没有合适的资源?快使用搜索试试~ 我知道了~
基于椭圆估计误差界的动态输出反馈鲁棒模型预测控制
1 下载量 106 浏览量
2021-03-18
04:09:17
上传
评论
收藏 319KB PDF 举报
温馨提示
本文提出了一种具有多变量不确定性和有界干扰的准LPV(准线性参数变化)系统的动态输出反馈鲁棒模型预测控制(DOFRMPC)。我们以前的研究表明,更新估计误差集(EES)是提高控制性能的关键旋钮。在我们先前的工作中,为了计算EES,使用了多面体集的递归,它还涉及椭球体的多面体外逼近和多面体集的外椭圆体逼近。通过应用S-过程,本文提出了一种新的刷新EES的方法,该方法直接利用了椭球EES的递归。数值例子说明了该方法的有效性。
资源推荐
资源详情
资源评论
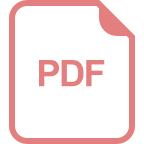
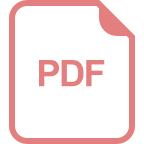
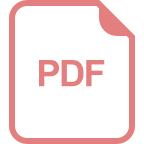
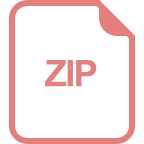
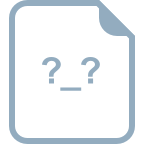
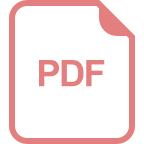
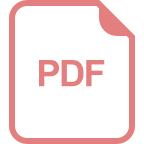
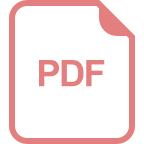
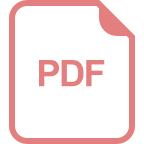
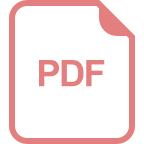
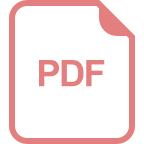
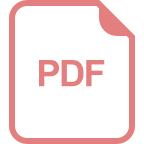
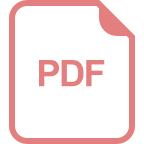
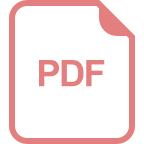
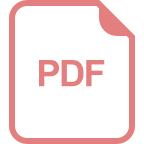
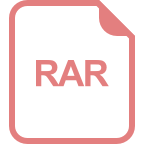
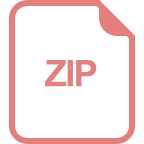
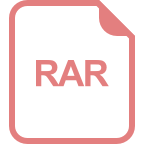
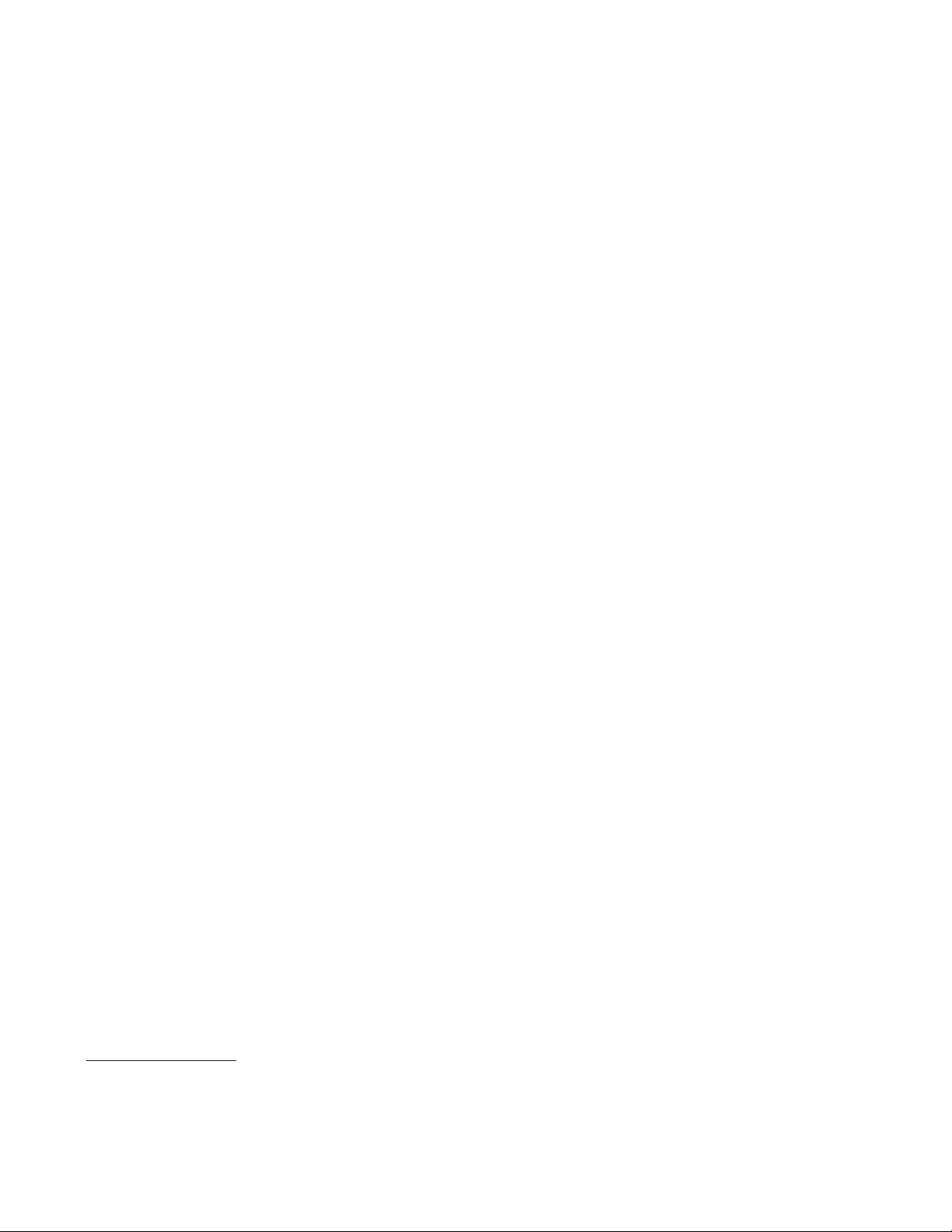
Dynamic output feedback robust model predictive control based on
ellipsoidal estimation error bound for quasi-LPV systems
Xubin Ping, Baocang Ding
∗
Ministry of Education Key Lab For Intelligent Networks and Network Security (MOE KLINNS Lab), Department of Automation,
School of Electronic and Information Engineering, Xi’an Jiaotong University, Xi’an, 710049, P.R. China
E-mail: baocang.ding@gmail.com
Abstract: This paper presents a dynamic output feedback robust model predictive control (DOFRMPC) for the quasi-
LPV (quasi-linear parameter varying) systems with both polytopic uncertainty and bounded disturbance. Our previous
studies have shown that the refreshment of the estimation error set (EES) is a key knob for improving the control perfor-
mance. In our previous work, for calculating the EES, the recursion of polyhedral set is utilized, which also involves the
polyhedral outer approximation of the ellipsoid and the outer ellipsoidal approximation of polyhedral set. By applying
S-procedure, a new method for refreshing EES is proposed in this paper which directly utilizes the recursion of ellipsoidal
EES. A numerical example is given to illustrate the effectiveness of the approach.
Key Words: Dynamic output feedback, model predictive control, quasi-linear parameter varying systems, estimation
error set
1 INTRODUCTION
The model predictive control (MPC), also known as the
receding horizon control, is a class of optimization based
control method, which explicitly utilizes the process model
and measurements to optimize a performance function. At
each time, the optimization is carried out, and the control
input sequence generated. However, only the first element
in this sequence is implemented. Due to its ability to han-
dle the systems with hard constraints explicitly, it has at-
tracted a lot of attentions, from both academic community
and industrial society (see e.g., [1–4]). However, in the
real processes, the precise model parameters are seldomly
available. Hence, the robust MPC (RMPC) is more practi-
cal for the real applications.
