没有合适的资源?快使用搜索试试~ 我知道了~
Combining clustering and classification for remote-sensing image...
0 下载量 142 浏览量
2021-02-09
20:09:37
上传
评论
收藏 216KB PDF 举报
温馨提示
A joint clustering and classification approach is proposed. This approach exploits unlabeled data for efficient clustering, which is applied in the classification with support vector machine (SVM) in the case of small-size training samples. The proposed method requires no prior information on data labels, and yields better cluster structures. Through cluster assumption and the notions of support vectors, the most confident k cluster centers and data points near the cluster boundaries are labeled
资源推荐
资源详情
资源评论
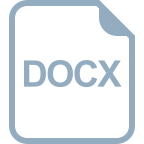
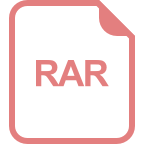
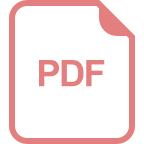
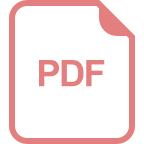
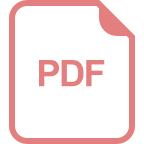
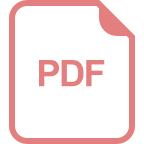
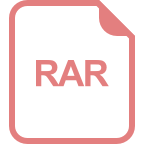
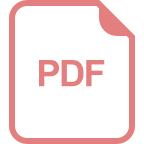
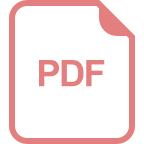
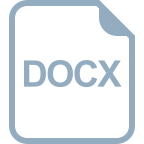
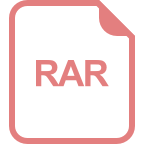
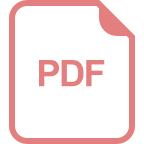
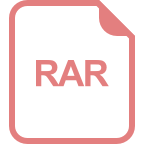
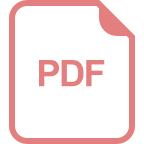
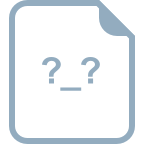
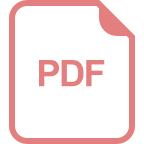
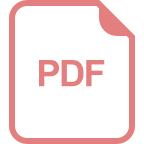
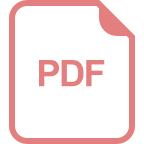
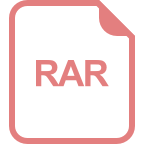
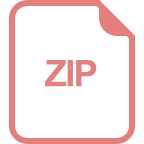
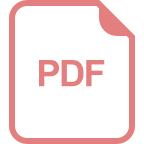
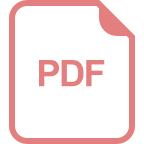
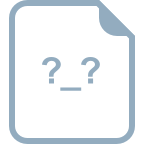
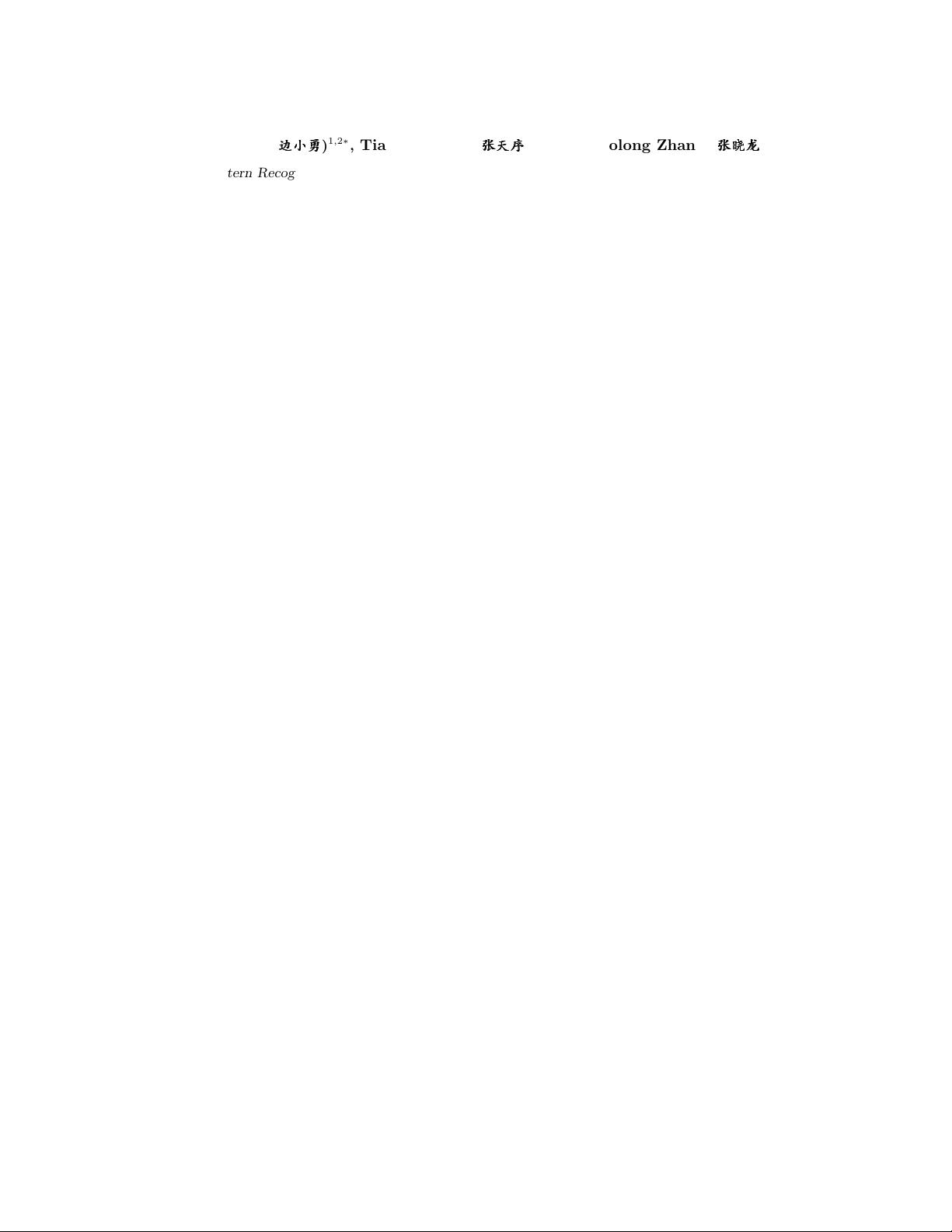
January 10, 2011 / Vol. 9, No. 1 / CHINESE OPTICS LETTERS 011002-1
Combining clustering and classification for remote-sensing
images using unlabel ed data
Xiaoyong Bian (
>>>
℄℄℄
)
1,2∗
, Tianxu Zhang (
ÜÜÜ
UUU
SSS
)
1
, and Xiaolong Zhang (
