# VDO-SLAM
# Prerequisites
We have tested the library in **Mac OS X 10.14** and **Ubuntu 16.04**, but it should be easy to compile in other platforms.
## c++11, gcc and clang
We use some functionalities of c++11, and the tested gcc version is 9.2.1 (ubuntu), the tested clang version is 1000.11.45.5 (Mac).
## OpenCV
We use [OpenCV](http://opencv.org) to manipulate images and features. Download and install instructions can be found at: http://opencv.org. **Required at least 3.0. Tested with OpenCV 3.4**.
## Eigen3
Required by g2o (see below). Download and install instructions can be found at: http://eigen.tuxfamily.org. **Required at least 3.1.0**.
## g2o (Included in dependencies folder)
We use modified versions of [g2o](https://github.com/RainerKuemmerle/g2o) library to perform non-linear optimizations. The modified libraries (which are BSD) are included in the *dependencies* folder.
## Use Dockerfile for auto installation
For Ubuntu users, a Dockerfile is added for automatically installing all dependencies for reproducible environment, built and tested with KITTI dataset. (Thanks @satyajitghana for the contributions ���� )
# Building VDO-SLAM Library
We provide a script `build.sh` to build the *dependencies* libraries and *VDO-SLAM*.
Please make sure you have installed all required dependencies (see section 2).
**Please also change the library file suffix, i.e., '.dylib' for Mac (default) or '.so' for Ubuntu, in the main CMakeLists.txt.**
Then Execute:
```
cd VDO-SLAM
chmod +x build.sh
./build.sh
```
This will create
1. **libObjSLAM.dylib (Mac)** or **libObjSLAM.so (Ubuntu)** at *lib* folder,
2. **libg2o.dylib (Mac)** or **libg2o.so (Ubuntu)** at */dependencies/g2o/lib* folder,
3. and the executable **vdo_slam** in *example* folder.
# 4. Running Examples
## KITTI Tracking Dataset
1. Download the demo sequence: [kitti_demo](https://drive.google.com/file/d/1LpjIdh6xL_UtWOkiJng0CKSmP7qAQhGu/view?usp=sharing), and uncompress it.
2. Execute the following command.
```
./example/vdo_slam example/kitti-0000-0013.yaml PATH_TO_KITTI_SEQUENCE_DATA_FOLDER
```
## Oxford Multi-motion Dataset
1. Download the demo sequence: [omd_demo](https://drive.google.com/file/d/1t4rG685a_7r0bHuW0bPKNbOhyiugnJK7/view?usp=sharing), and uncompress it.
2. Execute the following command.
```
./example/vdo_slam example/omd.yaml PATH_TO_OMD_SEQUENCE_DATA_FOLDER
```
# 5. Processing Your Own Data
You will need to create a settings (yaml) file with the calibration of your camera. See the settings files provided in the *example/* folder. RGB-D input must be synchronized and depth registered. A list of timestamps for the images is needed for input.
The system also requires image pre-processing as input, which includes instance-level semantic segmentation and optical flow estimation. In our experiments, we used [Mask R-CNN](https://github.com/matterport/Mask_RCNN) for instance segmentation (for KITTI only; we applied colour-based method to segment cuboids in OMD, check the matlab code in [tools](https://github.com/halajun/VDO_SLAM/blob/master/tools) folder), and [PWC-NET](https://github.com/NVlabs/PWC-Net) (PyTorch version) for optic-flow estimation. Other state-of-the-art methods can also be applied instead for better performance.
For evaluation purpose, ground truth data of camera pose and object pose are also needed as input. Details of input format are shown as follows,
## Input Data Pre-processing
1. The input of segmentation mask is saved as matrix, same size as image, in .txt file. Each element of the matrix is integer, with 0 stands for background, and 1,2,...,n stands for different instance label. Note that, to easily compare with ground truth object motion in KITTI dataset, we align the estimated mask label with the ground truth label. The .txt file generation (from .mask) and alignment code is in [tools](https://github.com/halajun/VDO_SLAM/blob/master/tools) folder.
2. The input of optical flow is the standard .flo file that can be read and processed directly using OpenCV.
## Ground Truth Input for Evaluation
1. The input of ground truth camera pose is saved as .txt file. Each row is organized as follows,
```
FrameID R11 R12 R13 t1 R21 R22 R23 t2 R31 R32 R33 t3 0 0 0 1
```
Here Rij are the coefficients of the camera rotation matrix **R** and ti are the coefficients of the camera translation vector **t**.
