# YOLOv7 on Triton Inference Server
Instructions to deploy YOLOv7 as TensorRT engine to [Triton Inference Server](https://github.com/NVIDIA/triton-inference-server).
Triton Inference Server takes care of model deployment with many out-of-the-box benefits, like a GRPC and HTTP interface, automatic scheduling on multiple GPUs, shared memory (even on GPU), dynamic server-side batching, health metrics and memory resource management.
There are no additional dependencies needed to run this deployment, except a working docker daemon with GPU support.
## Export TensorRT
See https://github.com/WongKinYiu/yolov7#export for more info.
```bash
#install onnx-simplifier not listed in general yolov7 requirements.txt
pip3 install onnx-simplifier
# Pytorch Yolov7 -> ONNX with grid, EfficientNMS plugin and dynamic batch size
python export.py --weights ./yolov7.pt --grid --end2end --dynamic-batch --simplify --topk-all 100 --iou-thres 0.65 --conf-thres 0.35 --img-size 640 640
# ONNX -> TensorRT with trtexec and docker
docker run -it --rm --gpus=all nvcr.io/nvidia/tensorrt:22.06-py3
# Copy onnx -> container: docker cp yolov7.onnx <container-id>:/workspace/
# Export with FP16 precision, min batch 1, opt batch 8 and max batch 8
./tensorrt/bin/trtexec --onnx=yolov7.onnx --minShapes=images:1x3x640x640 --optShapes=images:8x3x640x640 --maxShapes=images:8x3x640x640 --fp16 --workspace=4096 --saveEngine=yolov7-fp16-1x8x8.engine --timingCacheFile=timing.cache
# Test engine
./tensorrt/bin/trtexec --loadEngine=yolov7-fp16-1x8x8.engine
# Copy engine -> host: docker cp <container-id>:/workspace/yolov7-fp16-1x8x8.engine .
```
Example output of test with RTX 3090.
```
[I] === Performance summary ===
[I] Throughput: 73.4985 qps
[I] Latency: min = 14.8578 ms, max = 15.8344 ms, mean = 15.07 ms, median = 15.0422 ms, percentile(99%) = 15.7443 ms
[I] End-to-End Host Latency: min = 25.8715 ms, max = 28.4102 ms, mean = 26.672 ms, median = 26.6082 ms, percentile(99%) = 27.8314 ms
[I] Enqueue Time: min = 0.793701 ms, max = 1.47144 ms, mean = 1.2008 ms, median = 1.28644 ms, percentile(99%) = 1.38965 ms
[I] H2D Latency: min = 1.50073 ms, max = 1.52454 ms, mean = 1.51225 ms, median = 1.51404 ms, percentile(99%) = 1.51941 ms
[I] GPU Compute Time: min = 13.3386 ms, max = 14.3186 ms, mean = 13.5448 ms, median = 13.5178 ms, percentile(99%) = 14.2151 ms
[I] D2H Latency: min = 0.00878906 ms, max = 0.0172729 ms, mean = 0.0128844 ms, median = 0.0125732 ms, percentile(99%) = 0.0166016 ms
[I] Total Host Walltime: 3.04768 s
[I] Total GPU Compute Time: 3.03404 s
[I] Explanations of the performance metrics are printed in the verbose logs.
```
Note: 73.5 qps x batch 8 = 588 fps @ ~15ms latency.
## Model Repository
See [Triton Model Repository Documentation](https://github.com/triton-inference-server/server/blob/main/docs/model_repository.md#model-repository) for more info.
```bash
# Create folder structure
mkdir -p triton-deploy/models/yolov7/1/
touch triton-deploy/models/yolov7/config.pbtxt
# Place model
mv yolov7-fp16-1x8x8.engine triton-deploy/models/yolov7/1/model.plan
```
## Model Configuration
See [Triton Model Configuration Documentation](https://github.com/triton-inference-server/server/blob/main/docs/model_configuration.md#model-configuration) for more info.
