2023年美赛特等奖论文-E-2307336-解密.pdf
2.虚拟产品一经售出概不退款(资源遇到问题,请及时私信上传者)
### 2023年美赛特等奖论文-E-2307336-解密 #### 大学生数学建模与美国大学生数学建模竞赛(MCM/ICM) 在2023年的美国大学生数学建模竞赛(MCM/ICM)中,编号为E-2307336的团队凭借其出色的数学模型和解决方案获得了特等奖(O奖)。这篇论文主要关注的是光污染问题,并提出了一系列创新的方法来评估和减轻光污染的影响。 #### 光污染风险评估模型 对于任务1,研究团队提出了一个综合性的“光污染风险评估模型”。该模型考虑了四个维度的风险因素:人类、野生动物、植物以及由光污染造成的能源浪费。为了更准确地评估这些因素的影响程度,模型采用了扩展权重多指标排序技术(EWM-TOPSIS),这是一种能够处理多个指标并给出总体风险评分的有效方法。根据评分结果,可以将地点的风险水平划分为四个等级:脆弱(0-1)、较差(1-2)、一般(2-3)和良好(3-4)。 #### 四个典型区域的应用案例 在任务2中,研究团队将这个光污染风险评估模型应用于深圳市的四个典型区域:城市区、郊区、乡村以及保护区。