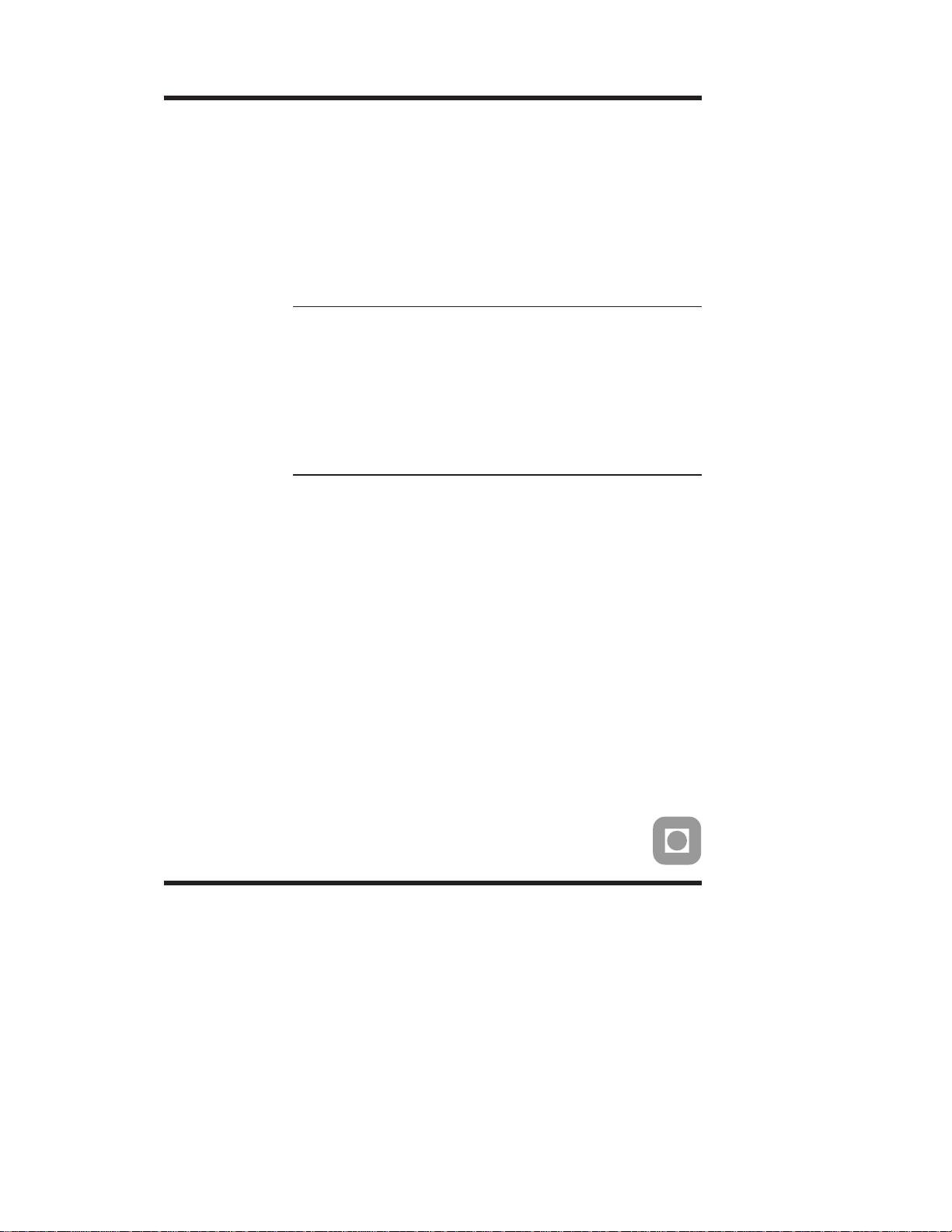
Aleksander Øhrn
Discernibility and Rough
Sets in Medicine:
Tools and Applications
Department of Computer and Information Science
Norwegian University of Science and Technology
N-7491 Trondheim, Norway
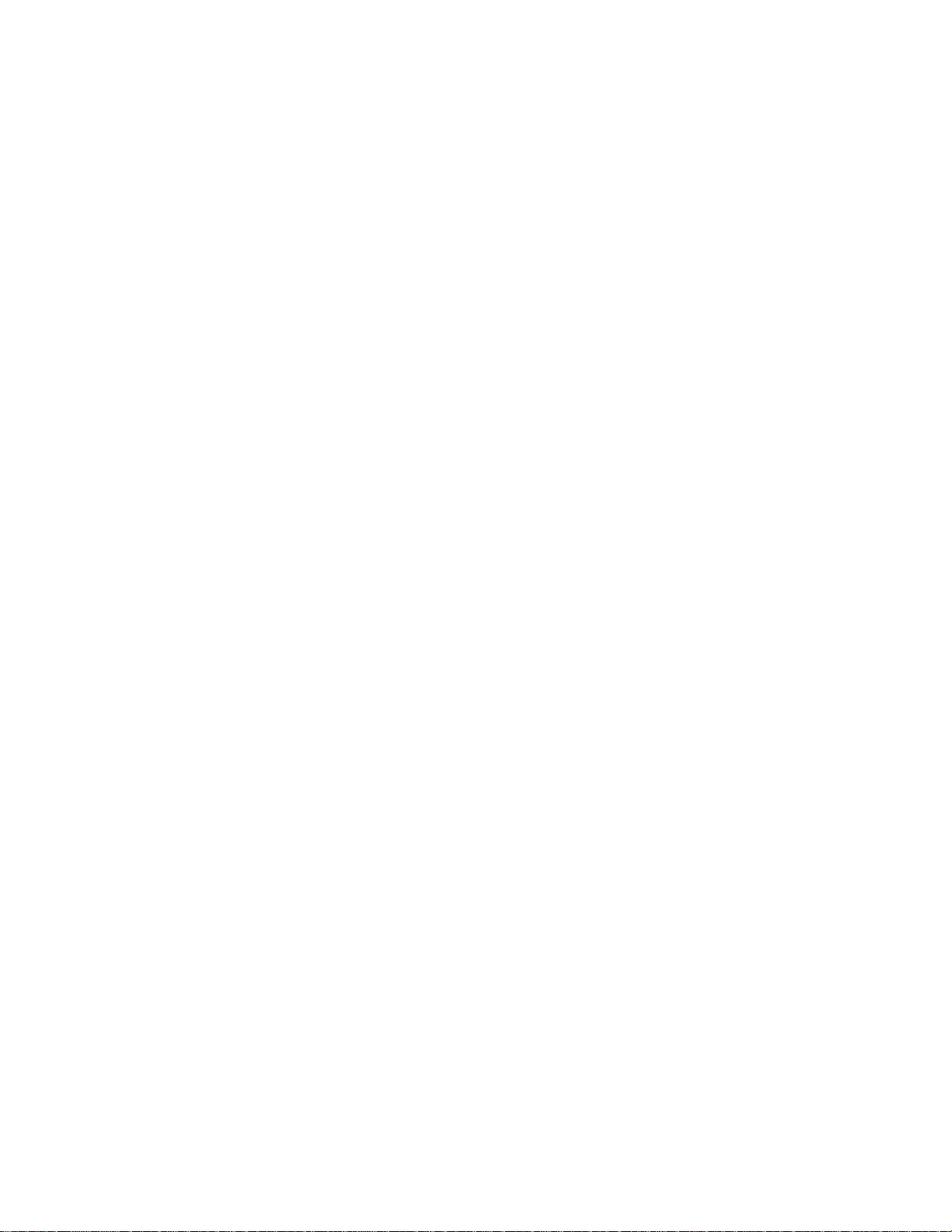
NTNU Trondheim
