[pypi-image]: https://badge.fury.io/py/MinkowskiEngine.svg
[pypi-url]: https://pypi.org/project/MinkowskiEngine/
[pypi-download]: https://img.shields.io/pypi/dm/MinkowskiEngine
[slack-badge]: https://img.shields.io/badge/slack-join%20chats-brightgreen
[slack-url]: https://join.slack.com/t/minkowskiengine/shared_invite/zt-piq2x02a-31dOPocLt6bRqOGY3U_9Sw
# Minkowski Engine
[![PyPI Version][pypi-image]][pypi-url] [![pypi monthly download][pypi-download]][pypi-url] [![slack chat][slack-badge]][slack-url]
The Minkowski Engine is an auto-differentiation library for sparse tensors. It supports all standard neural network layers such as convolution, pooling, unpooling, and broadcasting operations for sparse tensors. For more information, please visit [the documentation page](http://nvidia.github.io/MinkowskiEngine/overview.html).
## News
- 2021-08-11 Docker installation instruction added
- 2021-08-06 All installation errors with pytorch 1.8 and 1.9 have been resolved.
- 2021-04-08 Due to recent errors in [pytorch 1.8 + CUDA 11](https://github.com/NVIDIA/MinkowskiEngine/issues/330), it is recommended to use [anaconda for installation](#anaconda).
- 2020-12-24 v0.5 is now available! The new version provides CUDA accelerations for all coordinate management functions.
## Example Networks
The Minkowski Engine supports various functions that can be built on a sparse tensor. We list a few popular network architectures and applications here. To run the examples, please install the package and run the command in the package root directory.
| Examples | Networks and Commands |
|:---------------------:|:-------------------------------------------------------------------------------------------------------------------------------------------------------------------------------:|
| Semantic Segmentation | <img src="https://nvidia.github.io/MinkowskiEngine/_images/segmentation_3d_net.png"> <br /> <img src="https://nvidia.github.io/MinkowskiEngine/_images/segmentation.png" width="256"> <br /> `python -m examples.indoor` |
| Classification | 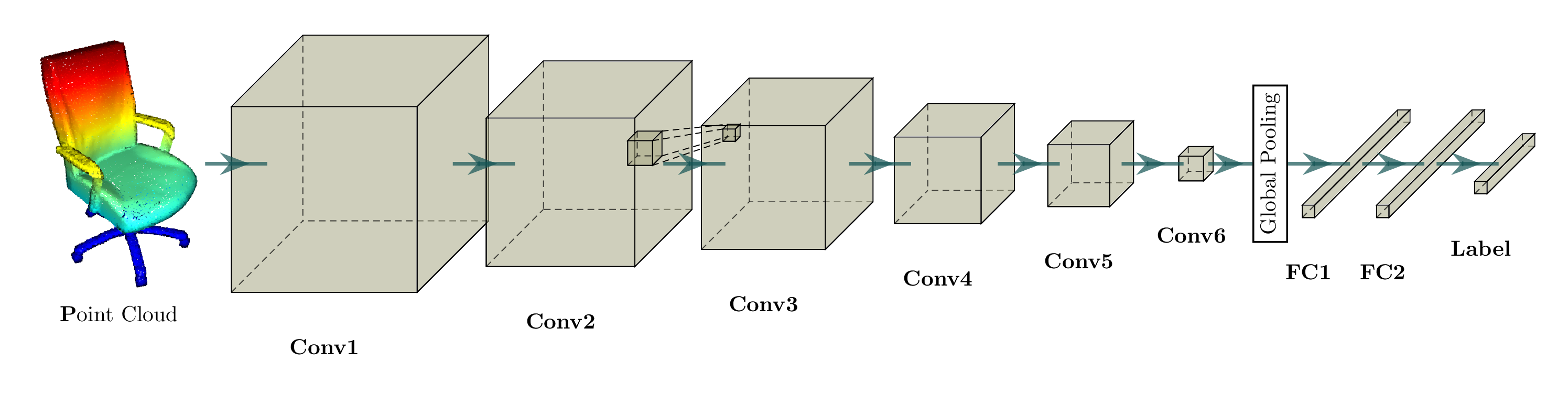 <br /> `python -m examples.classification_modelnet40` |
| Reconstruction | <img src="https://nvidia.github.io/MinkowskiEngine/_images/generative_3d_net.png"> <br /> <img src="https://nvidia.github.io/MinkowskiEngine/_images/generative_3d_results.gif" width="256"> <br /> `python -m examples.reconstruction` |
| Completion | <img src="https://nvidia.github.io/MinkowskiEngine/_images/completion_3d_net.png"> <br /> `python -m examples.completion` |
| Detection | <img src="https://nvidia.github.io/MinkowskiEngine/_images/detection_3d_net.png"> |
## Sparse Tensor Networks: Neural Networks for Spatially Sparse Tensors
Compressing a neural network to speedup inference and minimize memory footprint has been studied widely. One of the popular techniques for model compression is pruning the weights in convnets, is also known as [*sparse convolutional networks*](https://www.cv-foundation.org/openaccess/content_cvpr_2015/papers/Liu_Sparse_Convolutional_Neural_2015_CVPR_paper.pdf). Such parameter-space sparsity used for model compression compresses networks that operate on dense tensors and all intermediate activations of these networks are also dense tensors.