Minimal configuration for `triton-deploy/models/yolov7/config.pbtxt`:
```
name: "yolov7"
platform: "tensorrt_plan"
max_batch_size: 8
dynamic_batching { }
```
Example repository:
```bash
$ tree triton-deploy/
triton-deploy/
└── models
└── yolov7
├── 1
│ └── model.plan
└── config.pbtxt
3 directories, 2 files
```
## Start Triton Inference Server
```
docker run --gpus all --rm --ipc=host --shm-size=1g --ulimit memlock=-1 --ulimit stack=67108864 -p8000:8000 -p8001:8001 -p8002:8002 -v$(pwd)/triton-deploy/models:/models nvcr.io/nvidia/tritonserver:22.06-py3 tritonserver --model-repository=/models --strict-model-config=false --log-verbose 1
```
In the log you should see:
```
+--------+---------+--------+
| Model | Version | Status |
+--------+---------+--------+
| yolov7 | 1 | READY |
+--------+---------+--------+
```
## Performance with Model Analyzer
See [Triton Model Analyzer Documentation](https://github.com/triton-inference-server/server/blob/main/docs/model_analyzer.md#model-analyzer) for more info.
Performance numbers @ RTX 3090 + AMD Ryzen 9 5950X
Example test for 16 concurrent clients using shared memory, each with batch size 1 requests:
```bash
docker run -it --ipc=host --net=host nvcr.io/nvidia/tritonserver:22.06-py3-sdk /bin/bash
./install/bin/perf_analyzer -m yolov7 -u 127.0.0.1:8001 -i grpc --shared-memory system --concurrency-range 16
# Result (truncated)
Concurrency: 16, throughput: 590.119 infer/sec, latency 27080 usec
```
Throughput for 16 clients with batch size 1 is the same as for a single thread running the engine at 16 batch size locally thanks to Triton [Dynamic Batching Strategy](https://github.com/triton-inference-server/server/blob/main/docs/model_configuration.md#dynamic-batcher). Result without dynamic batching (disable in model configuration) considerably worse:
```bash
# Result (truncated)
Concurrency: 16, throughput: 335.587 infer/sec, latency 47616 usec
```
## How to run model in your code
Example client can be found in client.py. It can run dummy input, images and videos.
```bash
pip3 install tritonclient[all] opencv-python
python3 client.py image data/dog.jpg
```

```
$ python3 client.py --help
usage: client.py [-h] [-m MODEL] [--width WIDTH] [--height HEIGHT] [-u URL] [-o OUT] [-f FPS] [-i] [-v] [-t CLIENT_TIMEOUT] [-s] [-r ROOT_CERTIFICATES] [-p PRIVATE_KEY] [-x CERTIFICATE_CHAIN] {dummy,image,video} [input]
positional arguments:
{dummy,image,video} Run mode. 'dummy' will send an emtpy buffer to the server to test if inference works. 'image' will process an image. 'video' will process a video.