Norges teknisk-naturvitenskapelige universitet
Doktor ingeniøravhandling 1999:133
Institutt for datateknikk og informasjonsvitenskap
IDI-rapport 1999:14
ISBN 82-7984-014-1
ISSN 0802-6394
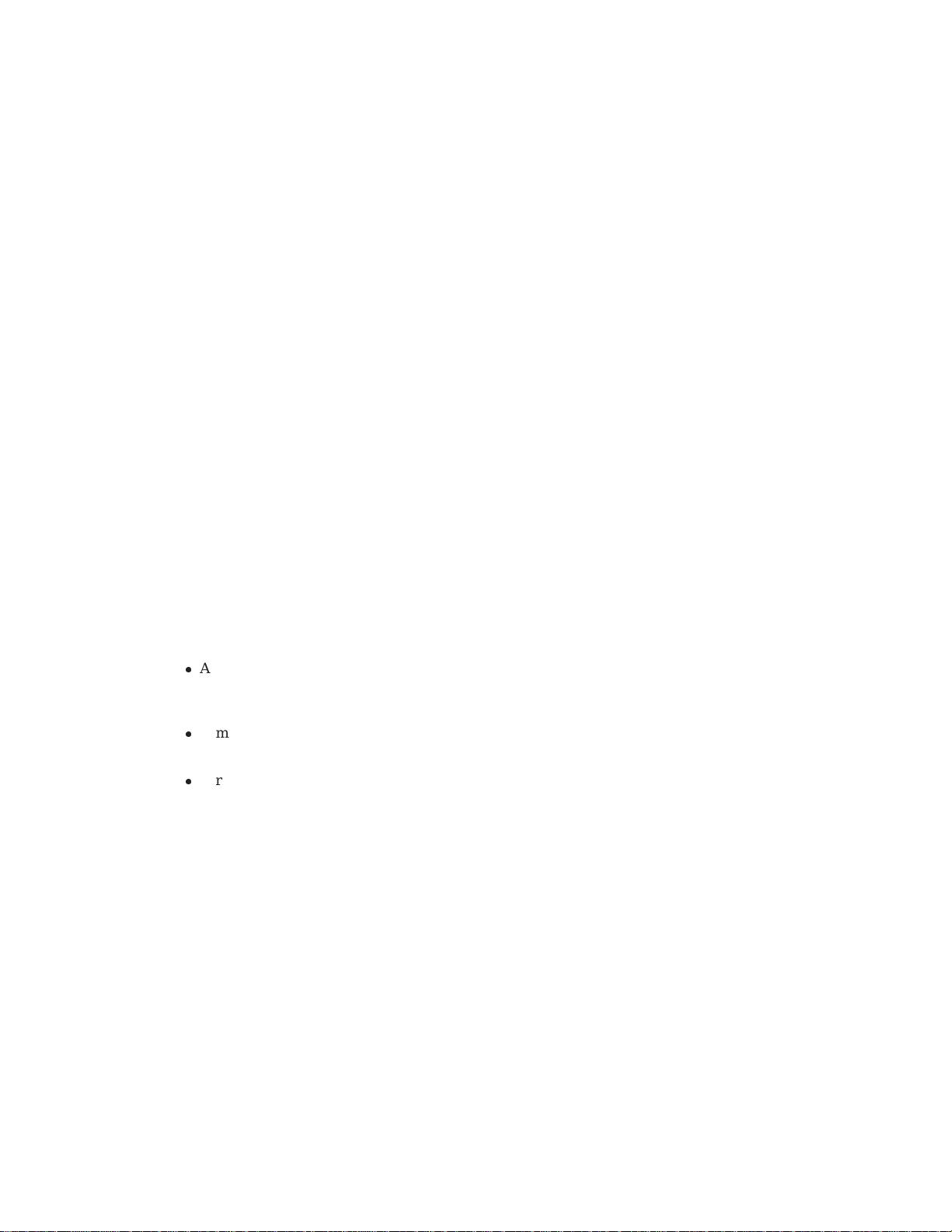
Abstract
This thesis examines how discernibility-based methods can be equipped to posses
several qualities that are needed for analyzing tabular medical data, and how these
models can be evaluated according to current standard measures used in the health
sciences. To this end, tools have been developed that make this possible, and some
novel medical applications have been devised in which the tools are put to use.
