没有合适的资源?快使用搜索试试~ 我知道了~
Linear Algebra and Its Applications (Lax)2nd课后习题答案

温馨提示
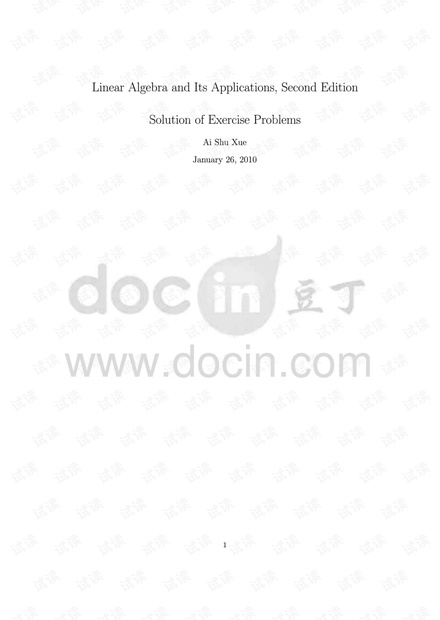

试读
55页
Linear Algebra and Application(Peter.D.Lax著)答案 英文版,很好懂的!
资源推荐
资源评论
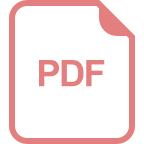
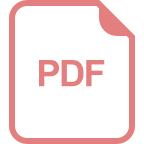
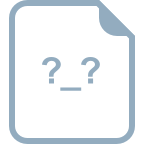
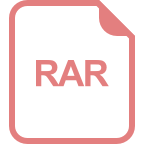
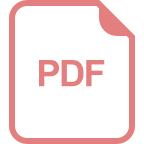
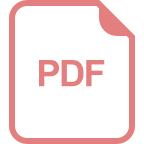
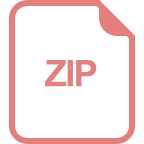
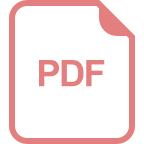
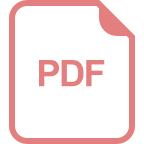
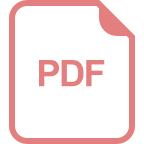
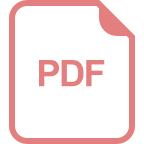
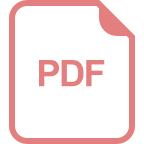
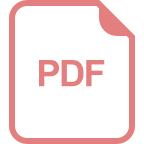
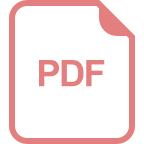
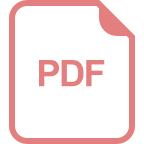
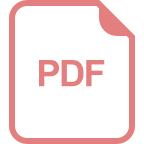
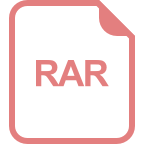
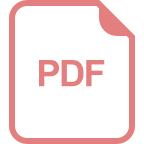
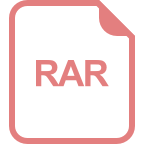
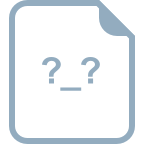
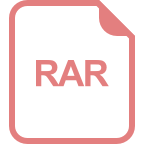
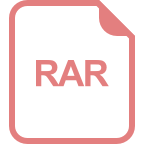
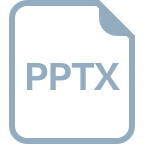

ZY15881001865
- 粉丝: 1
- 资源: 2
上传资源 快速赚钱
我的内容管理 展开
我的资源 快来上传第一个资源
我的收益
登录查看自己的收益我的积分 登录查看自己的积分
我的C币 登录后查看C币余额
我的收藏
我的下载
下载帮助

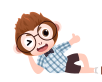
安全验证
文档复制为VIP权益,开通VIP直接复制

- 1
- 2
前往页