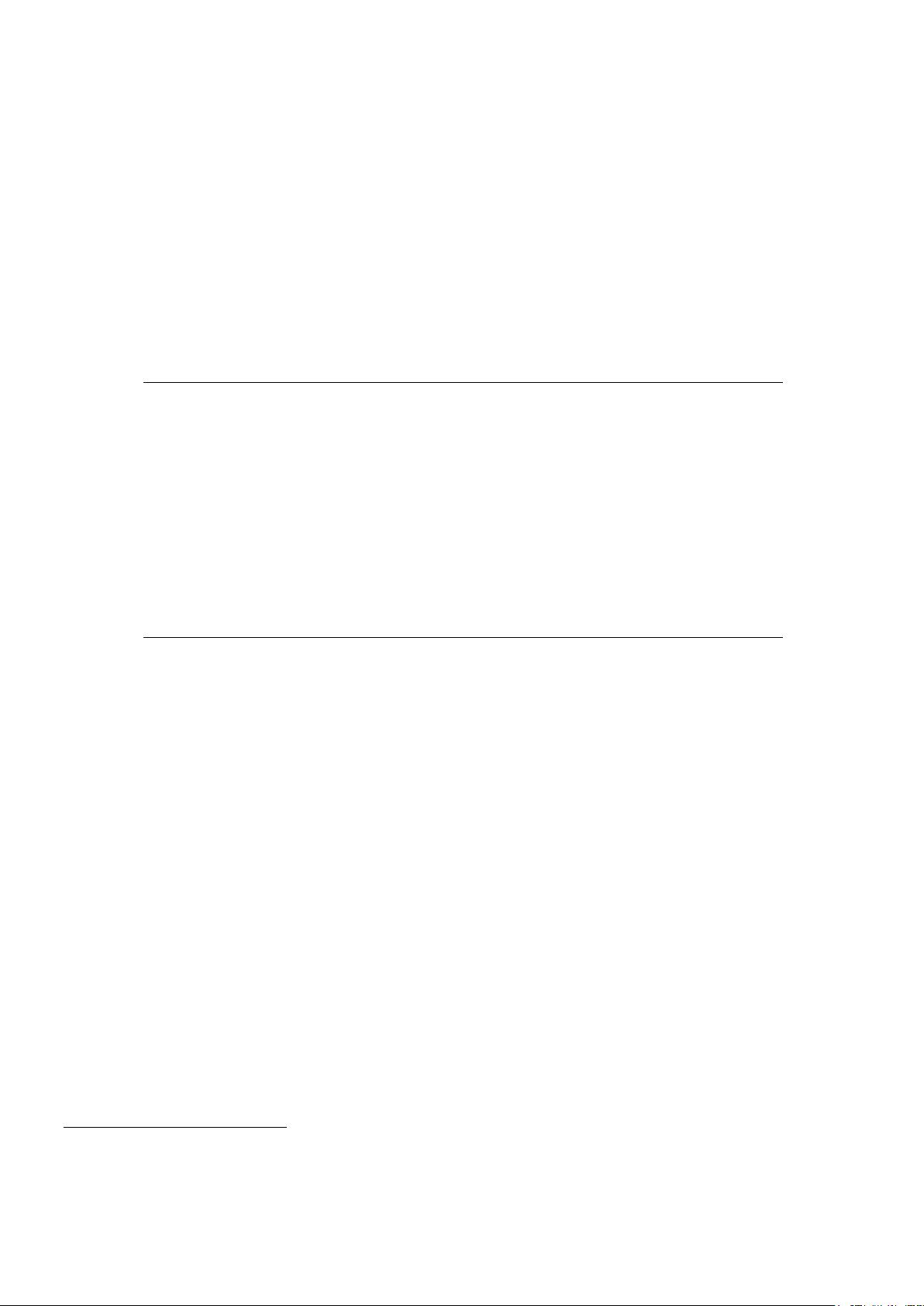
A Canonical Variate Analysis based Process
Monitoring Scheme and Benchmark Study
Zhiwen Chen
∗
, Kai Zhang
∗
, Haiyang Hao
∗
, Steven X. Ding
∗
Minjia Krueger
∗
, Zhangming He
∗, ∗∗
∗
Institute for Automatic Control and Complex Systems, University of
Duisburg-Essen, Bismarckstrasse 81 BB, 47057 Duisburg, Germany
(e-mail: zhiwen.chen@uni-due.de).
