没有合适的资源?快使用搜索试试~ 我知道了~
Quantile regression and its empirical likelihood with missing re...
2 下载量 196 浏览量
2020-03-02
16:35:46
上传
评论
收藏 174KB PDF 举报
温馨提示
随机丢失下的分位数回归及其经验似然,沈煜,梁汉营,本文研究反映变量随机丢失下的线性分位数回归模型. 基于逆概率权方法,我们建立了分位数回归的估计方程并定义未知参数的分位数回归
资源推荐
资源详情
资源评论
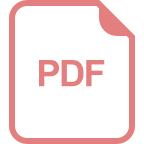
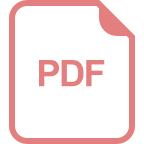
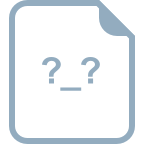
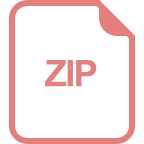
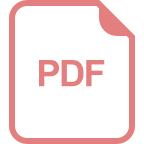
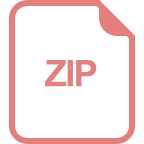
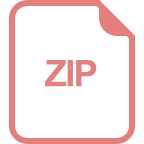
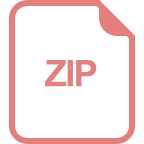
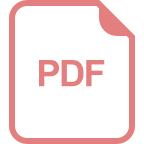
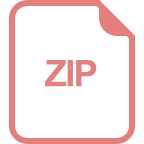
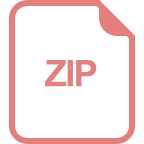
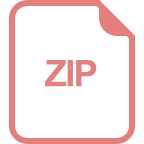
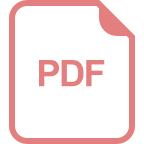
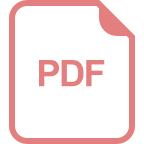
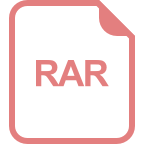
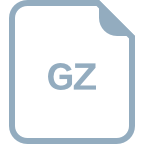
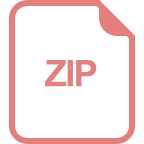
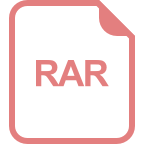
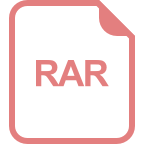
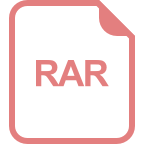
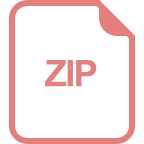
