---
title: LeNet MNIST Tutorial
description: Train and test "LeNet" on the MNIST handwritten digit data.
category: example
include_in_docs: true
priority: 1
---
# Training LeNet on MNIST with Caffe
We will assume that you have Caffe successfully compiled. If not, please refer to the [Installation page](/installation.html). In this tutorial, we will assume that your Caffe installation is located at `CAFFE_ROOT`.
## Prepare Datasets
You will first need to download and convert the data format from the MNIST website. To do this, simply run the following commands:
cd $CAFFE_ROOT
./data/mnist/get_mnist.sh
./examples/mnist/create_mnist.sh
If it complains that `wget` or `gunzip` are not installed, you need to install them respectively. After running the script there should be two datasets, `mnist_train_lmdb`, and `mnist_test_lmdb`.
## LeNet: the MNIST Classification Model
Before we actually run the training program, let's explain what will happen. We will use the [LeNet](http://yann.lecun.com/exdb/publis/pdf/lecun-01a.pdf) network, which is known to work well on digit classification tasks. We will use a slightly different version from the original LeNet implementation, replacing the sigmoid activations with Rectified Linear Unit (ReLU) activations for the neurons.
The design of LeNet contains the essence of CNNs that are still used in larger models such as the ones in ImageNet. In general, it consists of a convolutional layer followed by a pooling layer, another convolution layer followed by a pooling layer, and then two fully connected layers similar to the conventional multilayer perceptrons. We have defined the layers in `$CAFFE_ROOT/examples/mnist/lenet_train_test.prototxt`.
## Define the MNIST Network
This section explains the `lenet_train_test.prototxt` model definition that specifies the LeNet model for MNIST handwritten digit classification. We assume that you are familiar with [Google Protobuf](https://developers.google.com/protocol-buffers/docs/overview), and assume that you have read the protobuf definitions used by Caffe, which can be found at `$CAFFE_ROOT/src/caffe/proto/caffe.proto`.
Specifically, we will write a `caffe::NetParameter` (or in python, `caffe.proto.caffe_pb2.NetParameter`) protobuf. We will start by giving the network a name:
name: "LeNet"
### Writing the Data Layer
Currently, we will read the MNIST data from the lmdb we created earlier in the demo. This is defined by a data layer:
layer {
name: "mnist"
type: "Data"
transform_param {
scale: 0.00390625
}
data_param {
source: "mnist_train_lmdb"
backend: LMDB
batch_size: 64
}
top: "data"
top: "label"
}
Specifically, this layer has name `mnist`, type `data`, and it reads the data from the given lmdb source. We will use a batch size of 64, and scale the incoming pixels so that they are in the range \[0,1\). Why 0.00390625? It is 1 divided by 256. And finally, this layer produces two blobs, one is the `data` blob, and one is the `label` blob.
### Writing the Convolution Layer
Let's define the first convolution layer:
layer {
name: "conv1"
type: "Convolution"
param { lr_mult: 1 }
param { lr_mult: 2 }
convolution_param {
num_output: 20
kernel_size: 5
stride: 1
weight_filler {
type: "xavier"
}
bias_filler {
type: "constant"
}
}
bottom: "data"
top: "conv1"
}
This layer takes the `data` blob (it is provided by the data layer), and produces the `conv1` layer. It produces outputs of 20 channels, with the convolutional kernel size 5 and carried out with stride 1.
The fillers allow us to randomly initialize the value of the weights and bias. For the weight filler, we will use the `xavier` algorithm that automatically determines the scale of initialization based on the number of input and output neurons. For the bias filler, we will simply initialize it as constant, with the default filling value 0.
`lr_mult`s are the learning rate adjustments for the layer's learnable parameters. In this case, we will set the weight learning rate to be the same as the learning rate given by the solver during runtime, and the bias learning rate to be twice as large as that - this usually leads to better convergence rates.
### Writing the Pooling Layer
Phew. Pooling layers are actually much easier to define:
layer {
name: "pool1"
type: "Pooling"
pooling_param {
kernel_size: 2
stride: 2
pool: MAX
}
bottom: "conv1"
top: "pool1"
}
This says we will perform max pooling with a pool kernel size 2 and a stride of 2 (so no overlapping between neighboring pooling regions).
