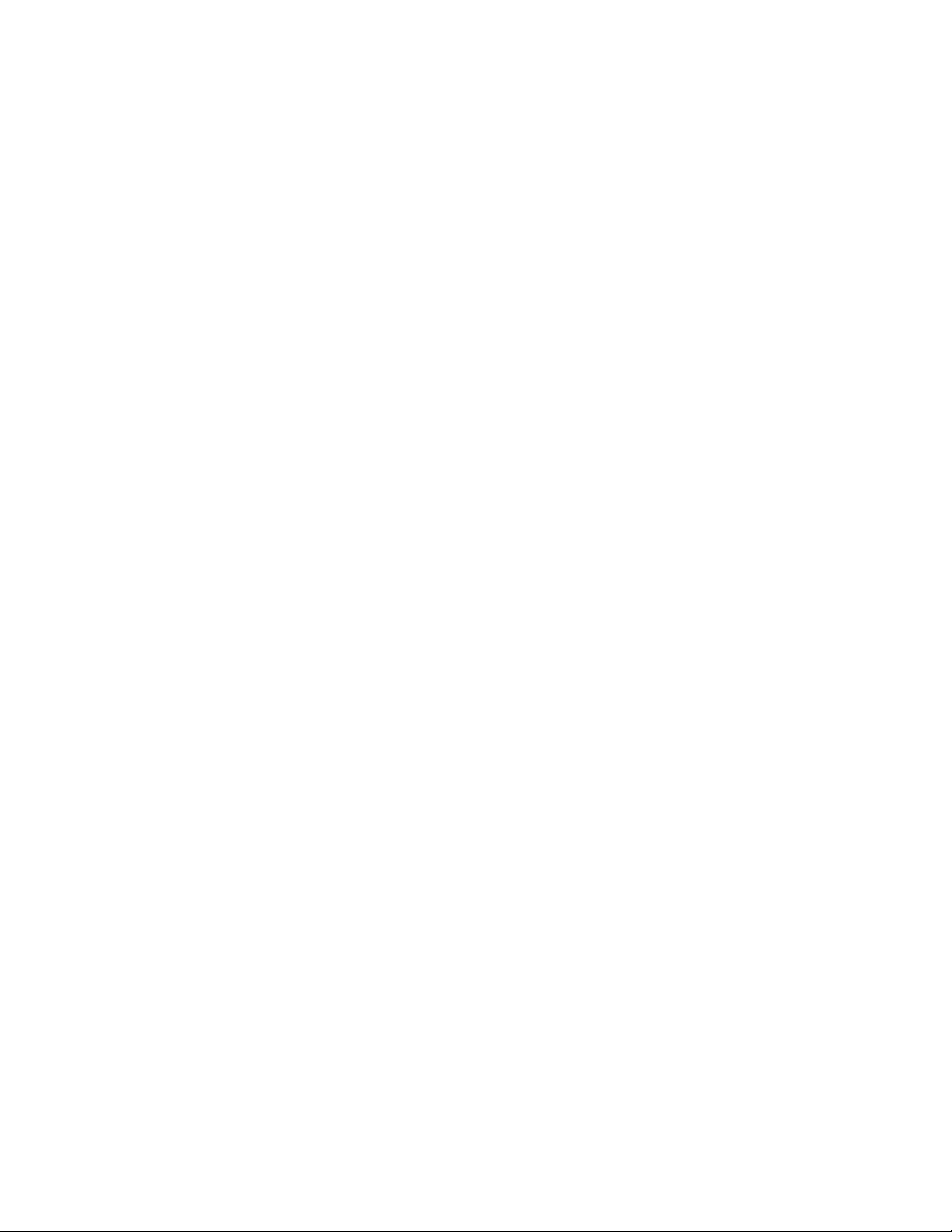
functionality make it attractive for most drivers. Even drivers who do not cur-
rently own a (P)HEV could use this application to predict the energy and en-
vironmental impacts if they switch to (P)HEVs. Moreover, the aggregated data
from a large number of drivers can be invaluable for vehicle manufacturers and
researchers as they keep improving the design of (P)HEVs.
A number of sensing capabilities are available in modern smartphones, mak-
ing it possible for personalized driving behavior monitoring and analysis. How-
ever, signals obtained from individual sensors suffer from a number of intrinsic
and contextual issues: (1) noise, drift, and mis-synchronization of multi-modality
driver–vehicle data; (2) substantial disorientation of vehicle movement sensing
at the start of and during each driving trip; and (3) complex (P)HEV operation
mechanisms and driver-dependent vehicle performance.
To address these issues, we propose innovative techniques that “fuse” to-
gether multiple types of sensor readings to enhance signal quality and driver–
vehicle sensing, as well as techniques that classify driver-specific operation
modes and analyze the corresponding energy and environmental impacts. Our
work makes the following contributions:
(1) Multi-modality driver–vehicle sensing. We propose fully-automated,
phone-based sensing techniques that effectively correct the noise, drift, mis-
synchronization in multiple types of sensor data, as well as compensating ini-
tial/dynamic phone–vehicle disorientation using wavelet-based analysis.
(2) Driver-specific (P)HEV analysis. We propose operation mode classifi-
cation, run-time energy use and fuel–CO
2
emission modeling, to map specific
driving behavior to (P)HEV energy and environmental impacts; and
(3) Real-world deployment and user driving studies. System evaluation
demonstrates high correlation for vehicle sensing (0.88-0.96), energy use and
fuel use modeling (0.918, 0.996), operation mode classification (89.9%, 87.8%
accuracy).
The rest of the paper is organized as follows. Section 2 presents the prob-
lem formulation and design overview. Section 3 and Section 4 describe in de-
tail multi-modality driver-vehicle sensing and driver-specific (P)HEV analysis.
Section 5 describes system deployment and user studies. Section 6 evaluates
the proposed system. Section 7 discusses related work. Section 8 concludes.
2 Problem Formulation and Design Overview
HEVs and PHEVs are the emerging solutions for transportation electrification.
HEVs feature a gasoline internal combustion engine (ICE) and an electric mo-
tor equipped with a battery system for harnessing and storing run-time braking
energy. PHEVs have an additional electrical plug to directly recharge the bat-
tery system from the electrical grid. The energy and environmental impacts of
(P)HEVs are primarily determined by (P)HEV operation, which in turn is heav-
ily affected by user-specific run-time driving behavior, such as speed, accelera-
tion, and road condition, etc. Figure 2 shows the fuel use, CO
2
emissions, and
battery system long-term capacity degradation based on eight different users’