没有合适的资源?快使用搜索试试~ 我知道了~
温馨提示
已经提出了许多用于红外小目标检测的最新技术。 它们在具有均一背景和高对比度目标的图像上效果很好。 然而,当面对高度异质的背景时,它们将不能很好地工作,主要是由于:1)存在强边缘和其他干扰组件,2)没有充分利用先验条件。 受此启发,我们提出了一种同时利用本地先验和非本地先验的新颖方法。 首先,我们采用新的红外补丁张量(IPT)模型来表示图像并保留其空间相关性。 利用目标稀疏先验和背景非局部自相关先验,将目标背景分离建模为鲁棒的低秩张量恢复问题。此外,借助结构张量和权重思想,我们设计了一种局部局部自适应和稀疏性增强权重来代替全局常数加权参数。 根据目标检测的实际情况,可以通过元素重加权的高阶稳健主成分分析以及附加的收敛条件来实现分解。广泛的实验表明,我们的模型优于其他最新技术,特别是对于图像质量非常高的图像昏暗的目标和沉重的杂物。
资源推荐
资源详情
资源评论
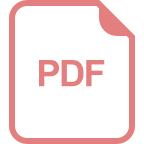
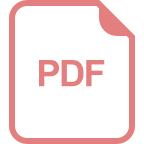
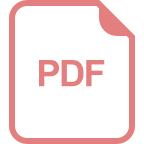
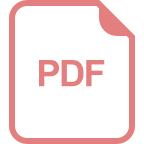
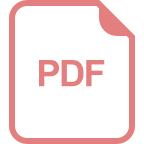
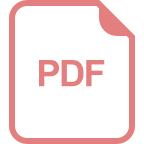
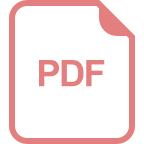
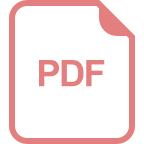
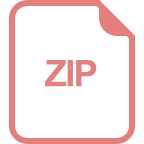
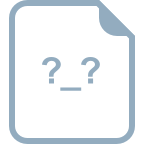
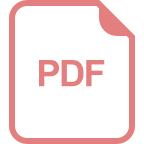
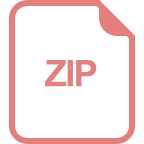
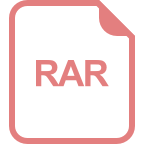
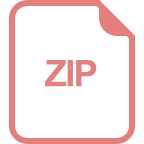
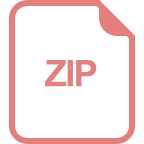
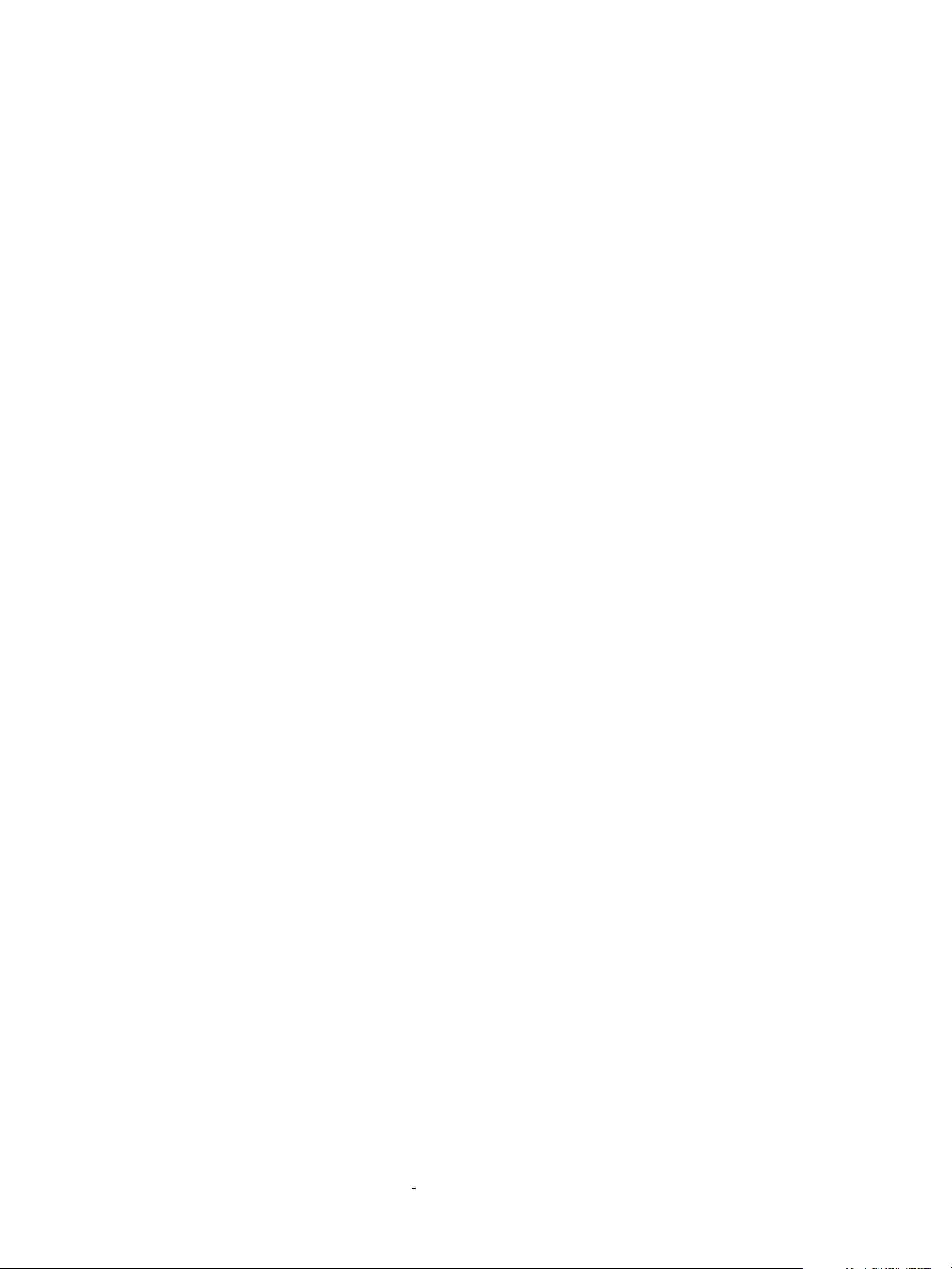
3752 IEEE JOURNAL OF SELECTED TOPICS IN APPLIED EARTH OBSERVATIONS AND REMOTE SENSING, VOL. 10, NO. 8, AUGUST 2017
Reweighted Infrared Patch-Tensor Model With Both
Nonlocal and Local Priors for Single-Frame
Small Target Detection
Yimian Dai and Yiquan Wu
Abstract—Many state-of-the-art methods have been proposed
for infrared small target detection. They work well on the images
with homogeneous backgrounds and high-contrast targets. How-
ever, when facing highly heterogeneous backgrounds, they would
not perform very well, mainly due to: 1) the existence of strong
edges and other interfering components, 2) not utilizing the priors
fully. Inspired by this, we propose a novel method to exploit both
local and nonlocal priors simultaneously. First, we employ a new
infrared patch-tensor (IPT) model to represent the image and pre-
serve its spatial correlations. Exploiting the target sparse prior and
background nonlocal self-correlation prior, the target-background
separation is modeled as a robust low-rank tensor recovery prob-
lem. Moreover, with the help of the structure tensor and reweighted
idea, we design an entrywise local-structure-adaptive and sparsity
enhancing weight to replace the globally constant weighting pa-
rameter. The decomposition could be achieved via the elementwise
reweighted higher order robust principal component analysis with
an additional convergence condition according to the practical sit-
uation of target detection. Extensive experiments demonstrate that
our model outperforms the other state-of-the-arts, in particular for
the images with very dim targets and heavy clutters.