Similarly, you can write up the second convolution and pooling layers. Check `$CAFFE_ROOT/examples/mnist/lenet_train_test.prototxt` for details.
### Writing the Fully Connected Layer
Writing a fully connected layer is also simple:
layer {
name: "ip1"
type: "InnerProduct"
param { lr_mult: 1 }
param { lr_mult: 2 }
inner_product_param {
num_output: 500
weight_filler {
type: "xavier"
}
bias_filler {
type: "constant"
}
}
bottom: "pool2"
top: "ip1"
}
This defines a fully connected layer (known in Caffe as an `InnerProduct` layer) with 500 outputs. All other lines look familiar, right?
### Writing the ReLU Layer
A ReLU Layer is also simple:
layer {
name: "relu1"
type: "ReLU"
bottom: "ip1"
top: "ip1"
}
Since ReLU is an element-wise operation, we can do *in-place* operations to save some memory. This is achieved by simply giving the same name to the bottom and top blobs. Of course, do NOT use duplicated blob names for other layer types!
After the ReLU layer, we will write another innerproduct layer:
layer {
name: "ip2"
type: "InnerProduct"
param { lr_mult: 1 }
param { lr_mult: 2 }
inner_product_param {
num_output: 10
weight_filler {
type: "xavier"
}
bias_filler {
type: "constant"
}
}
bottom: "ip1"
top: "ip2"
}
### Writing the Loss Layer
Finally, we will write the loss!
layer {
name: "loss"
type: "SoftmaxWithLoss"
bottom: "ip2"
bottom: "label"
}
