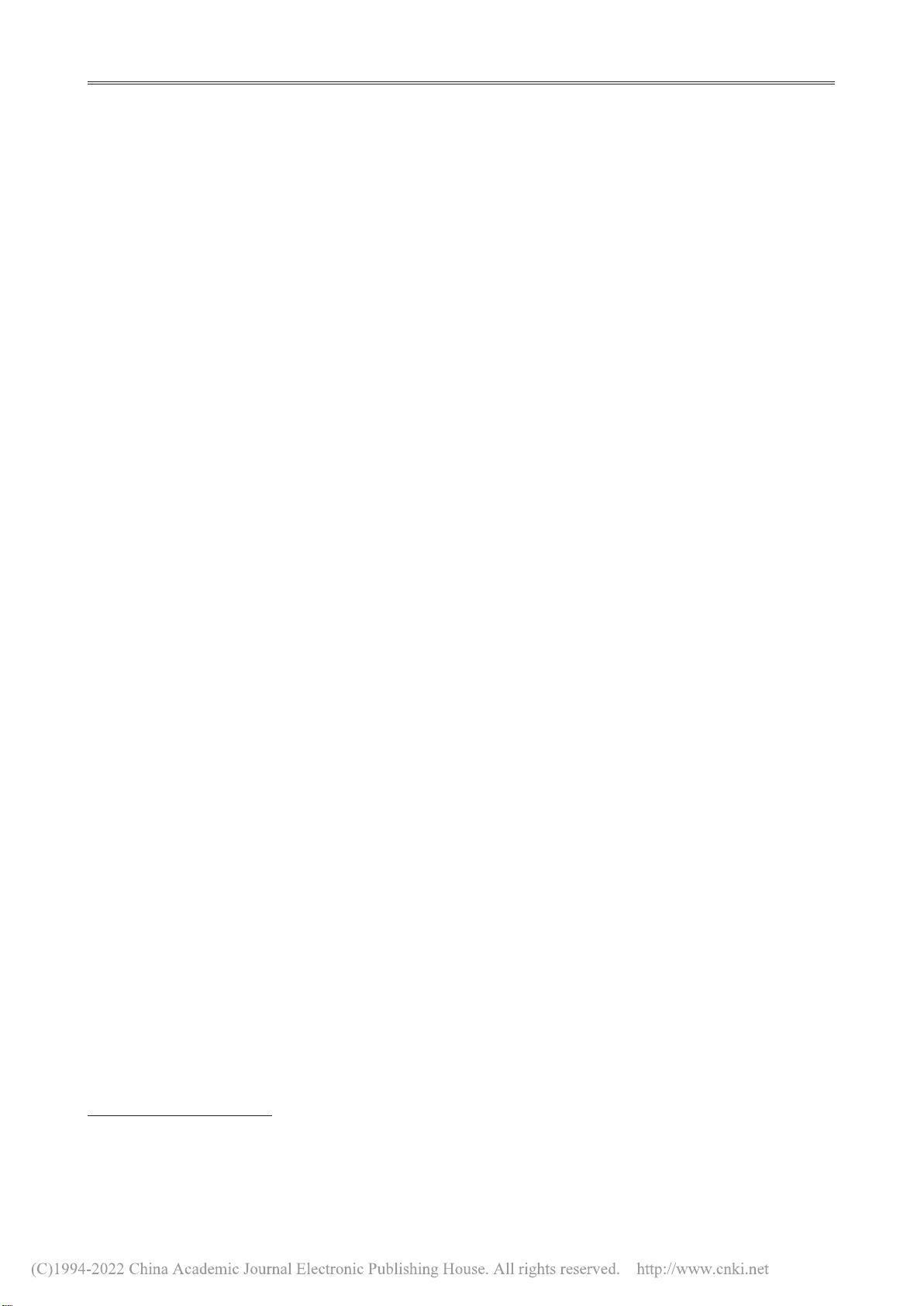
第 54 卷 第 5 期
2 0 2 2 年 5 月
哈 尔 滨 工 业 大 学 学 报
JOURNAL OF HARBIN INSTITUTE OF TECHNOLOGY
Vol. 54 No. 5
May 2022
DOI:10.11918 / 202010082
融合有效方差置信上界的 Q 学习智能干扰决策算法
饶 宁,许 华,宋佰霖
(空军工程大学 信息与导航学院,西安 710077)
摘 要: 为进一步提升基于值函数强化学习的智能干扰决策算法的收敛速度,增强战场决策的有效性,设计了一种融合有效
方差置信上界思想的改进 Q 学习智能通信干扰决策算法。 该算法在 Q 学习算法的框架基础上,利用有效干扰动作的价值方
差设置置信区间,从干扰动作空间中剔除置信度较低的干扰动作,减少干扰方在未知环境中不必要的探索成本,加快其在干
扰动作空间的搜索速度,并同步更新所有干扰动作的价值,进而加速学习最优干扰策略。 通过将干扰决策场景建模为马尔科
夫决策过程进行仿真实验,所构造的干扰实验结果表明:当通信方使用干扰方未知的干扰躲避策略变更通信波道时,与现有
基于强化学习的干扰决策算法相比,该算法在无通信方的先验信息条件下,收敛速度更快,可达到更高的干扰成功率,获得更
大的干扰总收益。 此外,该算法还适用于“多对多”协同对抗环境,可利用动作剔除方法降低联合干扰动作的空间维度,相同
实验条件下,其干扰成功率比传统 Q 学习决策算法高 50%以上。
关键词: 干扰决策;强化学习;有效方差置信上界;Q 学习;干扰动作剔除;马尔科夫决策过程
中图分类号: TN975 文献标志码: A 文章编号: 0367
-
6234(2022)05
-
0162
-
09
Q⁃learning intelligent jamming decision algorithm based on
efficient upper confidence bound variance
RAO Ning, XU Hua, SONG Bailin
(Information and Navigation College, Air Force Engineering University, Xi’an 710077, China)
Abstract: To further improve the convergence speed of the intelligent jamming decision⁃making algorithm based on
value function in reinforcement learning and enhance its effectiveness, an improved Q⁃learning intelligent
communication jamming decision algorithm was designed integrating the efficient upper confidence bound variance.
Based on the framework of Q⁃learning algorithm, the proposed algorithm utilizes the value variance of effective
jamming action to set the confidence interval. It can eliminate the jamming action with low confidence from the