Index Terms—Infrared patch-tensor model, infrared small tar-
get detection, local structure prior, nonlocal self-correlation prior,
reweighted higher order robust principal component analysis.
I. INTRODUCTION
I
NFRARED small target detection is a key technique for
many applications, including early-warning system, preci-
sion guided weapon, missile tracking system, and maritime
surveillance system [1]–[3]. Traditional sequential detection
methods, such as three-dimensional (3-D) matched filter [4], im-
proved 3-D filter [5], and multiscan adaptive matched filter [6],
Manuscript received December 16, 2016; revised March 27, 2017; accepted
April 20, 2017. Date of publication May 22, 2017; date of current version August
9, 2017. This work was supported in part by the National Natural Science
Foundation of China under Grant 61573183, and in part by Open Research
Fund of Key Laboratory of Spectral Imaging Technology, Chinese Academy of
Sciences under Grant LSIT201401. (Corresponding author: Yiquan Wu).
Y. Dai is with the College of Electronic and Information Engineering, Nanjing
University of Aeronautics and Astronautics, Nanjing 211106, China (e-mail:
yimian.dai@gmail.com).
Y. Wu is with the College of Electronic and Information Engineering, Nanjing
University of Aeronautics and Astronautics, Nanjing 211106, China, and also
with Key Laboratory of Spectral Imaging Technology CAS, Xi’an Institute
of Optics and Precision Mechanics of CAS, Xi’an 710000, China (e-mail:
nuaaimagestrong@163.com).
Color versions of one or more of the figures in this paper are available online
at http://ieeexplore.ieee.org.
Digital Object Identifier 10.1109/JSTARS.2017.2700023
are workable in the case of static background, exploiting the tar-
get spatial-temporal information. Nevertheless, with the recent
fast development of high-speed aircrafts [7] like antiship mis-
siles, the imaging backgrounds generally change quickly due to
rapid relative motion between the imaging sensor and the target.
The performance of the spatial-temporal detection method de-
grades rapidly. Therefore, the research of single-frame infrared
small target detection is of great importance and has attracted a
lot of attention in recent years.
Different from general object or saliency detection tasks,
the main challenge of infrared small target detection is lack-
ing enough information. Due to the long imaging distance, the
target is always small without any other texture or shape fea-
tures. As the target type, imaging distance, and neighboring
environment differ a lot in real scenes, the target brightness
could vary from extremely dim to very bright (see Fig. 5, for
example). In the absence of spatial-temporal information and
the target features like shape and size, the characteristics of the
background [8] and the relation between the background and
target are very important priors for single-frame infrared small
target detection. Thus, how to design a model to incorporate and
exploit these priors is vital for infrared small target detection in
a single image.
A. Prior Work on Single-Frame Infrared Small Target
Detection
The previously proposed single-frame infrared small target
detection methods could be roughly classified into two cat-
egories. In the first type, a local background consistent prior
is exploited, assuming the background is slowly transitional
and nearby pixels are highly correlated. As a result, the target
is viewed as the one that breaks this local correlation. Under
this assumption, the classical methods, such as 2-D least mean
square (TDLMS) filter [9] and Max-Median filter [10], enhance
the small target by subtracting the predicted background from
the original image. Unfortunately, besides the targets, they
enhance the edges of the sky-sea surface or heavy cloud clutter
as well, since these structures also break the background
consistency as the target does. To differentiate the real target
and high-frequency change, some edge analysis approaches
[11], [12] have been proposed to extend these methods to
estimate the edge direction in advance and preserve the edges.
Bai et al. [13] designed a new Top-Hat transformation using
1939-1404 © 2017 IEEE. Personal use is permitted, but republication/redistribution requires IEEE permission.
