《基于文本分类的游客满意度分析》是一篇深入探讨如何运用现代信息技术进行游客满意度评估的研究文章。随着“互联网+旅游”模式的兴起,游客在网上留下的评论已成为衡量旅游服务质量的重要依据。通过对这些评论进行文本分析,可以为景区规划、旅游管理等提供科学的数据支持。 文章首先介绍了研究背景和意义,强调了在信息化时代,利用文本挖掘技术提升旅游服务质量的重要性。文献综述部分,作者回顾了文本分类、LDA主题模型等相关领域的研究进展,为后续的方法选择和实证分析奠定了基础。 在相关理论和技术部分,文章详细阐述了中文文本情感分类的过程,包括文本预处理(如清洗、分词、去除停用词)以及数据的统计特征描述。接着,作者介绍了LDA(Latent Dirichlet Allocation)主题模型,这是一种用于发现文本隐藏主题的统计建模方法,能够从大量文本中抽取出关键主题。通过LDA,作者在4000条游客评论中识别出了预定服务、旅游景点、旅游体验和导游评价四大主题,并提取出与这些主题相关的特征词。 在实证研究阶段,文章使用Python编程语言构建了多种机器学习和深度学习模型,包括朴素贝叶斯、K近邻、随机森林、决策树、逻辑回归、支持向量机和卷积神经网络。