# MDP-DP-RL
The goal of this project was to develop all Dynamic Programming and Reinforcement Learning algorithms
from scratch (i.e., with no use of standard libraries, except for basic numpy and scipy tools). The
"develop from scratch" goal was motivated by educational purposes - students learning this topic
can understand the concepts throroughly only when they develop and work with code developed from
scratch. I teach courses on this topic to a variety of student backgrounds, and each such course
is big on precise programming implementations of the techniques/algorithms. In particular, I
use this codebase when I teach Stanford CME 241: Reinforcement Learning for Stochastic
Control Problems in Finance (http://cme241.stanford.edu).
Any feedback on code readability, performance and bugs will be greatly appreciated as the code
is still fairly raw and untested in various parts (started working on this code in August 2018,
and have mainly been in code-growth mode so far).
The project started by implementing the foundational data structures for finite Markov Processes
(a.k.a. Markov Chains), Markov Reward Processes (MRP), and Markov Decision Processes (MDP). This was followed by
Dynamic Programming (DP) algorithms, where the focus was to represent Bellman equations in clear mathematical
terms within the code. Next was the core educational material of Reinforcement Learning, implementing
the Generalized Policy Iteration algorithms based on simulations (Monte Carlo and Temporal Difference,
including eligibility traces). However, the emphasis was to first implement the tabular methods so that
one can work with actual data structures (finite, hence tabular), rather than functions to represent
MDP rewards and transition specifications as well as value functions and policies. Once the tabular RL
methods were implemented, it was straightforward to write the same algorithms as functional approximation-based
algorithms. However, this required a detour to build some foundation for function approximation. I chose
to implement linear and deep neural network approximations, both of which require a specification of
feature functions. Backpropagation was developed from scratch, again for educational purposes. On a whim,
I also implemented Approximate Dynamic Programming (ADP) Algorithms, which was basically the same old
Policy Iteration and Value Iteration algorithms but now using the output of function approximation for
the right-hand-side of the Bellman update, and using the updated values as training data for gradient
descent on the parameters of the function approximation. So far, I am finding ADP
as the most valuable algorithm for the MDP problems I typically work with. I am a bit surprised that the
"literature" focuses so much on model-free whereas I often know the model for many of the MDPs I work on,
and so, ADP is ideal.
I have chosen Python 3 as the language, mainly because I can't expect my students to have expertise in
the potentially more-appropriate languages for this project, such as Scala, Ocaml and Haskell. These are
functional programming languages and this topic/project is best done through a tasteful application of
Functional Progamming. But Python 3 is not such a bad choice as functions are fast-class entities. My core
technique in this project is indeed Functional Programming, but I had to very careful in getting around
Python's "naughty" handling of function closures. I have also made heavy use of classes and TypeVars.
Object-oriented polymorphism as well as type-parametrized polymorphism enabled me to cover a wide range of
