# FaceDataset
制作亚洲人脸数据集
## 依赖环境
```
pip3 install baidu-aip -i https://mirrors.aliyun.com/pypi/simple/
pip3 install tqdm -i https://mirrors.aliyun.com/pypi/simple/
pip3 install pillow -i https://mirrors.aliyun.com/pypi/simple/
pip3 install tensorflow==1.14.0 -i https://mirrors.aliyun.com/pypi/simple/
pip3 install mtcnn -i https://mirrors.aliyun.com/pypi/simple/
pip3 install opencv-python -i https://mirrors.aliyun.com/pypi/simple/
pip3 install face_recognition -i https://mirrors.aliyun.com/pypi/simple/
```
# 制作人脸数据集
下面我们就介绍如何制作自己的人脸数据集,项目的开源地址:https://github.com/yeyupiaoling/FaceDataset 。该项目可以分为两个阶段,第一阶段是人脸图片的获取和简单的清洗,第二阶段是人脸图片的高级清洗和标注人脸信息。人脸信息的标注和清洗使用到了百度的人脸识别服务。
## 第一阶段
爬取人脸图片的核心思路就是获取中国明星的名字,然后使用明星的名字作为图片搜索的关键字进行获取图片,然后删除下载过程损坏的图片和没有包含人脸的图片,或者过多人脸的图片(我们只保存一张图片只包含一张人脸的图片)。
首先获取中国明星的名字,该功能主要在`get_star_name.py`中实现。获取明显的名字核心代码如下,获取的名字不能保证百分之百正确,所以可能需要手动去检查。
```python
# 获取明星的名字并保存到文件中
def get_page(pages, star_name):
params = []
# 设置访问的请求头,包括分页数和明星所在的地区
for i in range(0, 12 * pages + 12, 12):
params.append({
'resource_id': 28266,
'from_mid': 1,
'format': 'json',
'ie': 'utf-8',
'oe': 'utf-8',
'query': '明星',
'sort_key': '',
'sort_type': 1,
'stat0': '',
'stat1': star_name,
'stat2': '',
'stat3': '',
'pn': i,
'rn': 12})
# 请求的百度接口获取明星的名字
url = 'https://sp0.baidu.com/8aQDcjqpAAV3otqbppnN2DJv/api.php'
x = 0
# 根据请求头下载明星的名字
for param in params:
try:
# 获取请求数据
res = requests.get(url, params=param, timeout=50)
# 把网页数据转换成json数据
js = json.loads(res.text)
# 获取json中的明星数据
results = js.get('data')[0].get('result')
except AttributeError as e:
print('【错误】出现错误:%s' % e)
continue
# 从数据中提取明星的名字
for result in results:
img_name = result['ename']
f.write(img_name + '\n',)
```
然后根据明星的名字从网上下载图片,该功能主要在`download_image.py`中实现,以下就是下载图片的核心代码片段。
```python
# 获取百度图片下载图片
def download_image(key_word, download_max):
download_sum = 0
str_gsm = '80'
# 把每个明显的图片存放在单独一个文件夹中
save_path = 'star_image' + '/' + key_word
if not os.path.exists(save_path):
os.makedirs(save_path)
while download_sum < download_max:
# 下载次数超过指定值就停止下载
if download_sum >= download_max:
break
str_pn = str(download_sum)
# 定义百度图片的路径
url = 'http://image.baidu.com/search/flip?tn=baiduimage&ie=utf-8&' \
'word=' + key_word + '&pn=' + str_pn + '&gsm=' + str_gsm + '&ct=&ic=0&lm=-1&width=0&height=0'
try:
# 获取当前页面的源码
result = requests.get(url, timeout=30).text
# 获取当前页面的图片URL
img_urls = re.findall('"objURL":"(.*?)",', result, re.S)
if len(img_urls) < 1:
break
# 把这些图片URL一个个下载
for img_url in img_urls:
# 获取图片内容
img = requests.get(img_url, timeout=30)
img_name = save_path + '/' + str(uuid.uuid1()) + '.jpg'
# 保存图片
with open(img_name, 'wb') as f:
f.write(img.content)
with open('image_url_list.txt', 'a+', encoding='utf-8') as f:
f.write(img_name + '\t' + img_url + '\n')
download_sum += 1
if download_sum >= download_max:
