# 3D U-Net model for segmentation of prostate structures
In this project, I use the [3D U-Net](https://arxiv.org/pdf/1606.06650.pdf) model to segment prostate structures from the [NCI-ISBI 2013 challenge dataset](https://wiki.cancerimagingarchive.net/display/Public/NCI-ISBI+2013+Challenge+-+Automated+Segmentation+of+Prostate+Structures). Given a 3D MRI scan, the aim is to automatically annotate the peripheral zone (PZ) and central gland (CG) regions, as shown in Figure 1.

*Figure 1:* Segmentation of prostate structures: MRI scan (left) and its annotation (right), where the peripheral zone (PZ) is coloured *dark gray*, central gland (CG) *bright gray*, and the background *black*.
## Dataset
| | # 3D scans | Total # scan slices |
| :--- | :---: | :---: |
| Training set | 60 | 1544 |
| Validation set | 10 | 261 |
| Test set | 10 | 271 |
## Data pre-processing
In the pre-processing stage, I performed histogram equalisation on the input MR images, in order to increase image contrast. As shown in Figure 2, this technique redistributes pixel intensity values to achieve linear cumulative distribution function.

*Figure 2:* Example of the employed histogram equalisation.
## Data augmentation
Following the 3D U-Net paper, besides random rotations, scalings, and gray value variations, I also performed a smooth dense deformation field augmentation (also known as elastic distortion/transformation). The same random deformation was applied to a voxel tile and its annotation, as shown in Figure 3.
All data augmentations were performed in the pre-processing stage as it allowed faster training, although at the expense of higher memory requirements (when compared to the augmentation on-the-fly).

*Figure 3:* Example of elastic deformation used for data augmentation.
## Results
The best and the worst predictions are shown in Figures 4 and 5 respectively.

*Figure 4:* The test scan with the highest score (in terms of Intersection Over Union), i.e. the **best** prediction.

*Figure 5:* The test scan with the lowest score (in terms of Intersection Over Union), i.e. the **worst** prediction.

__AtYou__
- 粉丝: 3513
- 资源: 2177
最新资源
- 强化学习控制电动汽车储能系统的Matlab项目.rar
- 强化学习算法的基准案例:网格世界和推车上的倒立摆Matlab代码.rar
- 天邦达上位机软件2.35
- 轻型包裹运输的自主无人机递送系统附matlab代码.rar
- 深度强化学习应用无人机附python代码.rar
- 人类强化学习中的无模型过程Matlab代码.rar
- 设计和开发一种受蝙蝠启发的微型无人机,可以通过救援和监视行动协助灾害管理matlab代码.rar
- 实现分层强化学习算法Matlab实现.rar
- 使用 FDM 和 PWE 方法计算谐振微腔中麦克斯韦方程组的解matlab代码.rar
- 使用 EKF 的 Cubesat 姿态确定Matlab代码.rar
- Matlab实现TSOA-CNN-GRU-Mutilhead-Attention凌日优化算法优化卷积门控循环单元融合多头注意力机制多特征分类预测(含完整的程序,GUI设计和代码详解)
- 使用MATLAB的平方根无迹卡尔曼滤波器(SR-UKF)的简单、快速、可读的实现.rar
- 使用EKF、IECF和UKF估算非线性预测和测量Matlab代码.rar
- 基于Python语言的OpenSees算例(重点在于Python语言在OpenSees中的应用)
- 使用了随机梯度下降法(SGD)和批量梯度下降法(BGD)解决单层感知机问题Matlab代码.rar
- c语言课程设计-ktv歌曲系统
资源上传下载、课程学习等过程中有任何疑问或建议,欢迎提出宝贵意见哦~我们会及时处理!
点击此处反馈


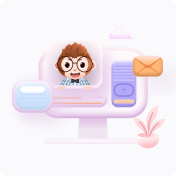