Rough set theory provides a framework in which discernibility-based methods can be
formulated and interpreted, and also forms an appealing foundation for data mining
and knowledge discovery. When the medical domain is targeted, several factors be-
come important. This thesis examines some of these factors, and holds them up to the
current state-of-the-art in discernibility-based empirical modelling. Bringing together
pertinent techniques, suitable adaptations of relevant theory for model construction
and assessment are presented. Rough set classifiers are brought together with ROC
analysis, and it is outlined how attribute costs and semantics can enter the modelling
process.
ROSETTA, a comprehensive software system for conducting data analyses within the
framework of rough set theory, has been developed. Under the hypothesis that the ac-
cessibility of such tools lowers the threshold for abstract ideas to migrate into concrete
realization, this aids in reducing a gap between theoreticians and practitioners, and
enables existing problems to be more easily attacked. The ROSETTA system boasts a
set of flexible and powerful algorithms, and sets these in a user-friendly environment
designed tosupport all phases of the discernibility-based modelling methodology. Re-
searchers world-wide have alreadyput the system touse in a wide variety of domains.
By and large, discernibility-based data analysis can be varied along two main axes:
Which objects in the universe of discourse that we deem it necessary to discern be-
tween, and how we define that discernibility among these objects is allowed to take
place. Using ROSETTA, this thesis has explored various facets of this also in three
novel and distinctly different medical applications:
A method is proposed for identifying population subgroups for which expen-
sive tests may be avoided, and experiments with a real-world database on a
cardiological prognostic problem suggest that significant savings are possible.
A method is proposed for anonymizing medical databases with sensitive con-
tents via cell suppression, thus aiding to preserve patient confidentiality.
Very simple rule-based classifiers are employed to diagnose acute appendicitis,
and their relative performance is compared to a team of experienced surgeons.
The added value of certain biochemical tests is also demonstrated.
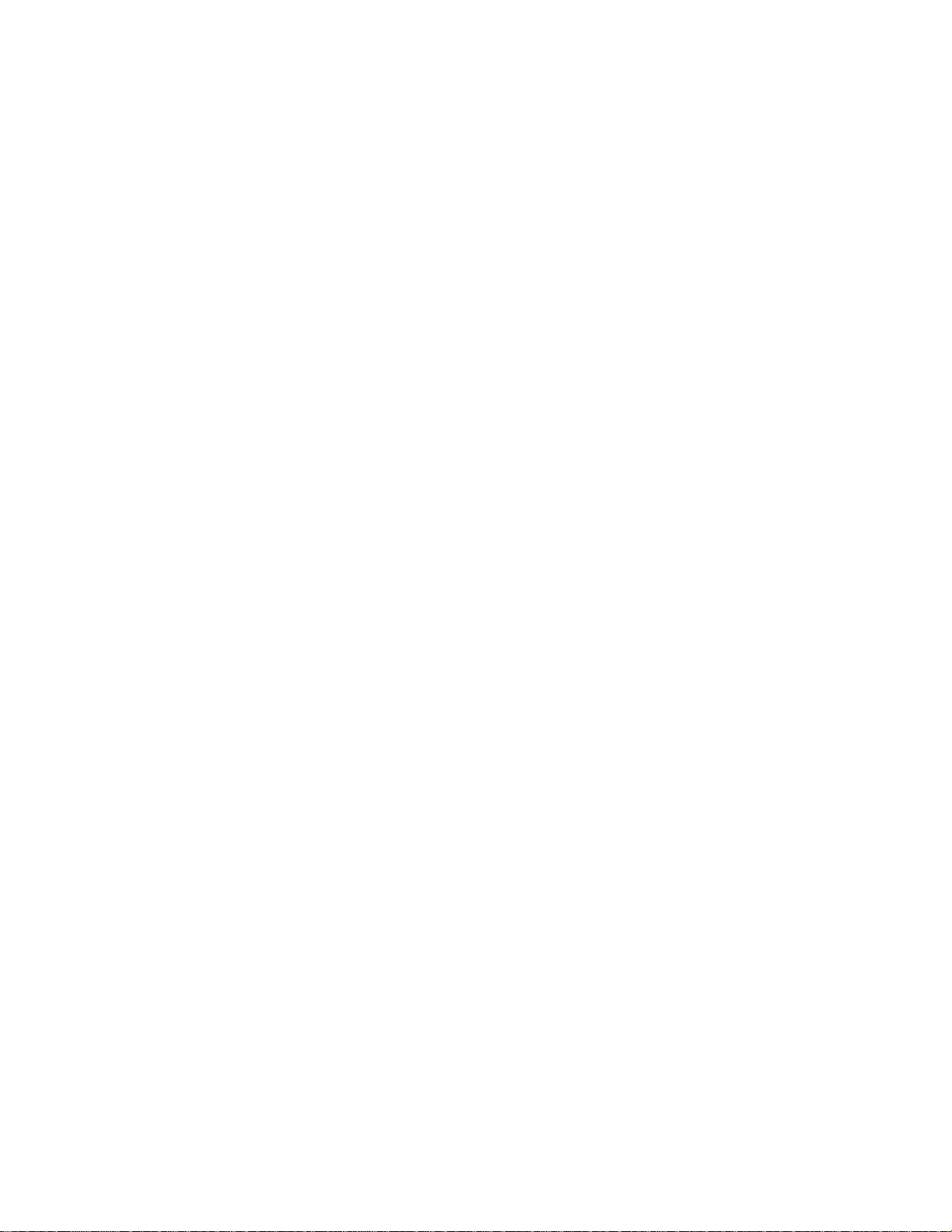
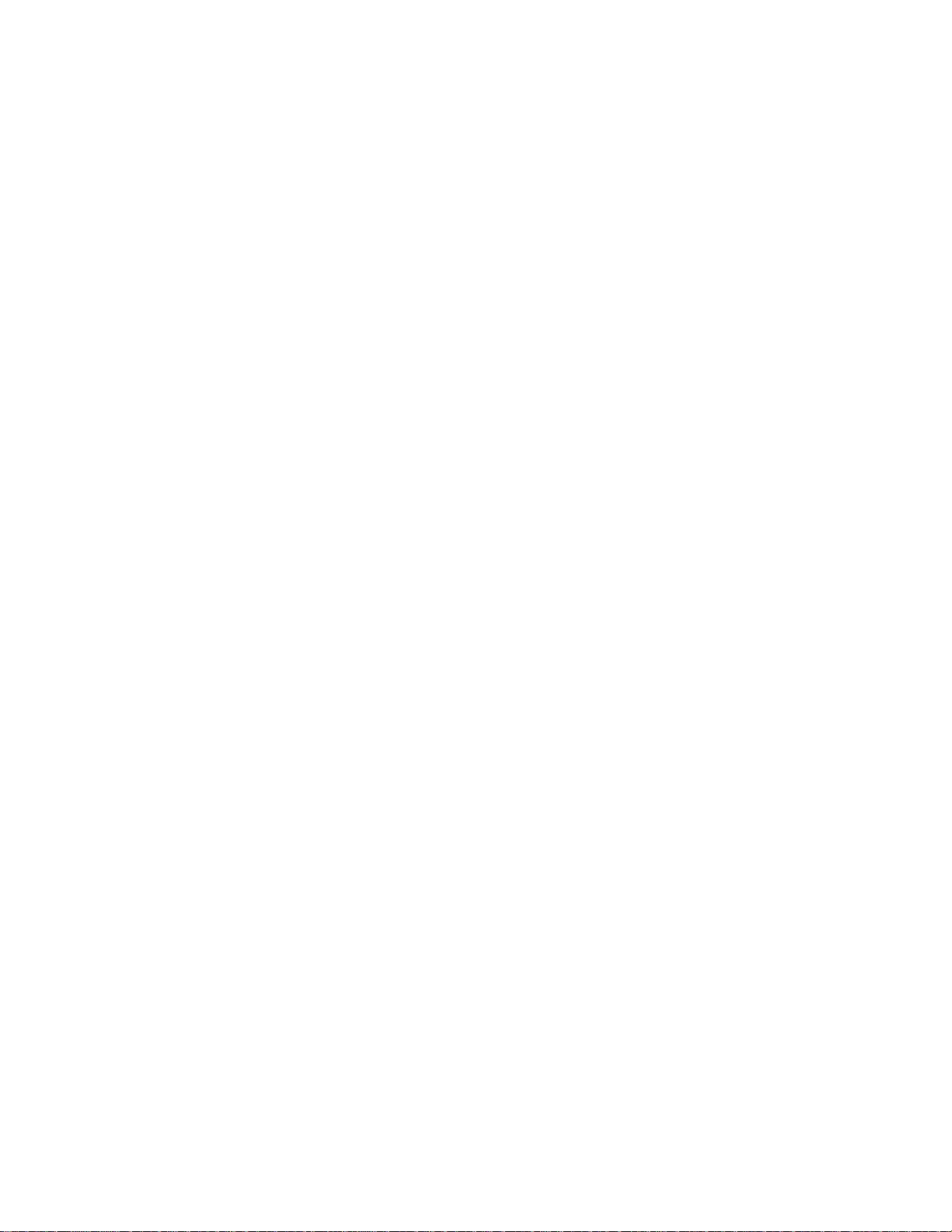
Contents
Preface xiii
I Setting 1
1 Introduction 3
1.1 Introduction . . . ................................ 3
1.2 Objectives . . . . ................................ 4
1.3 Results . . . . . . ................................ 5
1.4 Thesis Outline . . ................................ 6
1.4.1 A Roadmap . . . . . . . . . ...................... 8
