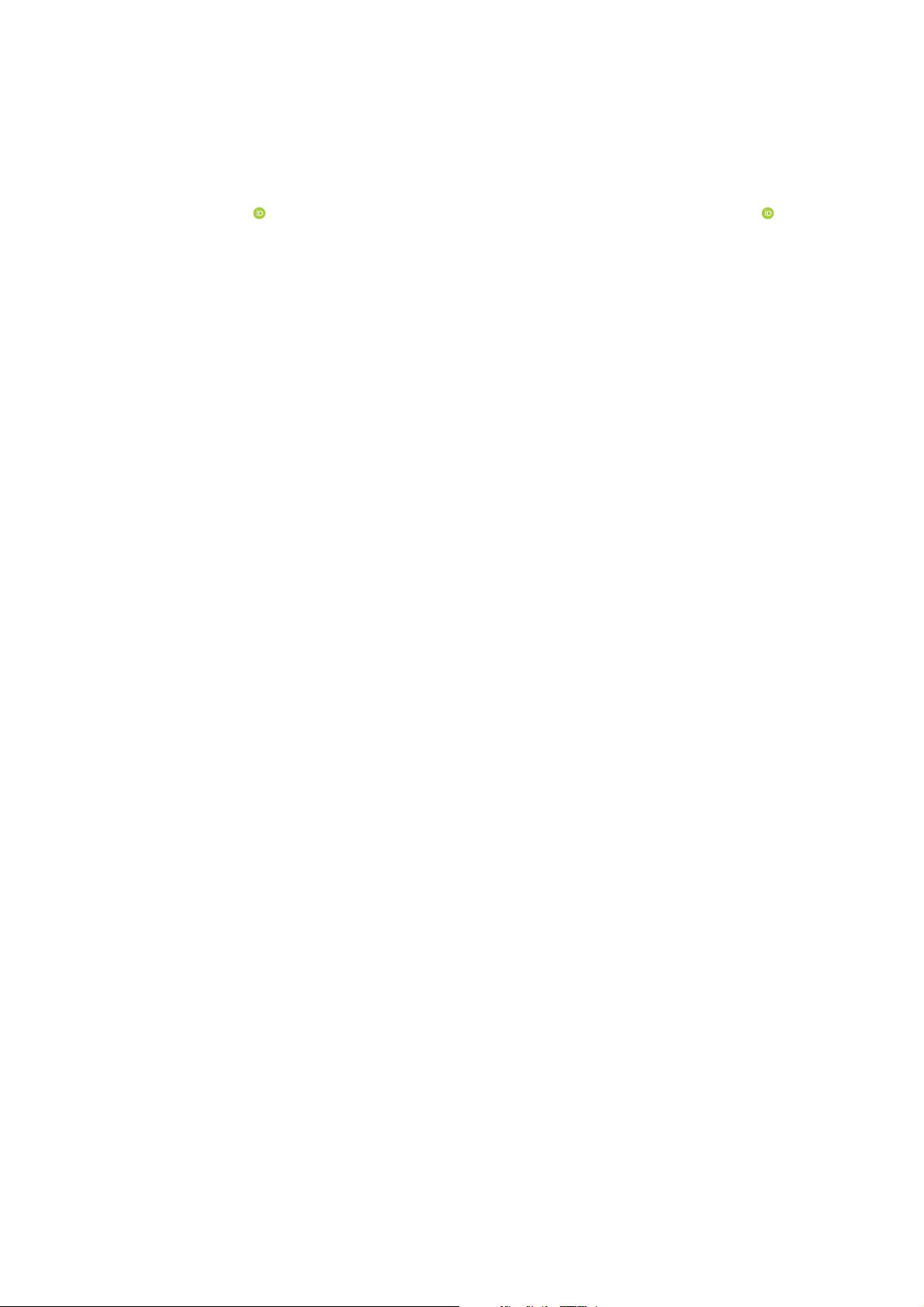
This article has been accepted for inclusion in a future issue of this journal. Content is final as presented, with the exception of pagination.
IEEE TRANSACTIONS ON CONTROL SYSTEMS T ECHNOLOGY 1
Multivariate Statistical Monitoring of Key Operation Units
of Batch Processes Based on Time-Slice CCA
Qingchao Jiang , Member, IEEE, Furong Gao, Member, IEEE, Hui Yi, and Xuefeng Yan
Abstract— A modern batch process can be characterized by a
large scale and multiple operation units, and local fault detection
for the key units of such a batch process is imperative. A time-
slice canonical correlation analysis (CCA)-based multivariate
statistical monitoring scheme f or the key operation units of batch
processes is proposed. First, the three-way batch process data
are unfolded into the time-slice data. Second, CCA modeling is
performed at each time instant to explore the correlation between
the key units and the entire process. Then, a fault detection
residual is generated and monitoring statistics are constructed.
The statistics discriminate both the process status and the type of
a detected fault, a fault relevant or irrelevant to the other units.
