没有合适的资源?快使用搜索试试~ 我知道了~
资源推荐
资源详情
资源评论
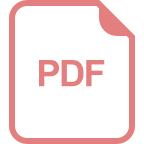
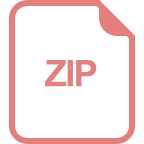
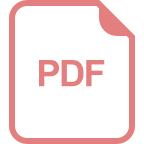
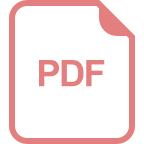
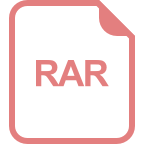
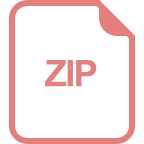
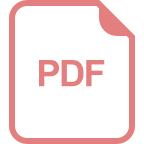
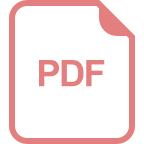
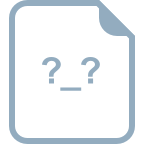
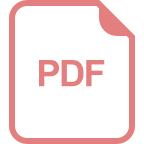
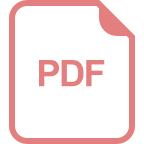
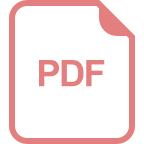
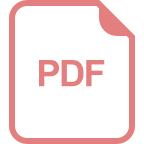
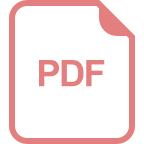
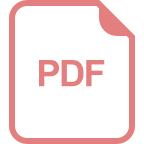
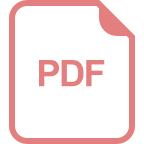
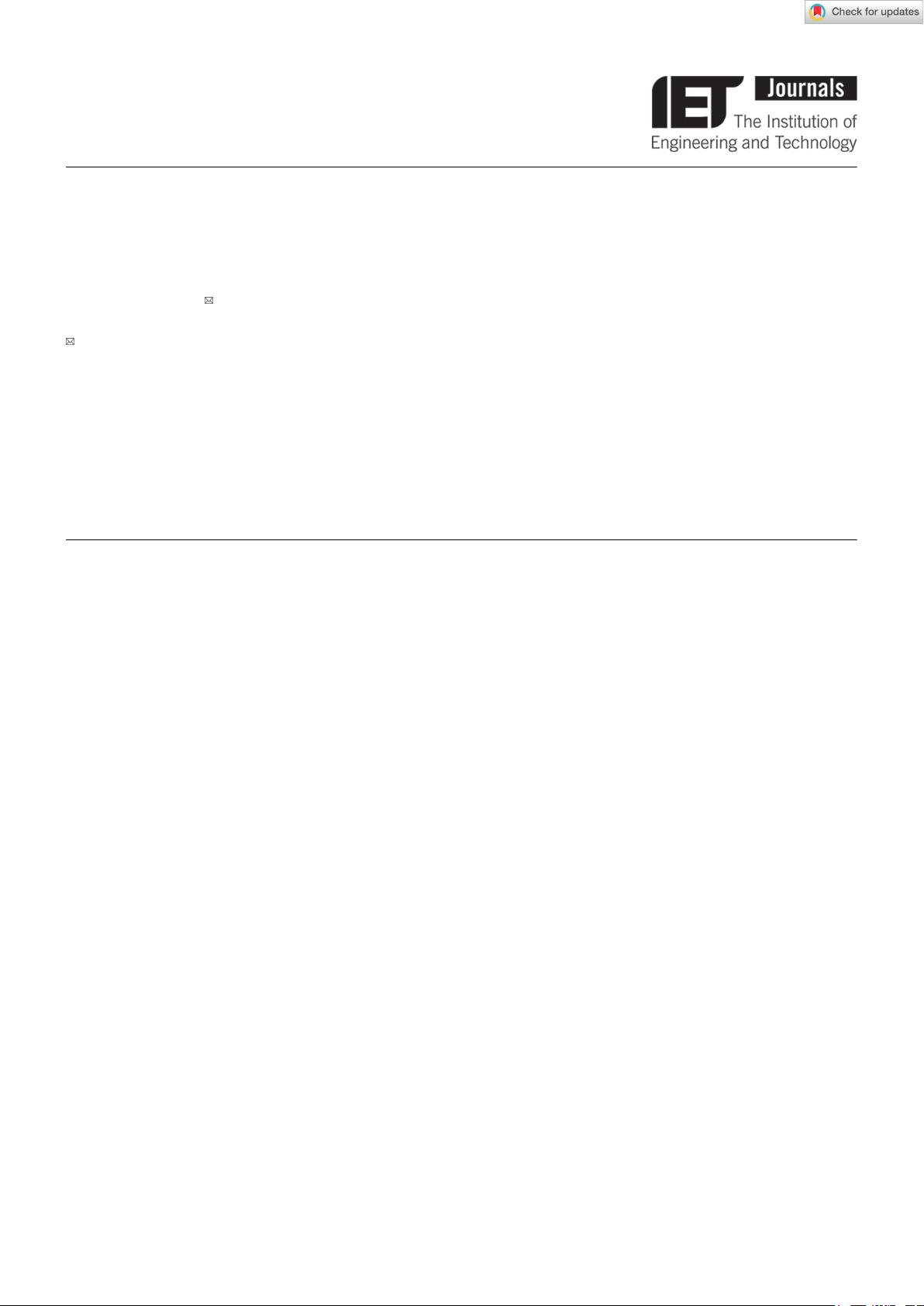
IET Image Processing
Research Article
Nighttime image enhancement using a new
illumination boost algorithm
ISSN 1751-9659
Received on 1st December 2018
Revised 21st January 2019
Accepted on 18th March 2019
E-First on 29th May 2019
doi: 10.1049/iet-ipr.2018.6585
www.ietdl.org
Zohair Al-Ameen
1
1
Department of Computer Science, College of Computer Science and Mathematics, University of Mosul, Mosul, Nineveh, Iraq
E-mail: qizohair@gmail.com
Abstract: Nighttime
images
are
often obtained with low brightness, deficient contrast, and latent colours. Thus, it is important to
improve such aspects in order to obtain acceptable quality images. Hence, a new illumination boost algorithm is proposed in this
study, in which it can improve the brightness, ameliorate contrast and process the colours of nighttime images properly.
Accordingly, the proposed algorithm utilises only a small number of steps and uses several processing concepts to achieve the
desired results. Intensive experiments and tests with various natural-degraded nighttime images are made to validate the
performance of the proposed algorithm. In addition, it is compared with eight contemporary algorithms, and the obtained results
