A nonlinear inverse scale space method for a convex multiplicati...
这篇文章的标题是《一种凸多变量噪声模型的非线性反尺度空间方法》,描述指出这是一篇关于图像复原算法的研究,特别是基于TV(Total Variation,全变分)正则化的去噪模型,旨在有效去除图像噪声。从这些信息中,我们可以提炼出以下几个关键知识点: 1. 图像复原与去噪 图像复原是图像处理中的一个重要领域,目的是尽可能恢复出质量更高、噪声更少的图像。去噪是图像复原的一个子领域,专注于从图像中移除或减少噪声成分,以提高图像质量。图像噪声通常是由成像设备、传输过程中的干扰或环境因素造成的。 2. TV正则化模型 TV正则化是一种广泛应用于图像处理中的方法,尤其是在图像去噪领域。它的核心思想是将图像看作是二维或三维信号的变分问题,并通过最小化图像的全变分来求解,其中全变分度量了图像像素或体素值的变化。TV正则化能够保留图像的边缘信息,因此在去除噪声的同时尽量减少边缘模糊。 3. 凸多变量噪声模型 凸多变量噪声模型是指在噪声模型中,噪声项具有凸性质,这使得优化问题的解具有较好的全局唯一性。文章中提到的多变量噪声模型和早期的加性噪声模型不同,其特点是噪声是乘性的,与图像信号相乘,而非简单相加。