However, in this work, we focus on [*spatially* sparse data](https://arxiv.org/abs/1409.6070), in particular, spatially sparse high-dimensional inputs and 3D data and convolution on the surface of 3D objects, first proposed in [Siggraph'17](https://wang-ps.github.io/O-CNN.html). We can also represent these data as sparse tensors, and these sparse tensors are commonplace in high-dimensional problems such as 3D perception, registration, and statistical data. We define neural networks specialized for these inputs as *sparse tensor networks* and these sparse tensor networks process and generate sparse tensors as outputs. To construct a sparse tensor network, we build all standard neural network layers such as MLPs, non-linearities, convolution, normalizations, pooling operations as the same way we define them on a dense tensor and implemented in the Minkowski Engine.
We visualized a sparse tensor network operation on a sparse tensor, convolution, below. The convolution layer on a sparse tensor works similarly to that on a dense tensor. However, on a sparse tensor, we compute convolution outputs on a few specified points which we can control in the [generalized convolution](https://nvidia.github.io/MinkowskiEngine/sparse_tensor_network.html). For more information, please visit [the documentation page on sparse tensor networks](https://nvidia.github.io/MinkowskiEngine/sparse_tensor_network.html) and [the terminology page](https://nvidia.github.io/MinkowskiEngine/terminology.html).
| Dense Tensor | Sparse Tensor |
|:---------------------------------------------------------------------------:|:----------------------------------------------------------------------------:|
| <img src="https://nvidia.github.io/MinkowskiEngine/_images/conv_dense.gif"> | <img src="https://nvidia.github.io/MinkowskiEngine/_images/conv_sparse.gif"> |
--------------------------------------------------------------------------------
## Features
- Unlimited high-dimensional sparse tensor support
- All standard neural network layers (Convolution, Pooling, Broadcast, etc.)
- Dynamic computation graph
- Custom kernel shapes
- Multi-GPU training
- Multi-threaded kernel map
- Multi-threaded compilation
- Highly-optimized GPU kernels
## Requirements
- Ubuntu >= 14.04
- CUDA >= 10.1.243 and **the same CUDA version used for pytorch** (e.g. if you use conda cudatoolkit=11.1, use CUDA=11.1 for MinkowskiEngine compilation)
- pytorch >= 1.7 To specify CUDA version, please use conda for installation. You must match the CUDA version pytorch uses and CUDA version used for Minkowski Engine installation. `conda install -y -c nvidia -c pytorch pytorch=1.8.1 cudatoolkit=10.2`)
- python >= 3.6
- ninja (for installation)
- GCC >= 7.4.0
## Installation
You can install the Minkowski Engine with `pip`, with anaconda, or on the system directly. If you experience issues installing the package, please checkout the [the installation wiki page](https://github.com/NVIDIA/MinkowskiEngine/wiki/Installation).
If you cannot find a relevant problem, please report the issue on [the github issue page](https://github.com/NVIDIA/MinkowskiEngine/issues).
- [PIP](https://github.com/NVIDIA/MinkowskiEngine#pip) installation
- [Conda](https://github.com/NVIDIA/MinkowskiEngine#anaconda) installation
- [Python](https://github.com/NVIDIA/MinkowskiEngine#system-python) installation
- [Docker](https://github.com/NVIDIA/MinkowskiEngine#docker) installation
### Pip
The MinkowskiEngine is distributed via [PyPI MinkowskiEngine][pypi-url] which can be installed simply with `pip`.
First, install pytorch following the [instruction](https://pytorch.org). Next, install `openblas`.
```
sudo apt install build-essential python3-dev libopenblas-dev
pip install torch ninja
pip install -U MinkowskiEngine --install-option="--blas=openblas" -v --no-deps
# For pip installation from the latest source
# pip install -U git+https://github.com/NVIDIA/MinkowskiEngine --no-deps
```
If you want to specify arguments for the setup script, please refer to the following command.
