For our
purpose of fault detection,
S
,
)(Q
can be estimated using
Proceedings of the 34th Chinese Control Conference
Jul
y
28-30, 2015, Han
g
zhou, China
6362
资源评论
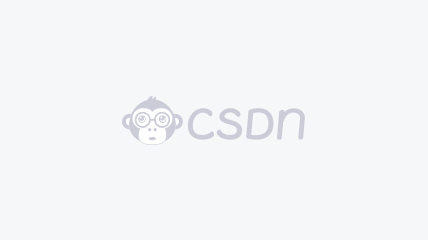

weixin_38709312
- 粉丝: 3
- 资源: 913
上传资源 快速赚钱
我的内容管理 展开
我的资源 快来上传第一个资源
我的收益
登录查看自己的收益我的积分 登录查看自己的积分
我的C币 登录后查看C币余额
我的收藏
我的下载
下载帮助

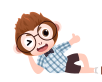
最新资源
- 白色清爽风格的企业网站模板下载.rar
- 白色清新大气的商务企业网站模板下载.rar
- 白色清新风格的HTML5个人简历模板.zip
- 白色清新风格的电商家具商城整站网站源码下载.zip
- 白色清新风格的多用途企业网站模板.rar
- 白色清新风格的工艺品展览馆企业网站模板下载.zip
- 白色清新风格的房地产官网模板下载.zip
- 白色清新风格的工作商务网页CSS模板下载.zip
- 白色清新风格的家具装修设计模板下载.zip
- 白色清新风格的家居公司网站模板下载.zip
- 白色清新风格的陌上花开博客模板下载.zip
- 白色清新风格的家政服务企业网站模板下载.zip
- 白色清新风格的律师法律企业网站模板.zip
- 白色清新风格的皮包设计网站模板下载.zip
- 白色清新风格的甜品网上商城模板.zip
- 白色清新风格的食谱烹饪HTML网站模板.zip
资源上传下载、课程学习等过程中有任何疑问或建议,欢迎提出宝贵意见哦~我们会及时处理!
点击此处反馈


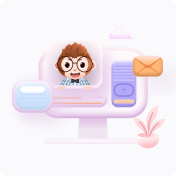
安全验证
文档复制为VIP权益,开通VIP直接复制
