import cv2
import numpy as np
import random
from PIL import Image, ImageEnhance
import matplotlib.pyplot as plt
import os
from tqdm import tqdm
# 随机缩放
def random_scale(image, label, scale_range=(0.8, 1.2)):
scale = random.uniform(*scale_range) # 随机生成缩放比例
height, width = image.shape[:2] # 获取原始图像尺寸
new_size = (int(width * scale), int(height * scale)) # 计算新的图像尺寸
image = cv2.resize(image, new_size, interpolation=cv2.INTER_LINEAR) # 按新尺寸缩放图像
label = cv2.resize(label, new_size, interpolation=cv2.INTER_NEAREST) # 按新尺寸缩放标签图像
return image, label
# 随机亮度
def random_brightness(image, brightness_range=(0.7, 1.3)):
enhancer = ImageEnhance.Brightness(Image.fromarray(image)) # 创建亮度增强对象
brightness = random.uniform(*brightness_range) # 在指定范围内生成随机亮度因子
image = enhancer.enhance(brightness) # 调整图像亮度
return np.array(image) # 转换图像格式并返回
# 随机翻转
def random_flip(image, label):
# 随机水平翻转
if random.random() > 0.5:
image = cv2.flip(image, 1)
label = cv2.flip(label, 1)
# 随机垂直翻转
if random.random() > 0.5:
image = cv2.flip(image, 0)
label = cv2.flip(label, 0)
return image, label
# 随机裁剪
def random_crop(image, label, crop_size=(256, 256)):
height, width = image.shape[:2]
crop_h, crop_w = crop_size # 获取图像的高度和宽度
if height > crop_h and width > crop_w: # 获取图像的高度和宽度
top = random.randint(0, height - crop_h) # 随机生成裁剪的左上角坐标
left = random.randint(0, width - crop_w)
image = image[top: top + crop_h, left: left + crop_w] # 从图像和标签中裁剪出指定大小的区域
label = label[top: top + crop_h, left: left + crop_w]
else:
image = cv2.resize(image, crop_size, interpolation=cv2.INTER_LINEAR)
label = cv2.resize(label, crop_size, interpolation=cv2.INTER_NEAREST)
return image, label
# 随机旋转
def random_rotation(image, label, angle_range=(-30, 30)):
angle = random.uniform(*angle_range) # 在指定角度范围内随机生成旋转角度
height, width = image.shape[:2] # 获取图像的高度和宽度
matrix = cv2.getRotationMatrix2D((width // 2, height // 2), angle, 1) # 计算旋转矩阵
image = cv2.warpAffine(image, matrix, (width, height), flags=cv2.INTER_LINEAR, borderMode=cv2.BORDER_REFLECT) # 应用旋转变换到图像
label = cv2.warpAffine(label, matrix, (width, height), flags=cv2.INTER_NEAREST, borderMode=cv2.BORDER_REFLECT) # 应用旋转变换到标签
return image, label # 返回旋转后的图像和标签
def augment_image_and_label(image_path, label_path, crop_size=(256, 256)):
image = cv2.imread(image_path)
label = cv2.imread(label_path, 0) # 读取标签图像,灰度模式
# 随机缩放
image, label = random_scale(image, label)
# 随机亮度
image = random_brightness(image)
# 随机翻转
image, label = random_flip(image, label)
# 随机旋转
image, label = random_rotation(image, label)
# 随机裁剪
# image, label = random_crop(image, label, crop_size)
return image, label
# 获取文件路径
image_path = "Enhance_INF_Images/JPEGImages"
label_path = "Enhance_INF_Images/SegmentationClass"
# 增强后输出保存的文件路径
image_enhance_path = 'Enhance_INF_Images/JPEGImages_Enhance_2'
label_enhance_path = 'Enhance_INF_Images/SegmentationClass_Enhance_2'
# 如果增强后的文件夹不存在,创建它们
os.makedirs(image_enhance_path,exist_ok=True)
os.makedirs(label_enhance_path,exist_ok=True)
# 获取文件夹中所有的文件
file_list_image = sorted(os.listdir(image_path))
file_list_label = sorted(os.listdir(label_path))
enhanced_num = 5
# 遍历文件列表,确保文件列表长度相同,并一一对应
for i in tqdm(range(enhanced_num),desc = "Processing images"):
for file_name_image,file_name_label in zip(file_list_image,file_list_label):
# for file_name_image, file_name_label in zip(file_list_image, file_list_label):
# 拼接文件路径
file_path_image = os.path.join(image_path, file_name_image)
file_path_label = os.path.join(label_path,file_name_label)
augmented_image, augmented_label = augment_image_and_label(file_path_image, file_path_label)
output_name_image = file_name_image[:-4]+'_enhanced_'+str(i)+'.jpg'
output_name_label = file_name_label[:-4] + '_enhanced_' + str(i) + '.png'
print(output_name_image)
# 保存增强后的图像和标签
cv2.imwrite(os.path.join(image_enhance_path, output_name_image), augmented_image)
cv2.imwrite(os.path.join(label_enhance_path, output_name_label), augmented_label)
print("数据增强完成!")