```
# Uncomment some options if things don't work
# export CXX=c++; # set this if you want to u
Minkowski engine代码
需积分: 0 177 浏览量
更新于2024-02-20
收藏 5.62MB ZIP 举报
《Minkowski Engine:深度学习中的点云语义理解利器》
在当今的计算机视觉领域,3D点云数据处理正逐渐成为研究的热点。点云数据作为一种非结构化的数据形式,广泛存在于激光雷达、无人机、3D扫描仪等设备中,为自动驾驶、室内导航、虚拟现实等领域提供了丰富的信息。然而,由于其数据量大、结构复杂,处理起来颇具挑战。Minkowski Engine的出现,为解决这一问题提供了一种高效且灵活的解决方案。
Minkowski Engine 是一个专门用于处理3D点云数据的深度学习库,它主要由Python编写,并利用CUDA技术进行GPU加速,以实现高效计算。该库的核心是将3D点云数据转化为可被卷积神经网络(CNN)处理的格式,即所谓的“稀疏三维卷积”(Sparse 3D Convolution)。这种转换使得深度学习模型能直接对点云数据进行操作,避免了传统方法中繁琐的数据预处理步骤。
深度学习,尤其是卷积神经网络,在2D图像处理上取得了显著的成功。然而,直接将2D卷积应用到3D点云数据并不适用,因为3D空间的复杂性和数据的稀疏性。Minkowski Engine 引入了“体素化”(Voxelization)的概念,即将3D空间分割成小的立方体(体素),然后对每个体素内的点进行统计,如计数或平均,将其转换为连续的特征向量。接着,这些特征向量可以作为输入,通过稀疏卷积进行学习,从而处理整个3D空间。
在Minkowski Engine中,稀疏卷积运算的实现采用了动态图(Dynamic Graph)策略,这使得该库能够灵活地处理不同大小和密度的点云数据。与传统的固定大小网格相比,这种灵活性大大降低了内存需求,提高了计算效率,尤其是在处理大规模点云数据时更为明显。
Minkowski Engine 的代码库,如`MinkowskiEngine-master`,包含了完整的源代码、示例和文档,方便开发者进行二次开发和研究。开发者可以基于此进行点云语义分割、实例分割、3D目标检测等任务。同时,这个库支持多GPU并行计算,进一步提升了模型训练的速度。
在实际应用中,Minkowski Engine 已经被广泛用于自动驾驶领域的语义地图构建,以及室内环境的语义理解。通过这个库,研究人员和工程师可以构建出更精确的3D环境模型,提高智能系统的感知能力和决策质量。
Minkowski Engine是点云处理领域的一个重要工具,它结合了深度学习和3D几何的理解,提供了一种高效且灵活的点云数据处理方案。对于涉及3D点云的科研和工程实践,掌握Minkowski Engine的使用无疑将大大提升工作效率和研究水平。

kfchandsome
- 粉丝: 0
- 资源: 4
最新资源
- 西门子S7-1200流水线贴膜机项目程序,有KTP700触摸屏组态操作界面 程序包含.上下气缸控制,夹紧气缸控制,输送带电机控制,贴膜伺服控制旋转电机控制等类容 适合简单控制工艺及运动控制初学
- comsol电力变压器电磁场计算模型,通过简化高低压绕组,铁心,结构件和绝缘油的几何模型,得到变压器内部电磁场分布
- 西门子PLC1500大型程序 西门子PLC1500大型程序fanuc机器人焊装 包括1台西门子1500PLC程序,2台触摸屏TP1500程序 9个智能远程终端ET200SP Profinet连接 15
- Task-113-出租车计价
- HttpUrlConnectionUtilDemo-大炮打蚊子
- fishing-game-大炮打蚊子
- 有限信息,多智能体系统,鲁棒无碰撞编队控制,有文献参考 符合要求请放心联系,MATLAB,保证能够运行 simulink,复现
- MATLAB全桥或者半桥LLC谐振DC DC变器的设计与仿真 内含开环仿真、电压闭环仿真两个仿真文件 并含有电路参数仿真计算过程 支持最高版本2018b
- 基于模型参考自适应的永磁同步电机无感FOC 1.采用模型参考自适应MRAS来估计转速和转子位置,实现中高速下PMSM的无感运行,估计精度较高; 2.提供算法对应的参考文献和仿真模型,支持技术解答;拿后
- hustoj-重庆大学python题库答案
- 基于阻抗的微电网功率分配策略研究(文章复现),关键词:下垂控制,并联逆变器,阻抗
- Util-大炮打蚊子c++
- 一种微电网分布式电源的下垂控制策略(文章复现),关键词:下垂控制,并联逆变器,对等控制
- 汽车手动变速器sw18可编辑全套设计资料100%好用.zip
- 基于PreScan与Carsim的车道保持联合仿真 其中车道线识别算法通过采集单目摄像头数据,Ransac算法提取车道线;采用MPC控制器实现车道保持与循迹控制 内附
- 西门子1200博图程序冷却油泵PID控制系统,和多台油泵及水泵G120西门子变频器Modbud RTU通讯,画面采用西门子KTP700触摸屏,内有变频器参数 Modbus通讯报文详细讲解,PID带手动