∗∗
College of Science, National University of Defense Tech., Yanwachi
47, 410073 Changsha, China
Abstract: Principal component analysis (PCA) and Partial least square (PLS) are powerful
multivariate statistical tools that have been successfully applied for process monitoring. They
are efficient in dimension reduction and are suitable for processing large amount of data.
Nevertheless, their application scope is restricted to static processes where the dynamics are
ignored. In order to achieve improved monitoring performance for dynamic processes, in this
paper, we propose an effective dynamic monitoring scheme based on the canonical variate
analysis (CVA) technique. Different from the standard PCA- and PLS-based techniques which
rely on mean-extraction for residual generation, the proposed CVA-based scheme takes process
dynamics into account as well. The properties of all three methods are then compared in detail
and finally, the improvements of the proposed method are demonstrated on the well-accepted
Tennessee Eastman benchmark process.
Keywords: Canonical variate analysis, principal component analysis, dynamic process
monitoring, fault detection.
1. INTRODUCTION
Process monitoring and fault diagnosis techniques have
been applied in many areas, such as steel industrial, chem-
ical engineering, electronic engineering and so on. As a
major part of those methods, multivariate statistical pro-
cess monitoring methods (MSPM) have attracted much at-
tention, like principal component analysis (PCA), partial
least square (PLS) and canonical variate analysis (CVA)
[MacGregor et al., 1995, Qin, 2003, Ding et al., 2013]. PCA
is characterized with simplicity and efficiency in processing
huge amount of correlated process data. Typically, a few of
principal components are extracted from highly correlated
process data, and proper test statistics are established
for pro cess monitoring purpose. When using two blocks
of data, PLS aims to find combinations of variables that
are highly correlated. Meanwhile, it selects those linear
combinations in a way that eliminates redundancies in the
data blocks and defines a new set of variables in each block,
which are uncorrelated. A limitation of PCA- and PLS-
based approaches is that they rely on static property and a
prior assumption is that the observations are uncorrelated
in time [Hu et al., 2012, Yin et al., 2012]. By using time-
lagged variables to develop dynamic model, Ku et al. [1995]
proposed a dynamic PCA to deal with this issue.
The CVA technique was originally developed by Hotelling
[1933], it was called canonical correlation analysis. Thanks
1
Zhiwen Chen, Kai Zhang and Zhangming He appreciate the
founding support from China Scholarship Council(CSC); Chen also
appreciates the partial support from the Natural Science Foundation
of China (No.61273159).
to Akaike and Larimore’s work, CVA method has become a
main tool in system identification [Akaike, 1974, Larimore,
1983]. A model identified employing CVA can develop
several methods for process monitoring. Negiz and Cinar
[1997] proposed a T
2
statistic based on estimated state
variables for a milk pasteurization process. Russell et al.
[2000] studied a residual generation based on state vari-
ables to measure the variations outside the state space. In
order to detect process changes, Juricek et al. [2004] pro-
posed a local approach based on CVA. Further applications
of CVA for process monitoring can be found in [Simoglou
et al., 2002, Odiowei et al., 2010]. We notice that little
attentions have been focused on the residual generation
based on canonical pair. The objective of this paper is
to address residual generation based on canonical pair for
dynamic process monitoring. According to the generated
residual vector, the complete process monitoring scheme is
designed. In order to verify that the proposed CVA-based
scheme can provide improved performance, a comparison
between the PCA, PLS and CVA monitoring schemes is
carried out.
The rest of this paper is organized as follows. In Section 2,
some preliminaries on static process monitoring are firstly
given. Motivated by the state of the art and industri-
al requirements, we then formulated the problem to be
solved. In Section 3, the CVA-based process description
and monitoring are proposed. A brief comparison between
PCA, PLS and CVA is also done. The comparison results
among them on Tennessee Eastman process are shown in
Section 4. The paper ends with conclusions in Section 5.
Preprints of the 19th World Congress
The International Federation of Automatic Control
Cape Town, South Africa. August 24-29, 2014
Copyright © 2014 IFAC 10634