algorithms with plenty of common code. Python 3 also provided me the benefit of type annotations, which I
have taken heavy advantage of in this project. Type annotations support turned out to be extremely valuable
in the project as my IDE (PyCharm) caught a lot of errors/warnings statically, in fact as I was typing code,
it would spot errors. More importantly, type annotations makes the interfaces very clear and I believe any
sort of mathematical programming needs a strong style of type annotations (if not static typing).
This is how the modules of the project are organized.
processes: All about Markov Processes, MRP, MDP and classes that serve as minimal but complete representations
of an MDP for specific classes of algorithms, eg: a representation for tabular RL, a representation for function
approximation RL, and a representation for ADP. A lot of the heavy lifting is done in the "helper" sub-module
mp_funcs.py
func_approx: Linear and Deep-Neural Network (DNN) function approximation. Implements function evaluation (forward
propagation for DNN) and gradient calculation/gradient descent (backward propagation for DNN) using ADAM. Took
advantage of numpy vectors, matrices, tensors and efficiently computing with them.
algorithms: within this, we have the modules dp (for Dynamic Programming), adp (for Approximate Dynamic Programming),
rl_tabular (for Tabular RL - Monte Carlo, SARSA, Q-Learning, Expected SARSA), rl_func_aprprox (for Function
Approximation RL - same algorithms as Tabular RL). Note that I have implemented TD(0) and TD(Lambda) separately
for both Tabular RL and Function Approximation RL, although TD(0) is a special case of TD(Lambda). TD(0) was
implemented separately for the usual reason in the project - that I find it easy to introduce a special case (in
this case TD(0)) for pedagogical reasons and so showing students TD(0) as a special case with simpler/lighter
code that focuses on the concept (versus the complication of eligibility traces) is quite beneficial. This is the
same reason I implemented Tabular (Tabular is a special case of Linear Function Approximation where the features
are indicator functions, one for each of the states/state-action pairs). Note the deep object-oriented inheritance
hiereracy - rooted at the abstract base class OptBase. Note also that a lot of heavy lifting happens in the
module helper_funcs.py. A couple of semi-advanced algorithms such as LSTD/LSPI and Policy Gradient are also implemented here (LSPI provides batch-efficiency and Policy Gradient is valuable when the action space is large/continuous). Some special but highly useful model-based algorithms such as Backward Induction (backward_dp.py) and Adapative Multistage Sampling (ams.py) have also been implemented.
examples: Implemented a few common examples of problems that are ideal for RL: Windy Grid, Inventory Control. For http://cme241.stanford.edu, I have also implemented initial versions of two important and interesting finance problems that can be solved by modeling them as MDPs and solving with DP/RL: 1) Optimal Asset-Allocation and Consumption when managing a portfolio of risky assets and 1 riskless asset, 2) Optimal Exercise of American Options when the option-payoff is either path-dependent or if the state space of the option is high-dimensional.
utils: Some generic utility functions to transform data structures.
没有合适的资源?快使用搜索试试~ 我知道了~
MDP-DP-RL:马尔可夫决策过程,动态规划和强化学习
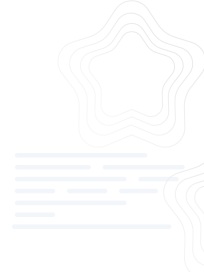
共93个文件
py:89个
ipynb:2个
gitignore:1个


