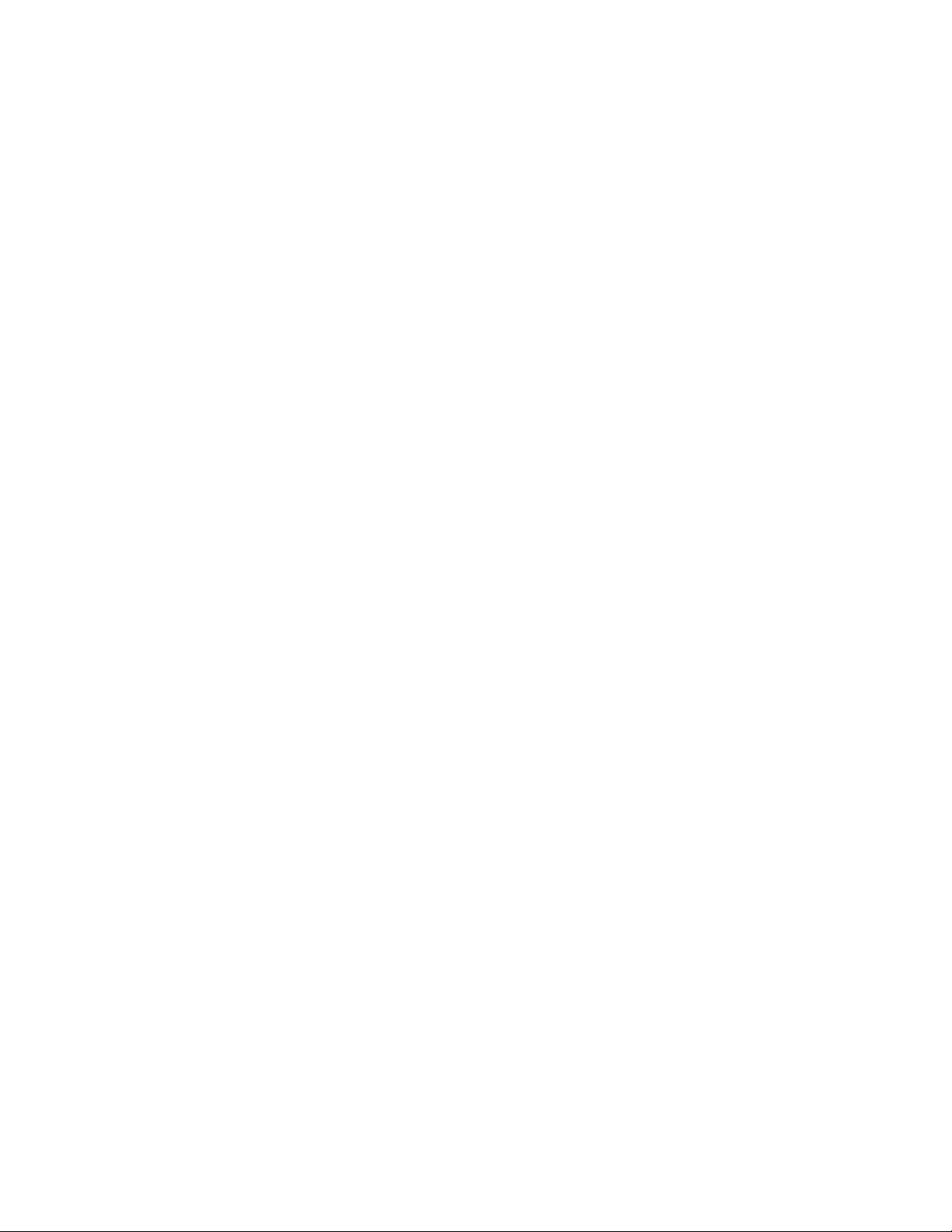
better ensure that at-risk u sers can engage safely online, and
in the process, to improve digital safety for everyone.
II. WHO ARE AT-RISK USERS?
To provide co ntext, we discuss terminology used in this
work, outline prio r taxonomies of attacks an d harms that
influenced our framework, and underscore the necessity for
the dig ital-safety community to su pport at-risk users.
A. Defining at-risk users
We use at-risk user as an umbrella term for anyone who ex-
periences heightened digital-safety threats. We r efer to groups
targeted by t hreats t hat affect an entire population—such as
children or teenagers navigating online privacy with peers
and family [107]—as at-risk populations. We did not find
consensus across the digital-safety community on how to refer
to such users or populations; we chose these terms with the
goal of drawing focus to external t hreats these users face.