2 Context 11
2.1 Introduction . . . ................................ 11
2.2 Data Mining and Knowledge Discovery . . . . . . . . . . ......... 12
2.2.1 The KDD Process . . . . . . ...................... 12
2.2.2 KDD and Rough Sets . . . . ...................... 14
2.2.3 Themes in KDD . . . . . . . ...................... 15
2.3 Medical Informatics . . . . . . . . . ...................... 16
2.3.1 Themes in Medical Informatics . . . . . . . . . . . ......... 17
2.4 Aspects of KDD in Medicine . . . . ...................... 18
2.4.1 On Data Availability and Quality . . . . . . . . . . ......... 18
2.4.2 On Model Induction and Selection . . . . . . . . . ......... 20
2.4.3 On Model Assessment and Deployment . . . . . . ......... 21
3 Rough Sets in Medicine 25
3.1 Introduction . . . ................................ 25
3.2 Literature Review................................ 25
3.2.1 Diagnosis and Outcome Prediction . . . . . . . . . ......... 26
3.2.2 Feature Selection . . . . . . ...................... 27
3.2.3 Miscellaneous . . . . . . . . ...................... 28
3.3 Appeal . . . . . . ................................ 29
3.4 Discussion . . . . ................................ 30
3.4.1 On Prerequisites and Assumptions . . . . . . . . . ......... 30
3.4.2 On Interpretation and Deployment . . . . . . . . . ......... 31
3.4.3 On Model Maintenance and Assessment . . . . . ......... 32
iii