input Input file to load from in image or video mode
optional arguments:
-h, --help show this help message and exit
-m MODEL, --model MODEL
Inference model name, default yolov7
--width WIDTH Inference model input width, default 640
--height HEIGHT Inference model input height, default 640
-u URL, --url URL Inference server URL, default localhost:8001
-o OUT, --out OUT Write output into file instead of displaying it
-f FPS, --fps FPS Video output fps, default 24.0 FPS
-i, --model-info Print model status, configuration and statistics
-v, --verbose Enable verbose client output
-t CLIENT_TIMEOUT, --client-timeout CLIENT_TIMEOUT
Client timeout in seconds, default no timeout
-s, --ssl Enable SSL encrypted channel to the server
-r ROOT_CERTIFICATES, --root-certificates ROOT_CERTIFICATES
File holding PEM-encoded root certificates, default none
-p PRIVATE_KEY, --private-key PRIVATE_KEY
File holding PEM-encoded private key, default is none
-x CERTIFICATE_CHAIN, --certificate-chain CERTIFICATE_CHAIN
File holding PEM-encoded certicate chain default is none
```
没有合适的资源?快使用搜索试试~ 我知道了~
温馨提示
基于Jupyter Notebook+yolov7+python实现的铁轨缺陷检测+源码,适合毕业设计、课程设计、项目开发。项目源码已经过严格测试,可以放心参考并在此基础上延申使用~ 基于Jupyter Notebook+yolov7+python实现的铁轨缺陷检测+源码,适合毕业设计、课程设计、项目开发。项目源码已经过严格测试,可以放心参考并在此基础上延申使用~ 基于Jupyter Notebook+yolov7+python实现的铁轨缺陷检测+源码,适合毕业设计、课程设计、项目开发。项目源码已经过严格测试,可以放心参考并在此基础上延申使用~ 基于Jupyter Notebook+yolov7+python实现的铁轨缺陷检测+源码,适合毕业设计、课程设计、项目开发。项目源码已经过严格测试,可以放心参考并在此基础上延申使用~
资源推荐
资源详情
资源评论
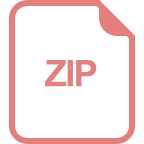
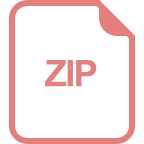
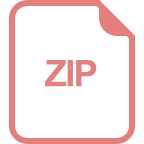
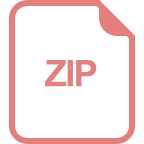
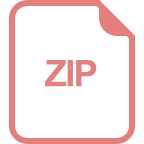
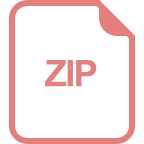
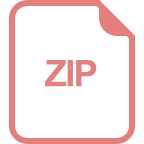
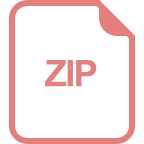
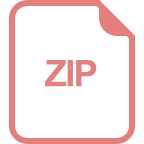
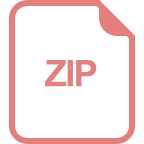
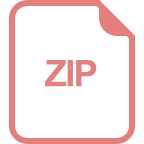
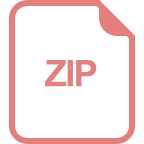
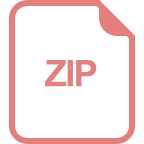
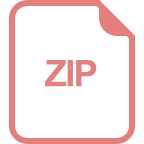
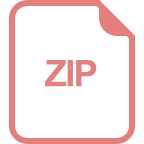
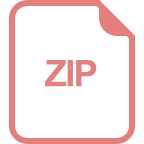
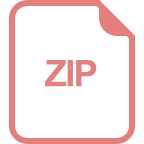
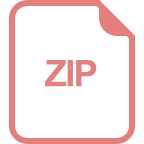
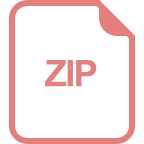
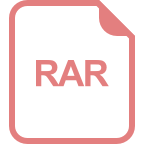
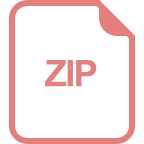
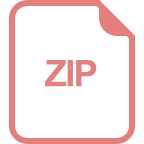