温馨提示
MDP-DP-RL 该项目的目标是从头开始开发所有动态编程和强化学习算法(即,除了基本的numpy和scipy工具之外,不使用标准库)。 “从头开始开发”目标是出于教育目的-学习此主题的学生只有在他们开发和使用从头开始开发的代码时才能彻底理解这些概念。 我针对不同的学生背景讲授了该主题的课程,每门此类课程都以技巧/算法的精确编程实现为基础。 特别是,当我教Stanford CME 241:金融中的随机控制问题的强化学习( )时,会使用此代码库。 关于代码可读性,性能和错误的任何反馈将不胜感激,因为代码仍相当原始且未经各个部分的测试(2018年8月开始使用此代码,到目前为止主要处于代码增长模式)。 该项目开始于为有限的马尔可夫过程(又名马尔可夫链),马尔可夫奖励过程(MRP)和马尔可夫决策过程(MDP)实现基础数据结构。 其次是动态编程(DP)算法,其重点是在代码内以清晰的数学术语表
资源详情
资源评论
资源推荐
收起资源包目录




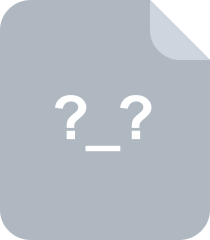
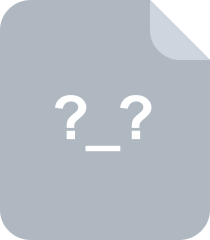
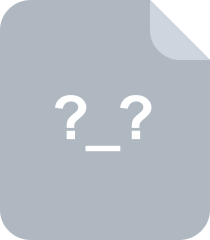
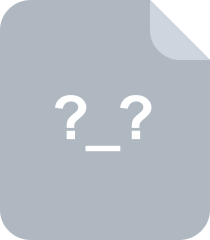
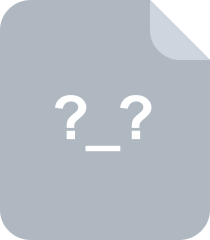
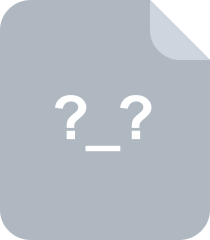
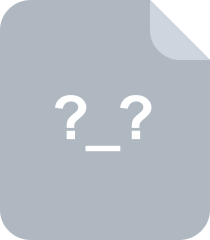
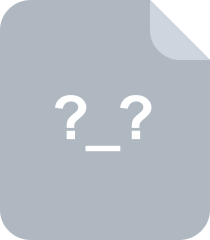
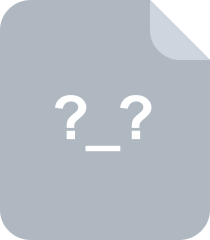
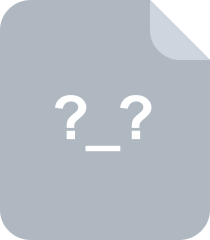
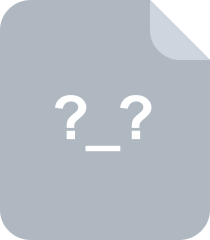
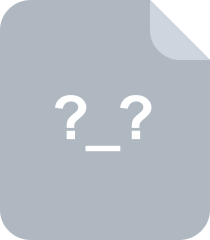
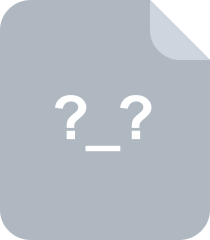
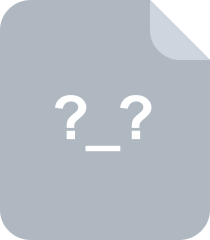
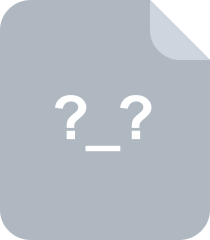

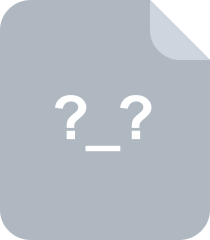
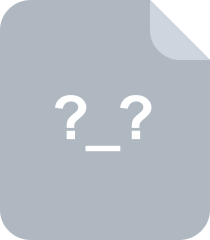
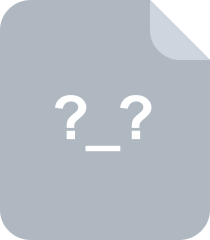
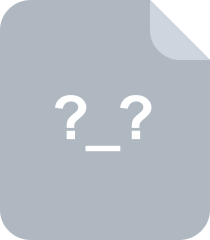
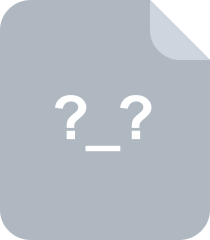
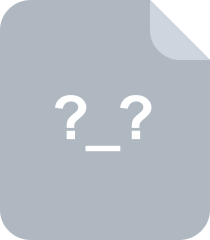

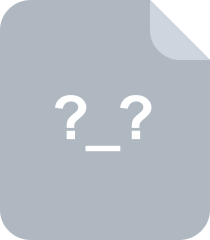
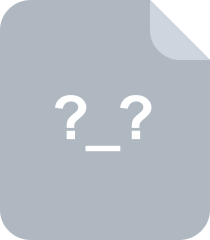

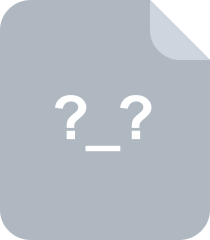
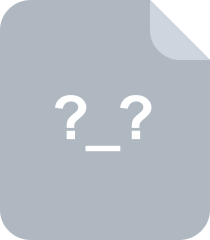