ÜÜÜ
¡¡¡
999
)
2
1
Institute for Pattern Recognition and Artificial Intelligence, Huazhong University of Science and Technology,
Wuhan 430074, China
2
School of Computer Science and Technology, Wuhan University of Science and Technology, Wuh an 430081, China
∗
Corresponding author: xyjconf100@163.com
Received August 12, 2010; accepted October 28, 2010; posted online January 1, 2011
A joint clustering and classification app roach is proposed. This approach exploits unlabeled data for
efficient clustering, which is applied in the classification with support vector machine (SVM) in the case
of small-size training samples. The proposed method requires n o prior information on data labels, and
yields better cluster structures. Through cluster assumption and the notions of support vectors, the most
confident k cluster centers and data points near the cluster boundaries are labeled and used to train
a reliable SVM classifier. Our method gains better estimation of d ata distributions and mitigates the
unrepresentative problem of small-size training samples. The data set collected from Landsat Thematic
Mapper (Landsat TM-5) validates the effectiveness of the proposed approach.
OCIS codes: 100.3008, 100.5010.
doi: 10.3788/COL201109.011002.
Clustering and classification techniques have bee n widely
studied and applied in the fields of information pro-
cessing, data analysis, and computer vision, among
others
[1,2]
. Clus tering in the form of unsupervised meth-
ods involves partitioning the unlabeled data points into
disjoint subsets (clusters) based on the underlying struc-
ture of the data. One of the most widely used clustering
algorithms is k- means and its variation
[3]
. For the su-
pervised classification methods, most existing algorithms
require sufficient training samples to train a reliable clas-
sifier and aim to generalize well on new data points.