See http://www.ieee.org/publications
standards/publications/rights/index.html for more information.
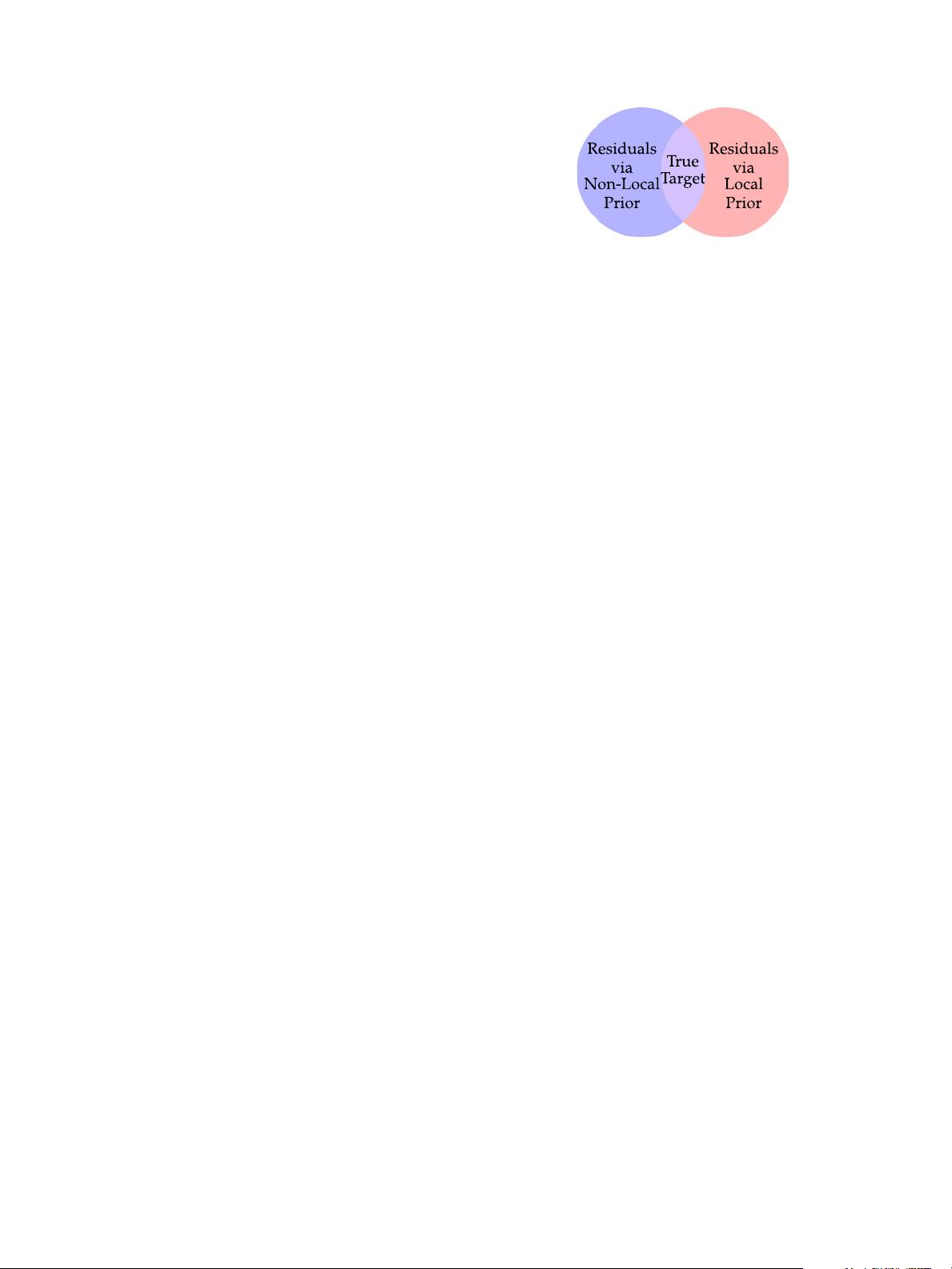
DAI AND WU: REWEIGHTED INFRARED PATCH-TENSOR MODEL WITH BOTH NONLOCAL AND LOCAL PRIORS 3753
two different but correlated structuring elements. Another class
of local prior-based methods exploits the local contrast, which
is computed by comparing a pixel or a region only with its
neighbors. The seminal work of Laplacian of Gaussian (LoG)
filter-based method [14] has motivated a broad range of studies
on the Human Visual System (HVS), and has led to a series of
HVS-based methods, e.g., difference of Gaussians (DoG) [15],
second-order directional derivative (SODD) filter [16], local
contrast measure (LCM) [17], improved local contrast measure
(ILCM) [18], multiscale patch-based contrast measure (MPCM)
[19], multiscale gray difference weighted image entropy [20],
improved difference of Gabors (IDoGb) [21], local saliency
map (LSM) [22], weighted local difference measure (WLDM)
[23], local difference measure (LDM) [24], etc.