这种模型更符合一些实际图像信号处理场景,比如在遥感图像处理中,噪声往往与图像信号的亮度成正比。 4. 非线性反尺度空间方法 尺度空间理论是图像处理中的一种理论框架,用于表征图像的多尺度特性。非线性反尺度空间方法是一种用于图像处理的数学技术,它基于尺度空间理论,通过逐步去除图像信号的细节特征来达到去噪的目的。文章中提出的非线性反尺度空间方法能够有效地处理凸多变量噪声模型,并展示了其在去噪上的优越性能。 5. Bregman距离 Bregman距离是一种用于优化问题的数学度量,用于衡量解的迭代过程中目标函数值的变化。在本文中,研究者展示了所提出的非线性反尺度空间方法与Bregman距离的关系,这有助于理解算法在去除噪声的同时如何维护图像的全局结构。 6. 收敛性与正则化效应 文章中证明了提出的方法对于多变量噪声模型的收敛性,并讨论了其正则化效应。这意味着算法不仅能够有效去除噪声,还能保证图像的质量不会因去噪过程而过度退化。 从以上知识点可以看出,这篇文章是在图像处理领域的深入研究,它不仅回顾了现有的一些方法,并提出了一种新的针对多变量噪声模型的TV正则化框架。通过数值实验验证了新方法在去除噪声方面的优异性能和对早期多变量噪声模型的显著改进。此外,研究者还探讨了算法的参数依赖性,为实际应用提供了理论依据和指导。