通过收集夜间遥感数据和多光谱遥感数据,研究者计算了这些区域的归一化植被指数(NDVI)、夜间辐射强度以及人口密度等关键指标。根据这些数据,保护区的风险评分为0.357992,乡村社区的风险评分为1.859474,郊区社区的风险评分为2.114942,而城市社区的风险评分为3.19662。这些评分分别对应于脆弱、较差、一般和良好的风险等级。 #### 干预策略与模型建立 为了进一步解决光污染问题,在任务3中,研究团队开发了三种干预策略:改善光源、降低照明强度以及优化区域灯光布局。每种策略都包含了一系列具体行动方案。基于微分方程的干预策略模型被用来量化这些策略对降低风险等级的具体影响。 - **改善光源**:这包括使用更环保的LED灯取代传统的白炽灯或荧光灯,以减少能源消耗和环境污染。 - **降低照明强度**:通过调整照明设备的设计和设置,例如使用智能控制系统,可以根据实际需求动态调节照明强度,避免过度照明。 - **优化区域灯光布局**:通过对城市规划进行调整,合理安排公共照明设施的位置和数量,以最小化对自然环境的影响。 #### 模型验证与未来展望 在任务4中,研究团队可能会继续探讨如何验证这些干预策略的有效性,并对未来可能遇到的问题提出解决方案。例如,可以通过模拟不同场景下的光污染情况来评估模型的预测能力,或者研究如何将这些理论应用到其他城市和地区中去。 这篇特等奖论文不仅展示了数学建模在解决现实世界问题中的强大作用,还提供了一套系统性的方法来应对光污染这一全球性挑战。通过采用科学的方法和技术手段,我们可以更好地保护地球上的生态环境,创造一个更加可持续的未来。
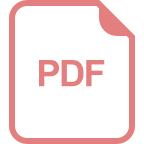
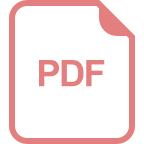
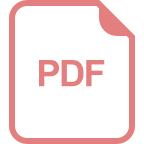
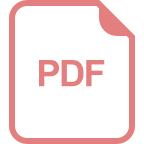
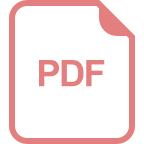
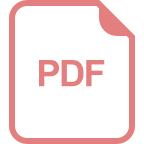
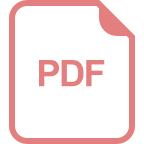