The `softmax_loss` layer implements both the softmax and the multinomial logistic loss (that saves time and improves numerical stability). It takes two blobs, the first one being the prediction and the second one being the `label` provided by the data layer (remember it?). It does not produce any outputs - all it does is to compute the loss function value, report it when backpropagation starts, and initiates the gradient with respect to `ip2`. This is where all magic starts.
### Additional Notes: Writing Layer Rules
Layer definitions can include rules for whether and when they are included in the network definition, like the one below:
layer {
// ...layer definition...
include: { phase: TRAIN }
}
This is a rule, which controls layer inclusion in the network, based on current network's state.
You can refer to `$CAFFE_ROOT/src/caffe/proto/caffe.proto` for more information about layer rules and model schema.
In the above example, this layer will be included only in `TRAIN` phase.
If we change `TRAIN` with `TEST`, then this layer will be used only in test phase.
By default, that is without layer rules, a layer is always included in the network.
Thus, `lenet_train_test.prototxt` has two `DATA` layers defined (with different `batch_size`), one for the training phase and one for the testing phase.
Also, there is an `Accuracy` layer which is included only in `TEST` phase for reporting the model accuracy every 100 iteration, as defined in `lenet_solver.prototxt`.
## Define t
没有合适的资源?快使用搜索试试~ 我知道了~
温馨提示
1、该资源内项目代码经过严格调试,下载即用确保可以运行! 2、该资源适合计算机相关专业(如计科、人工智能、大数据、数学、电子信息等)正在做课程设计、期末大作业和毕设项目的学生、或者相关技术学习者作为学习资料参考使用。 3、该资源包括全部源码,需要具备一定基础才能看懂并调试代码。 基于深度学习方法对灰度图片上色的算法源码+项目说明+数据集.zip
资源推荐
资源详情
资源评论
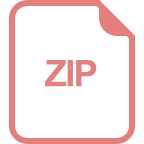
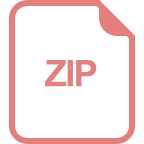
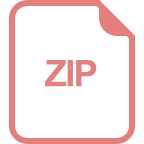
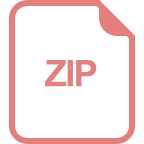
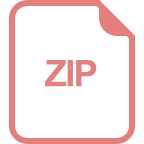
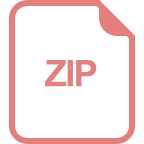
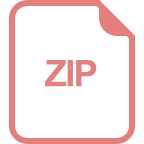
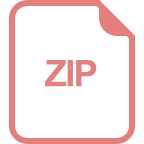
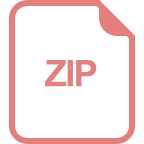
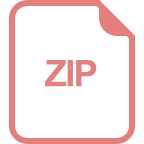
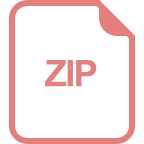
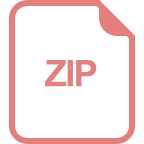
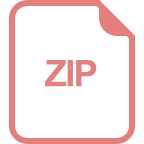
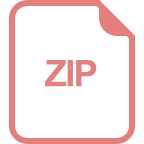
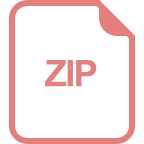
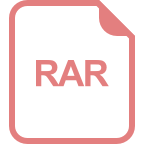
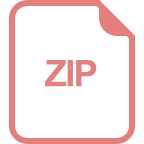
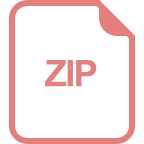
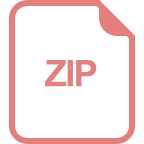
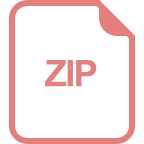
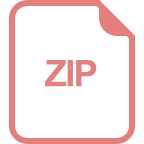
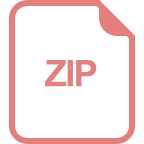
收起资源包目录

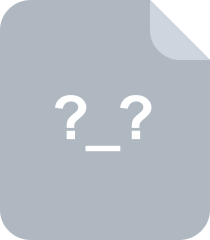
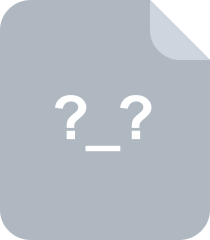
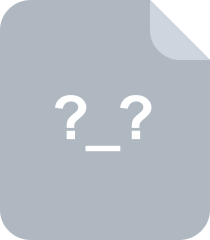
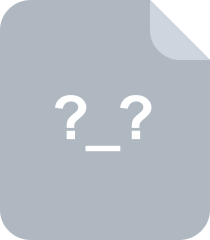
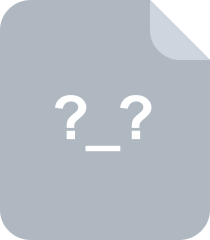
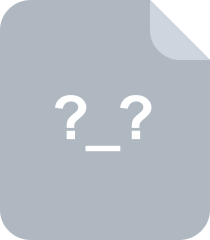
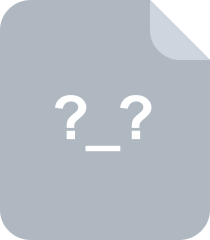