视觉研坊
- 粉丝: 8w+
- 资源: 3
最新资源
- 电力电子仿真设计:探讨带平衡电抗器的双反星形与两级三相桥式全控整流电路、交流调压及DC-DC变换电路的设计与应用,电力电子仿真设计:探讨双反星形可控整流电路与三相桥式全控整流电路串联组合的技术,交流调
- 基于Yolov2与GoogleNet深度学习算法的疲劳驾驶检测系统Matlab仿真研究及GUI界面实现,基于Yolov2与GoogleNet的疲劳驾驶检测系统Matlab仿真及GUI界面实现,基于Yo
- 基于Yolov2深度学习算法的驾驶员打电话行为实时监测与预警系统Matlab仿真研究,基于Yolov2深度学习算法的驾驶员打电话行为实时监测与预警系统Matlab仿真研究,基于Yolov2深度学习网络
- 基于CNN卷积神经网络的IBDFE单载波频域均衡策略与应用算法研究,基于CNN卷积神经网络的优化算法:IBDFE单载波频域均衡策略,基于CNN卷积神经网络的IBDFE单载波频域均衡算法 ,基于CNN的
- 基于Vue框架的贷款项目设计源码
- 太阳能电池板局部遮阴仿真模型:探究串联与并联阵列在阴影条件下的输出特性及I-U与P-U特性曲线分析,太阳能电池板局部遮阴仿真模型:探究串联与并联阵列输出特性及I-U与P-U曲线变化,3行3列的太阳能电
- 基于遗传算法的MATLAB作业调度优化:甘特图展示最小惩罚与最大收益解决方案,基于遗传算法的Matlab作业调度优化:最小化惩罚,最大化效益展示甘特图解决方案,基于matlab的作业调度问题 采用遗
- 基于MATLAB的AS-NSGA2自适应非支配排序遗传算法优化截止阀响应面设计源码
- 基于前馈补偿的龙伯格观测器在永磁同步电机负载转矩估计中的应用与优化,基于前馈补偿的龙伯格观测器在永磁同步电机负载转矩估计中的应用与优化,基于前馈补偿的龙伯格观测器永磁同步电机负载转矩估计 ①采用降阶负
- 基于Dart语言的Flutter跨平台UI框架设计源码
- 基于Comsol多层冻土地基的冻涨模型研究:低温热流固三场耦合模型与固体力学模拟,基于comsol的多层冻土地基冻涨模型:低温环境下的热流固三场耦合模型与固体力学模拟研究,comsol多层冻土地基冻涨
- 基于Simulink仿真的稳定频差光锁相环系统性能分析与优化研究,基于Simulink的稳定频差分析:光锁相环系统性能仿真研究,基于Simulink的稳定频差光锁相环系统性能仿真 ,基于Simulin
- Matlab YALMIP 接口 CPLEX 求解:带储能系统的微电网优化调度策略研究,Matlab YALMIP 接口 CPLEX 求解:带储能微电网优化调度问题的策略与实现,Matlab+YALM
- 除灰控制监控系统设计报告:全面监控管理,智能高效运行体验,实时数据采集分析与报警处理,用户友好的界面与通讯协议支持 ,《智能化除灰控制监控系统设计报告:高效管理与实时监控一体化解决方案》,除灰控制监控
- 《基于MATLAB2016b的5MW永磁同步风机与1200V直流混合储能并网系统仿真研究》,基于MATLAB2016b的5MW永磁同步风机与混合储能并网仿真研究,5MW永磁同步风机-1200V直流混合
- 基于三菱PLC与触摸屏的病床呼叫控制系统组态设计详解:梯形图程序、接线图与组态画面全解析,基于三菱PLC与触摸屏的病床呼叫控制系统组态设计详解:梯形图程序、接线图与组态画面全解析,基于三菱PLC和三菱
资源上传下载、课程学习等过程中有任何疑问或建议,欢迎提出宝贵意见哦~我们会及时处理!
点击此处反馈


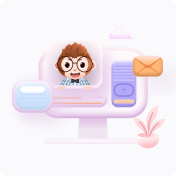