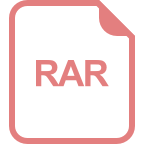
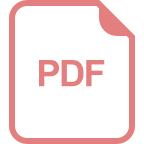
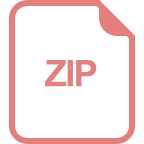
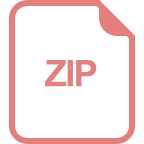
收起资源包目录

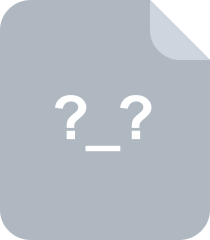
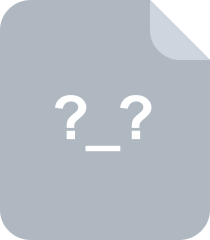
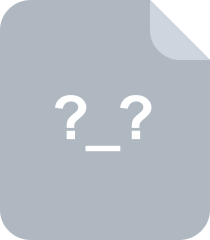
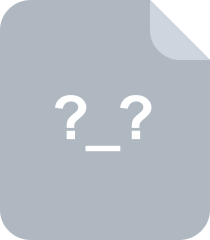
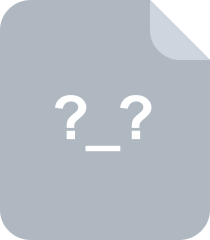
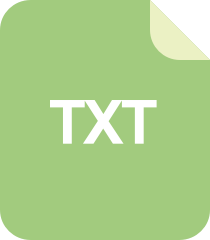
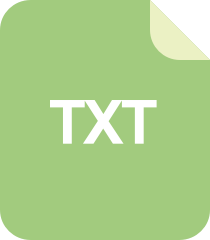
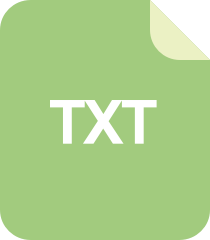
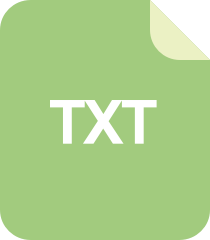
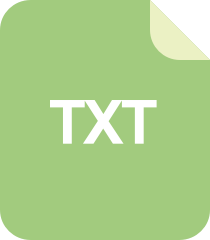
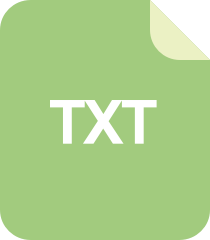
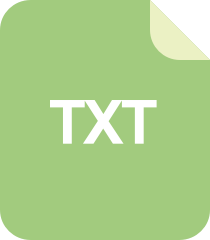
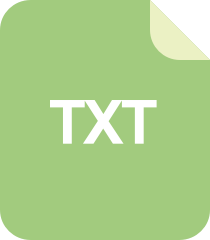
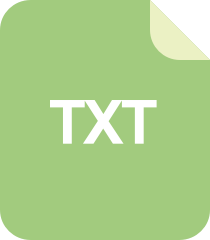
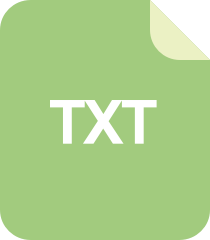
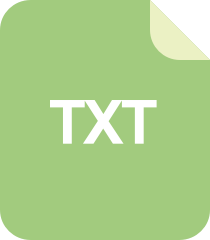
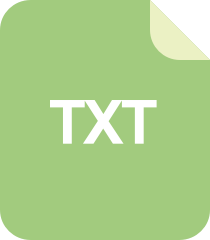
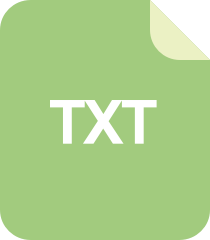
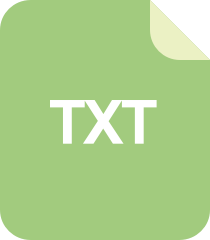
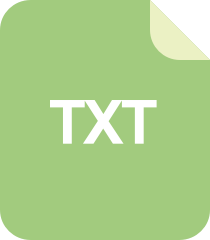
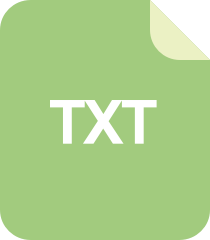
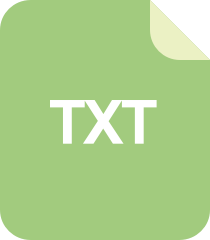
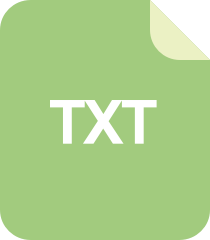
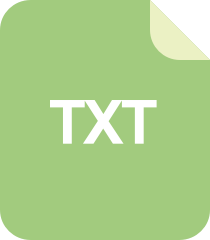
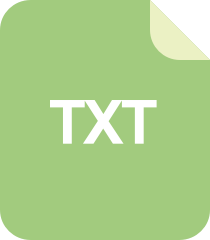