from these comparisons are evaluated using two specialised image quality assessment metrics. Using the results of the
achieved experiments and comparisons, it became evident that the proposed algorithm can provide satisfactory outcomes, in
which it provided visually pleasing results and outperformed the comparison algorithms in terms of scored accuracy and visual
quality.
1 Introduction
Modern computer vision
and digital image processing applications
require images with high visibility to achieve the designated tasks
properly [1]. As known, the night environment is somewhat
intricate as the light is insufficient for proper image acquisition [2].
In addition, most of the available digital cameras cannot capture
acceptable quality nighttime images, in that the acquired images in
a low-light environment are often degraded by several artefacts and
have a low perceived quality [3]. Thus, it is highly required to
improve the quality of such images to become suitable for further
processing and interpretation [4]. The foremost aim of nighttime
image enhancement is to recover acceptable quality images in
terms of brightness, contrast, and colours without introducing any
undesirable effects, so that the recovered images appear better and
become more suitable for many existing image-related applications
[5]. The illumination of nighttime images is characterised as non-
uniform, wherein processing such images is deemed a challenge
and a hot research field that is still open for research since many of
the available enhancement algorithms produce results with
unnatural appearances [6]. Thus, different inspiring research works
have been conducted by various specialists to address this problem
in order to provide new algorithms that have better processing
abilities and are able to produce improved results.