B. Previous taxonomies of attacks, threats, and harms
Previous systematizations developed frameworks to br oadly
categorize attacks, threats, and harms, alt hough none capture
how these elements overlap or differ across distinct at-risk
populations. In particular, Scheuerman et al. [80] and Thomas
et al. [91] develop e d frameworks for un derstanding classes
of h arms that may result from digital-safety attacks, such
as reputational harm, financial harm, reduced sexual safety,
reduced physical safety, an d coercion. Scheuerman et al. [80]
also provided a framework for assessing the severity of threats
based on such harms. Thomas et al. [91], Sambasivan et
al. [76], and Levy a nd Schneier [51] detailed how attacks
vary based on the capabilities of attackers, such as having
intimate access to a target, or privileged access to a target’s
devices or data. Our at-risk framework differs in that we
isolate the contextual risk factors that may make at-risk users
particularly vulnerable to such atta cks, threats, or harms. We
also docu ment common protective practices at-risk users adopt
and discuss barriers they face to staying safe.
C. Value of focusing on at-risk users
The challenges experienced by at-risk users can be in-
ordinately complex, reflecting broade r, societal “structural
inequalities and soc ia l norms” [ 30, 5 7]. Th ese inequalities,
which vary globally, mean that particular c are is require d
to integrate at-risk users’ experiences and identities into the
design process [43, 57, 98].
We advocate for increased focus on at-risk user s’ nee ds
among the digital-safety c ommunity during threat modeling,
research, and design. Accounting for at-risk users can a lso
elevate the digital safety of all users by making “more pro-
nounc e d the need[s] th at many of us have” [24]. Providing
better digital-safety tools and guarantees can have far-reaching
impact both to at-risk users an d general users. Additionally,
providing choices and controls for at-risk users who know
intimately the digital-safety threats they face can also benefit
general users who may desire similar protections.
III. METHODS
We synthesize 85 research papers from a cross-section
of computer scienc e conferences. Here, we discuss h ow we
identified and analyzed these papers.
A. Paper selection
Our dataset for this analysis was 85 papers describing
digital- safety-related issues for various at-risk populations. Our
goal was not to obtain an exhaustive survey of relevant papers
but to collect a diverse set that would allow us to extract
theme s relevant to our research questions
2
.
We collected papers from five years (2016–2020) of con-
ferences spanning the security, privacy, and hu man-computer
interaction (HCI) communities: CCS, CHI, CSCW, IEEE S&P,
NDSS, PETS, SOUPS, and USENIX Security. We first gath-
ered links to every paper from these conferences on DBLP.
3
From those links, we collected paper titles, abstracts, and
publication dates, resulting in 6,428 papers.
To refine this list, three researchers independently read titles
and abstracts for each paper and marked them as ‘relevant’ to
our research questions or not. At this stage, we interpreted
relevance broadly, selecting any paper even slightly within
scope. Papers that no researcher marked as relevant were
removed. Papers marked as relevant by only one r e searcher
were reviewed by a fourth researcher and discussed. This
process identified 115 potentially relevant papers.
Authors with extensive exper ience working with at-risk
populations also added 12 papers from other sources and/or
from outside the target date range, in order to cover a broader
range o f populations, for a total of 127.
B. Codebook development
Our goal was to identify contextual risk factors, protective
practices, and other patterns discussed by the papers in ou r
dataset (Table I). A s a first step, we inductively built a cod e-
book by a nalyzing, in detail, a subset of papers well-aligned
with our research questions. Most of the core concepts in our
framework were identified at this stage, altho ugh inductive
refinement continued throughout our analysis.
To select this initial subset, we extracted from t he dataset
an initial list of populations (e. g., survivors of intimate part-
ner abuse [ 56], refugees [8 4], activists [20], children [109],
etc.). We also synthesized an initial list of risk factors, for
example, attributes of the population or the threats t hey faced
that contributed to their digital-safety-related risks. We then
selected our subset to ensure each population and risk factor on
our list was represented, m a king sur e to include some papers
that combined multiple risk factors (e.g., low-incom e African
American New York City residents [26] and foster teens [10]).
This process yielded 27 papers. Although we endeavored to
cover a broad range of pop ulations and risk factors, it was
not necessary that the subset be exhaustive; all paper s were
eventually analyzed, and we con tinued to refine the codebook
throughout our analysis.
2
The complete list of 85 papers in t he dataset can be found at
this link.
3
https://dblp.uni-trier.de/search
2