The second type of single-frame infrared small target detec-
tion methods which has not been explored extensively, exploits
the nonlocal self-correlation property of background patches,
assuming that all background patches come f rom a single
subspace or a mixture of low-rank subspace clusters. Then,
target-background separation can be realized with the low-rank
matrix recovery [25]. Essentially, this type of methods attempts
to model the infrared small target as an outlier in the input
data. To this end, Gao et al. [26] generalized the traditional
infrared image model to a new infrared patch-image model via
local patch construction. Then, the target-background separation
problem is reformulated as a robust principal component anal-
ysis (RPCA) [27] problem of recovering low-rank and sparse
matrices, achieving a state-of-the-art background suppressing
performance. To correctly detect the small target located in a
highly heterogeneous background, He et al. [28] proposed a low-
rank and sparse representation model under the multisubspace-
cluster assumption.
B. Motivation
Existing methods detect the infrared small target by either uti-
lizing the local pixel correlation or exploiting the nonlocal patch
self-correlation. From our observation, the unsatisfying perfor-
mance of local prior methods [20], [23] in detecting the dim
target under complicated background mainly lies in their imper-
fect grayscale-based center-difference measures. The saliency
of a dim but true target would be easily overwhelmed by the mea-
sured saliency of some rare structures. We call this phenomenon
the rare structure effect. In contrast, the nonlocal prior meth-
ods [26], [29], [30] suffer from the salient edge residuals. Its
intrinsic reason is because the strong edge is also a sparse com-
ponent as the s ame as the target due to lack of sufficient similar
samples. Since the target might be dimmer than the edge, they
would simultaneously be wiped out by simply increasing the
threshold.
Our key observation is that the nonlocal prior and local prior
are not equivalent, and in fact they are complementary for the
problem of infrared small target detection, as illustrated in Fig. 1.
The often appearing false alarm components in local (nonlocal)
prior methods could be well suppressed by the nonlocal (local)
prior methods. For example, the stubborn strong edges in the
nonlocal prior-based methods can be easily identified by the
Fig. 1. Illustration of our motivation behind exploiting both nonlocal and local
priors.
local edge analysis approaches. Naturally, an intuitive way to
solve abovementioned dilemma is to extract the local structure
information and merge it into the nonlocal prior-based detection
framework. Therefore, how to simultaneously and appropriately
utilize both the local and nonlocal priors has been an impor-
tant issue for improving the detection performance under very
complex backgrounds. To the best of our knowledge, very few
single-frame infrared small target detection methods concern
this problem.
To address this issue, we propose a single-frame small tar-
get detection framework with reweighted infrared patch-tensor
model (RIPT). Our main contributions consist of the following
three folds:
1) To dig out more information from the nonlocal self-
correlationship in patch space, we generalize the patch-
image model to a novel infrared patch-tensor model (IPT)
and formulate the target-background separation task as an
optimization problem of recovering low-rank and sparse
tensors.