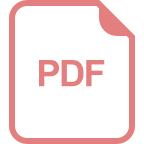
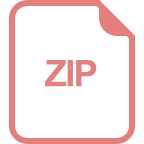
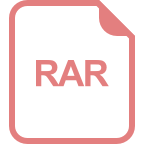
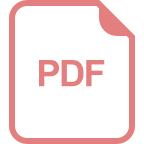
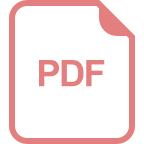
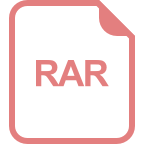
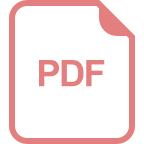
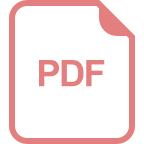
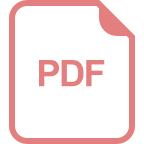
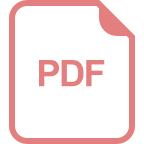
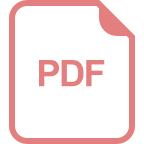
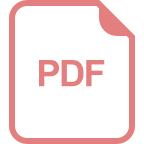
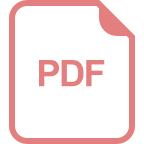
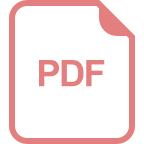
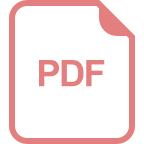
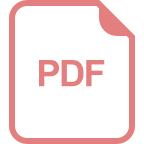
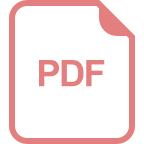
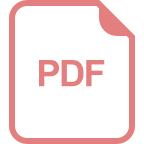
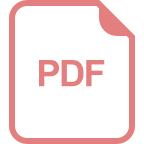
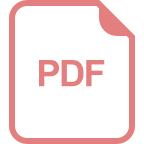
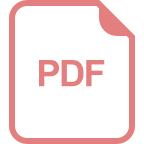
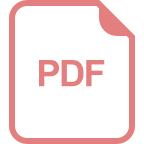
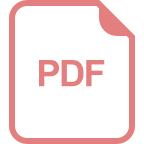
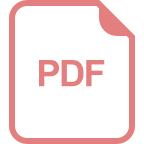
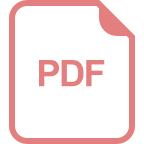
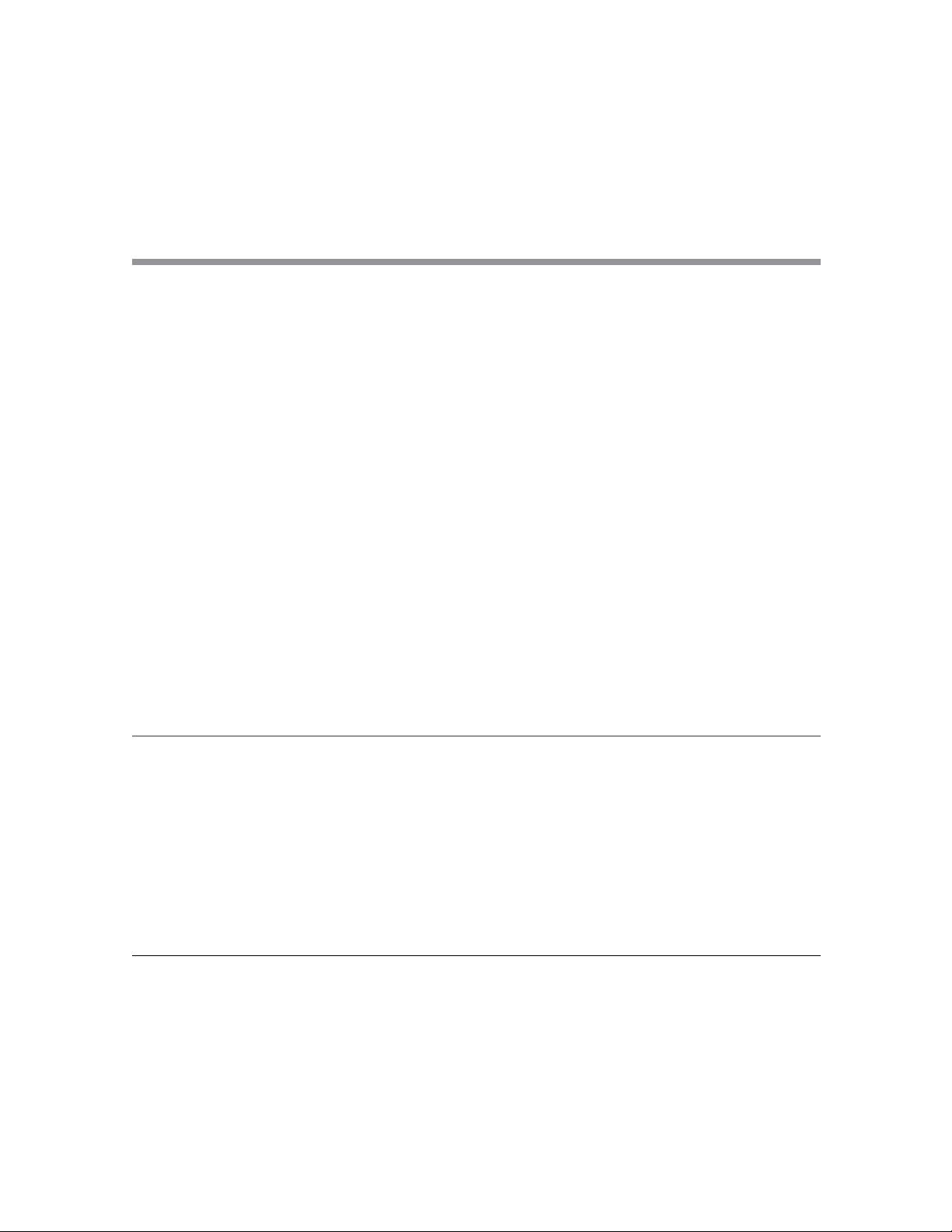
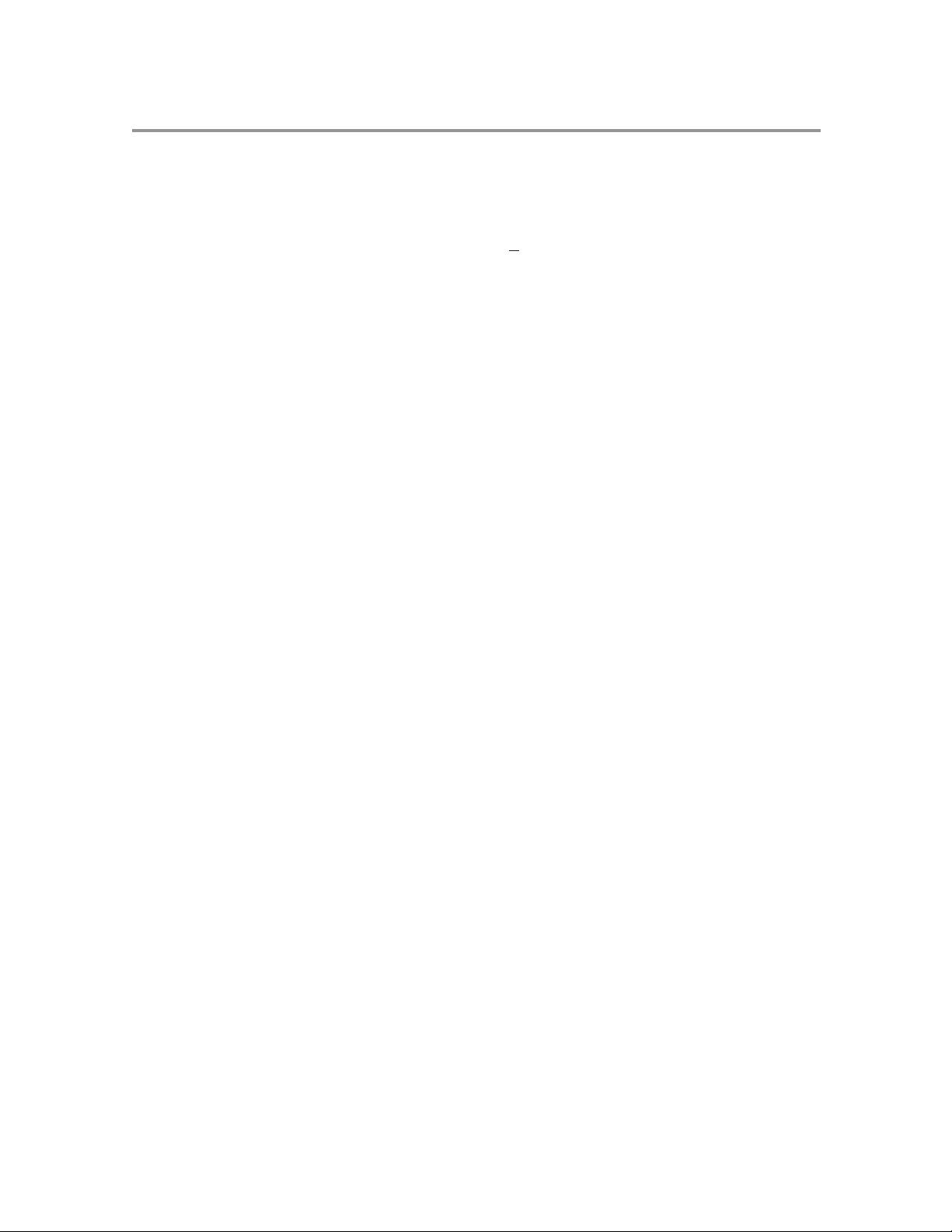