2. The input of ground truth object pose is also saved as .txt file. One example of such file (**KITTI Tracking Dataset**), which each row is organized as follows,
```
FrameID ObjectID B1 B2 B3 B4 t1 t2 t3 r1
```
Where ti are the coefficients of 3D object location **t** in camera coordinates, and r1 is the Rotation around Y-axis in camera coordinates. B1-4 is 2D bounding box of object in the image, used for visualization. Please refer to the details in **KITTI Tracking Dataset** if necessary.
The provided object pose format of **OMD** dataset is axis-angle + translation vector. Please see the provided demos for details. A user can input a custom data format, but need to write a new function to input the data.
没有合适的资源?快使用搜索试试~ 我知道了~
资源推荐
资源详情
资源评论
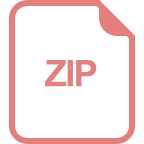
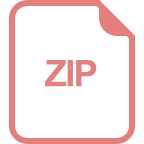
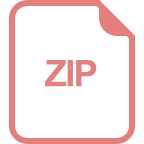
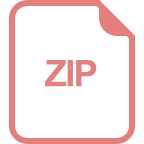
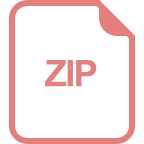
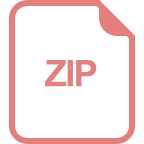
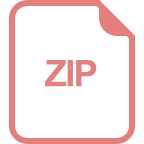
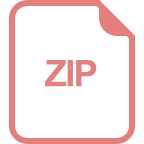
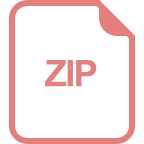
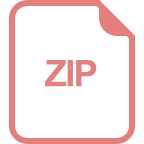