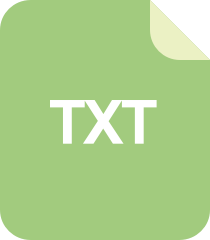
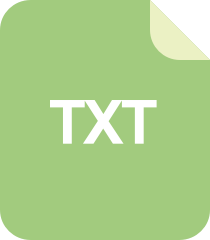
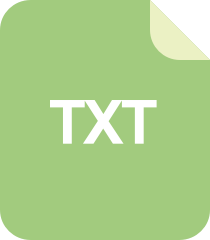
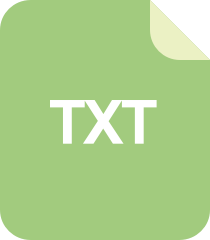
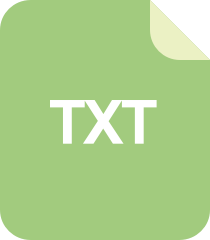
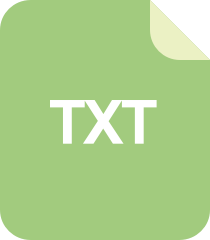
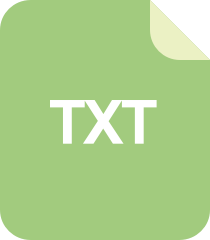
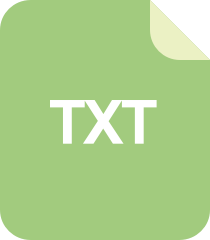
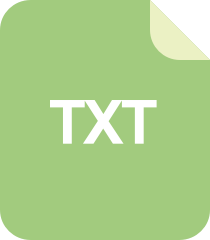
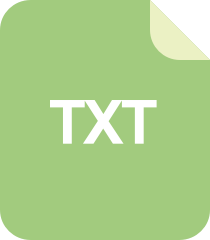
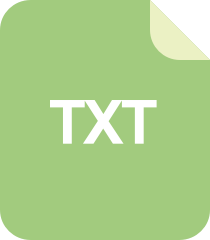
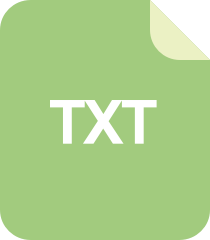
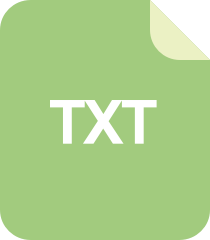
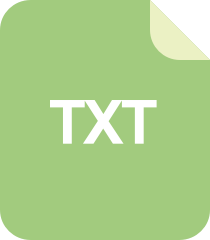
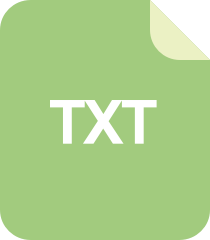
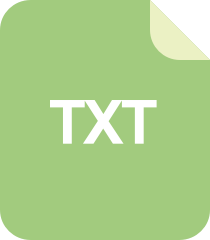
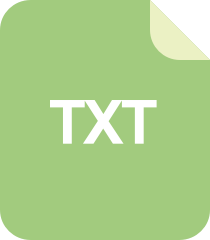
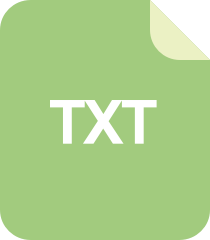
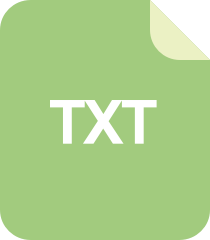
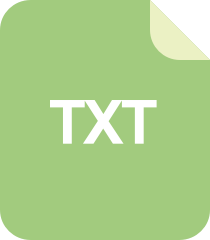
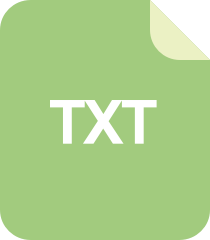
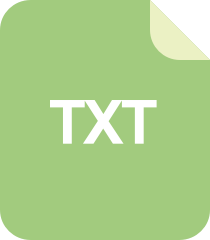
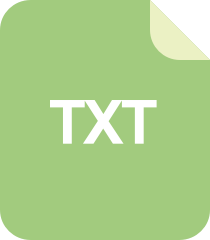
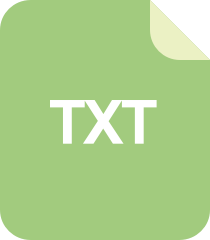
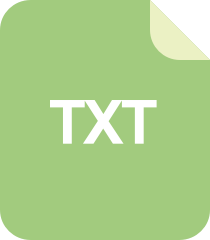
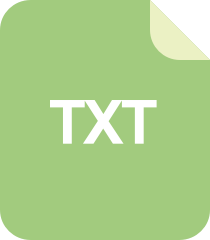
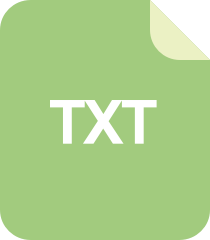
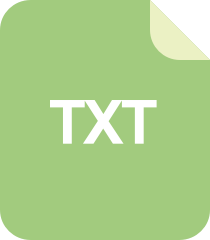
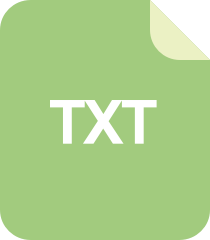