The feasibility and superiority of the proposed fault detection
scheme are demonstrated by case studies on a numerical example
and an industrial injection molding process.
Index Terms— Batch processes, canonical correlation analy-
sis (CCA), key operation unit monitoring, multivariate statistical
process monitoring.
I. INTRODUCTION
P
ROCESS monitoring is gaining significant attention in
process control and process system engineering com-
munities [1 ]–[5]. Batch processes play an impo r tant role in
chemical, pharmaceutical, and semiconductor industries, and
multivariate statistical batch process monitoring methods are
of p articular interests [6], [7], in which multiway principal
Manuscript received August 24, 2017; revised November 4, 2017; accepted
February 3, 2018. Manuscript receiv e d in final form February 3, 2018.
This work was supported in part by the National Natural Science Founda-
tion of China under Grant 61603138 and Grant 61503181, in part by the
Guangdong Innova tive and Entrepreneurial Research Team Program under
Grant 2013G076, in part by Fundamental Research Funds for the Central
Uni versities under Grant 222201717006 and Grant 222201714027, in part
by Science Foundation of Jiangsu Pro vince under Grant BK20140953, and
in part by the Program of Introducing Talents of Discipline to Uni versities
(the 111 Project) under Grant B17017. Recommended by Associate Editor
A. Serrani. (Corresponding author: Furong Gao.)
Q. Jiang is with the Department of Chemical and Biomolecular Engineering,
Hong Kong University of Science and Technology, Hong Kong 999077,
and also with the Key Laboratory of Advanced Control and Optimiza-
tion for Chemical Processes of Ministry of Education, East China Uni-
versity of Science and Technology, Shanghai 200237, China (e-mail:
qchjiang@ecust.edu.cn).
F. Gao is with the Department of Chemical and Biomolecular Engineering,
The Hong Kong University of Science and Technology, Hong Kong 999077
(e-mail: kefgao@ust.hk).
H. Yi is with the College of Electronic Engineering and Control Sci-
ence, Nanjing University of Technology, Nanjing 211816, China (e-mail:
jsyihui@126.com).
X. Yan is with the Key Laboratory of Advanced Control and Opti-
mization for Chemical Processes of Ministry of Education, East China
Uni versity of Science and Technology, Shanghai 200237, China (e-mail:
xfyan@ecust.edu.cn).
Color versions of one or more of the figures in this paper are available
online at http://ieeexplore.ieee.org.
Digital Object Identifier 10.1109/TCST.2018.2803071
component analysis and multiway partial least squares are
the basic ones [8], [9]. Many extensions and applications of
the multivariate statistical batch process monitoring methods
have been reported [10]–[13]. However, most of these methods
focus on monitoring the entire plant but not on a specific key
operation unit.
A batch process can be characterized by a large scale and
multiple operation un its and the correlations among the entire
process are complex [14]–[16]. Some operation units play the
key role in guaranteeing process safety or product quality, and
therefore, they are fault sensitive. T hese key operation units
should be monitored emphatically and a fault with even a
small amplitude should be detected timely. However, the local
fault detection issue for a key operation unit has rarely been
discussed. Monitoring a key unit using only the measured
variables from the local unit will ignore the correlation with
the other un its, whereas involving the variables from other
units may cause monitoring redundancy and degrade the
monitoring performance. Efficient fault detection methods, for
a key operation unit in a batch process, are under investigation.
Canonical correlation analysis (CCA) is a basic multivariate
analysis method which has been widely u sed for system identi-
fication as well as process monitoring [1], [17], [18]. Recently,
CCA-based fault detection methods under the residual genera-
tion framework are developed. Chen et al. [19], [20] proposes
a CCA-based fault d etection method, and then improves it
to deal with incipient multiplicative faults. More recently,
Jiang et al. [21] proposes a genetic algorithm-regularized
CCA (GAR-CCA) monitoring method for large-scale multiunit
continuous processes. Although the efficiency is both theo-
retically analyzed and experimentally tested, the monitoring
scheme is designed for continuous processes and assumes
that the co rrelations among the process keep co nstant within
the duration of an operation cycle. Evidently, this assumption
is easily v iolated in batch processes since the correlation
relations may change time to time. In addition, the GAR-CCA-
based fault detection generates an overall monitoring statistic,
without further considering the type of a detected fault.
The main contributions of this brief are summarized as
follows.
1) A time-slice CCA-based local fault detection scheme
for monitoring key operation units of a batch process
is proposed. In a batch process, the correlation rela-
tions among the entire process may change time to
time or phase to phase. Therefore, the three-way batch
process data are unfolded into time-slice data and the
CCA modeling is performed at each time instant to
explore the relation between the local unit and the
1063-6536 © 2018 IEEE. Personal use is permitted, but republication/redistribution requires IEEE permission.
See http://www.ieee.org/publications_standards/publications/rights/index.html for more information.