没有合适的资源?快使用搜索试试~ 我知道了~
some notes from the book statiscal learning from a regression pe...
需积分: 0 1 下载量 76 浏览量
2015-11-05
15:25:35
上传
评论
收藏 1.07MB PDF 举报
温馨提示
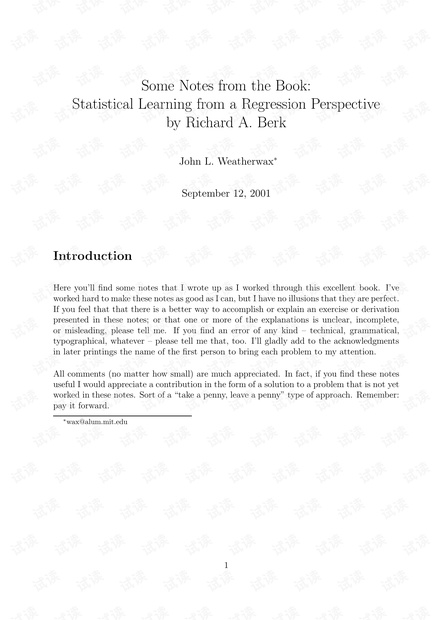

试读
26页
statiscal learning from a regression perspective这本书的一个读书笔记
资源推荐
资源评论
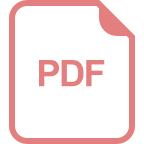
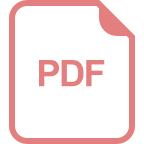
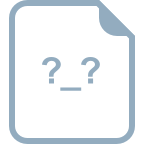
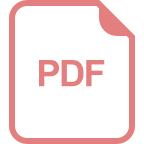
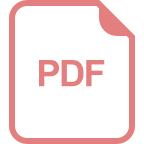
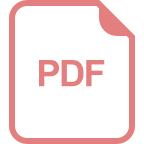
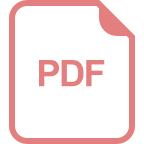
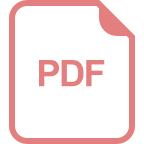
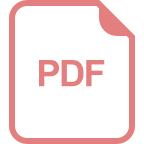
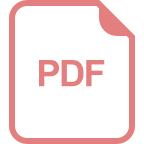
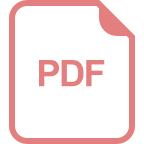
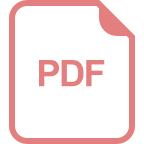
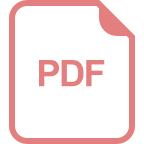
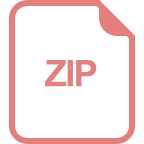
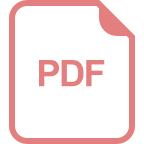
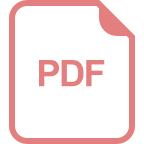
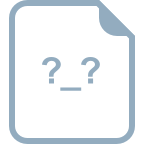
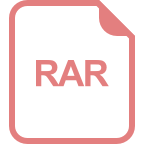
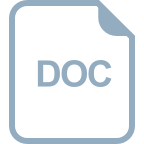
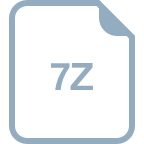
资源评论
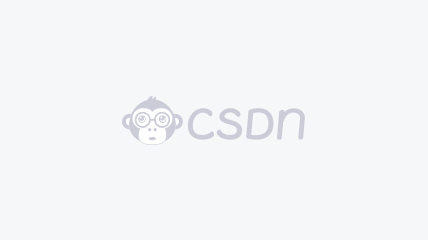

PeterKid314
- 粉丝: 0
- 资源: 4
上传资源 快速赚钱
我的内容管理 展开
我的资源 快来上传第一个资源
我的收益
登录查看自己的收益我的积分 登录查看自己的积分
我的C币 登录后查看C币余额
我的收藏
我的下载
下载帮助

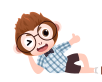
安全验证
文档复制为VIP权益,开通VIP直接复制