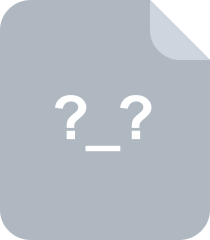
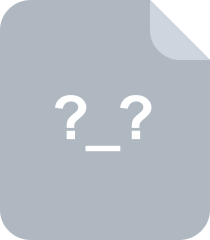
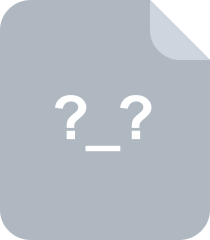
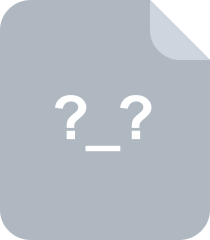
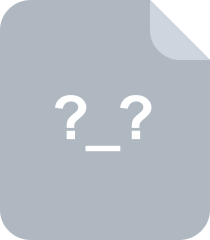
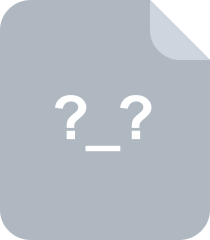
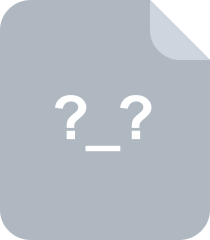
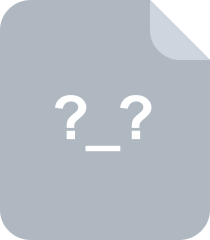
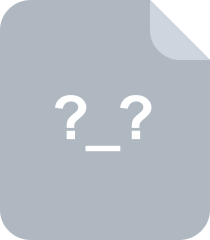
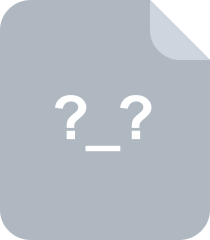
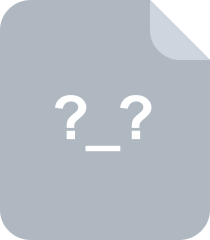
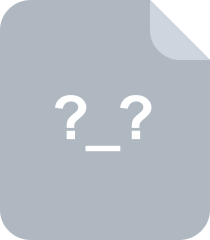
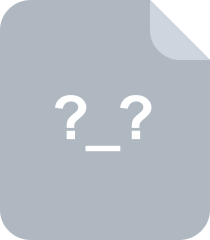
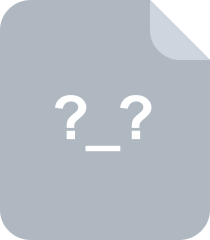
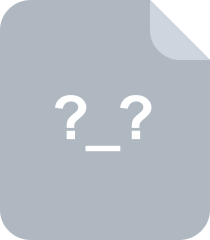
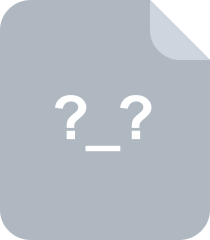
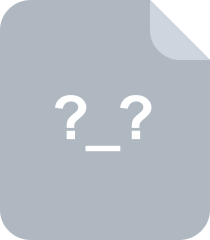
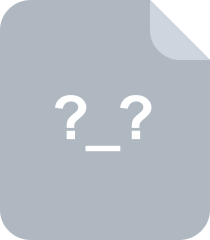
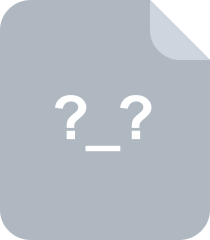
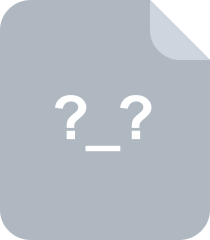
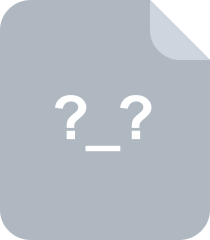
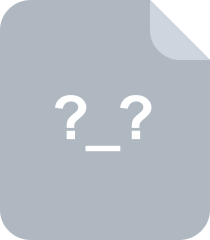
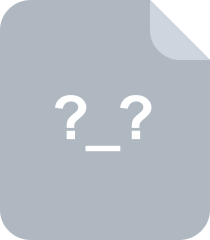
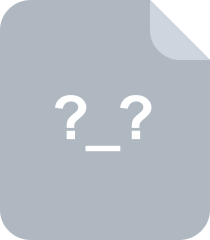
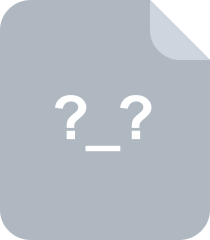
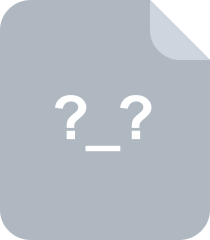
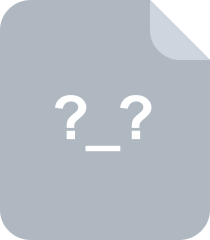
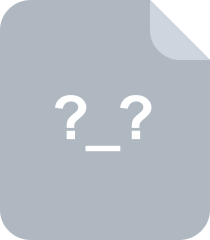
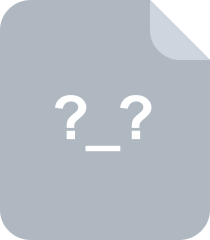
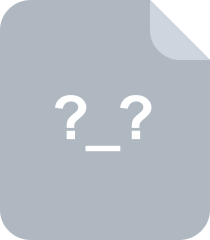
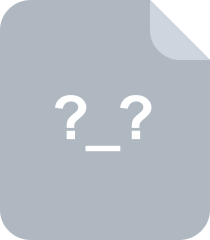
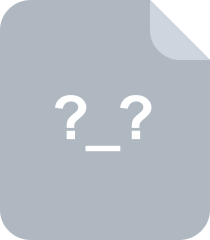
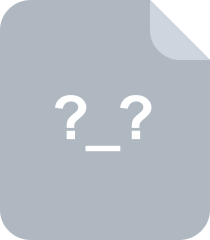
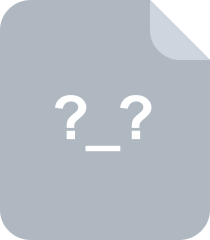