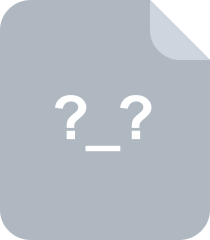
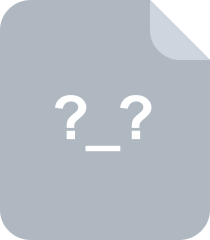
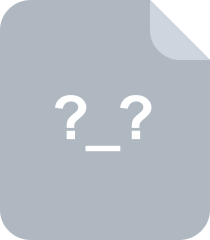
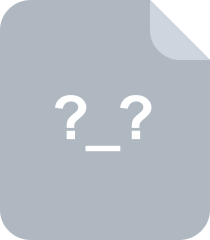
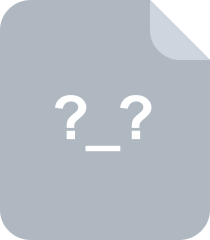
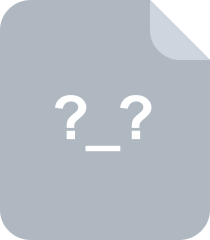

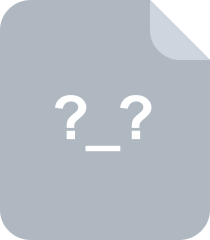
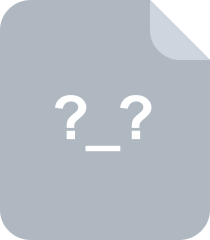
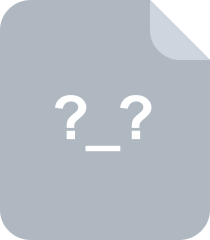
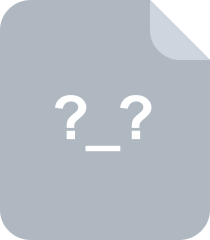
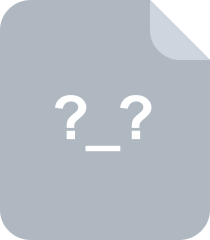

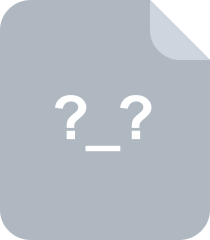
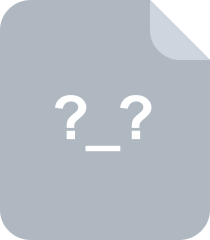
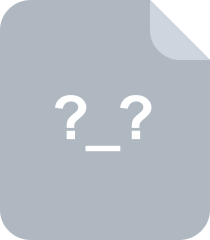
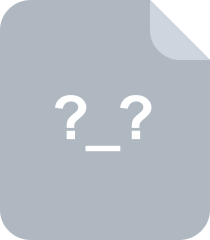
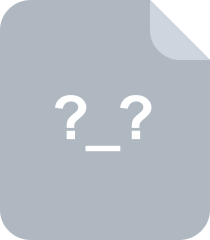

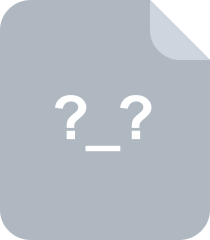
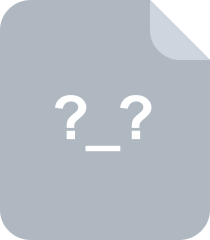
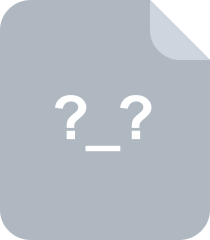
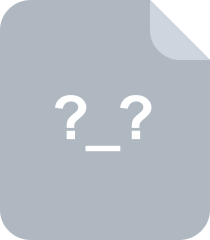
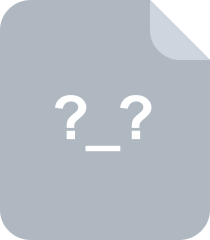
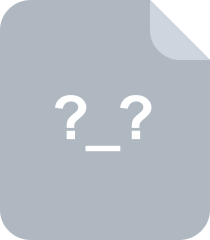