The linear parameter varying (LPV) systems have their dy-
namics depending on the time-varying parameters, but take
their real-time model parameters in the pre-specified sets.
When the time-varying parameters of the linear system can
be exactly known at the current time, the systems are called
quasi-LPV (quasi-linear parameter varying) systems — it
means that the current model parameters are exactly known
at each time, but their future evolutions are uncertain but
contained in the prescribed bounded sets. The parametric
uncertainty can be dealt with within the frame of LPV sys-
tems. In RMPC, at each sampling time, usually a min-max
optimization is utilized to enhance the performance of the
LPV system, which considers all the possible realization of
model parametric uncertainty (see e.g., [5]). The authors
in [6] extended the approach in [5] to the quasi-LPV system
with quasi-min-max optimization. The authors in [7] con-
This work was supported by the National Nature Science Foundation
of China under Grant 60934007 and Grant 61174095, and by the Foun-
dation from the State Key Laboratory of Industrial Control Technology
under Grant no. ICT1213.
sidered the quasi-LPV system with parameter-dependent
control law. In [8–11], the controller design considers the
time-varying parameters of the quasi-LPV system having
bounds on their rates of variations.
In the real processes, the true states are usually unmeasur-
able, and only outputs with disturbance are available. Un-
der this situation, the output feedback RMPC (OFRMPC)
is more practical than the state feedback one for the real
applications. For the OFRMPC with bounded disturbance
without model parametric uncertainty, see [12]. For on-line
OFRMPC with both polytopic uncertainty and bounded
disturbance, see [13–19]. In [14], the authors considered
the OFRMPC with model parametric uncertainty repre-
sented by Takagi-Sugeno (T-S) fuzzy model, which is the
same as the quasi-LPV model. For the off-line OFRMPC
with polytopic model parametric uncertainty, see [20–22].
For the system with norm-bounded model parametric un-
certainty, [23] combines the off-line control law with the
on-line optimization.
For the OFRMPC, the calculation of the bounds on the es-
timation error set (EES) is an important issue, which has to
be considered for robust stability and physical constraints.
The works [19, 21, 22] consider the fixed estimation error
constraints in the off-line optimization problems. Hence,
the on-line searched controller parameters can guarantee
the satisfaction of estimation error constraints in the on-line
searching stage. Both the off-line and the on-line optimiza-
tion problems in [23] consider the fixed estimation error
constraints. In [13–15, 17], the estimation error constraints
are not considered in the main optimization problem, which
are advantageous to enhance feasibility and optimality. In-
stead, the EESs can be refreshed in the separate on-line
auxiliary optimizations. In [18], the bounds of true state,
which plays the same role as the EESs, are calculated, and
726
978-1-4673-5532-2
c
2013 IEEE
资源评论
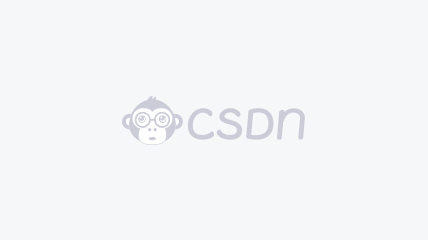

weixin_38659805
- 粉丝: 6
- 资源: 914
上传资源 快速赚钱
我的内容管理 展开
我的资源 快来上传第一个资源
我的收益
登录查看自己的收益我的积分 登录查看自己的积分
我的C币 登录后查看C币余额
我的收藏
我的下载
下载帮助

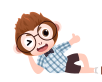
最新资源
资源上传下载、课程学习等过程中有任何疑问或建议,欢迎提出宝贵意见哦~我们会及时处理!
点击此处反馈


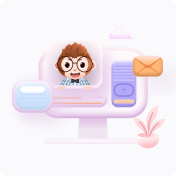
安全验证
文档复制为VIP权益,开通VIP直接复制