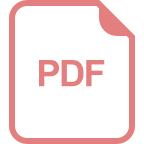
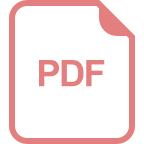
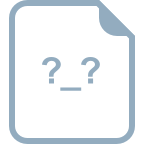
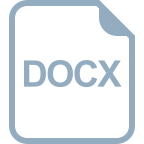
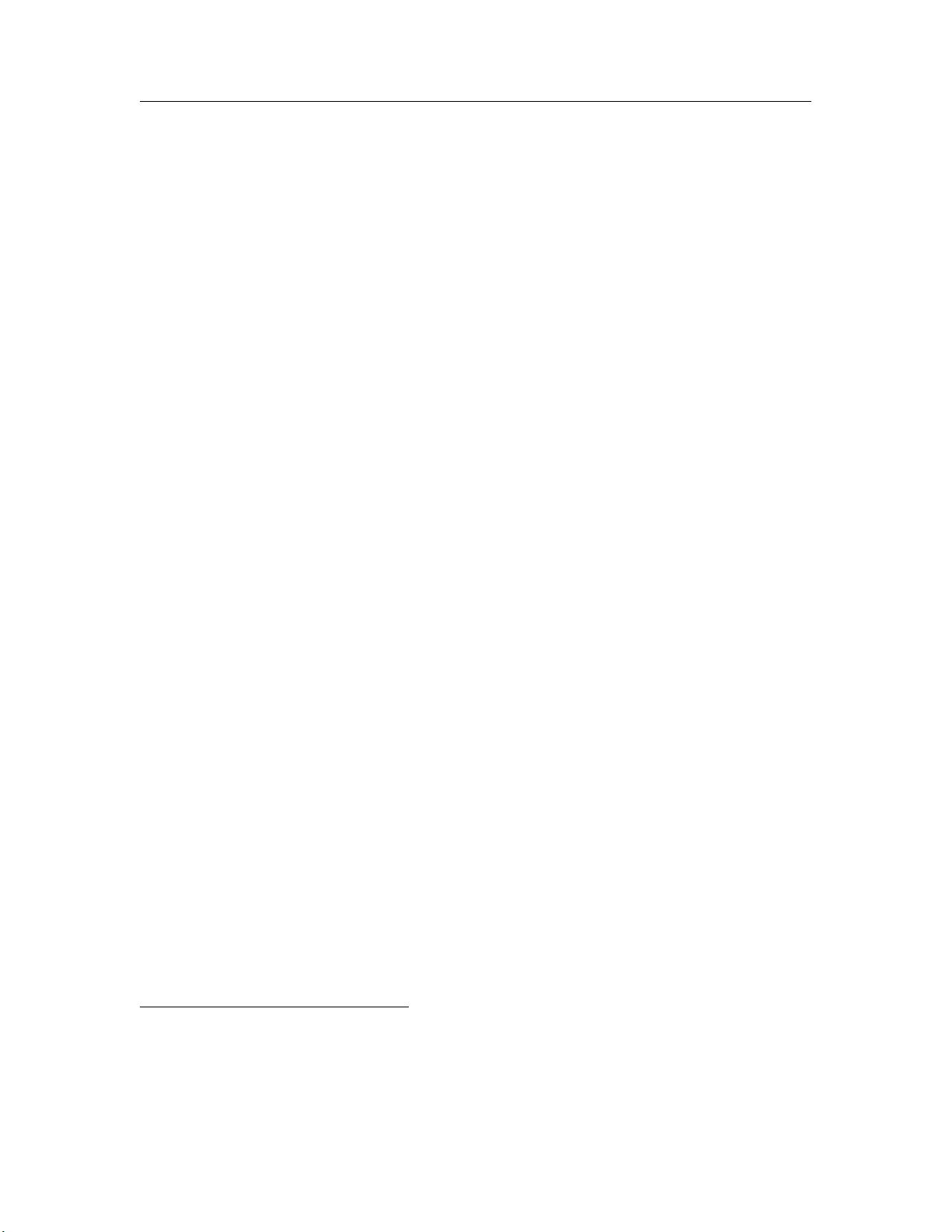
˖ڍመڙጲ
http://www.paper.edu.cn
随机丢失下的分位数回归及其经验似然
沈煜,梁汉营
同济大学数学系,上海 200092
摘要:本文研究反映变量随机丢失下的线性分位数回归模型. 基于逆概率权方法,我们建立了
分位数回归的估计方程并定义未知参数的分位数回归估计量,同时我构造出未知参数的经验似
然比函数,由此定义参数的极大经验似然估计量. 在适当的条件下,我们研究了构造估计量的
渐近正态性,证明经验似然比统计量具有标准的 χ
2
极限分布.
关键词:渐近正态性,经验似然,极大经验似然,随机丢失,分位数回归
中图分类号: O212.7
Quantile regression and its empirical likelihood
with missing response at random
SHEN Yu, LIANG Han-Ying
Department of Mathematics, Tongji University, Shanghai 200092
Abstract: In this paper, we study the linear quantile regression model when response data
are missing at random. Based on the inverse probability weight, we establish an estimation
equation on quantile regression and define the quantile regression estimator of unknown
parameter. At the same time, we construct the empirical likelihood (EL) ratio function for
the unknown parameter, and define the maximum EL estimator of the unknown parameter.