However, the general supervision information provided
by pairwise constraints or label information is often un-
available in certain application domains
[4,5]
.
To the best of our knowledge, abundant unlabeled data
available in remote-s e nsing images have hardly been fully
used in the classification process over the past decade,
even if they should be more representative and can be
exploited to enhance c lassification tasks. This charac-
teristic has recently motivated an increasing number of
research interests in the semi-supervised learning (SSL)
paradigm, which aims to improve learning performance
by incorporating unlabeled data into the learning pro-
cess. Therefore, combining clustering and classification
techniques to the analys is of remote-sensing images has
attracted great attention. It has b e e n shown in Ref. [4]
that semi-supervised clustering is guided by pairwise con-
straints pr ovided by the user, thus this method is not
always accurate. Chi et al. carried out directly in the
primal representation the optimization problems on sup-
port vector machine (SVM) for the classification of hy-
perspectral remote-sensing data, with the computation
complexity of O(nd
2
+ d
3
) when n ≪ d, where n is the
labeled samples and d is the fea tur e s
[5]
. As in all super-
vised learning methods, this alternative implementation
technique requires the manual selection of training data.
In Ref. [6], binary transductive SVM (TSVM) was pro-
posed to class ify multi-class hyper spectr al remote-sensing
images using transductive samples, which may result in
a nonconvex optimization problem. In addition, a recent
effort in Ref. [7] employed k Gaussian mixture mod-
els constrained by labeled samples to estimate the data
distribution, and the reported classification acc uracy in
each training data set was generally lower than 91% even
when there were hundreds of training samples per class.
All these methods explicitly use pairwise cons traints or
label information to guide clus tering and classification
learning.
One of the two major issues related to remote-sensing
image classification problems is that labeled data are of-
ten difficult to be obtained and very sparse in practical
applications. Another critical issue is that the training
samples are often c ollected from the sa me area of the
scene reg ardless of the variation of spectral signatures of
land cover classes in the spatial domain and fail to es-
timate the distributions for the entire data. Both prob-
lems result in the risk of overfitting the tra ining samples
and may involve poor generalizatio n capabilities in the
classifier
[6,7]
.
In this letter, a novel approach combining clustering
and classification techniques is proposed to handle the
unrepresentative problem of small-size training samples
for SVM classification. We propose to import appropri-
ately unlabeled data through the clustering method to
the classification of r e mote-sensing images; bipartition-
based k-means (BKM) is utilized to better estimate k
initial centers, and the confidently clustered data can
then be generated. Through cluster ass umption
[8]
and
the notions of convex hulls and reduced ellipse-like struc-
tures of the cluster regions
[9]
, the confident data points
from different cluster re gions can be further evaluated,
extracted, and labele d as training samples; subsequently,
SVM can be trained with the labeled data. The use of
clustering prior to classification appro ach is a natural and
practical choice because the labeled data may be unavail-
able. In contrast to Ref. [3], our version of cluster ing
1671-7694/2011/011002(4)
c
2011 Chinese Optics Letters
资源评论
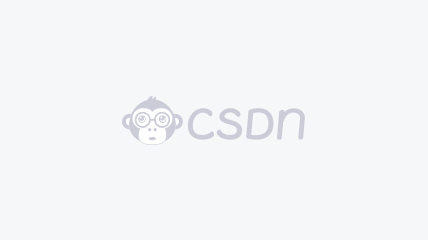

weixin_38701407
- 粉丝: 5
- 资源: 917
上传资源 快速赚钱
我的内容管理 展开
我的资源 快来上传第一个资源
我的收益
登录查看自己的收益我的积分 登录查看自己的积分
我的C币 登录后查看C币余额
我的收藏
我的下载
下载帮助

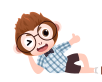
最新资源
资源上传下载、课程学习等过程中有任何疑问或建议,欢迎提出宝贵意见哦~我们会及时处理!
点击此处反馈


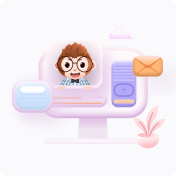
安全验证
文档复制为VIP权益,开通VIP直接复制
