# Lama-cleaner: Image inpainting tool powered by SOTA AI model
[](https://pepy.tech/project/lama-cleaner)
[](https://pepy.tech/project/lama-cleaner)

<a href="https://colab.research.google.com/drive/1e3ZkAJxvkK3uzaTGu91N9TvI_Mahs0Wb?usp=sharing" target="_parent"><img src="https://colab.research.google.com/assets/colab-badge.svg" alt="Open in Colab"/></a>
https://user-images.githubusercontent.com/3998421/153323093-b664bb68-2928-480b-b59b-7c1ee24a4507.mp4
- [x] Support multiple model architectures
1. [LaMa](https://github.com/saic-mdal/lama)
1. [LDM](https://github.com/CompVis/latent-diffusion)
- [x] Support CPU & GPU
- [x] High resolution support
- [x] Run as a desktop APP
- [x] Multi stroke support. Press and hold the `cmd/ctrl` key to enable multi stroke mode.
- [x] Zoom & Pan
## Install
```bash
pip install lama-cleaner
lama-cleaner --device=cpu --port=8080
```
Available commands:
| Name | Description | Default |
| ---------- | ------------------------------------------------ | -------- |
| --model | lama or ldm. See details in **Model Comparison** | lama |
| --device | cuda or cpu | cuda |
| --gui | Launch lama-cleaner as a desktop application | |
| --gui_size | Set the window size for the application | 1200 900 |
| --input | Path to image you want to load by default | None |
| --port | Port for flask web server | 8080 |
| --debug | Enable debug mode for flask web server | |
## Settings
You can change the configs of inpainting process in the settings interface of the web page.
<img src="./assets/settings.png" width="400px">