Under suitable assumptions, we investigate the asymptotic normality of the proposed
estimators and prove the EL ratio statistics has a standard chi-squared limiting distribution.
Key words: Asymptotic normality, Empirical likelihood, Maximum empirical likelihood
estimation, Missing at random, Quantile regression
0 Introduction
Quantile regression (QR) introduced by Koenker and Bassett
[1]
has become an increasingly
important tool in statistical analysis. Unlike the mean regression method that relies only on
the central tendency of the data, the QR approach allows the analyst to estimate the functional
dependence between variables for all portions of the conditional distribution of the response
variable. In other words, QR extends the framework of estimating only the behavior of the
基金项目: National Natural Science Foundation of China (11271286),Specialized Research Fund for the Doctor Program
of Higher Education of China (20120072110007)
作者简介: SHEN Yu(1990-),male,Ph.D. of Tongji University,major research direction:nonparametric statistical
analysis. Correspondence author:LIANG Han-Ying(1964-),male,professor,major research direction:nonparametric
statistical analysis.
- 1 -
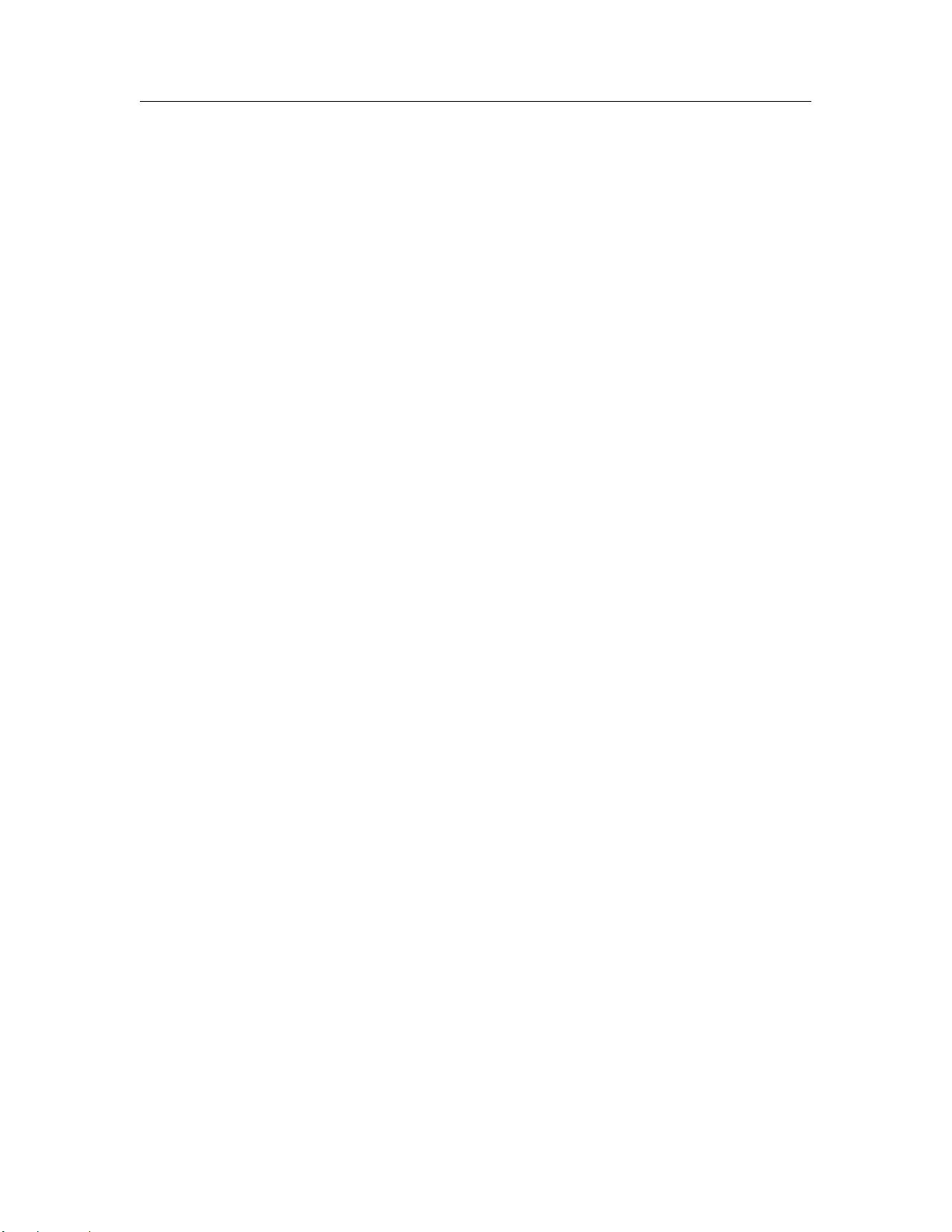
˖ڍመڙጲ
http://www.paper.edu.cn
central part of a cloud of data points onto all parts of the conditional distribution. In that
sense QR provides a more complete view of relationships between variables of interest. A
comprehensive review can be found in Koenker
[2]
.
Missing data are common in opinion survey, medical research, experiment science and other
applied fields. In many practical situations, missing response may occur due to happenstance
or design. For example, in a opinion doll some individuals may refuse to supply personal
information, the responses are so expensive to get that only part of them are available in
an experiment. A simple approach to deal with missing response data is complete-case (CC)
analysis which deletes all incomplete data. However, it has an important drawback that it will