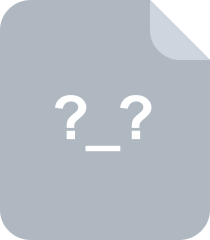
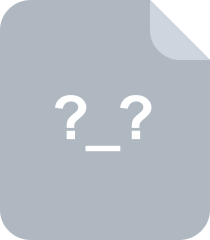
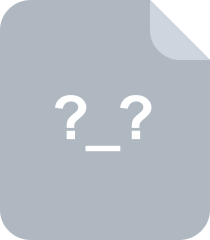
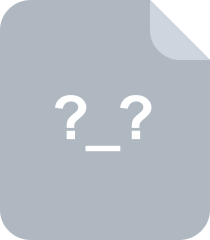
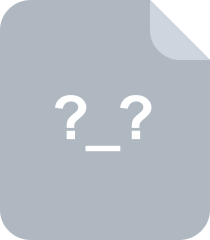
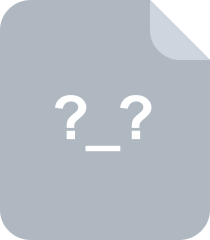
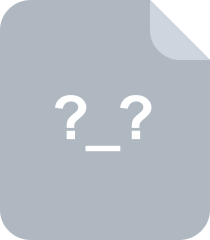
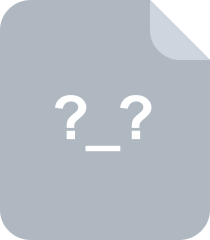
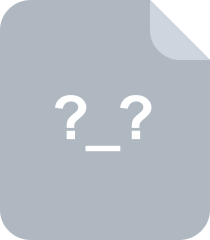
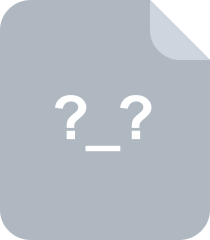
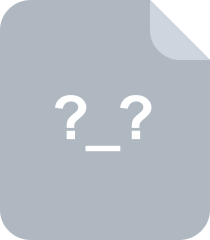
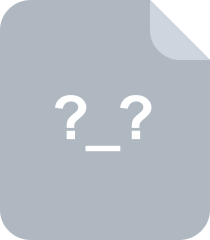
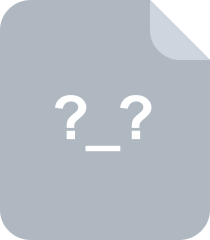
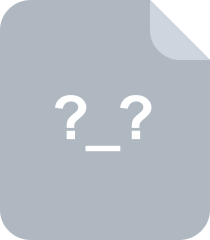
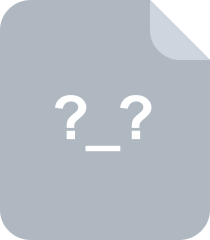
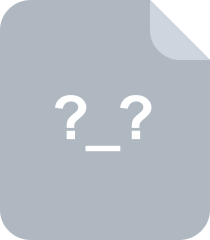
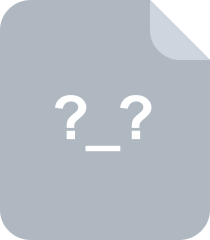
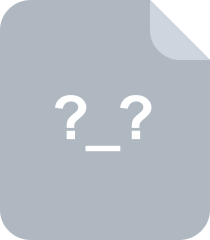
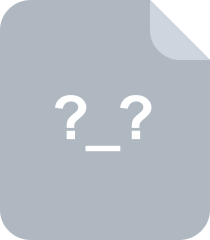
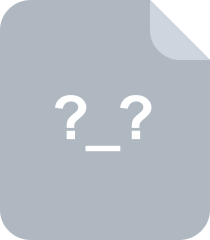
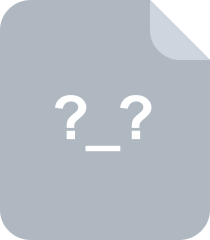
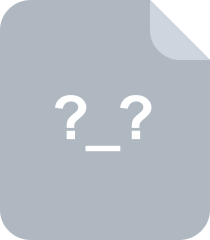
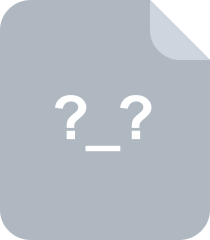
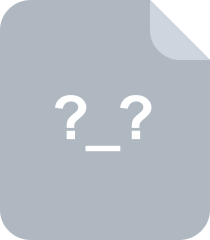
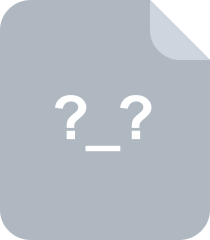
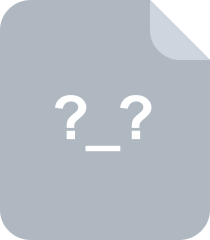
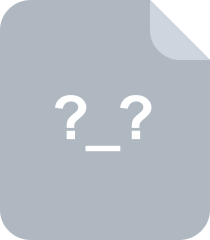
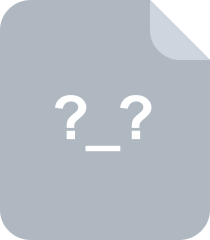
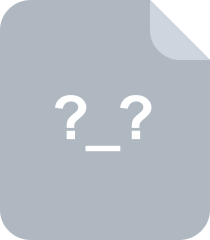
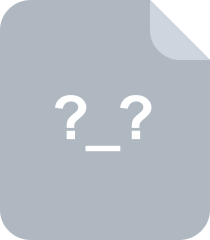
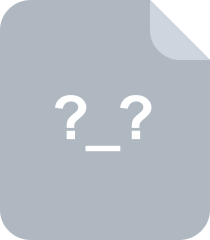
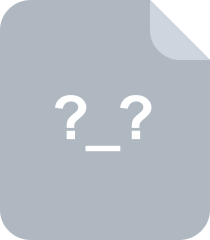
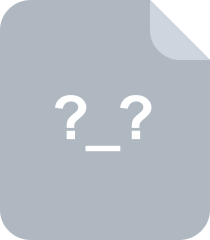
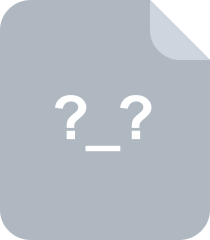
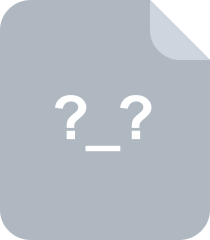