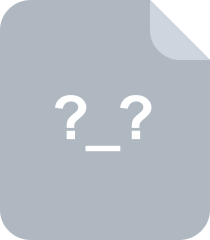
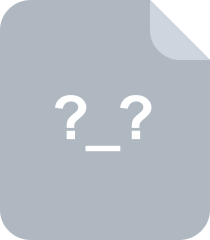
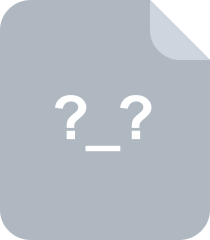

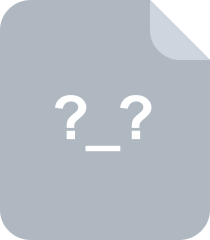
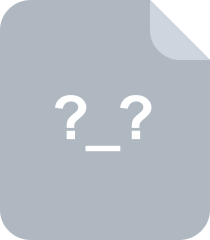
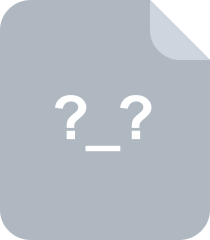
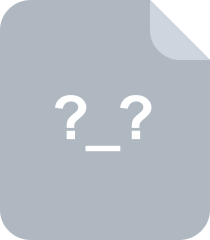
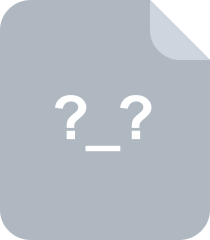
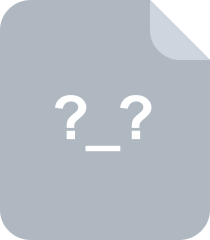
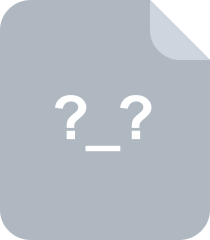
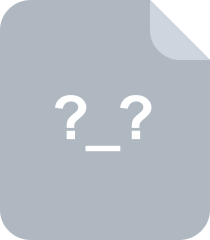
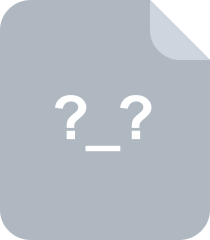

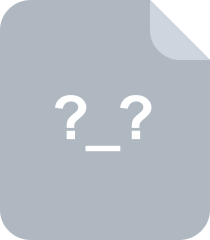
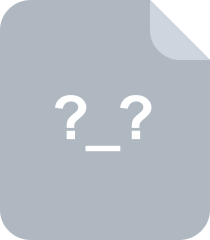
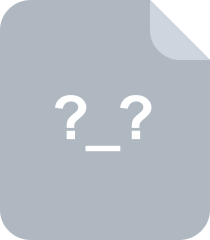
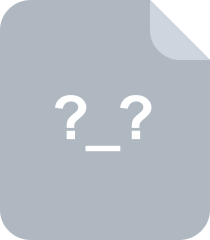
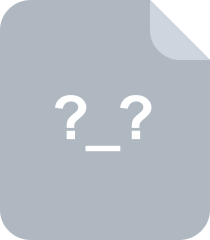
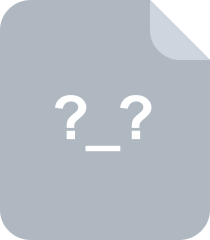
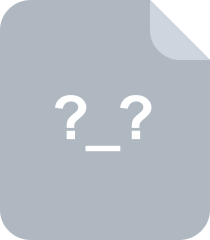

