### TensorFlow Models 安装知识点详解 #### 一、概述 TensorFlow 是一款由谷歌开发的开源机器学习框架,被广泛应用于各种深度学习任务之中。它提供了丰富的API接口,支持多种平台,包括Windows、Linux和MacOS等操作系统。为了进一步拓展其功能并方便用户使用,TensorFlow还提供了一系列预训练模型,这些模型可以快速部署到项目中,帮助开发者节省大量时间与计算资源。 在实际应用过程中,用户往往需要安装特定的TensorFlow模型库,以便于调用各种预训练模型进行研究或开发工作。本文将详细介绍如何安装TensorFlow Models,并对其相关知识点进行深入剖析。 #### 二、TensorFlow Models 简介 TensorFlow Models 库包含了多个官方维护和支持的模型,如目标检测、语义分割、自然语言处理等领域的经典算法实现。通过使用这些模型,用户无需从头构建整个神经网络结构,而是可以直接加载已经训练好的模型参数,在自己的数据集上进行微调或者直接用于推理任务。 #### 三、安装前准备 在开始安装之前,请确保已经完成了以下步骤: 1. **安装Python**:推荐使用Python 3.6及以上版本。 2. **安装TensorFlow**:可以通过pip命令安装CPU版或GPU版的TensorFlow。如果希望在GPU上运行程序,则还需要额外安装CUDA和cuDNN等组件。 3. **安装Git**:用于克隆GitHub仓库。 #### 四、安装TensorFlow Models ##### 1. 克隆TensorFlow Models 仓库 ```bash git clone https://github.com/tensorflow/models.git ``` ##### 2. 进入models目录 ```bash cd models ``` ##### 3. 安装依赖库 ```bash pip install -r requirements.txt ``` ##### 4. 编译Protobuf 协议缓冲区 ```bash # 进入到protos目录 cd research/object_detection/protos # 编译protos protoc object_detection.proto --python_out=. protoc train.proto --python_out=. ``` ##### 5. 将模型库添加到环境变量 ```bash export PYTHONPATH=$PYTHONPATH:`pwd`:`pwd`/slim ``` #### 五、下载预训练模型 根据题目中的描述,我们可以直接通过网盘链接下载所需的模型文件。但请注意,这种做法存在一定的风险,因为无法保证下载来源的安全性和可靠性。建议还是从官方渠道获取模型文件,这样可以避免潜在的安全隐患。 官方提供了两种方式来下载预训练模型: 1. **通过GitHub仓库下载**:在models仓库中包含了所有可用模型的配置文件和示例代码,可以在对应的子目录下找到相应模型的配置文件。 2. **通过Model Zoo下载**:访问[TensorFlow Model Zoo](https://github.com/tensorflow/models/blob/master/research/object_detection/g3doc/tf2_detection_zoo.md),查看所有可用模型列表及其下载链接。 假设我们按照题目中提供的百度网盘链接下载了模型文件,并将其放置在TensorFlow安装目录下的某个子文件夹内,例如`models/research/object_detection`。接下来,我们需要对模型进行加载和使用。 #### 六、加载并使用模型 假设我们已经下载了一个名为`ssd_mobilenet_v2_coco`的目标检测模型,其下载路径为`models/research/object_detection/ssd_mobilenet_v2_coco_2018_03_29.tar.gz`。 1. **解压模型文件** ```bash tar -xvf ssd_mobilenet_v2_coco_2018_03_29.tar.gz ``` 2. **加载模型** ```python import tensorflow as tf from object_detection.utils import label_map_util from object_detection.utils import visualization_utils as vis_util # 加载已保存的模型 detect_fn = tf.saved_model.load('ssd_mobilenet_v2_coco_2018_03_29/saved_model') # 加载标签映射文件 category_index = label_map_util.create_category_index_from_labelmap('mscoco_label_map.pbtxt', use_display_name=True) ``` 3. **进行推理** ```python import numpy as np import cv2 def run_inference_for_single_image(image, model): image = np.asarray(image) # 