# Overview
ResNet-50 is a convolutional neural network that is trained on more than a million images from the ImageNet database. As a result, the network has learned rich feature representations for a wide range of images. The network can classify images into 1000 object categories, such as keyboard, mouse, pencil, and many animals.
The network has an image input size of 224-by-224-by-3.
# Usage
This repository requires [MATLAB](https://www.mathworks.com/products/matlab.html) (R2018b and above) and the [Deep Learning Toolbox](https://www.mathworks.com/products/deep-learning.html).
This repository provides three functions:
- resnet50Layers: Creates an untrained network with the network architecture of ResNet-50
- assembleResNet50: Creates a ResNet-50 network with weights trained on ImageNet data
- resnet50Example: Demonstrates how to classify an image using a trained ResNet-50 network
To construct an untrained ResNet-50 network to train from scratch, type the following at the MATLAB command line:
```matlab
lgraph = resnet50Layers;
```
The untrained network is returned as a `layerGraph` object.
To construct a trained ResNet-50 network suitable for use in image classification, type the following at the MATLAB command line:
```matlab
net = assembleResNet50;
```
The trained network is returned as a `DAGNetwork` object.
To classify an image with the network:
```matlab
img = imresize(imread("peppers.png"),[224 224]);
predLabel = classify(net, img);
imshow(img);
title(string(predLabel));
```
# Documentation
For more information about the ResNet-50 pre-trained model, see the [resnet50](https://www.mathworks.com/help/deeplearning/ref/resnet50.html) function page in the [MATLAB Deep Learning Toolbox documentation](https://www.mathworks.com/help/deeplearning/index.html).
# Architecture
ResNet-50 is a residual network. A residual network is a type of DAG network that has residual (or shortcut) connections that bypass the main network layers. Residual connections enable the parameter gradients to propagate more easily from the output layer to the earlier layers of the network, which makes it possible to train deeper networks. This increased network depth can result in higher accuracies on more difficult tasks.
The network is 50 layers deep. The network depth is defined as the largest number of sequential convolutional or fully connected layers on a path from the input layer to the output layer. In total, ResNet-50 has 177 layers.
You can explore and edit the network architecture using [Deep Network Designer](https://www.mathworks.com/help/deeplearning/ug/build-networks-with-deep-network-designer.html).