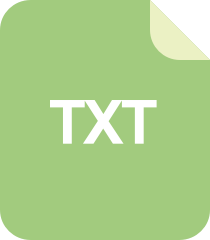
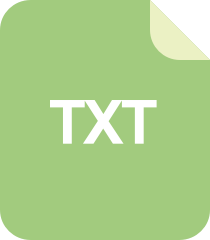
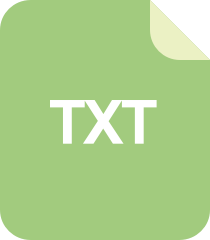
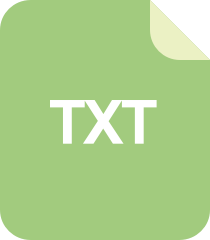
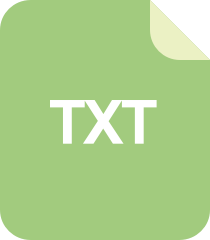
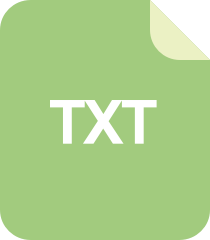
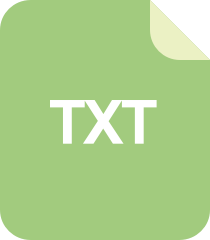
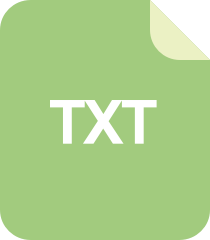
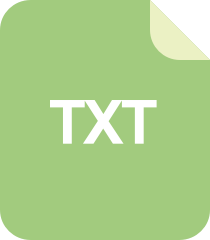
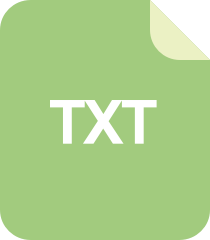
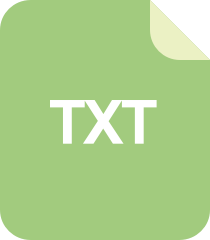
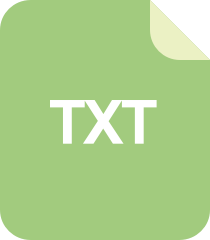
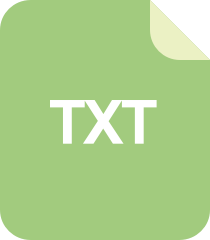
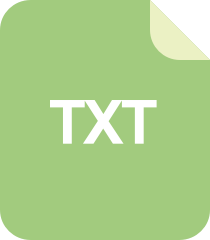
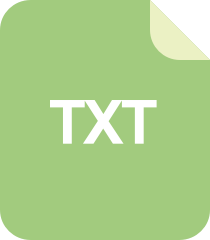
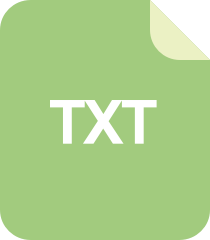
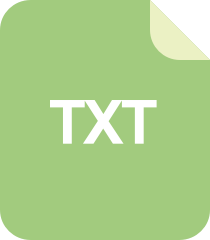
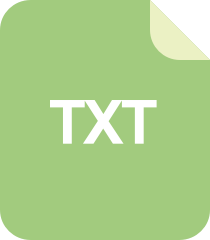
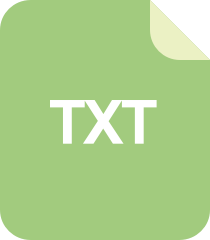
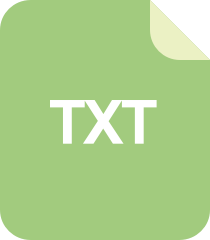
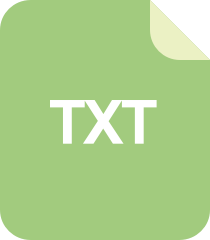
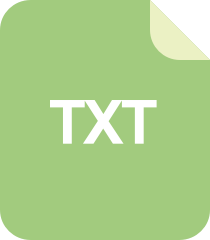
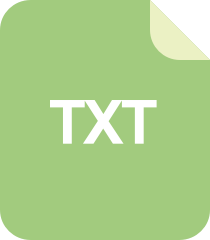
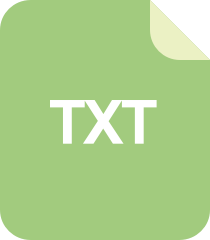
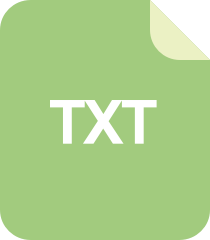
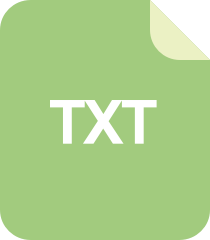
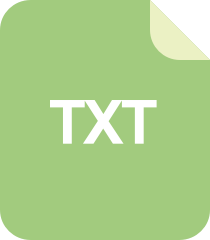
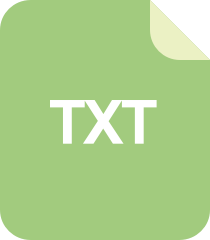
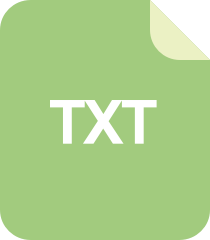