Accordingly, Fu et al. [7] proposed a bright channel prior
(BCP) based algorithm that utilises the BCP and the retinex model
to remove the colour distortions, over-enhancement, and halo
effects produced by the traditional model. This algorithm starts by
applying a specific filtering process to obtain the bright channel of
the image. Then, a quadratic function is applied to obtain the global
minimum. Next, the output is further refined using a specialised
function. Finally, the results are corrected according to certain pre-
detected priors. In addition, Wang et al. [8] provided a naturalness
preserved enhancement (NPE) algorithm, which utilises the retinex
model and works by applying a bright-pass filter to disintegrate the
input image into illumination and reflectance which are used later
to determine the naturalness and details of the image. Then, a bi-
log transformation is used to remap the illumination in order to
balance the naturalness and the details of the image. Moreover, an
improved multi-scale retinex (IMSR) algorithm is developed in [9],
in which the authors modified the algorithm by replacing the
logarithmic function of the traditional multi-scale retinex (MSR)
by an adapted sigmoid function to reduce the loss of image data.
Then, the obtained
results by the sigmoid-MSR method are
combined with the original images to produce the final result.
Furthermore, a probabilistic image enhancement (PIE) based
algorithm is introduced in [10], wherein it improves the processing
abilities of the traditional retinex model by using a probabilistic
concept to achieve a concurrent approximation of reflectance and
illumination in the linear domain via the use of a maximum a
posteriori (MAP) concept. Then, a characteristics analysis of the
logarithmic transformation is attained to identify the approximation
reliability of illumination and reflectance. Finally, the MAP
concept is converted into an energy minimisation concept to
disintegrate the illumination and reflectance properly, while an
alternating direction method of multipliers is implemented to
approximate the portions of reflectance and illumination
concurrently. Besides, Ying et al. [11] provided a camera response
model (CRM) based algorithm, which considers the effect of the
camera response function in the enhancement process. This
algorithm determines the proper CRM to compute the exposure
ratio map (ERM) using the observed histogram characteristics.
Then, the detected ERM is utilised to enhance the input image to
produce the output image. In addition, Ren et al. [12] introduced a
sequential decomposition (SD) based algorithm, in which it works
by decomposing the retinex model into a consecutive sequence to
approximate a reduced-noise reflectance and a piecewise
illumination. Then, the illumination layer is adjusted using a
specialised method, while the reflectance layer is denoised using
spatial smoothing via weight matrices.
Likewise, Li et al. [13] proposed a robust retinex model (RRM)
based algorithm, which uses the retinex model to modify the
approximated reflectance and illumination and takes into
consideration the noise map of a given image to obtain the
enhanced image. In this work, the authors developed an
optimisation function that involves new regularisation aspects for
reflectance and illumination. Specifically, the L1 norm is used to
limit the smoothness of the illumination layer, an adopted fidelity
term is used for the reflectance layer to reveal more structural
details, and a specialised method is used to estimate the noise of
the retinex model. As well, this algorithm utilises an augmented
Lagrange multiplier with no logarithmic operations to solve the
optimisation problem in a proper manner. Finally, Tanaka et al.
[14] proposed a gradient-based enhancement (GBE) algorithm,
IET Image Process., 2019, Vol. 13 Iss. 8, pp. 1314-1320
© The Institution of Engineering and Technology 2019
1314
资源评论
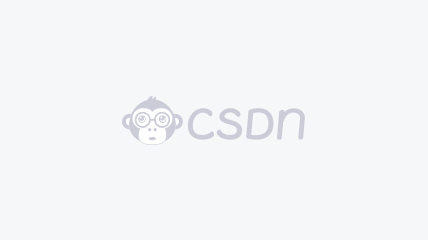

xxxx9300
- 粉丝: 705
- 资源: 12
上传资源 快速赚钱
我的内容管理 展开
我的资源 快来上传第一个资源
我的收益
登录查看自己的收益我的积分 登录查看自己的积分
我的C币 登录后查看C币余额
我的收藏
我的下载
下载帮助

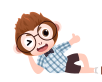
最新资源
资源上传下载、课程学习等过程中有任何疑问或建议,欢迎提出宝贵意见哦~我们会及时处理!
点击此处反馈


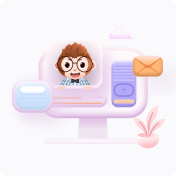
安全验证
文档复制为VIP权益,开通VIP直接复制
