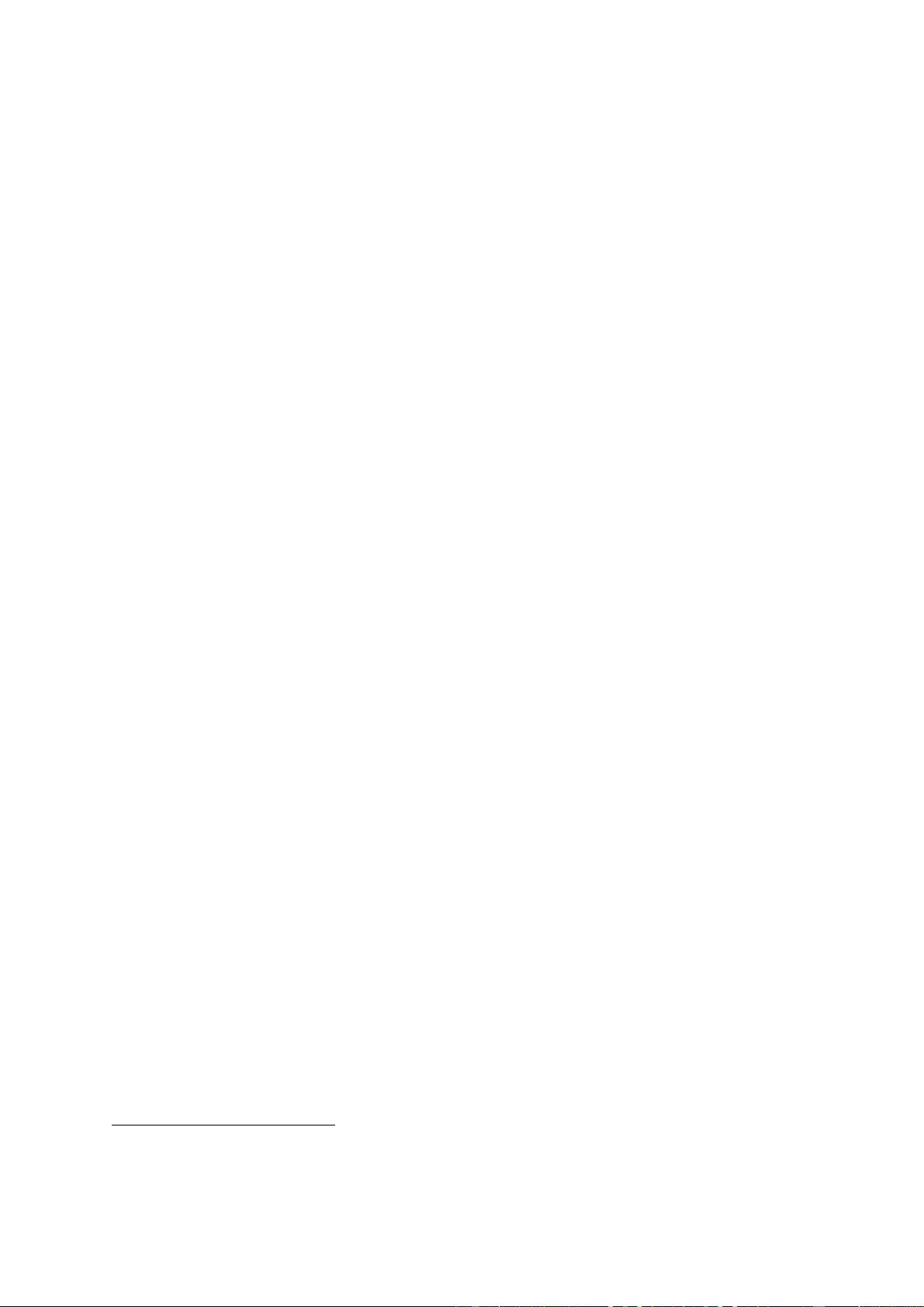
The MATLAB Genetic Algorithm Toolbox
A. J. Chipperfield and P. J. Fleming
1
1. Introduction
Genetic algorithms (GAs) are stochastic global search and optimization methods that mimic the metaphor of natural
biological evolution [1]. GAs operate on a population of potential solutions applying the principle of survival of the
fittest to produce successively better approximations to a solution. At each generation of a GA, a new set of
approximations is created by the process of selecting individuals according to their level of fitness in the problem
domain and reproducing them using operators borrowed from natural genetics. This process leads to the evolution of
populations of individuals that are better suited to their environment than the individuals from which they were
created, just as in natural adaptation.
GAs have been shown to be an effective strategy in the off-line design of control systems by a number of
practitioners. For example, Krishnakumar and Goldberg [2] and Bramlette and Cusin [3] have demonstrated how
genetic optimization methods can be used to derive superior controller structures in aerospace applications in less
time (in terms of function evaluations) than traditional methods such as LQR and Powell’s gain set design. Porter and
Mohamed [4] have presented schemes for the genetic design of multivariable flight control systems using
eigenstructure assignment, whilst others have demonstrated how GAs can be used in the selection of controller
structures [5].
2. The MATLAB GA Toolbox
Whilst there exist many good public-domain genetic algorithm packages, such as GENESYS [6] and GENITOR [7],
none of these provide an environment that is immediately compatible with existing tools in the control domain. The
MATLAB Genetic Algorithm Toolbox [8] aims to make GAs accessible to the control engineer within the framework
of an existing CACSD package. This allows the retention of existing modelling and simulation tools for building
objective functions and allows the user to make direct comparisons between genetic methods and traditional
procedures.
2.1 Data Structures
MATLAB essentially supports only one data type, a rectangular matrix of real or complex numeric elements. The main
data structures in the GA Toolbox are chromosomes, phenotypes, objective function values and fitness values. The
chromosome structure stores an entire population in a single matrix of size N
ind
× L
ind
, where N
ind
is the number of
individuals and L
ind
is the length of the chromosome structure. Phenotypes are stored in a matrix of dimensions N
ind
× N
var
where N
var
is the number of decision variables. An N
ind
× N
obj
matrix stores the objective function values,
where N
obj
is the number of objectives. Finally, the fitness values are stored in a vector of length N
ind
. In all of these
data structures, each row corresponds to a particular individual.
2.2 Toolbox Structure
The GA Toolbox uses MATLAB matrix functions to build a set of versatile routines for implementing a wide range of
genetic algorithm methods. In this section we outline the major procedures of the GA Toolbox.
Population representation and initialisation: crtbase, crtbp, crtrp
The GA Toolbox supports binary, integer and floating-point chromosome representations. Binary and integer
populations may be initialised using the Toolbox function to create binary populations, crtbp. An additional
function, crtbase, is provided that builds a vector describing the integer representation used. Real-valued
populations may be initialised using crtrp. Conversion between binary and real-values is provided by the routine
bs2rv that also supports the use of Gray codes and logarithmic scaling.
Fitness assignment: ranking, scaling
The fitness function transforms the raw objective function values into non-negative figures of merit for each
individual. The Toolbox supports the offsetting and scaling method of Goldberg [9] and the linear-ranking algorithm
1. Department of Automatic Control and Systems Engineering, University of Sheffield, PO Box 600,
Mappin Street, Sheffield, England. S1 4DU
From IEE Colloquium on Applied Control Techniques Using MATLAB, Digest No. 1995/014, 26/01/95