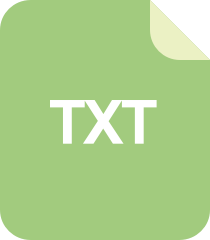
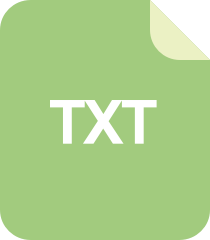
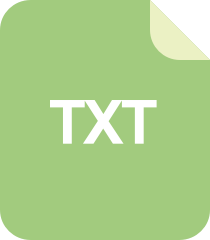
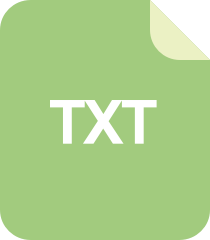
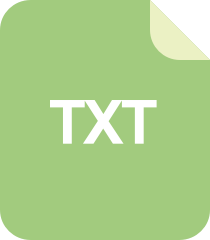
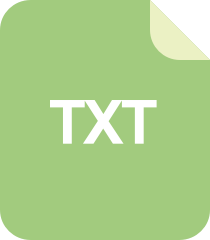
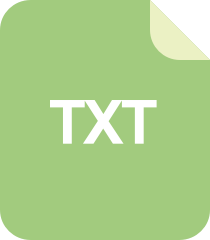
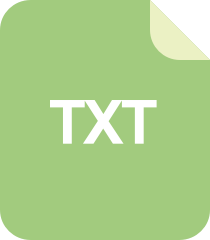
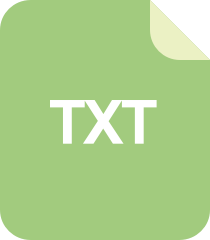
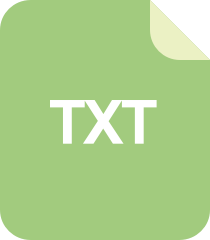
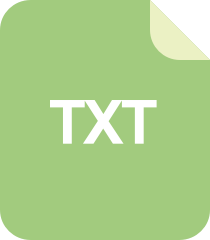
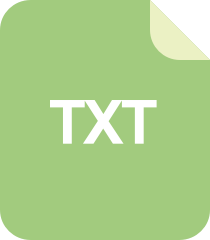
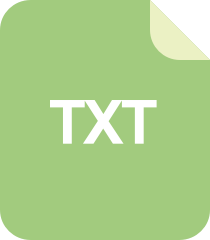
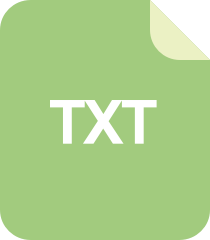
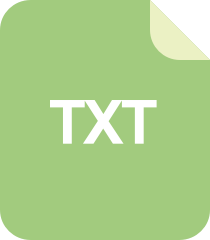
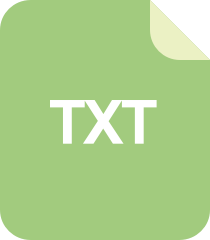
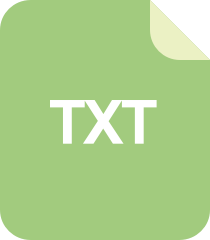
共 2000 条
- 1
- 2
- 3
- 4
- 5
- 6
- 20
资源评论
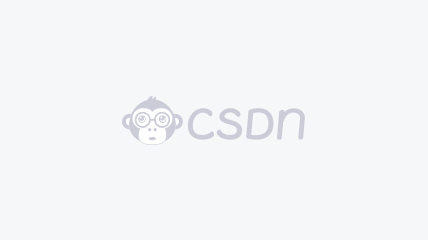
- ndb20042024-06-10非常有用的资源,可以直接使用,对我很有用,果断支持!
- m0_749617862024-06-06非常有用的资源,可以直接使用,对我很有用,果断支持!


梦回阑珊
- 粉丝: 5194
- 资源: 1681

下载权益

C知道特权

VIP文章

课程特权

开通VIP
上传资源 快速赚钱
我的内容管理 展开
我的资源 快来上传第一个资源
我的收益
登录查看自己的收益我的积分 登录查看自己的积分
我的C币 登录后查看C币余额
我的收藏
我的下载
下载帮助

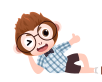
最新资源
资源上传下载、课程学习等过程中有任何疑问或建议,欢迎提出宝贵意见哦~我们会及时处理!
点击此处反馈


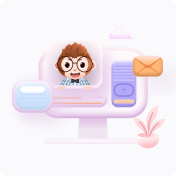
安全验证
文档复制为VIP权益,开通VIP直接复制