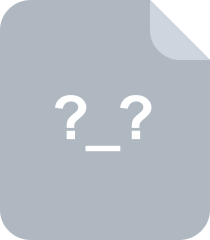
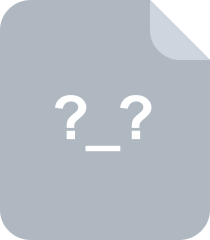
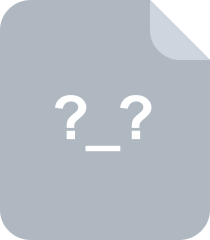
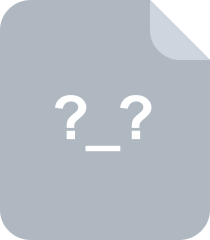
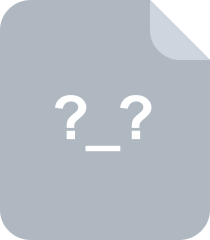
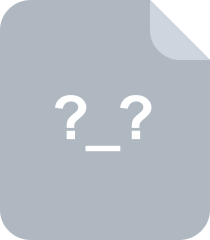

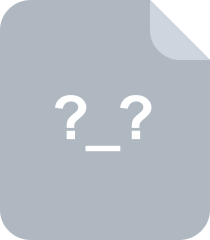
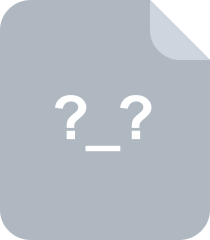
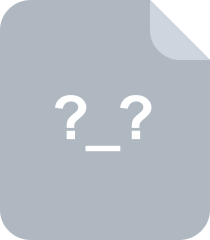
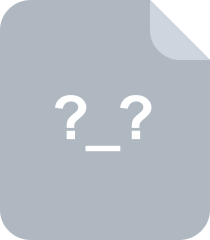
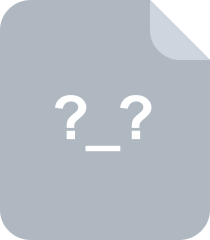
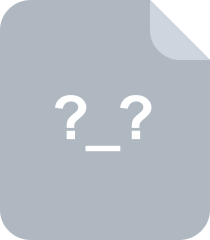
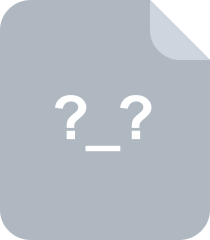

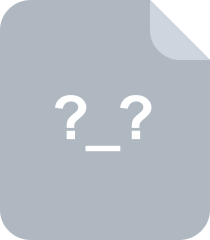
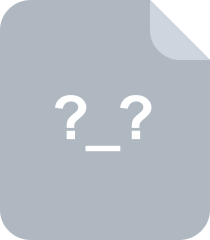
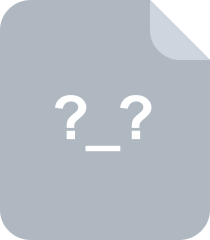
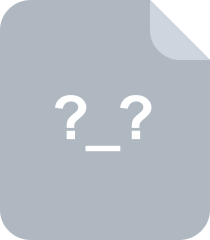

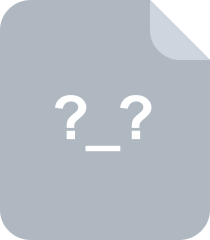
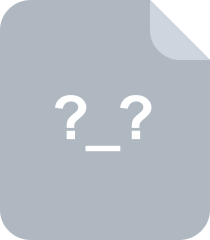
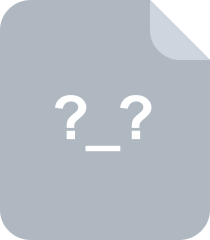