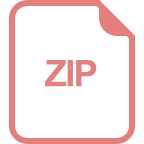
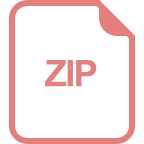
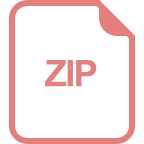
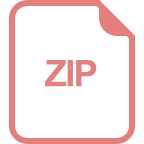
收起资源包目录

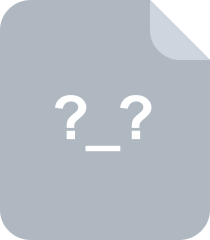
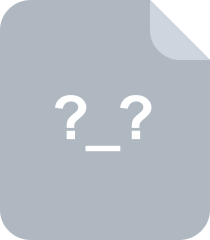
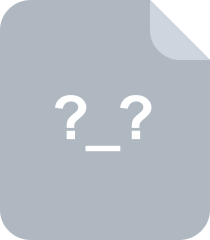
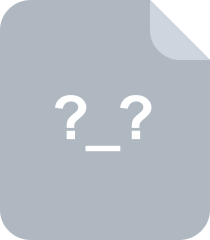
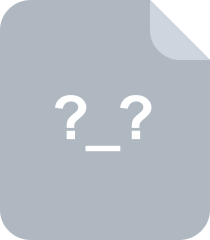
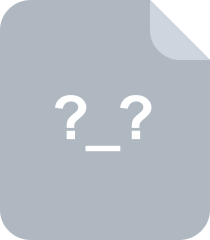
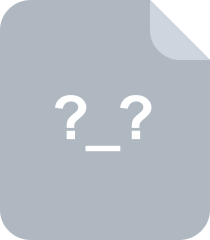
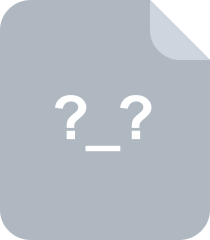
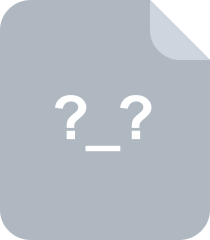
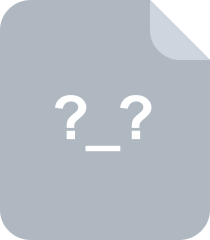
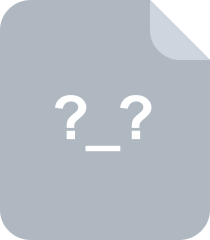
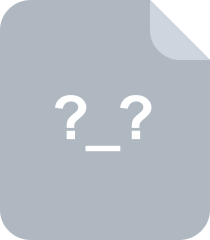
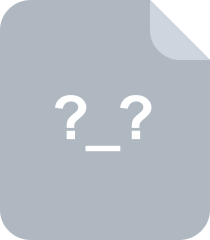
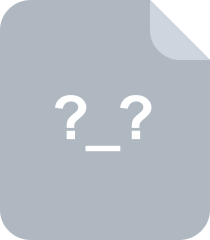
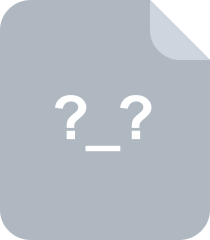
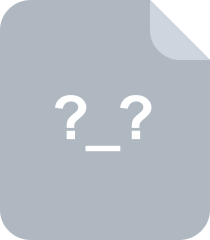
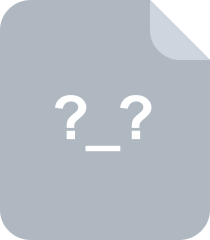
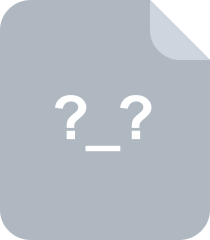
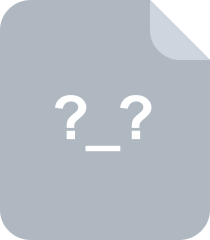
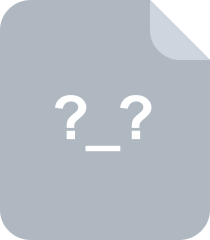
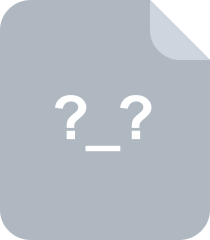
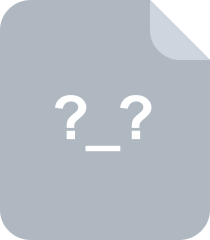
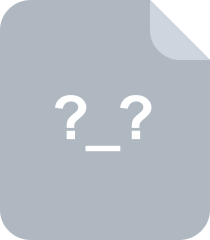
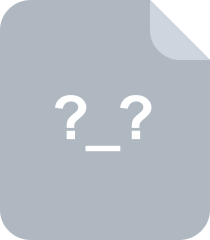
