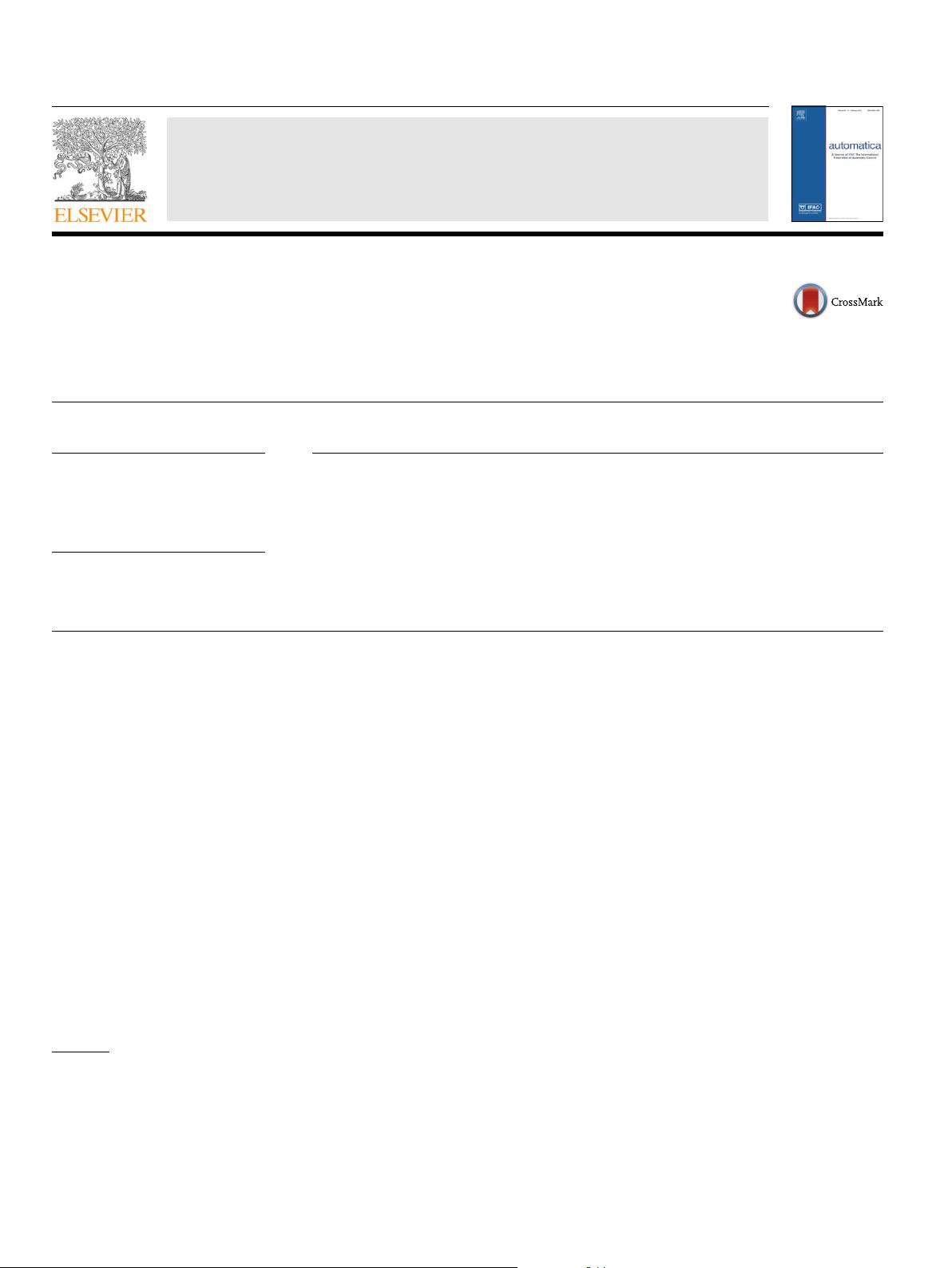
Automatica 71 (2016) 324–333
Contents lists available at ScienceDirect
Automatica
journal homepage: www.elsevier.com/locate/automatica
Brief paper
High-gain-predictor-based output feedback control for time-delay
nonlinear systems
✩
Jing Lei, Hassan K. Khalil
Department of Electrical and Computer Engineering, Michigan State University, East Lansing, MI, 48824, USA
a r t i c l e i n f o
Article history:
Received 25 June 2015
Received in revised form
3 February 2016
Accepted 12 May 2016
Keywords:
Nonlinear systems
Time-delay systems
High-gain observers
Output feedback control
a b s t r a c t
This paper designs a high-gain predictor for output feedback control of nonlinear systems in the presence
of input, output, and state delays. The high-gain predictor realizes the states appearing in the output
feedback control in terms of predictive state, delayed state, and current state. The system includes
internal and external dynamics, and the closed-loop system under state feedback is required to be
asymptotically stable and locally exponentially stable. Positively invariant sets are found to verify
boundedness, exponential stability, and performance recovery. In the simulation, a saturated sliding mode
control is applied to demonstrate the performance recovery of the closed-loop system, and the fact that
the high-gain-predictor parameter has a lower bound related to time delays.