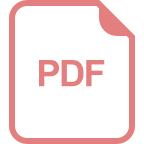
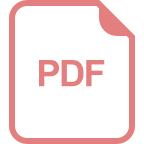
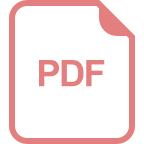
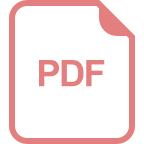
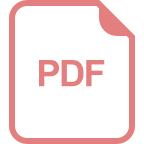
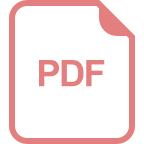
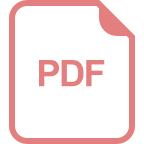
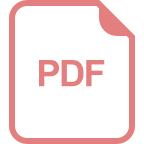
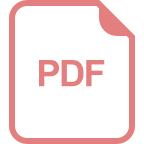
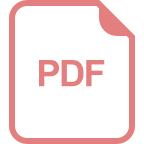
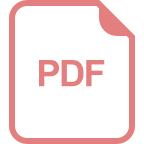
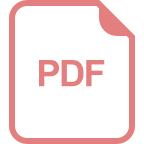
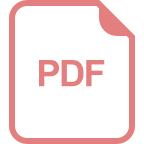
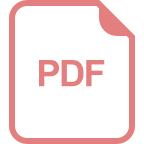
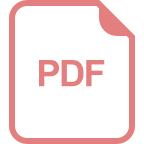
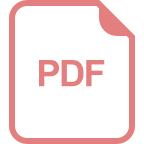
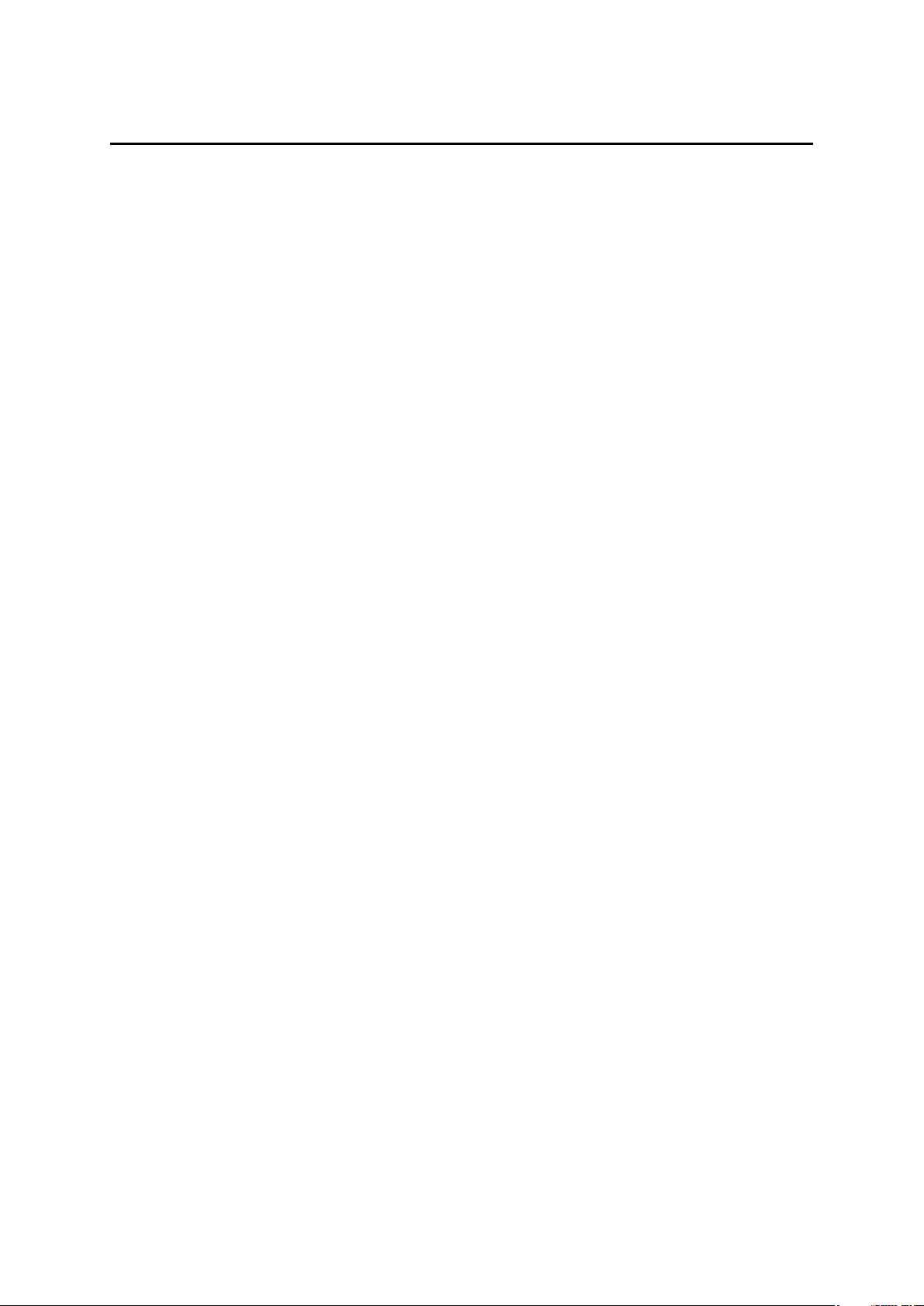
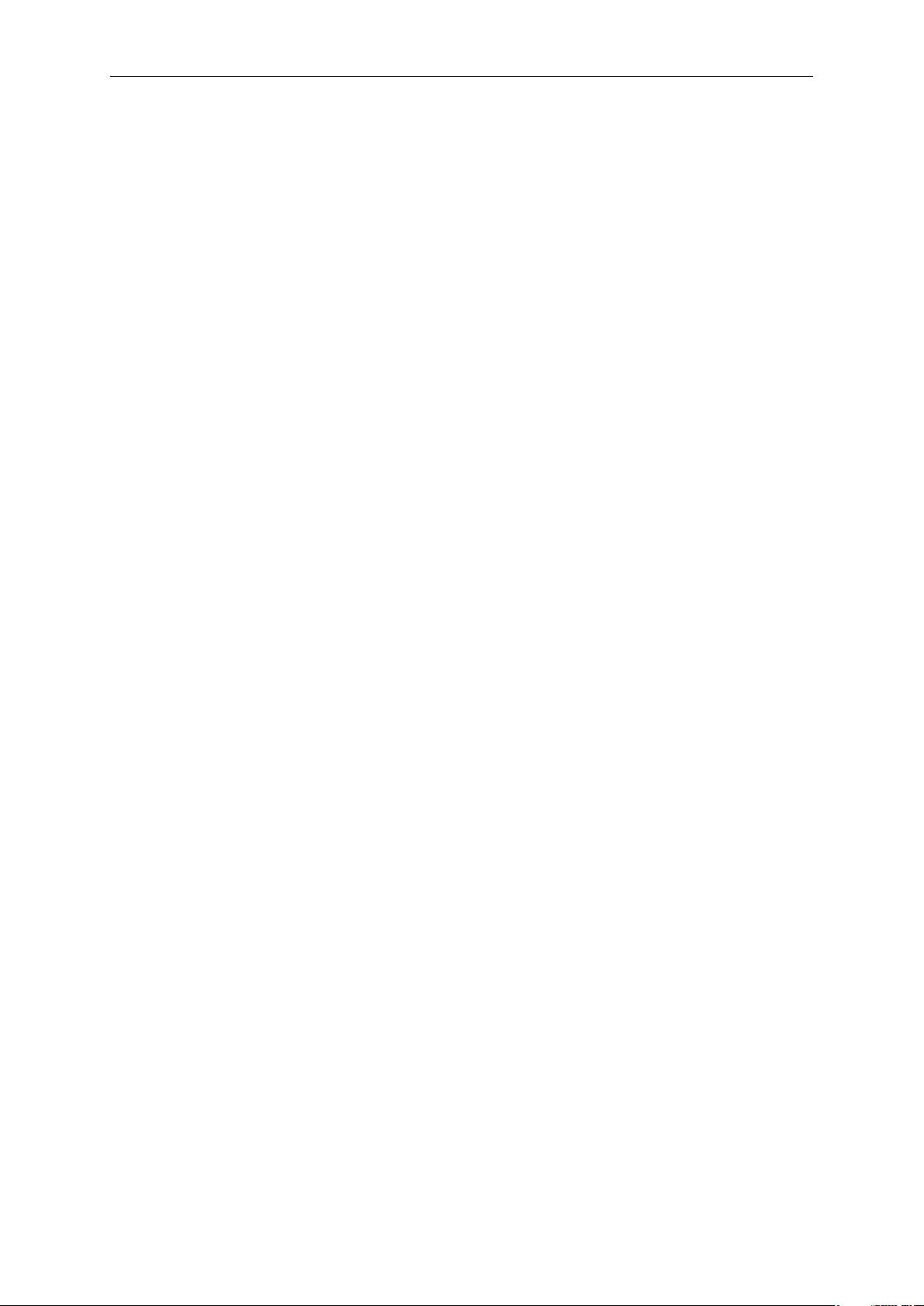
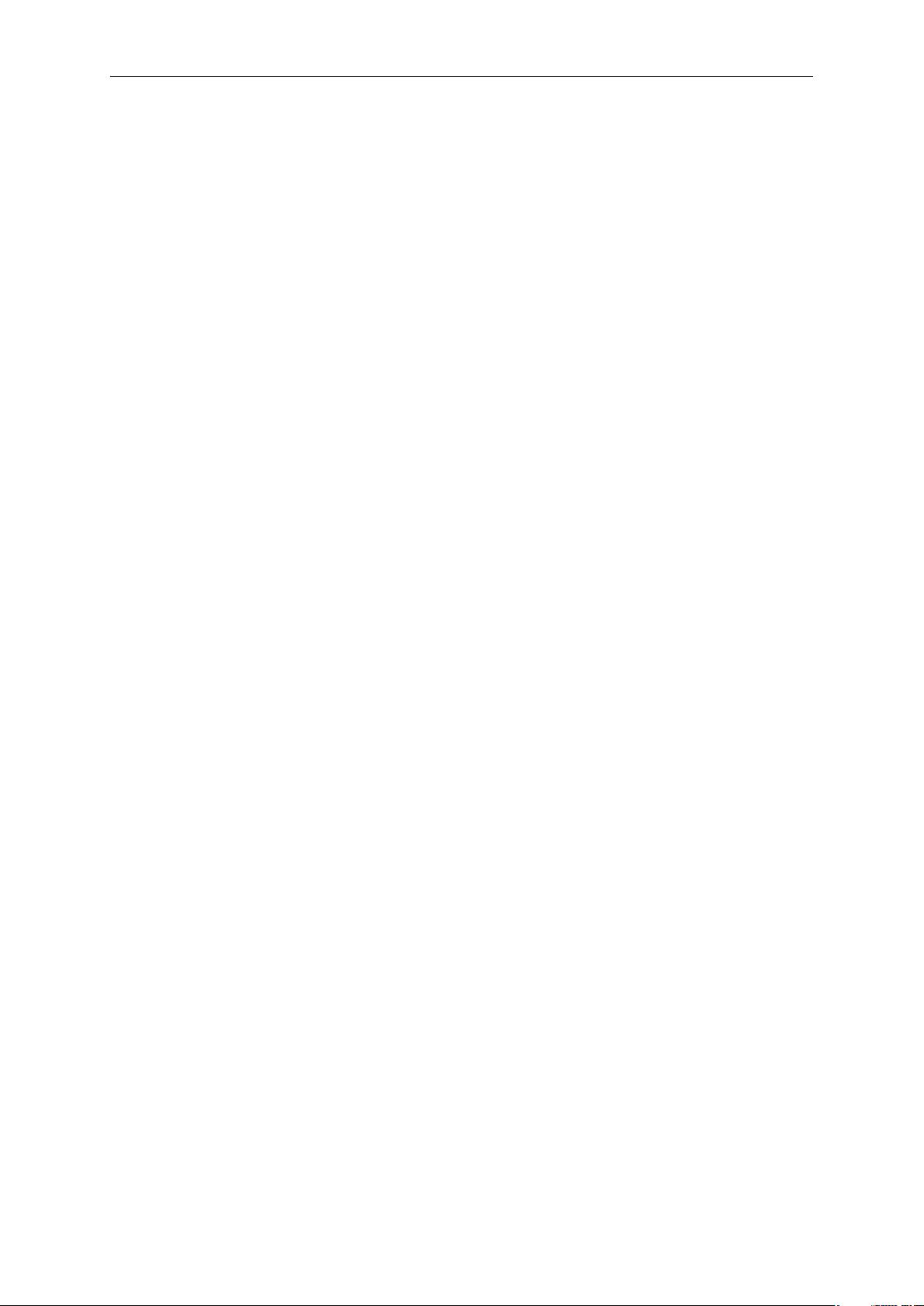
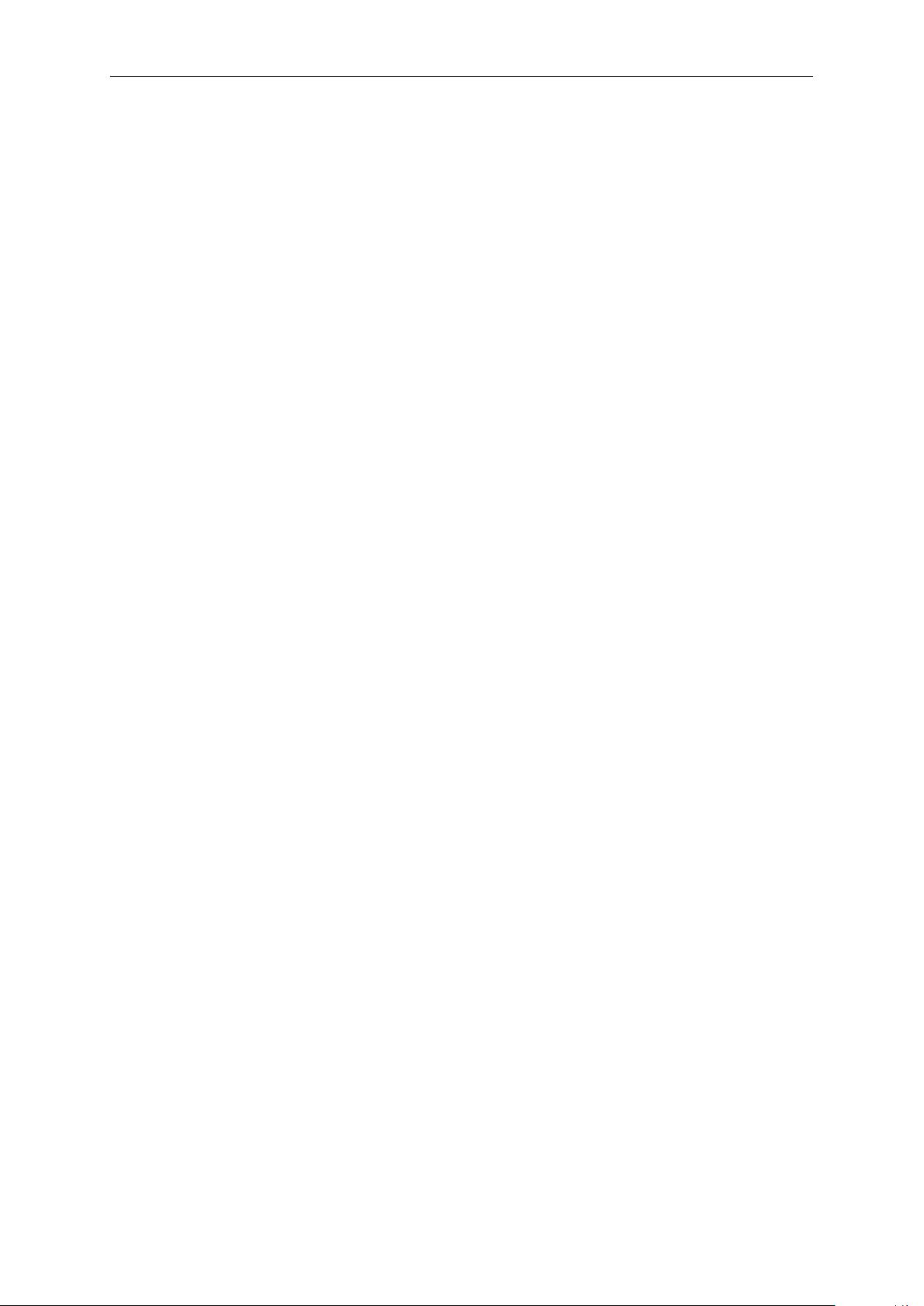
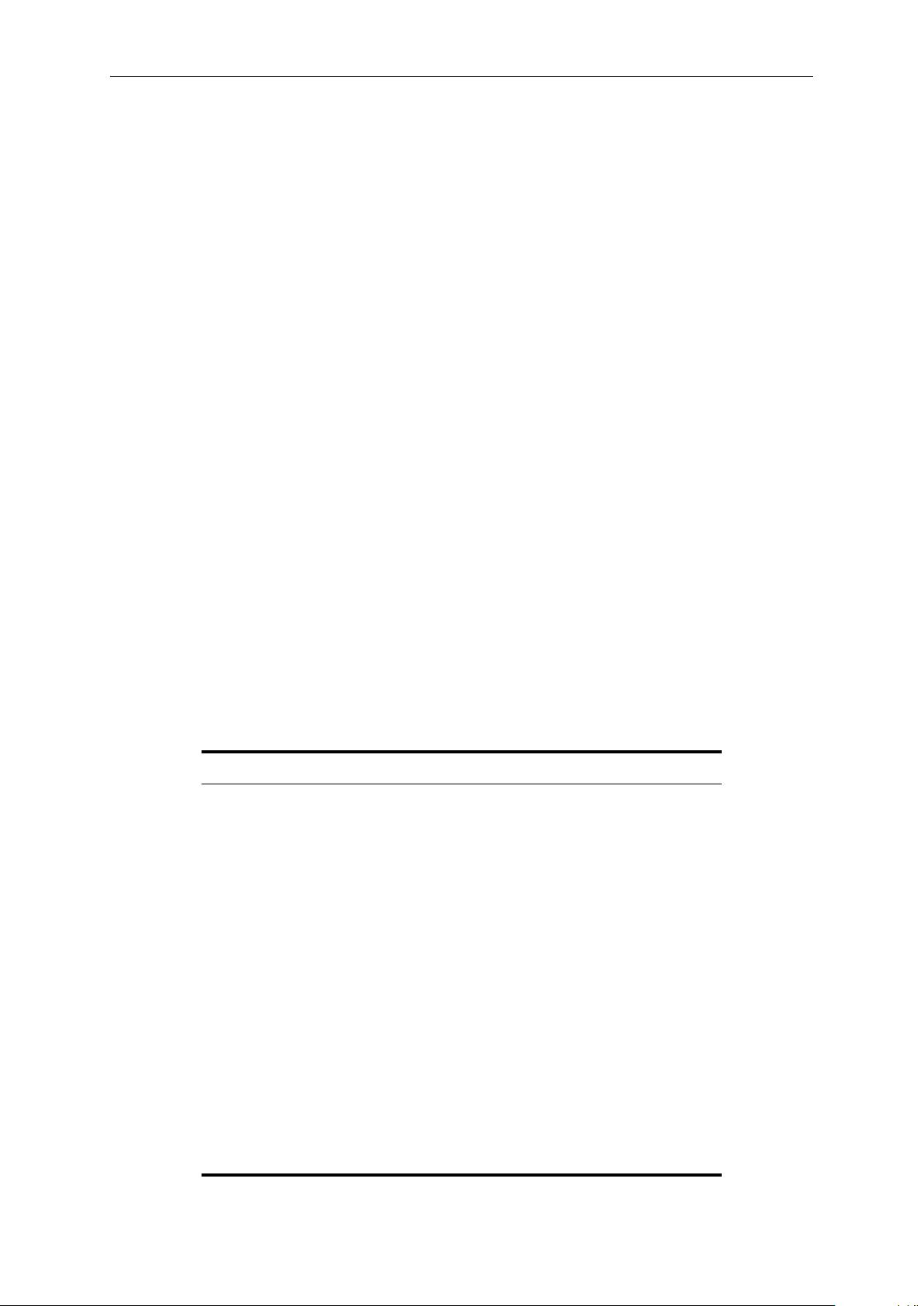
剩余23页未读,继续阅读
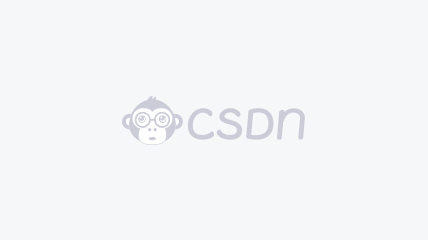

- 粉丝: 2686
- 资源: 5734
我的内容管理 展开
我的资源 快来上传第一个资源
我的收益
登录查看自己的收益我的积分 登录查看自己的积分
我的C币 登录后查看C币余额
我的收藏
我的下载
下载帮助

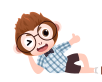
最新资源
- 阿尔法平台选择有批注(1-11).docx
- TA-Lib-0.4.28-cp311-cp311-win-amd64.whl
- 玄铁e907-r1s1用户手册-occ
- 阿尔法平台填空自测.pdf
- 匠芯创D13x芯片用户手册
- 阿尔法填空答案填空.pdf
- 匠芯创D13x硬件设计手册
- 阿尔法实验汇总.docx
- 匠芯创D13x数据手册
- 2024PPt资源02
- 手机拆螺丝机sw16可编辑全套技术资料100%好用.zip
- RISC-V 手册 中文版
- 四季除草机sw16可编辑全套技术资料100%好用.zip
- 水面垃圾自动收集装置sw18全套技术资料100%好用.zip
- 提砂机(砂水分离)sw18全套技术资料100%好用.zip
- 四柱油压机sw18可编辑全套技术资料100%好用.zip

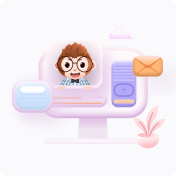
