# Overview
MobileNet-v2 is a convolutional neural network that has been trained on a subset of the ImageNet database. As a result, the network has learned rich feature representations for a wide range of images. The network can classify images into 1000 object categories, such as keyboard, mouse, pencil, and many animals. The network has been designed to have a low memory footprint, making it ideal for deployment to low-memory hardware.
The network has an image input size of 224-by-224-by-3.
# Usage
This repository requires [MATLAB](https://www.mathworks.com/products/matlab.html) (R2019a and above) and the [Deep Learning Toolbox](https://www.mathworks.com/products/deep-learning.html).
This repository provides three functions:
- mobilenetv2Layers: Creates an untrained network with the network architecture of MobileNet-v2
- assembleMobileNetv2: Creates a MobileNet-v2 network with weights trained on ImageNet data
- mobilenetv2Example: Demonstrates how to classify an image using a trained MobileNet-v2 network
To construct an untrained MobileNet-v2 network to train from scratch, type the following at the MATLAB command line:
```matlab
lgraph = mobilenetv2Layers;
```
The untrained network is returned as a `layerGraph` object.
To construct a trained MobileNet-v2 network suitable for use in image classification, type the following at the MATLAB command line:
```matlab
net = assembleMobileNetv2;
```
The trained network is returned as a `DAGNetwork` object.
To classify an image with the network:
```matlab
img = imresize(imread("peppers.png"),[224 224]);
predLabel = classify(net, img);
imshow(img);
title(string(predLabel));
```
# Documentation
For more information about the MobileNet-v2 pre-trained model, see the [mobilenetv2](https://www.mathworks.com/help/deeplearning/ref/mobilenetv2.html) function page in the [MATLAB Deep Learning Toolbox documentation](https://www.mathworks.com/help/deeplearning/index.html).
# Architecture
MobileNet-v2 is a residual network. A residual network is a type of DAG network that has residual (or shortcut) connections that bypass the main network layers. Residual connections enable the parameter gradients to propagate more easily from the output layer to the earlier layers of the network, which makes it possible to train deeper networks. This increased network depth can result in higher accuracies on more difficult tasks.
You can explore and edit the network architecture using [Deep Network Designer](https://www.mathworks.com/help/deeplearning/ug/build-networks-with-deep-network-designer.html).

# MobileNet-v2 in MATLAB
This repository demonstrates the construction of a residual deep neural network from scratch in MATLAB. You can use the code in this repository as a foundation for building residual networks with different numbers of residual blocks.
You can also create a trained MobileNet-v2 network from inside MATLAB by installing the Deep Learning Toolbox Model for MobileNet-v2 Network support package. Type `mobilenetv2` at the command line. If the Deep Learning Toolbox Model for MobileNet-v2 Network support package is not installed, then the function provides a link to the required support package in the Add-On Explorer. To install the support package, click the link, and then click Install.
Alternatively, you can download the MobileNet-v2 pre-trained model from the MathWorks File Exchange, at [Deep Learning Toolbox Model for MobileNet-v2 Network](https://www.mathworks.com/matlabcentral/fileexchange/70986-deep-learning-toolbox-model-for-mobilenet-v2-network).
You can create an untrained MobileNet-v2 network from inside MATLAB by importing a trained MobileNet-v2 network into the Deep Network Designer App and selecting Export > Generate Code. The exported code will generate an untrained network with the network architecture of MobileNet-v2.

AI拉呱
- 粉丝: 2861
- 资源: 5510
最新资源
- python爬虫实战开发之bs4应用和xpath结合实战操作.zip
- 电子课程设计项目《多功能数字时钟(包括了基本的计数显示,还有提高部分,如星期和月份的动态展示)》+项目源码+文档说明
- C#大型OA源码 网络在线办公平台源码数据库 SQL2008源码类型 WebForm
- RV1106编译速度、驱动加载
- tensorflow安装-不同操作系统环境下TensorFlow的安装指南与步骤
- 谷歌浏览器网页自动刷新插件
- 黑龙江大学数据库实验三、四,模拟数据库系统
- c语言中字符串的常用操作:搜索字符串的结尾、复制字符串
- 海克斯康三坐标输出Excel报告文件定制脚本
- html css网页制作成品-HTML与CSS配合制作的静态网站基本布局实例
资源上传下载、课程学习等过程中有任何疑问或建议,欢迎提出宝贵意见哦~我们会及时处理!
点击此处反馈


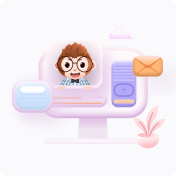