2) To incorporate the local structure prior into the IPT model,
an elementwise weight is designed based on structure
tensor, which helps us to suppress the remaining edges
and preserve the dim target simultaneously.
3) To reduce the computing time, we adopt a reweighted
scheme to enhance the sparsity of the target patch-tensor.
Considering the particularity of infrared small target de-
tection, an additional stopping criterion is used to avoid
excessive computation.
The proposed RIPT model is validated on different real
infrared image datasets. Compared with the state-of-the-art
methods, our proposed model achieves a better background sup-
pressing and target detection performance.
The remaining of this paper is organized as follows. We pro-
pose the nonlocal correlation-based IPT model in Section II.
The details of the local structure weight construction are de-
scribed in Section III. In Section IV, we further propose the
reweighted IPT model and its detailed optimization scheme is
also provided. Section V presents detailed experimental results
and discussion. Finally we conclude this paper in Section VI.
II. N
ONLOCAL CORRELATION DRIVEN INFRARED
PAT CH-TENSOR MODEL
To dig out more spatial correlationships, we develop a
novel target-background separation framework named infrared
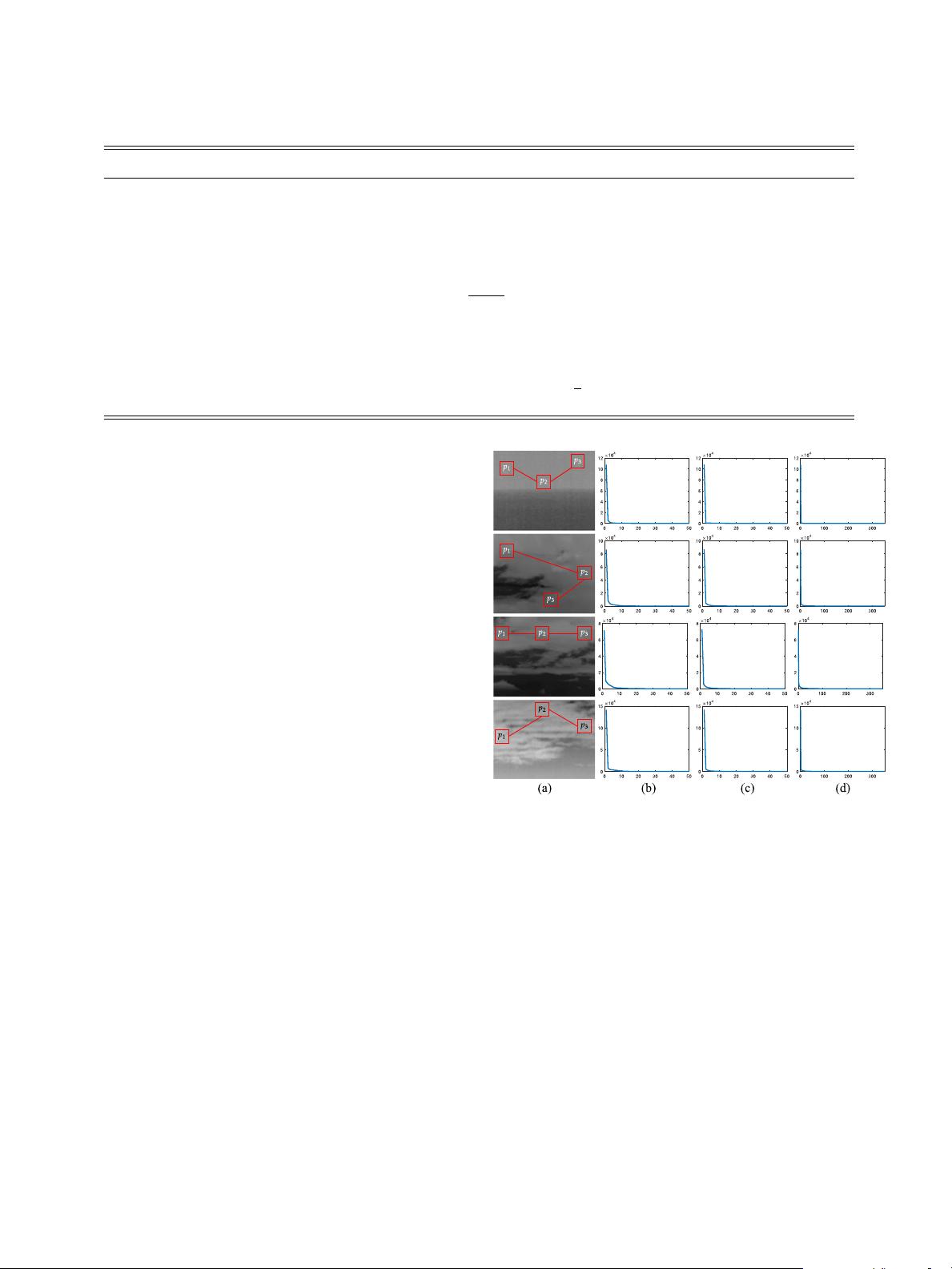
3754 IEEE JOURNAL OF SELECTED TOPICS IN APPLIED EARTH OBSERVATIONS AND REMOTE SENSING, VOL. 10, NO. 8, AUGUST 2017
TABL E I
M
ATHEMATICAL NOTATIONS
Notation Explanation
X , X, x,x tensor, matrix, vector, scalar.
X
( n )
mode-n matricization of tensor X ∈ R
I
1
×I
2
×···×I
N
, obtained by arranging the mode-n fibers as the columns of the resulting matrix of size
R
I
n
×
k = n