# ResNet-50 in MATLAB
This repository demonstrates the construction of a residual deep neural network from scratch in MATLAB. You can use the code in this repository as a foundation for building residual networks with different numbers of residual blocks.
You can also create a trained ResNet-50 network from inside MATLAB by installing the Deep Learning Toolbox Model for ResNet-50 Network support package. Type `resnet50` at the command line. If the Deep Learning Toolbox Model for ResNet-50 Network support package is not installed, then the function provides a link to the required support package in the Add-On Explorer. To install the support package, click the link, and then click Install.
Alternatively, you can download the ResNet-50 pre-trained model from the MathWorks File Exchange, at [Deep Learning Toolbox Model for ResNet-50 Network](https://www.mathworks.com/matlabcentral/fileexchange/64626-deep-learning-toolbox-model-for-resnet-50-network).
You can create an untrained ResNet-50 network from inside MATLAB by importing a trained ResNet-50 network into the Deep Network Designer App and selecting Export > Generate Code. The exported code will generate an untrained network with the network architecture of ResNet-50.
没有合适的资源?快使用搜索试试~ 我知道了~
资源推荐
资源详情
资源评论
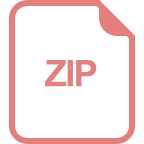
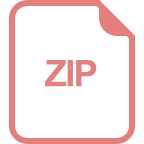
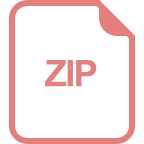
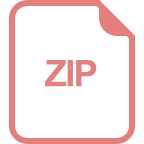
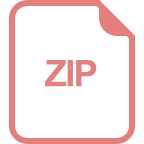
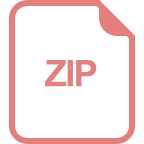
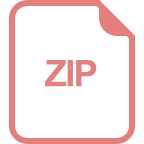
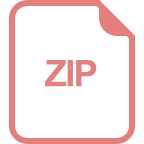
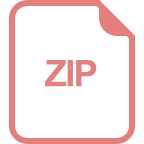
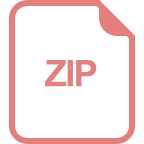
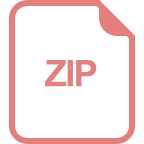
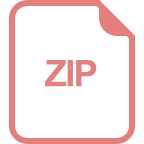
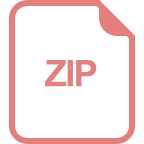
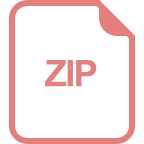
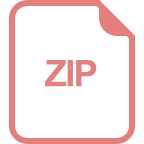
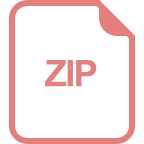
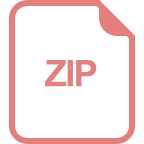
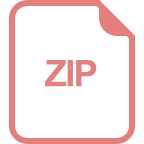
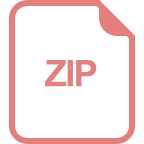
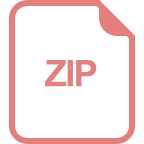
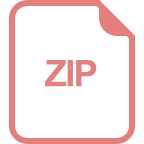
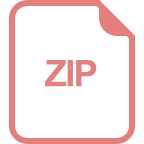
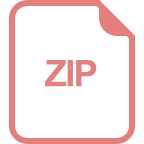
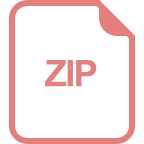
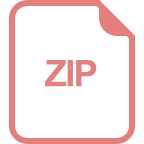
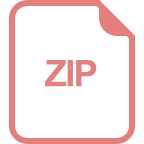
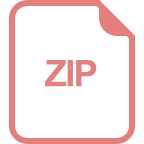
收起资源包目录

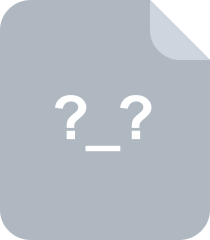
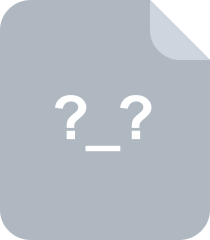
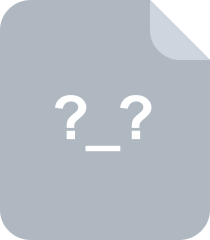
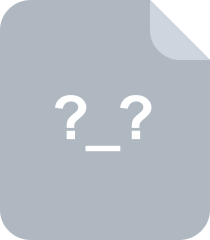

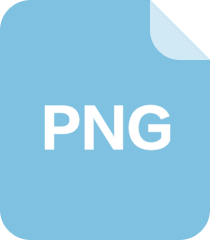
共 5 条
- 1
资源评论
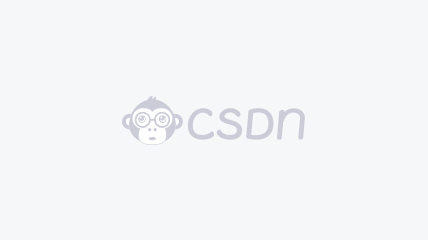

AI拉呱
- 粉丝: 2866
- 资源: 5510
上传资源 快速赚钱
我的内容管理 展开
我的资源 快来上传第一个资源
我的收益
登录查看自己的收益我的积分 登录查看自己的积分
我的C币 登录后查看C币余额
我的收藏
我的下载
下载帮助

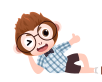
最新资源
资源上传下载、课程学习等过程中有任何疑问或建议,欢迎提出宝贵意见哦~我们会及时处理!
点击此处反馈


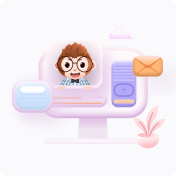
安全验证
文档复制为VIP权益,开通VIP直接复制