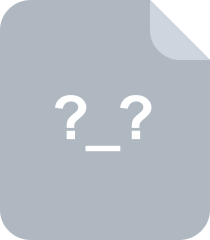
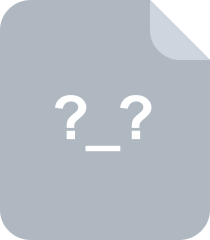
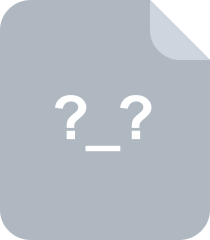
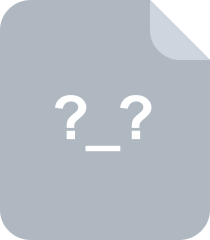
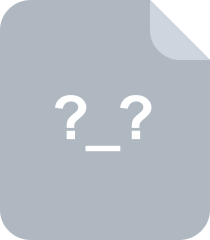
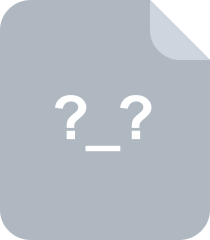
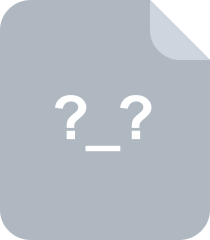
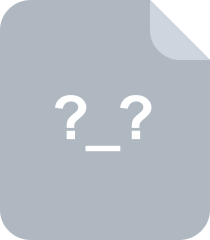
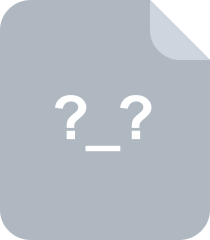
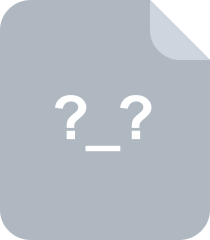
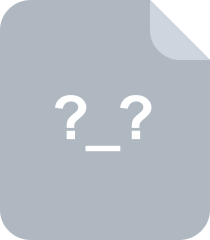
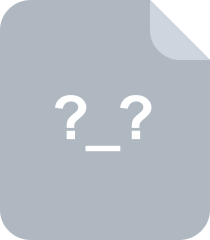
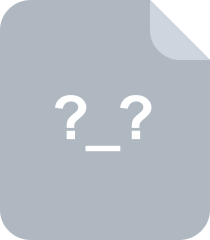
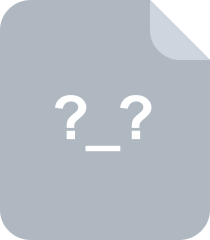
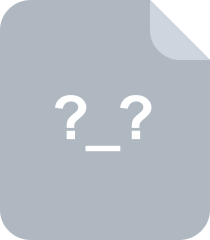
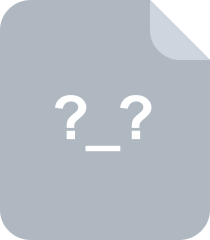
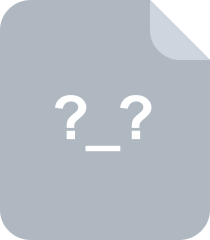
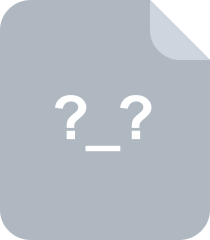
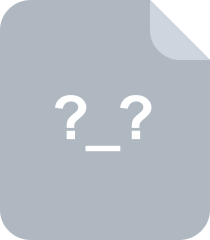
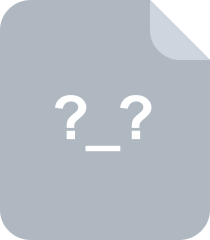
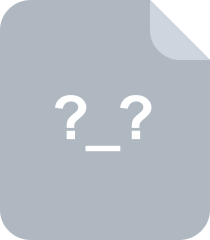
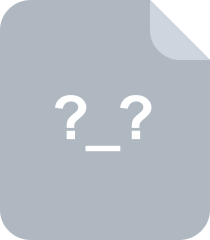
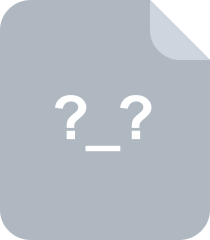
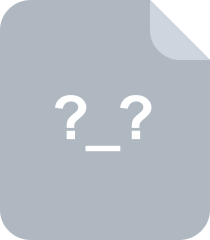
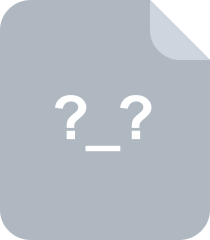
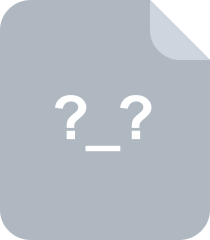
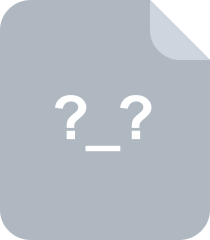
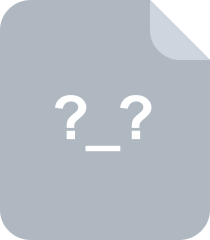