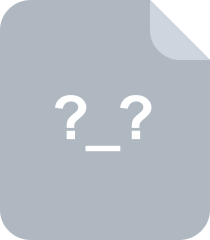
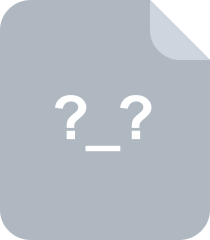
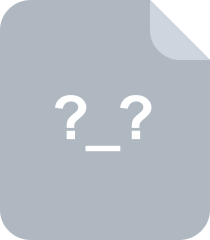
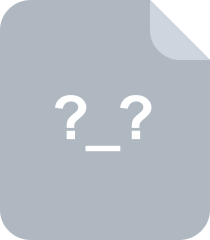
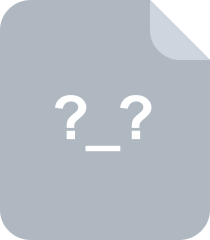
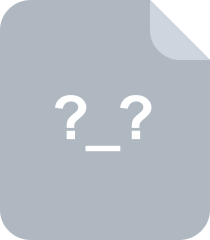
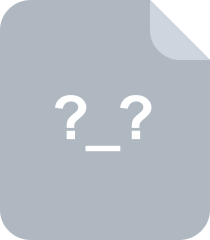
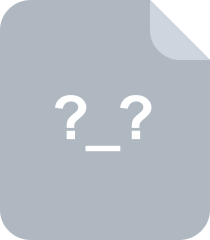
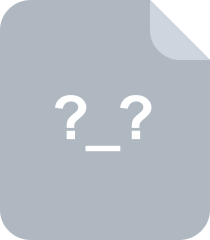
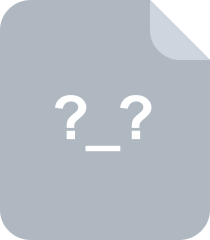
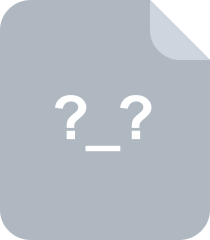
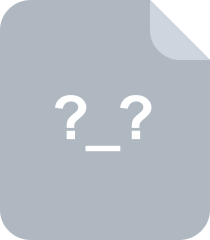
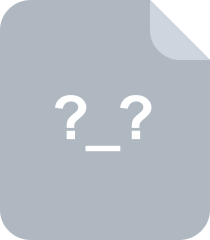
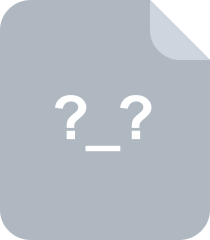
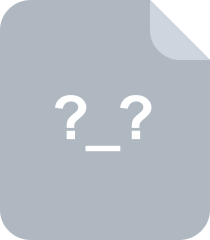
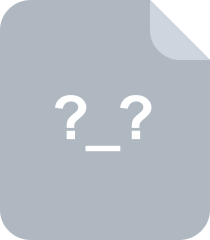
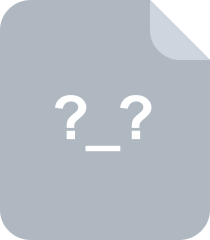
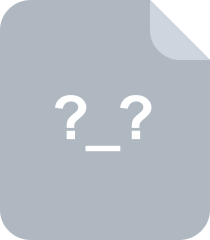
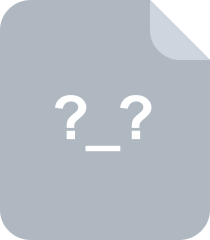
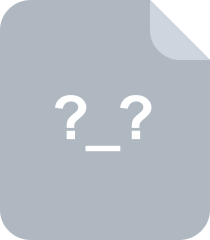
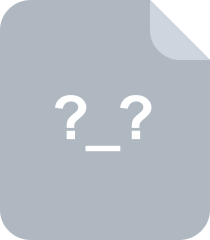
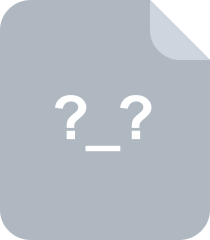
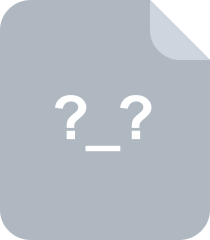
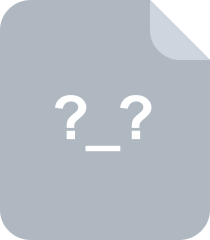
共 643 条
- 1
- 2
- 3
- 4
- 5
- 6
- 7
资源评论
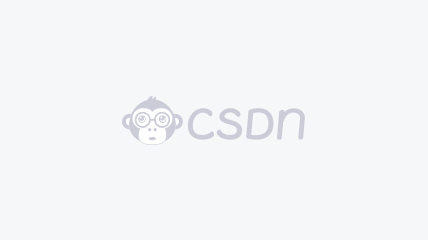
- 2301_779170842024-04-08资源很赞,希望多一些这类资源。


辣椒种子
- 粉丝: 4149
- 资源: 5805
上传资源 快速赚钱
我的内容管理 展开
我的资源 快来上传第一个资源
我的收益
登录查看自己的收益我的积分 登录查看自己的积分
我的C币 登录后查看C币余额
我的收藏
我的下载
下载帮助

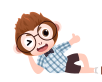
最新资源
资源上传下载、课程学习等过程中有任何疑问或建议,欢迎提出宝贵意见哦~我们会及时处理!
点击此处反馈


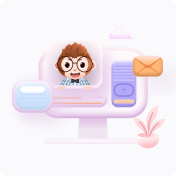
安全验证
文档复制为VIP权益,开通VIP直接复制