通过比较各种模型的准确率、召回率和F1值,得出卷积神经网络在游客满意度分类中的表现最优,而K近邻分类效果最不理想。这表明深度学习模型在特征提取上的优势,它能从更多角度和维度捕捉信息,减少高频无意义词汇对分类结果的影响。 作者提出深度学习模型相对于传统机器学习模型的优势,并开发了名为“旅评”的旅客满意度评价分析网站,提供直观且便捷的旅游数据分析服务。所有代码和原始数据都上传至GitHub,推动了数据分析的实践应用,为旅游行业及相关管理部门提供了新的数据获取和分析途径。 这篇研究揭示了如何运用现代信息技术,尤其是机器学习和深度学习,来提升旅游行业的服务质量。通过文本分类和LDA主题模型,可以从海量评论中提炼出有价值的信息,为旅游业的决策提供数据支持。同时,这一研究也为未来文本分析工作提供了参考和启示。
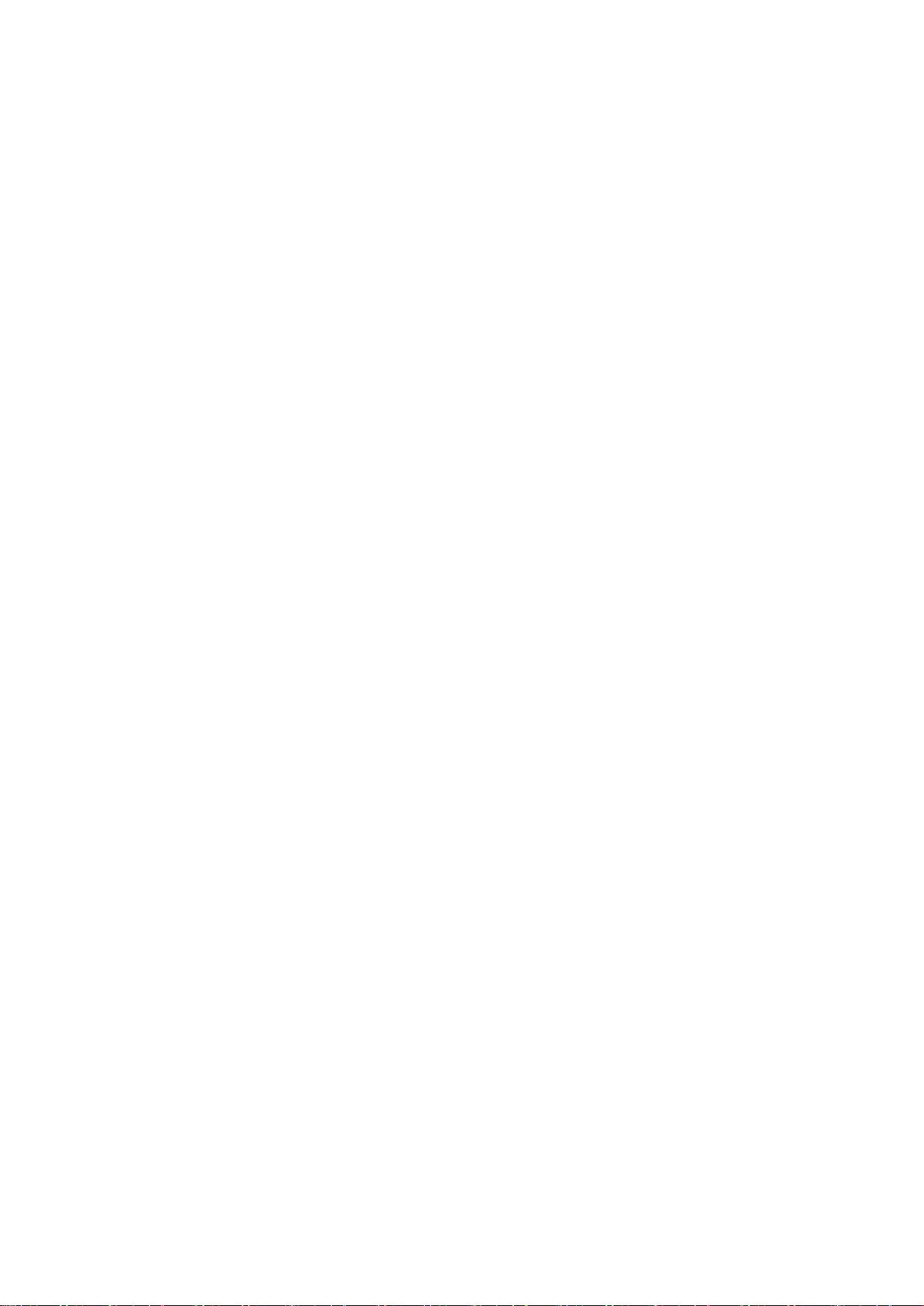
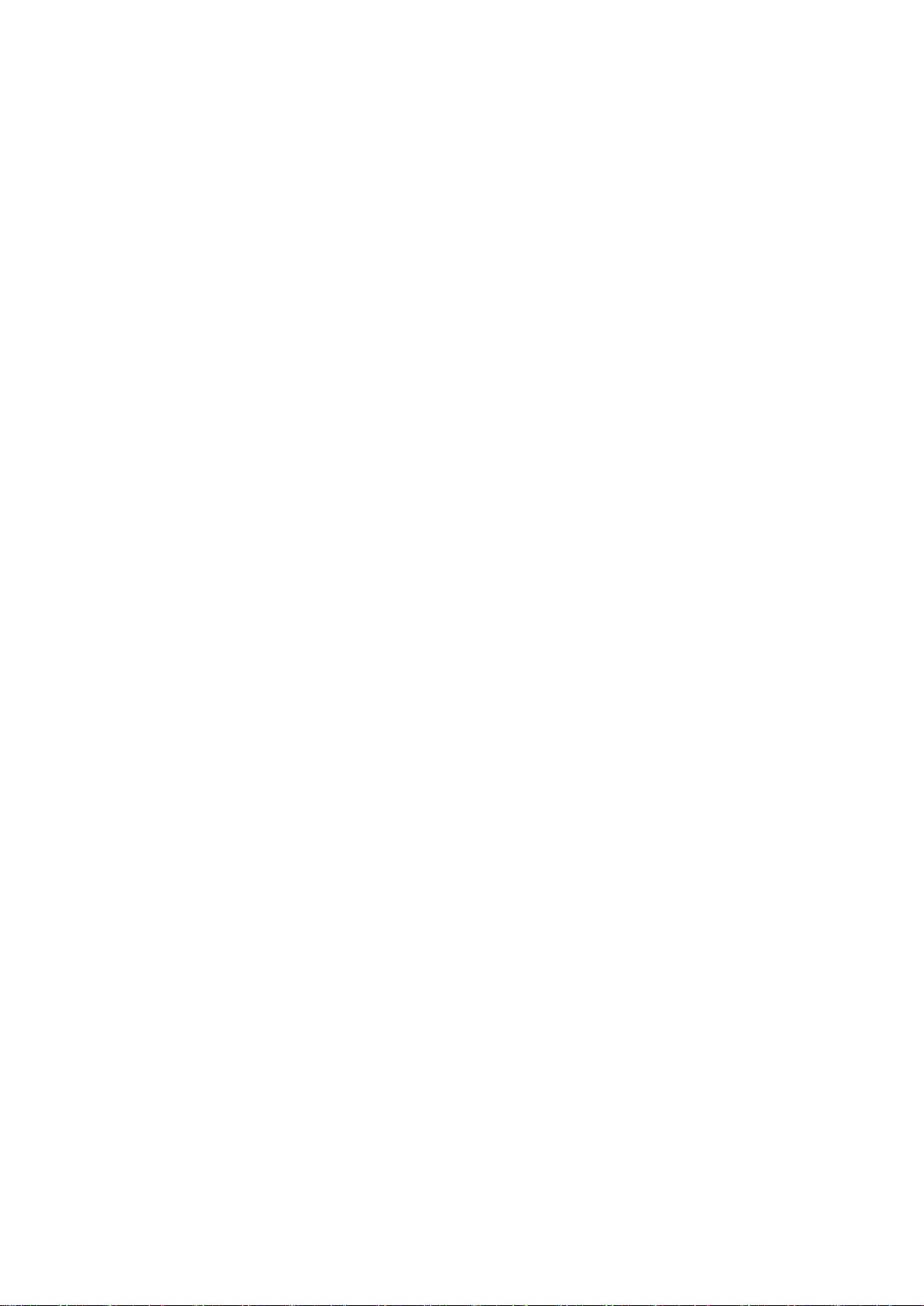
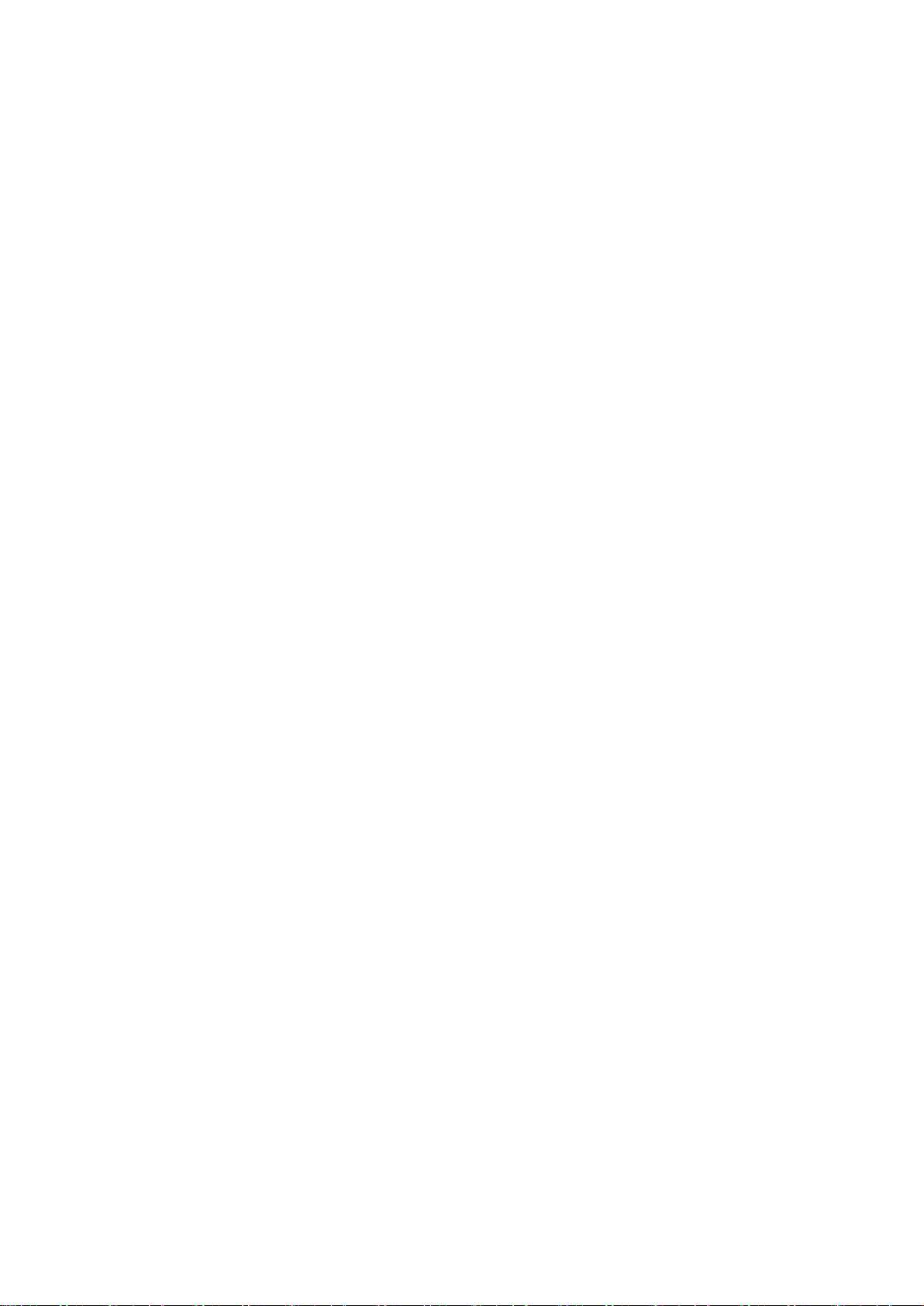