Published by Elsevier Ltd.
1. Introduction
In output feedback control of time-delay systems, state ob-
servers may be used to reconstruct the unmeasured states
(e.g. Germani, Manes, & Pepe, 2012; Germani & Pepe, 2005). But
observer design for nonlinear time-delay systems is challenging.
Germani, Manes, and Pepe (2002) applied the Gronwall lemma
to provide sufficient conditions on delay for a chain observer
to prove exponential convergence of the estimation error. These
conditions were relaxed in Kazantzi and Wright (2005). A Lya-
punov–Krasovskii functional was introduced in Ahmed-Ali, Cher-
rier, and M’Saad (2009) such that a relationship between the delay
and the number of cascade observers with specific vector gains was
proposed. Many authors employed this method in the later work
(e.g. Ahmed-Ali, Cherrier, & Lamnabhi-Lagarrigue, 2012; Ahmed-
Ali, Van Assche, Massieu, & Dorleans, 2013; Farza, Sboui, Cherrier, &
M’Saad, 2010; Ghanes, Leon, & Barbot, 2013). The aforementioned
papers are devoted to proving the exponential convergence of the
observer estimation errors. Recently, Karafyllis, Krstic, Ahmed-Ali,
and Lamnabhi-Lagarrigue (2014) proved exponential stability of
the closed-loop system in a disturbance free case. Those techniques
✩
This work was supported in part by the National Science Foundation (US) (ECCS-
1128476) and by the National Natural Science Foundation of China (61364012).
The material in this paper was partially presented at the 2015 American Control
Conference, July 1–3, 2015, Chicago, IL, USA. This paper was recommended for
publication in revised form by Associate Editor Fouad Giri under the direction of
Editor Miroslav Krstic.
E-mail addresses: elizabethia@126.com (J. Lei), khalil@msu.edu (H.K. Khalil).
for predictors (e.g., Germani et al., 2002; Karafyllis et al., 2014),
work with time delays that are not necessarily small; however,
they require exact models of the system. An advantage of high-gain
observers is that we can dominate uncertain nonlinearities, while
the price for that is restricting the delay to be small. Another ad-
vantage of using high-gain observers is that they can recover the
performance of state feedback control.
In our previous work Lei and Khalil (2016), we used a high-gain
predictor in feedback-linearization control. The system considered
is nonlinear with time-varying input and output delays. To verify
the performance recovery, we constructed a Lyapunov–Krasovskii
functional, proved boundedness and exponential stability of the
closed-loop system, and found a lower bound on the high-gain pa-
rameter, which relates to the maximum of involved delays. This
work is an extension of Lei and Khalil (2016), which does the fol-
lowing: (1) we allow zero dynamics; (2) instead of feedback lin-
earization, we allow any stabilizing state feedback control; (3) we
allow state delay in addition to the input and output delays.
Notations. Throughout the paper,
|
·
|
means the absolute
value; I denotes the identity matrix; λ denotes the eigenvalues,
λ
min
(·) and λ
max
(·) are the minimum and maximum eigenvalues
of the matrix respectively; the superscript of ‘‘T’’ stands for the
transposition of a matrix; R
n
denotes the n-dimensional Euclidean
space and
∥
·
∥
denotes the Euclidean norm; R
+
represents the set
of non-negative real numbers; given a positive constant r ∈ R
+
,
L
2
(
[−r, 0]; R
n
)
denotes the space of square integrable functions
φ : [−r, 0] → R
n
; C
n
denotes the space of absolutely continuous
functions φ : [−r, 0] → R
n
, which have square integrable first-
order derivatives; x : [−r, ∞) → R
n
is a function; x(t) means the
http://dx.doi.org/10.1016/j.automatica.2016.05.026
0005-1098/Published by Elsevier Ltd.