输入必须为float32类型 input_tensor = tf.convert_to_tensor(image) # 添加一个batch维度 input_tensor = input_tensor[tf.newaxis,...] # 运行模型 output_dict = model(input_tensor) # 所有输出都是batch维度的,因此去掉第一个维度 num_detections = int(output_dict.pop('num_detections')) output_dict = {key:value[0, :num_detections].numpy() for key,value in output_dict.items()} output_dict['num_detections'] = num_detections # 检测结果是缩放后的,需要还原回原图大小 output_dict['detection_boxes'] = output_dict['detection_boxes'][0, :num_detections].numpy() output_dict['detection_boxes'] = output_dict['detection_boxes'] * np.array([height, width, height, width]) return output_dict # 读取图像 image_np = cv2.imread('path/to/image.jpg') # 进行推理 output_dict = run_inference_for_single_image(image_np, detect_fn) # 可视化结果 vis_util.visualize_boxes_and_labels_on_image_array( image_np, output_dict['detection_boxes'], output_dict['detection_classes'], output_dict['detection_scores'], category_index, instance_masks=output_dict.get('detection_masks_reframed', None), use_normalized_coordinates=True, line_thickness=8) cv2.imshow('object detection', cv2.cvtColor(image_np, cv2.COLOR_BGR2RGB)) cv2.waitKey(0) cv2.destroyAllWindows() ``` 通过以上步骤,我们便可以成功地加载并使用下载的预训练模型进行目标检测任务。需要注意的是,在实际部署过程中可能还需要根据具体应用场景调整模型参数和优化性能。 #### 七、总结 本文详细介绍了如何安装TensorFlow Models以及如何加载和使用预训练模型。在整个过程中,我们首先克隆了官方仓库并安装了必要的依赖库;然后通过官方提供的下载链接获取了所需模型文件;利用Python脚本实现了模型的加载与推理过程。希望本文能够帮助读者更好地理解和掌握TensorFlow Models的相关知识,从而更高效地开展机器学习项目开发工作。
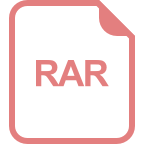
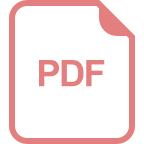
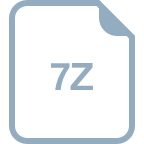
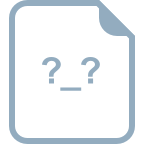
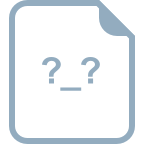
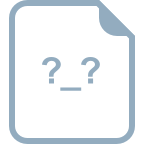
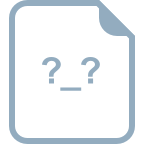
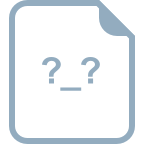
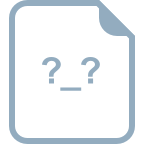
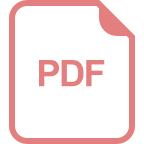
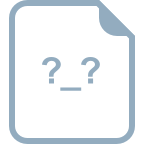
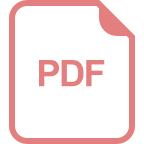
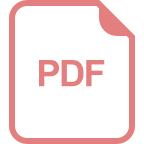
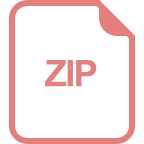
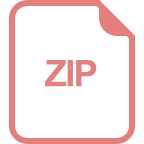
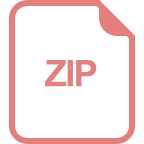
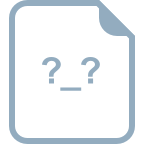
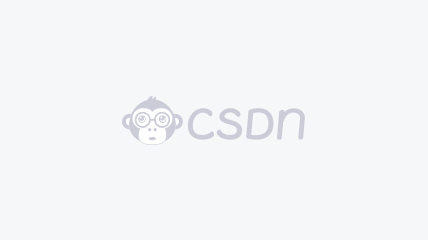


- 粉丝: 0
- 资源: 3
我的内容管理 展开
我的资源 快来上传第一个资源
我的收益
登录查看自己的收益我的积分 登录查看自己的积分
我的C币 登录后查看C币余额
我的收藏
我的下载
下载帮助

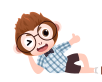
最新资源

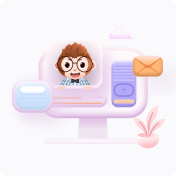