I
k
.
vec (X ) vectorization of tensor X .
X , Y inner product of tensor X and Y, which is defined as X , Y := vec(X )
vec(Y).
X
0
0
norm of tensor X which counts the number of non-zero elements.
X
1
1
norm of tensor X .
X
F
Frobenius norm of tensor X , which is defined by X
F
:=
X , X .
fold
i
(X) returns tensor Z that Z
( i )
= X.
X
∗
nuclear norm of matrix X, which is defined by X
∗
=
i
σ
i
, where the SVD of X = U diag(σ)V
.
S
μ
(x) element-wise shrinkage operator is defined as S
μ
(x):=sign(x)max(|x|−μ, 0). S
μ
(x) is the closed-form solution of the problem:
ˆ
y =argmin
y
x − y
2
F
+ λy
1
[31].
D
μ
(X) matrix singular value thresholding operator: D
μ
(X):=U diag(¯σ )V
, where X = U diag(σ)V
is the SVD of X and ¯σ := m a x( σ − μ,0).
D
μ
(X) is the closed-form solution of the problem:
ˆ
Y =argmin
Y
X −Y
2
F
+
μ
2
Y
∗
[32].
T
i,μ
(X ) T
i,μ
(X ):=fold
i
(D
μ
(X
( i )
)).
patch-tensor model in this section. Before describing the details,
several mathematical notations are defined first in Table I.
Given an infrared image, it could be modeled as a linear
superposition of target image, background image, and noise
image:
f
F
= f
B
+ f
T
+ f
N
(1)
where f
F
, f
B
, f
T
, and f
N
represent the input image, back-
ground image, target image, and noise image, respectively. Via
a window sliding from the top left to the bottom right over an
image, we stack the patches into a 3-D cube (see the construc-
tion step in Fig. 4). Then, (1) is transferred to the patch space
with spatial structure preserved:
F = B + T + N (2)
where F, B, T , N ∈ R
I ×J ×P
are the input patch-tensor, back-
ground patch-tensor, and target patch-tensor, respectively. I and
J are the patch height and width, P is the patch number.