cause biased estimation when the data is not missing completely at random. For more details,
see Little and Rubin
[3]
. Most of the existing literature with missing data have been interested
in the conditional mean of the response given the covariates in regression models. One method
to handle missing data is imputation. Healy and Westmacott
[4]
discussed a linear regression,
Wang and Sun
[5]
developed a semiparametric imputation in partially linear models, Chen and
Van Keilegom
[6]
studied a nonparametric imputation. See also Robins et al.
[7]
, Zhou et al.
[8]
and M¨uller and Van Keilegom
[9]
. The other popular one is the inverse probability weighted
method, such as, Wang et al.
[10]
with missing covariates, and Wang et al.
[11]
, Wang and Sun
[5]
with missing response. Although the missing data analysis has a long history in statistics, most
literature on QR does not contain missing data problems. This is mainly caused by the reason
that the estimator for QR parameters is not linear in responses. In this paper, we focus on the
QR model with missing response at random.
Consider the linear QR model
Y
i
= X
T
i
β
0
+ ϵ
i
, i = 1, 2, . . . , n, (1)
where Y
i
are response variables, X
i
are p ×1 random vectors of covariates, β
0
∈ R
p
is unknown
parameter and ϵ
i
are random errors whose τ th conditional quantile given X
i
equals zero for
τ ∈ (0, 1). Suppose (Y
i
, X
i
, δ
i
) is an iid sample of incomplete observations from (X, Y, δ), where
all the X
i
’s are observed, and δ
i
= 0 if Y
i
is missing, δ
i
= 1 otherwise. Throughout this paper,
we make the missing at random (MAR) assumption. The MAR assumption means that δ
i
and
Y
i
is conditionally independent given X
i
, that is, P (δ
i
= 1|Y
i
, X
i
) = P (δ
i
= 1|X
i
) := ∆
0
(X
i
).
Such missing mechanism is usually used for statistical analysis with missing response data and
is reasonable in many practical problems, see Little and Rubin
[3]
. There are many papers that
analyzed real data examples under MAR situations, such as Zhang and Zhu
[12]
, Liang et al.