### Inpainting Model
Select the inpainting model to use, and set the configs corresponding to the model.
LaMa model has no configs that can be specified at runtime.
LDM model has two configs to control the quality of final result:
1. Steps: You can get better result with large steps, but it will be more time-consuming
2. Sampler: ddim or [plms](https://arxiv.org/abs/2202.09778). In general plms can get better results with fewer steps
### High Resolution Strategy
There are three strategies for handling high-resolution images.
- **Original**: Use the original resolution of the picture, suitable for picture size below 2K.
- **Resize**: Resize the longer side of the image to a specific size(keep ratio), then do inpainting on the resized image.
The inpainting result will be pasted back on the original image to make sure other part of image not loss quality.
- **Crop**: Crop masking area from the original image to do inpainting, and paste the result back.
Mainly for performance and memory reasons on high resolution image. This strategy may give better results for ldm model.
## Model Comparison
| Model | Pron | Corn |
|-------|-----------------------------------------------------------------------------------------------------------------------------------------|------------------------------------------------------------------------------------------------|
| LaMa | - Perform will on high resolution image(~2k)<br/> - Faster than diffusion model | |
| LDM | - It's possible to get better and more detail result, see example below<br/> - The balance of time and quality can be achieved by steps | - Slower than GAN model<br/> - Need more GPU memory<br/> - Not good for high resolution images |
| Original Image | LaMa | LDM |
| ----------------------------------------------------------------------------------------------------------------------------------------- | ------------------------------------------------------------------------------------------------------------------------------------------------------ | ----------------------------------------------------------------------------------------------------------------------------------------------------- |
| 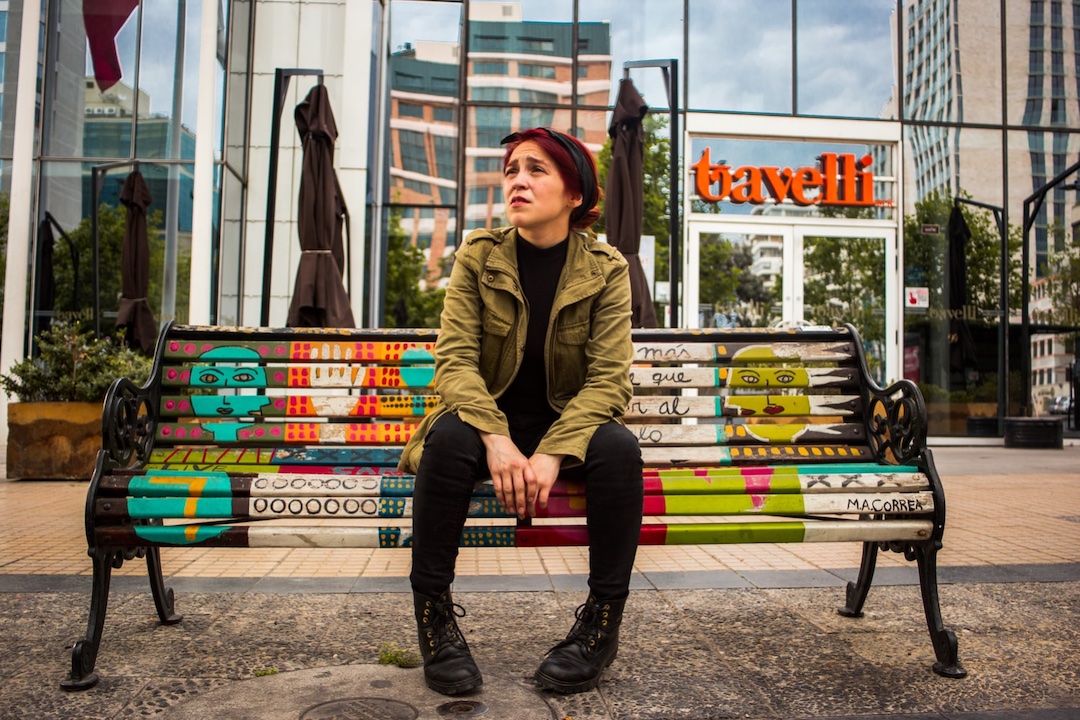 | 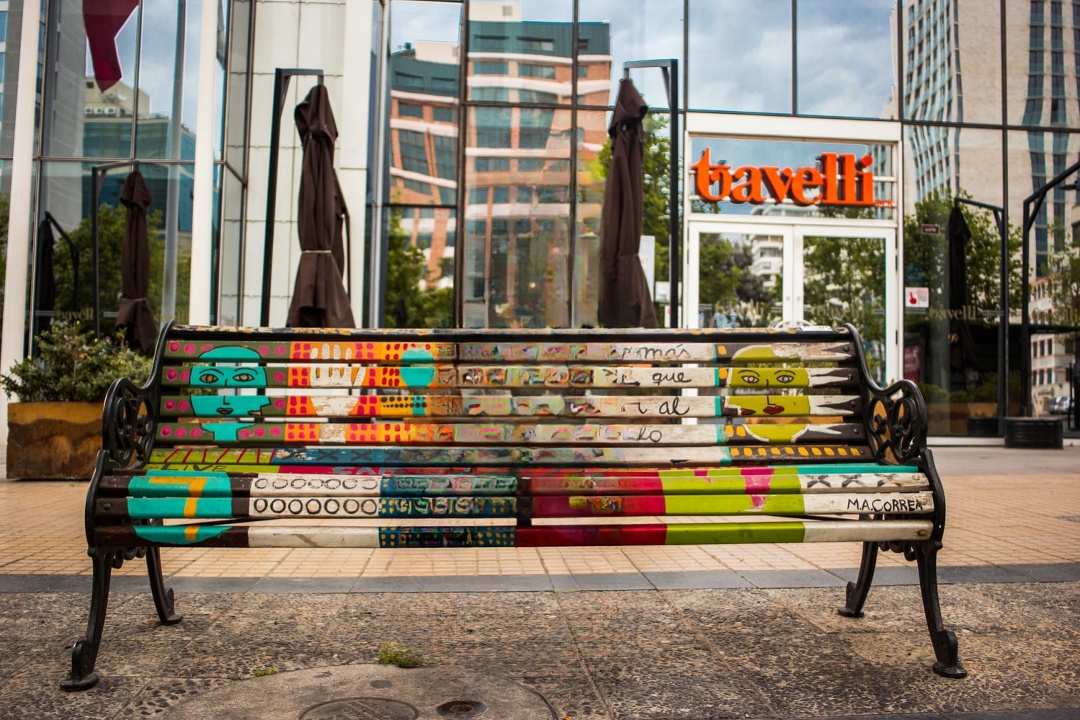 | 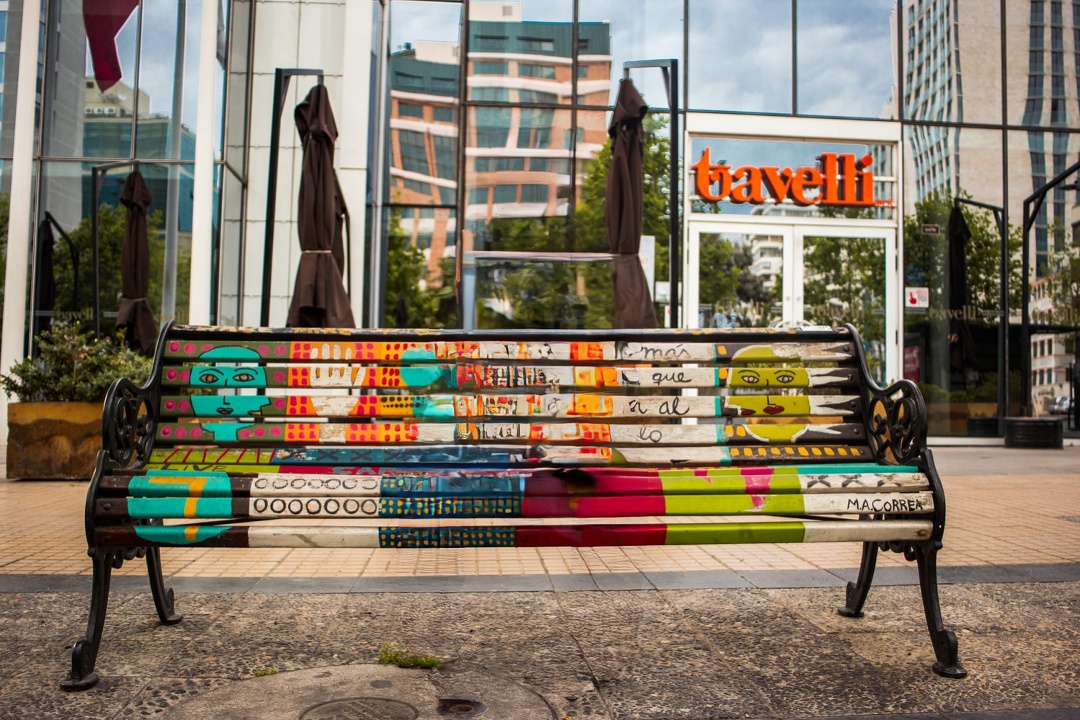 |
Blogs about diffusion models:
- https://lilianweng.github.io/posts/2021-07-11-diffusion-models/
- https://yang-song.github.io/blog/2021/score/
## Development
Only needed if you plan to modify the frontend and recompile yourself.
### Frontend
Frontend code are modified from [cleanup.pictures](https://github.com/initml/cleanup.pictures), You can experience their
great online services [here](https://cleanup.pictures/).
- Install dependencies:`cd lama_cleaner/app/ && yarn`
- Start development server: `yarn start`
- Build: `yarn build`
## Docker
Run within a Docker container. Set the `CACHE_DIR` to models location path. Optionally add a `-d` option to
the `docker run` command below to run as a daemon.
### Build Docker image
```
docker build -f Dockerfile -t lamacleaner .