Background patch-tensor B: Generally, the background is
considered as slowly transitional, which implies high correla-
tions among both the local and nonlocal patches in the image, as
illustrated in Fig. 2(a). Although patches p
1
,p
2
,p
3
locate in the
different region of the image, they are equivalent. Based on this
nonlocal correlationship, the state-of-the-art IPI model imposed
the low-rank constraint to background patch-image. As a patch-
image is the mode-3 unfolding matrix of a patch-tensor, the
patch-image model could be viewed as a special case of the pro-
posed patch-tensor model essentially. Since the main difficulty
of detecting the infrared small target in a single image is lacking
enough information, only considering the low-rank structure in
one unfolding is insufficient to deal with highly difficult scenes.
Naturally, it motivates us to think whether we can utilize the
other two unfolding modes. Actually, the mode-1 and mode-2
unfolding matrices of the infrared patch-tensor are also low-
rank. In Fig. 2(b)–(d), the singular values of all the unfolding
matrices rapidly decrease to zero, which demonstrates that every
unfolding mode of the background patch-tensor is intrinsically
low-rank. Therefore, we can consider the background patch-
tensor B as a low-rank tensor, and their unfolding matrices are
Fig. 2. Illustration of the nonlocal similarity and the low-rank property of
the mode-i (i =1, 2, 3) unfolding of the patch-tensor. (a) Four representative
background images. (b)–(d) Singular values of the mode-1, mode-2, and mode-3
unfolding matrices of the corresponding background patch-tensors.
also all low-rank defined as:
rank(B
(1)
) ≤ r
1
, rank(B
(2)
) ≤ r
2
, rank(B
(3)
) ≤ r
3
(3)
where r
1
, r
2
, and r
3
all are constants, representing the com-
plexity of the background image. The larger their values are, the
more complex the background is.
Target patch-tensor T : Since the small target merely occupies
several pixels in the whole image, the target patch-tensor is an
extremely sparse tensor in fact. That is:
T
0
≤ k (4)
where k is a small integer determined by the number and size
of the small target.
剩余15页未读,继续阅读
资源评论
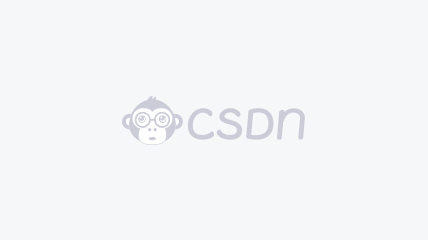

weixin_38660295
- 粉丝: 6
- 资源: 910
上传资源 快速赚钱
我的内容管理 展开
我的资源 快来上传第一个资源
我的收益
登录查看自己的收益我的积分 登录查看自己的积分
我的C币 登录后查看C币余额
我的收藏
我的下载
下载帮助

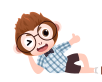
最新资源
- 基于AI的Python爬虫.zip
- opencv4.5.4 centos7相关资源,包含libopencv-java454.so、opencv-4.5.4.tar.gz、opencv-454.jar
- 基于 Backtrader 的量化示例 .zip
- 在虚幻引擎 4 中嵌入 Python.zip
- 在接下来的 30 天左右学习 Python .zip
- C++练习案例(类和对象):多态实现职工管理系统 包含源码和项目压缩包
- FASTJSON2 是一个性能卓越的 Java JSON 库
- vmware-VMnet8一键启动和停止脚本
- 在 PyMYSQL 上构建 MySQL 复制协议的纯 Python 实现.zip
- 在 Google Cloud Platform 上使用 Python 的代码示例.zip
资源上传下载、课程学习等过程中有任何疑问或建议,欢迎提出宝贵意见哦~我们会及时处理!
点击此处反馈


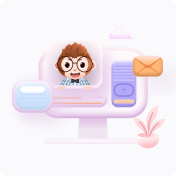
安全验证
文档复制为VIP权益,开通VIP直接复制