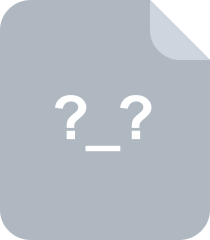
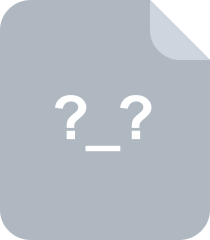
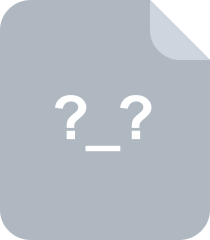
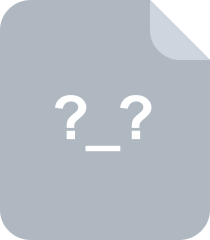
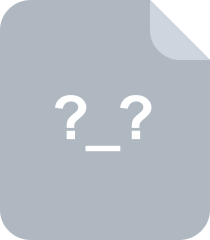
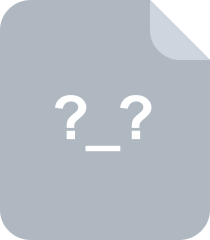
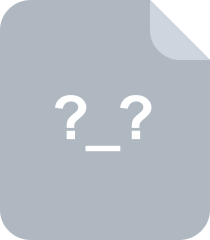
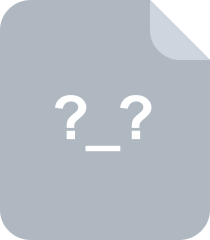
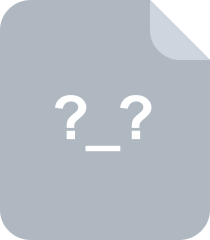
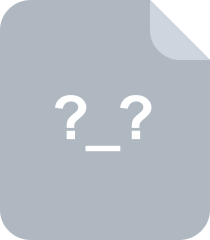
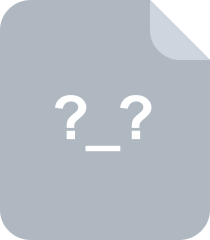
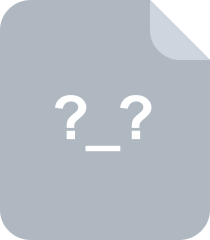
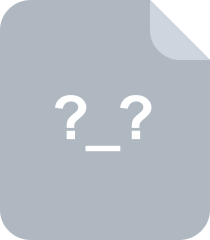
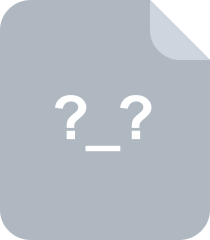
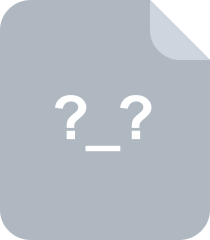
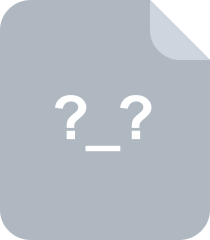
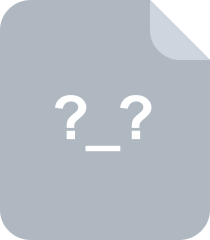
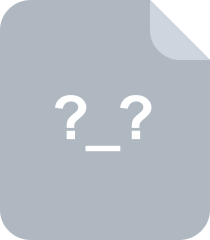
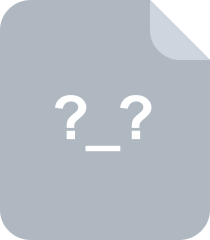
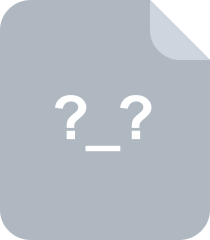
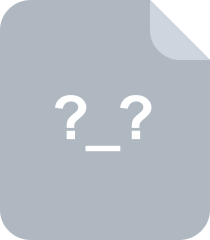
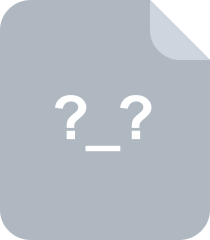
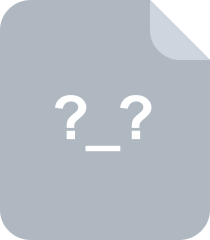
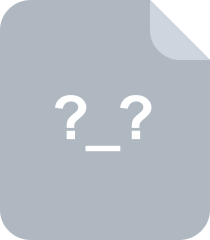
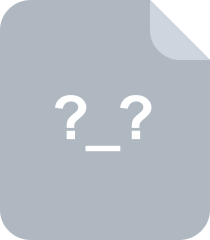
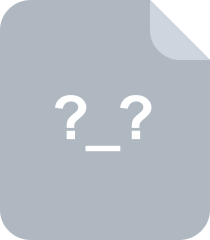
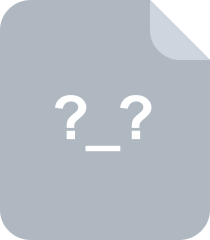
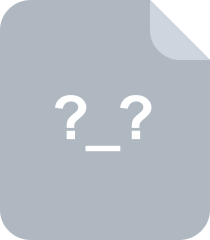