break
except Exception as e:
download_sum += 1
continue
```
下载图片完成之后,有很多损坏的图片,需要把这些损坏的图片删除,该功能主要在`delete_error_image.py`实现。下面是删除损坏图片的核心代码片段。
```python
# 删除不是JPEG或者PNG格式的图片
def delete_error_image(father_path):
# 获取父级目录的所有文件以及文件夹
image_paths = []
for root, dirs, files in os.walk(father_path):
for file in files:
image_paths.append(os.path.join(root, file))
for image in tqdm(image_paths):
try:
# 获取图片的类型
image_type = imghdr.what(image)
# 如果图片格式不是JPEG同时也不是PNG就删除图片
if image_type is not 'jpeg' and image_type is not 'png':
os.remove(image)
continue
# 删除灰度图
img = numpy.array(Image.open(image))
if len(img.shape) is 2:
os.remove(image)
except:
os.remove(image)
```
下载的图片中可能没有人脸,或者包含多张人脸,所以我们要把这些图片删除掉,该功能主要在`delete_more_than_one.py`中实现。删除没有人脸或者过多人脸图片的关键代码片段如下。
```python
# 删除两个人脸以上的图片或者没有人脸的图片
def delete_image(image_path):
try:
img = cv2.imdecode(np.fromfile(image_path, dtype=np.uint8), -1)
result = detector.detect_faces(img)
if len(result) != 1:
os.remove(image_path)
else:
confidence = result[0]['confidence']
if confidence < 0.85:
os.remove(image_path)
except Exception as e:
print(e)
print('delete: %s' % image_path)
os.remove(image_path)
```
## 第二阶段
第二阶段属于高级清理和标注人脸信息。这一个阶段首先是把每个文件夹中包含相同一个人的图片较多的人脸,选择其中一个作为主人脸图片。然后使用这个主图片来对比其他图片,判断是否是同一个人,如果不是就删除该图片。接着就删除URL文件中,一些删除的文件对应的URL。最好就使用百度的人脸检测服务标注清理后的图片,最终得到一个人脸数据集。
首先是从众多图片中选择一个主图片,这个功能主要在`find_same_person.py`中实现,以下是获取主图片的核心代码片段。这个程序消耗时间比较多,其实也可以通过手动标记的方式,选择一个主的人脸图片,当然这个是非常大的一个工作量。
```python
# 寻找同一个人的作为0.jpg,作为主的参考图片
def find_same_person(person_image_path):
# 获取该人中的所有图片
image_paths = os.listdir(person_image_path)
known_face_encodings = []
for image_path in image_paths:
img_path = os.path.join(person_image_path, image_path)
try:
image = face_recognition.load_image_file(img_path)
encodings = face_recognition.face_encodings(image, num_jitters=10)[0]
known_face_encodings.append(encodings)
except:
os.remove(img_path)
for image_path in image_paths:
try:
img_path = os.path.join(person_image_p

机智的程序员zero
- 粉丝: 2454
- 资源: 4700
最新资源
- 基于多源深度学习的软件漏洞自动发现框架研究与应用
- 基于 RAG 与大模型技术的医疗问答系统源码+文档说明+全部资料(高分毕设)
- lca_com.ss.android.ugc.livepro-1810-1736134856489.apk
- 银行通信系统介绍C-S架构,消息队列实现通信-C语言-2025.zip(C语言)
- 河南工程学院oj答案河南工程学院编程竞赛C/C++基础题解与应用实例
- 停车场管理系统的C语言简单实现及应用
- 机械设计塑料彩盒自动热压成型设备sw17全套技术资料100%好用.zip
- 考虑不同充电需求的电动汽车有序充电调度方法matlab 复现A coordinated charging scheduling method for electric vehicles consid
- win32汇编环境,在对话框程序中画三角形
- 信息安全领域的钓鱼邮件识别与防范技巧
- 2024动漫数据集(27k+ 记录,18特征)CSV
- Python快速排序算法的基础原理、优化与应用指南
- 汽车BCM Simulink模型 目前MBD是汽车电子的开发主流思路,如果你有向这方面发展的想法,可以参考此模型,里面包含了建模思路,Simulink中Stateflow的逻辑搭建 可以提供汽车电子
- 机械设计手机自动MC测试机sw21可编辑全套技术资料100%好用.zip
- 机械设计隧道污泥吊机sw17全套技术资料100%好用.zip
- 三相锁相环DSP代码 基于双二阶广义积分器的锁相环,采用Ti的28335实现,模块化编程
资源上传下载、课程学习等过程中有任何疑问或建议,欢迎提出宝贵意见哦~我们会及时处理!
点击此处反馈


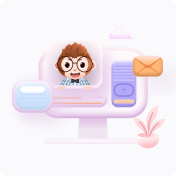