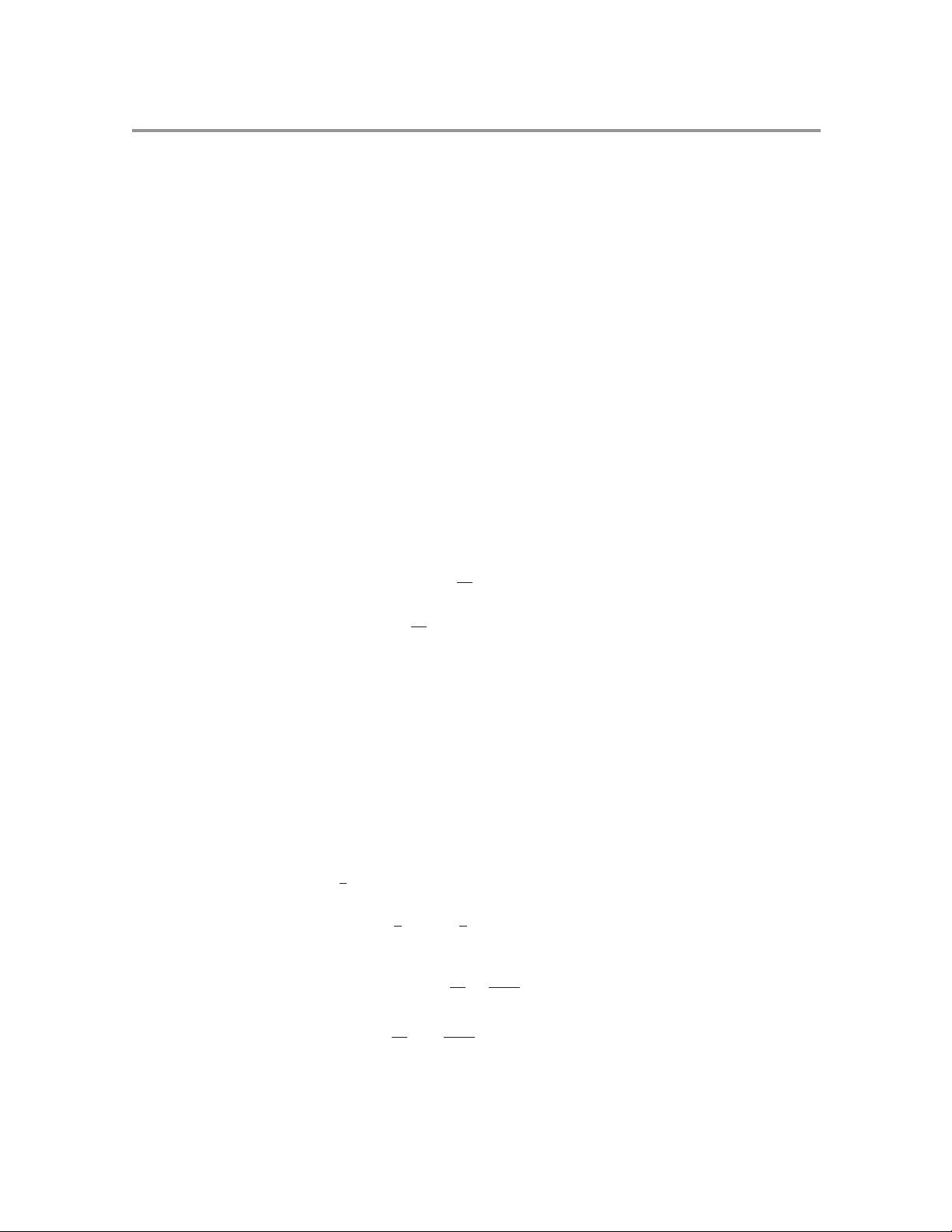
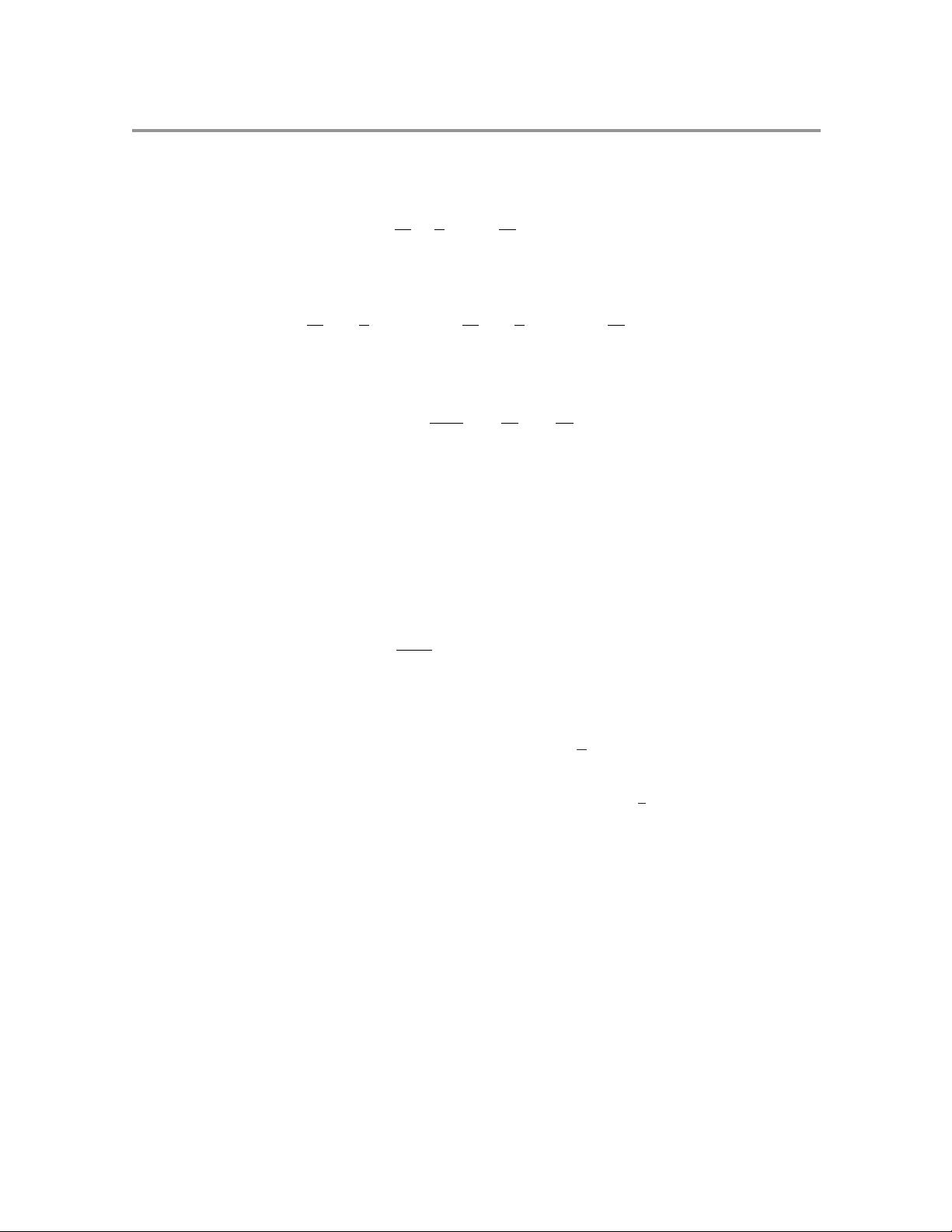
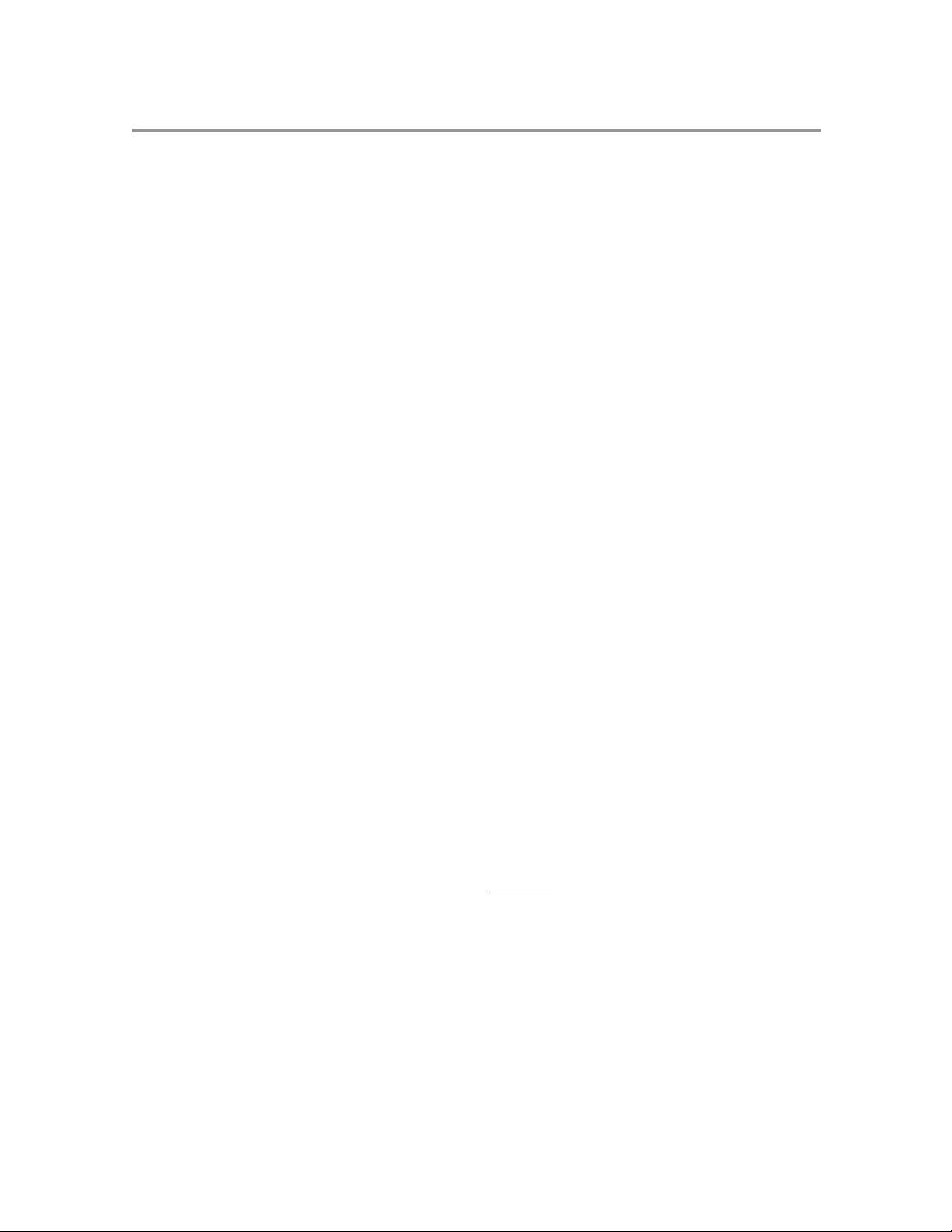
剩余27页未读,继续阅读
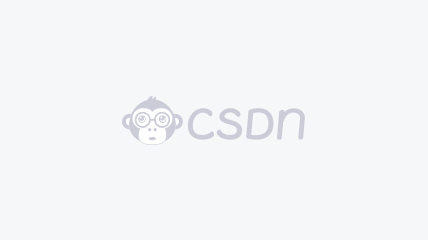

- 粉丝: 0
- 资源: 1
我的内容管理 展开
我的资源 快来上传第一个资源
我的收益
登录查看自己的收益我的积分 登录查看自己的积分
我的C币 登录后查看C币余额
我的收藏
我的下载
下载帮助

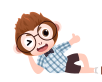
最新资源
- 小程序项目-基于微信小程序的游泳馆管理系统(包括源码,数据库,教程).zip
- 小程序项目-基于微信小程序的食堂线上预约点餐系统(包括源码,数据库,教程).zip
- 小程序项目-基于微信小程序的摄影竞赛小程序(包括源码,数据库,教程).zip
- 小程序项目-基于微信小程序的“健康早知道”微信小程序(包括源码,数据库,教程).zip
- 小程序项目-基于微信小程序的足浴城消费系统(包括源码,数据库,教程).zip