[13]
,
Ciuperca
[14]
, Karimi and Mohammadzadeh
[15]
and so on. Imputation is a common method
for managing missing data under MAR. Yi and He
[16]
studied median regression model for
longitudinal data with dropouts where the covariates are known at all time but the response
may be missing from a certain time point. Wei et al.
[17]
investigated a multiple imputation
for managing missing covariates. Sherwood et al.
[18]
adopted the inverse probability weighted
- 2 -
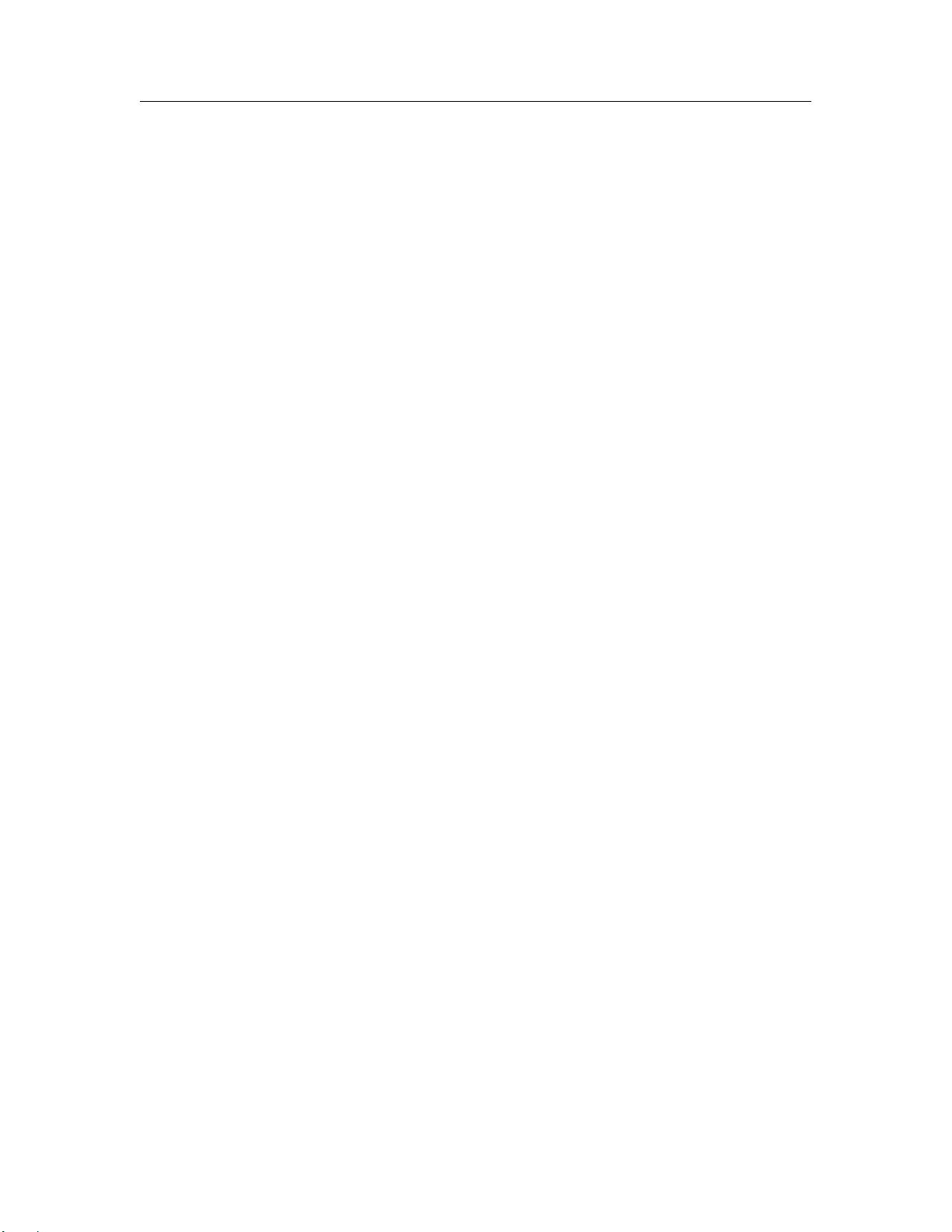
˖ڍመڙጲ
http://www.paper.edu.cn
method to develop weighted quantile regression with missing covariates.