```
### Run Docker (cpu)
```
docker run -p 8080:8080 -e CACHE_DIR=/app/models -v $(pwd)/models:/app/models -v $(pwd):/app --rm lamacleaner python3 main.py --device=cpu --port=8080
```
### Run Docker (gpu)
```
docker run --gpus all -p 8080:8080 -e CACHE_DIR=/app/models -v $(pwd)/models:/app/models -v $(pwd):/app --rm lamacleaner python3 main.py --device=cuda --port=8080
```
Then open [http://localhost:8080](http://localhost:8080)
## Like My Work?
<a href="https://www.buymeacoffee.com/Sanster">
<img height="50em" src="https://cdn.buymeacoffee.com/buttons/v2/default-blue.png" alt="Sanster" />
</a>

快撑死的鱼
- 粉丝: 2w+
- 资源: 9156
最新资源
- Java毕业设计-springboot-vue-工作量统计系统(源码+sql脚本+29页零基础部署图文详解+29页论文+环境工具+教程+视频+模板).zip
- Java毕业设计-springboot-vue-高校疫情防控web系统(源码+sql脚本+29页零基础部署图文详解+31页论文+环境工具+教程+视频+模板).zip
- 基于模型的六轴机器人阻抗控制算法演示与仿真参数设置指导(matlab simscape仿真机器人模型自定义切换,跟踪轨迹展示及算法学习),基于模型的六轴机器人阻抗力控制算法(matlab simsca
- Java毕业设计-springboot-vue-工资信息管理系统(源码+sql脚本+29页零基础部署图文详解+25页论文+环境工具+教程+视频+模板).zip
- 基于PaddlePaddle的眼疾识别项目(包含训练集、测试集、模型权重)
- Java毕业设计-springboot-vue-航班进出港管理系统(源码+sql脚本+29页零基础部署图文详解+29页论文+环境工具+教程+视频+模板).zip
- 基于ABAQUS、Opensees和Perform3d的结构易损性评估与IDA曲线绘制:matlab函数调用与地震波选波调幅实战指南,ABAQUS 、Opensees、Perform3d IDA曲线
- Java毕业设计-springboot-vue-果蔬作物疾病防治系统(源码+sql脚本+29页零基础部署图文详解+30页论文+环境工具+教程+视频+模板).zip
- Java毕业设计-springboot-vue-滑雪场管理系统(源码+sql脚本+29页零基础部署图文详解+27页论文+环境工具+教程+视频+模板).zip
- Java毕业设计-springboot-vue-家教管理系统(源码+sql脚本+29页零基础部署图文详解+32页论文+环境工具+教程+视频+模板).zip
- Java毕业设计-springboot-vue-驾校预约学习系统(源码+sql脚本+29页零基础部署图文详解+34页论文+环境工具+教程+视频+模板).zip
- 数据采集系统:下位机与上位机协同工作,高性能模数转换与灵活配置滤波功能,数据采集系统下位机与上位机代码 下位机采用开发板来完成 AD9226模数转芯片,最大65MHz采样,12bit量化 双通
- Java毕业设计-springboot-vue-集团门户网站(源码+sql脚本+29页零基础部署图文详解+33页论文+环境工具+教程+视频+模板).zip
- 蓝桥杯Java历年真题及其解析.docx
- Java毕业设计-springboot-vue-教师薪酬管理系统(源码+sql脚本+29页零基础部署图文详解+32页论文+环境工具+教程+视频+模板).zip
- Java毕业设计-springboot-vue-教学辅助系统(源码+sql脚本+29页零基础部署图文详解+31页论文+环境工具+教程+视频+模板).zip
资源上传下载、课程学习等过程中有任何疑问或建议,欢迎提出宝贵意见哦~我们会及时处理!
点击此处反馈


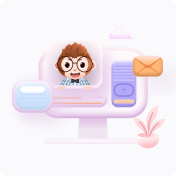