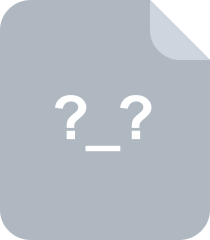
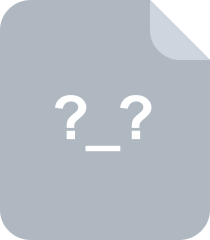
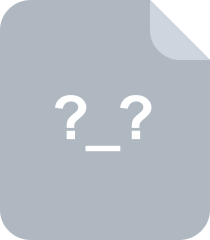
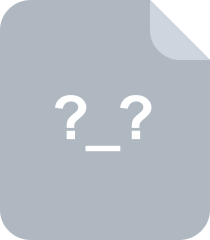
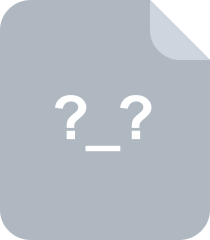
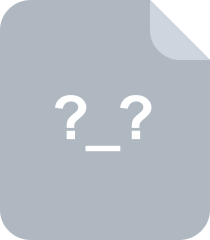
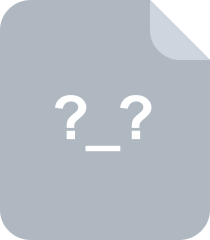
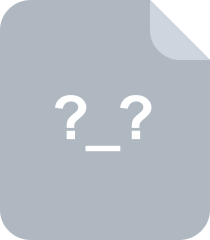
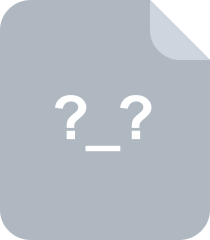
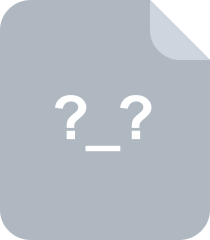
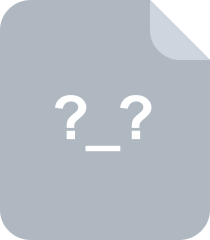
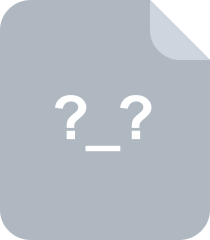
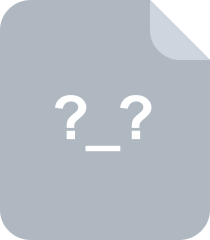
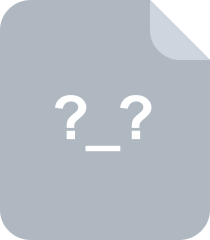
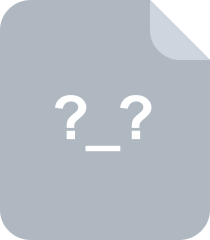
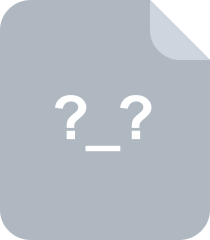
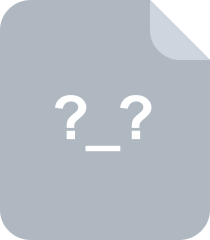
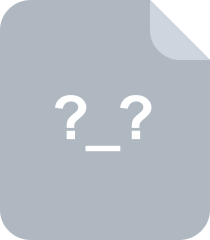
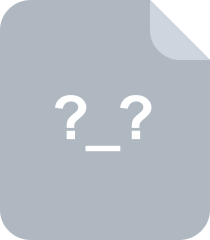
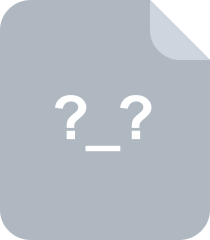
共 188 条
- 1
- 2
资源评论
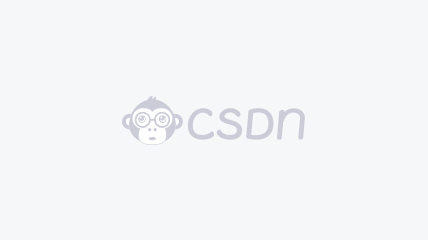

__AtYou__
- 粉丝: 3503
- 资源: 2157
上传资源 快速赚钱
我的内容管理 展开
我的资源 快来上传第一个资源
我的收益
登录查看自己的收益我的积分 登录查看自己的积分
我的C币 登录后查看C币余额
我的收藏
我的下载
下载帮助

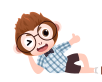
最新资源
资源上传下载、课程学习等过程中有任何疑问或建议,欢迎提出宝贵意见哦~我们会及时处理!
点击此处反馈


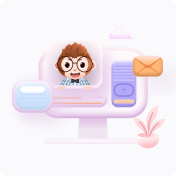
安全验证
文档复制为VIP权益,开通VIP直接复制