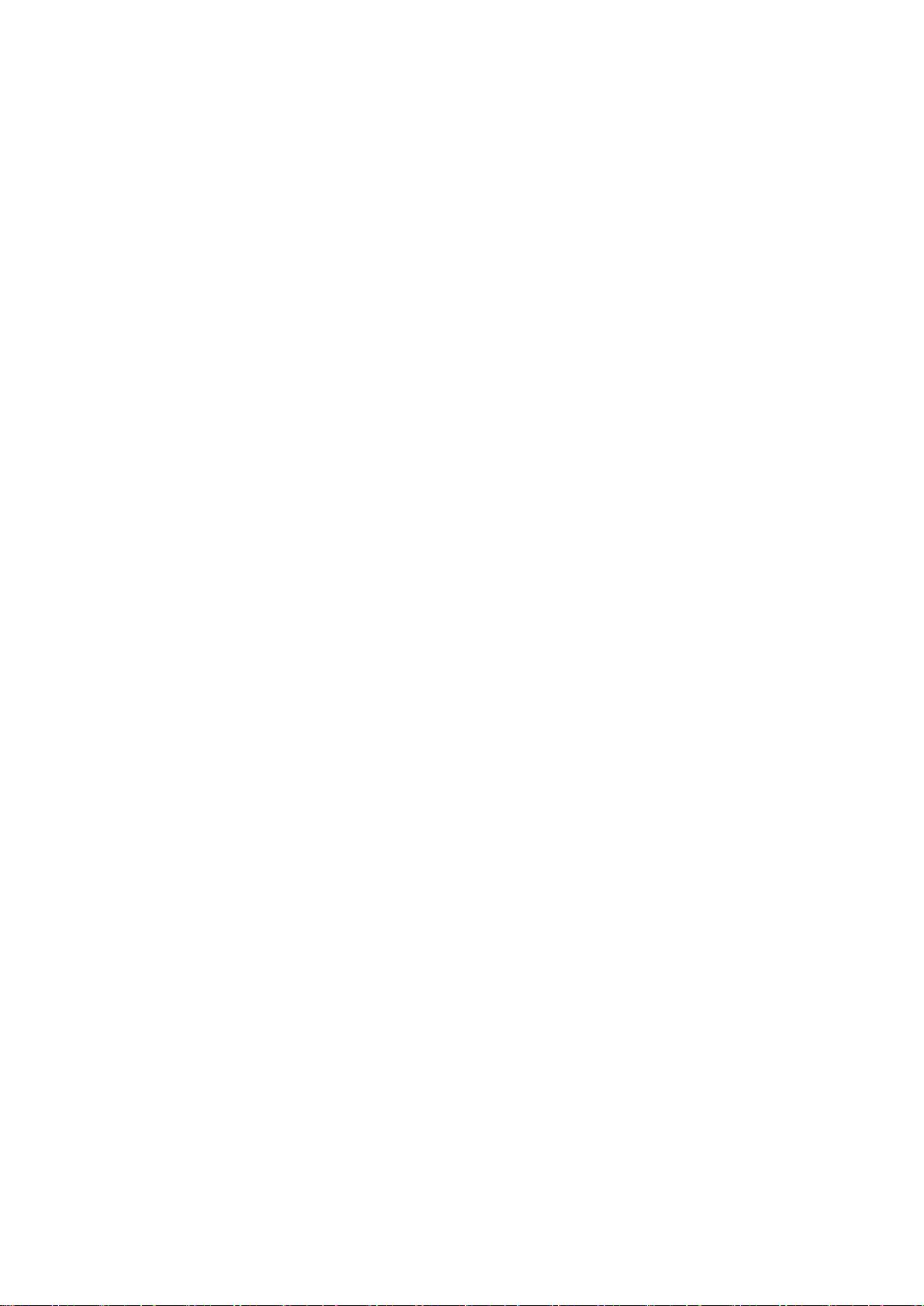
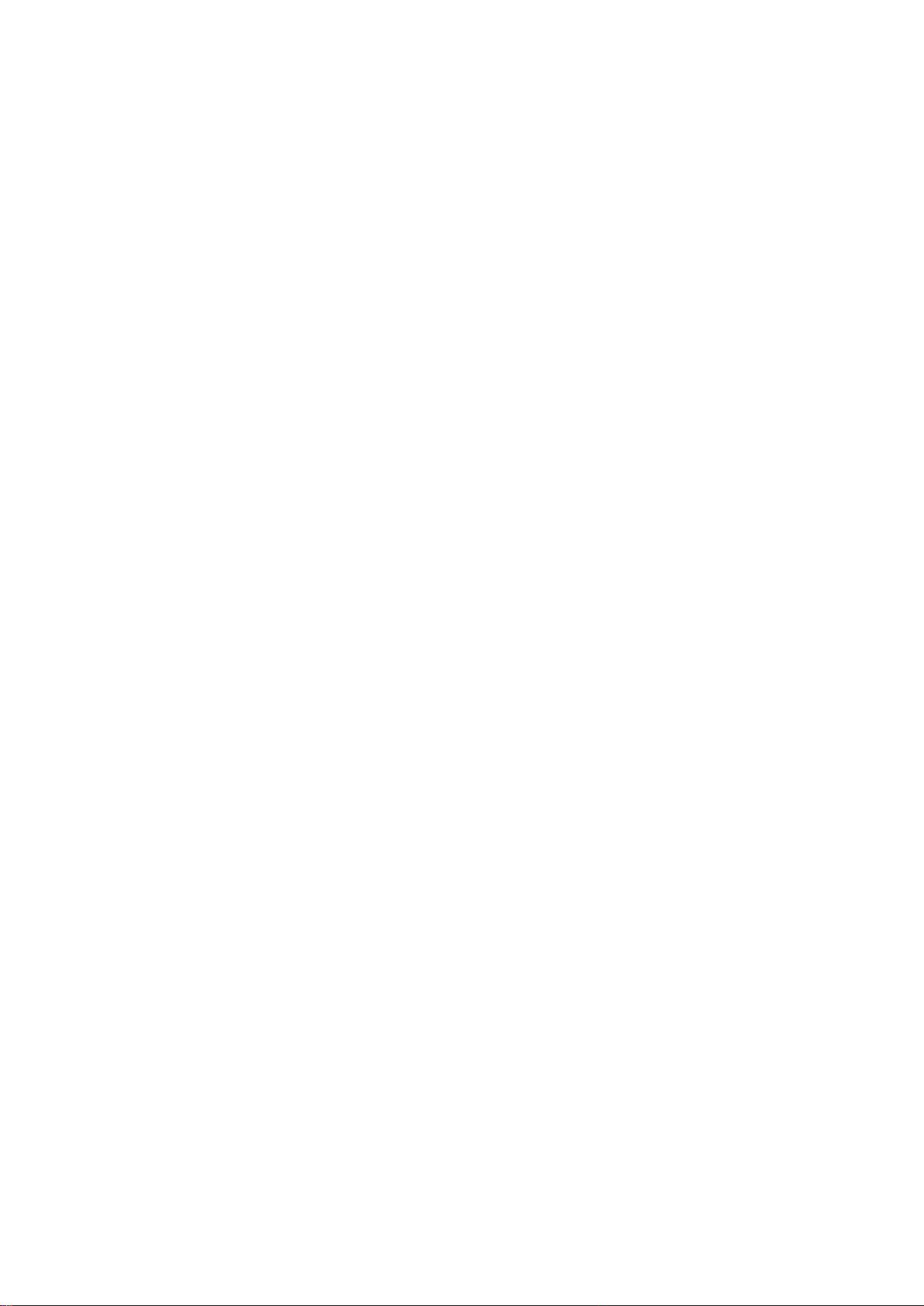
剩余43页未读,继续阅读
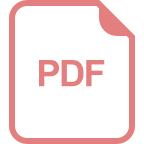
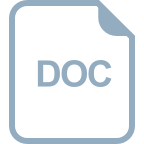
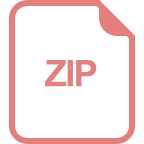
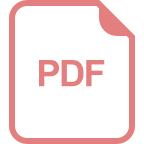
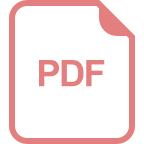
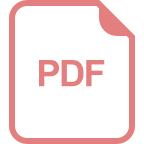
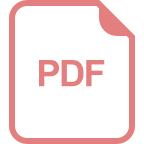
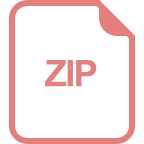
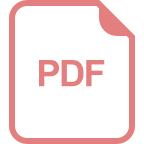
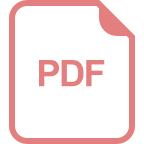