For constructing confidence regions, the empirical likelihood (EL) introduced by Owen
[19]
is an attractive method. The EL method shares the merits of parametric likelihood and is more
robust due to its nonparametric feature, see Owen
[20]
for a comprehensive overview, see also
Zhao et al.
[21]
, Fan et al.
[22]
, Zheng et al.
[23]
and Wei et al.
[24]
. Some authors have discussed EL
quantile regression. For example, Whang
[25]
developed a smoothed EL for QR model. Tang and
Leng
[26]
applied EL for QR model with auxiliary information, see also Qin and Tsao
[27]
, Otsu
[28]
and Wang and Zhu
[29]
. For missing data, to our best knowledge, only Lv and Li
[30]
investigated
the EL quantile regression, but they did not adopt imputation or inverse probability weighted
method.
In this paper, we consider the linear QR model (1) with MAR responses. Based on the
inverse probability weight, we establish an estimation equation on quantile regression and give
standard quantile regression estimator of β
0
. At the same time, we construct the EL ratio
function for β, and define the maximum EL estimator of β
0
. Under suitable assumptions, we
establish the asymptotic normality of the proposed estimators and prove the EL ratio statistics
has a standard chi-squared limiting distribution. A simulation study is done to investigate the
finite sample performance of the proposed method.
The rest of this paper is organized as follows. In Section 1, we define the estimators and
the empirical log-likelihood function. Main results are described in Section 2. Proofs of main
results are put in Section 3. Proofs of some lemmas, which are used in the proofs of the main
results, are given in Section 4.
1 Estimators
First, we briefly review the quantile regression with complete data. Let
Q
Y
i
(τ|X
i
) = inf{y : F
0
(y|X
i
) ≥ τ }
be the τth conditional quantile of Y
i
given X
i
, where F
0
(y|X
i
) is the conditional distribution
function of Y
i
given X
i
. Model (1) implies that Q
Y
i
(τ|X
i
) = X
T
i
β
0
. Therefore,
β
0
can be
estimated by minimizing the quantile objective function
Q
n
(β) = n
−1
n
i=1
ρ
τ
(Y
i
− X
T
i
β), (2)
where ρ
τ
(t) = t[τ − I(t < 0)] and I(·) is the indicator function. In view of the definition of
subgradient (see Koenker
[2]
, the minimizer of (2) also satisfies that
n
−1
n
i=1
X
i
τ − I(Y
i
− X
T
i
β < 0)
≈ 0. (3)
- 3 -
剩余15页未读,继续阅读
资源评论
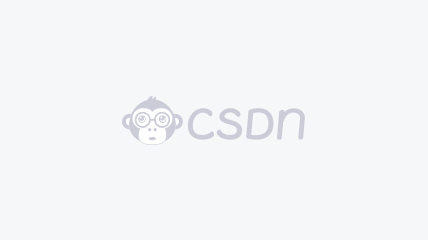

weixin_38518885
- 粉丝: 8
- 资源: 942
上传资源 快速赚钱
我的内容管理 展开
我的资源 快来上传第一个资源
我的收益
登录查看自己的收益我的积分 登录查看自己的积分
我的C币 登录后查看C币余额
我的收藏
我的下载
下载帮助

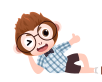
最新资源
资源上传下载、课程学习等过程中有任何疑问或建议,欢迎提出宝贵意见哦~我们会及时处理!
点击此处反馈


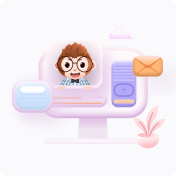
安全验证
文档复制为VIP权益,开通VIP直接复制