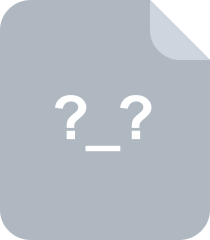
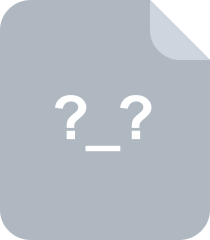
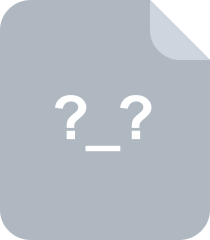
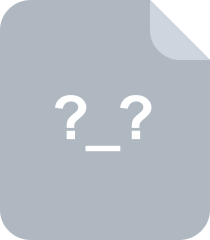
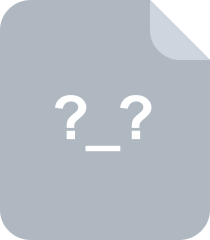
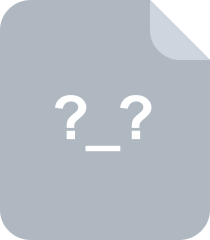
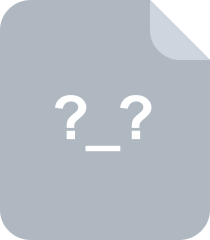
共 93 条
- 1
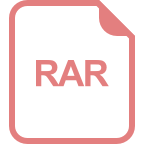
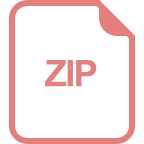
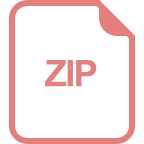
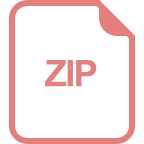
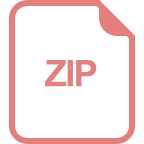
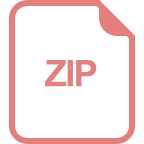
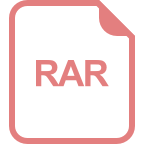
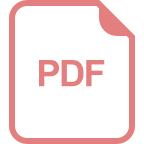
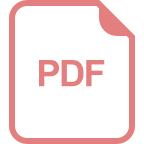
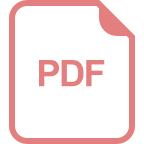
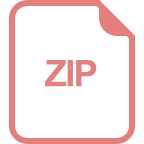
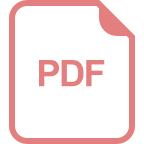
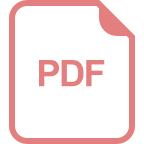
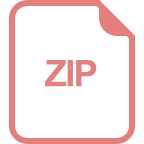
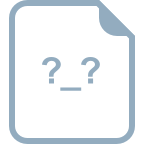
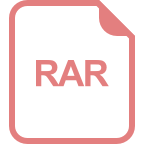
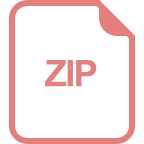

无分别
- 粉丝: 26
- 资源: 4574
上传资源 快速赚钱
我的内容管理 展开
我的资源 快来上传第一个资源
我的收益
登录查看自己的收益我的积分 登录查看自己的积分
我的C币 登录后查看C币余额
我的收藏
我的下载
下载帮助

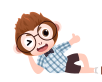
最新资源
- C语言-leetcode题解之70-climbing-stairs.c
- C语言-leetcode题解之68-text-justification.c
- C语言-leetcode题解之66-plus-one.c
- C语言-leetcode题解之64-minimum-path-sum.c
- C语言-leetcode题解之63-unique-paths-ii.c
- C语言-leetcode题解之62-unique-paths.c
- C语言-leetcode题解之61-rotate-list.c
- C语言-leetcode题解之59-spiral-matrix-ii.c
- C语言-leetcode题解之58-length-of-last-word.c
- 计算机编程课程设计基础教程
资源上传下载、课程学习等过程中有任何疑问或建议,欢迎提出宝贵意见哦~我们会及时处理!
点击此处反馈


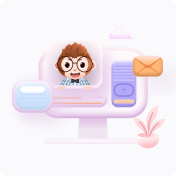
安全验证
文档复制为VIP权益,开通VIP直接复制

评论1