- 小程序项目-基于微信小程序的“最多跑一次”微信小程序(包括源码,数据库,教程).zip
- 小程序项目-基于微信小程序的青少年素质教育培训系统(包括源码,数据库,教程).zip
- 小程序项目-基于微信小程序的同城交易小程序(包括源码,数据库,教程).zip
- 小程序项目-基于微信小程序的书籍销售系统(包括源码,数据库,教程).zip
- 西门子1200程序和项目模板,适用初学者 本资料从一个项目出发,包含了 1.如何进行硬件设备的选型; 2.电气原理图绘制标准,给出了实际的CAD图进行参考; 3.如何设计触摸屏HMI的界面,功能等;
- 小程序项目-基于微信小程序的数学辅导(包括源码,数据库,教程).zip
- 小程序项目-基于微信小程序的node基于微信小程序的学生宿舍管理系统(包括源码,数据库,教程).zip
- QMA6100调试资料
- 小程序项目-基于微信小程序的django基于微信平台小区服务系统(包括源码,数据库,教程).zip
- 小程序项目-基于微信小程序的微信点餐系统(包括源码,数据库,教程).zip
- 小程序项目-基于微信小程序的微信小程序校园失物招领(包括源码,数据库,教程).zip

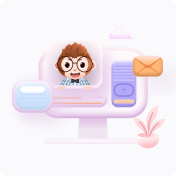