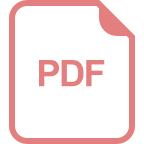
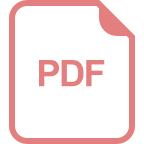
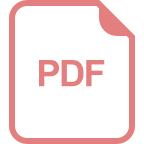
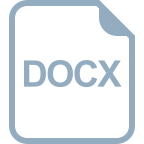
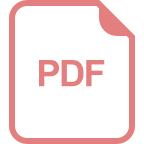
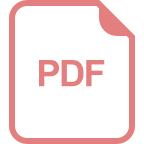
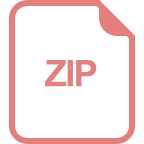
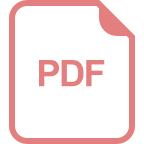
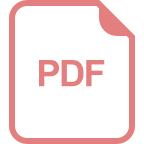
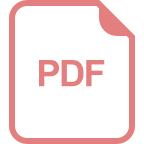
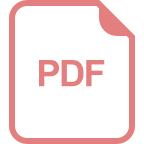
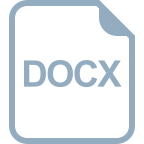
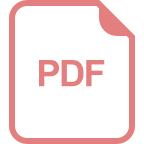
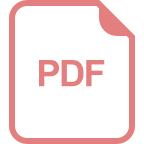
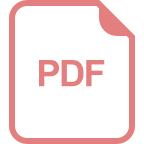
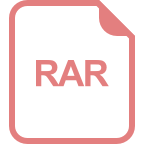
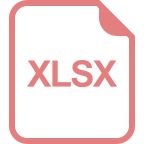

- 粉丝: 734
- 资源: 358
我的内容管理 展开
我的资源 快来上传第一个资源
我的收益
登录查看自己的收益我的积分 登录查看自己的积分
我的C币 登录后查看C币余额
我的收藏
我的下载
下载帮助

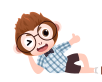
最新资源
- springboot项目志同道合交友网站.zip
- springboot项目在线考试系统.zip
- springboot项目在线互动学习网站设计.zip
- springboot项目制造装备物联及生产管理ERP系统.zip
- springboot项目智慧校园之家长子系统.zip
- springboot项目中国陕西民俗网.zip
- RISCV GD32VF103 中断向量模式以及非向量模式
- 基于Rust语言的快速异步与多路复用Redis驱动设计源码
- 基于Vue的教程:学生课业帮扶系统前端设计源码
- 基于JavaScript的在线中国象棋对战平台设计源码
- 基于Lua语言的ESP32嵌入式系统开源设计源码
- 基于Vue的云盘前端设计源码
- 自动驾驶控制-车辆三自由度动力学MPC跟踪双移线 matlab和simulink联合仿真,基于车辆三自由度动力学模型的mpc跟踪双移线
- 分布式驱动汽车稳定性控制 采用分层式直接横摆力矩控制,上层滑模控制,下层基于轮胎滑移率最优分配 滑模控制跟踪横摆角速度和质心侧偏角误差 七自由度整车模型输出实际质心侧偏角和横摆角速度,二自由度模
- 基于Vue.js框架的旅游舆情分析项目设计源码
- 基于TypeScript的轻量级JavaScript点阵库设计源码

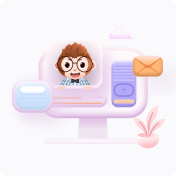

评论0