jamming action space, reduce the unnecessary exploration cost in the unknown environment, speed up its searching
speed in the interference action space, and synchronously update the value of all actions, thus accelerating the
optimal strategy learning process. The jamming decision⁃making scenario was modeled as the Markov decision
process for simulation. Results show that when the correspondent used interference avoidance strategy against the
jammer to change the communication channel, the proposed algorithm could achieve faster convergence speed,
higher jamming success rate, and greater total jamming rewards, under the condition of no prior information,
compared with the existing decision⁃making algorithms based on reinforcement learning. Besides, the algorithm
could be applied to the “many⁃to⁃many” cooperative countermeasure environment. The action elimination method
was used to reduce the dimension of joint jamming action, and the jamming success rate of the proposed algorithm
was 50% higher than those of the traditional Q⁃learning decision algorithms under the same conditions.
Keywords: jamming decision⁃making; reinforcement learning; efficient upper confidence bound variance;
Q⁃learning; jamming action elimination; Markov decision process
收稿日期: 2020
-
10
-
26
作者简介: 饶 宁(1997—),男,硕士研究生;
许 华(1976—),男,教授,博士生导师
通信作者: 许 华,13720720010@ 139.com
干扰决策是电子战中进行有效对抗的重要环
节,而人工决策由于实时性与科学性较差,很难满足
战场瞬息万变的态势要求。 随着认知无线电
[1]
等
技术的发展,干扰方要达到较好的干扰效果变得更
加困难,但无线通信媒介自身的开放性给干扰攻击
的实现保留了可行性。 近年来,智能干扰已经成为
认知电子战的一个重要研究领域,涌现出了利用遗
传算法、粒子群算法等
[2
-
3]
实现干扰参数寻优的理
论,但这些方法都需要通信方的先验通信参数。 强
化学习作为不需要先验信息的方法,能在未知环境
中通过不断与环境交互来学习最优行为策略,目前
在通 信 干 扰 与 抗 干 扰 领 域 已 有 初 步 应 用, 如 文