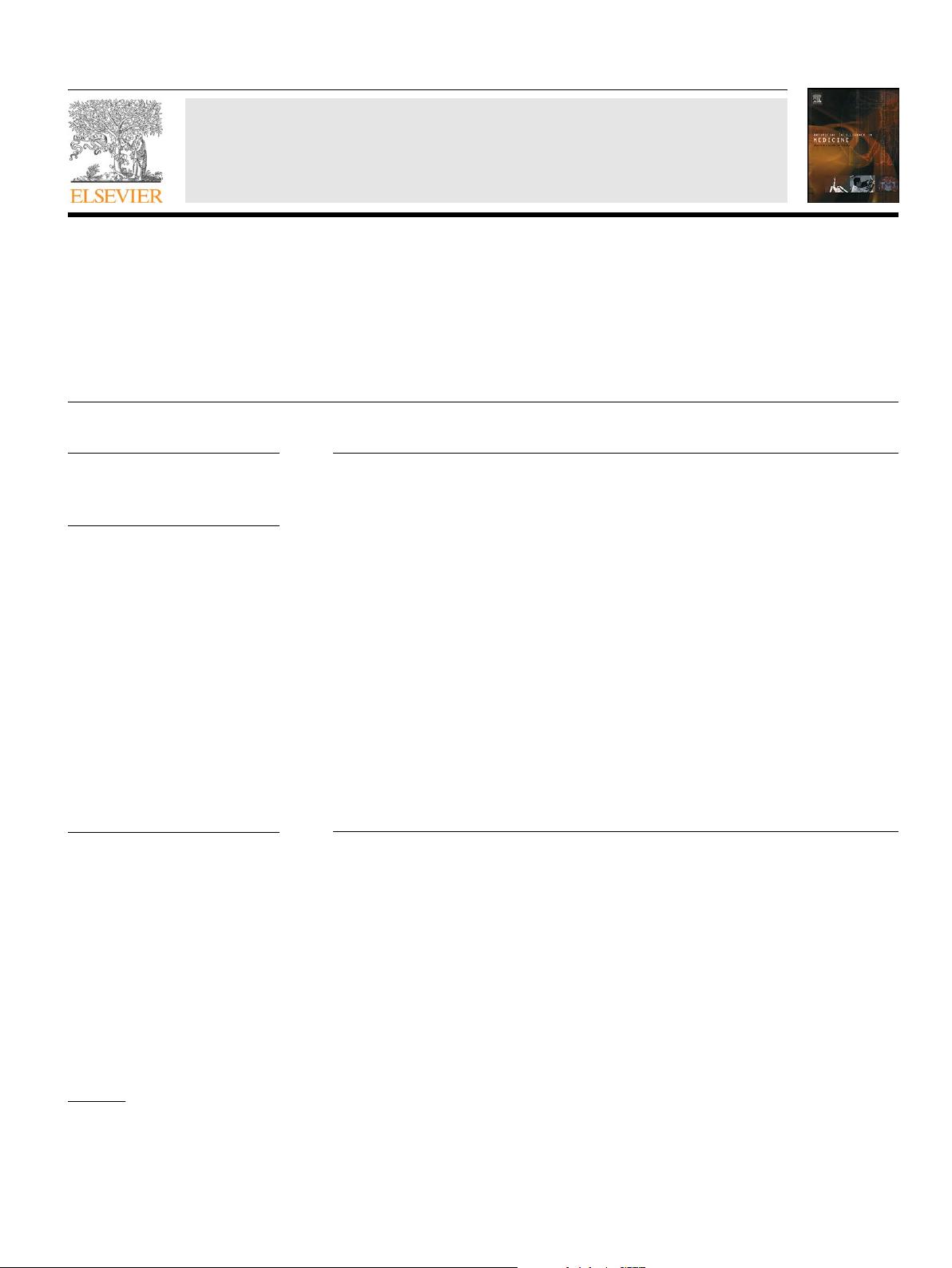
Quantitative prediction of MHC-II binding affinity using particle swarm
optimization
Wen Zhang
a,
*
, Juan Liu
a,
*
, Yanqing Niu
b
a
School of Computer Science, Wuhan University, Wuhan 430072, People’s Republic of China
b
School of Mathematics and Statistics, South-Center University For Nationalities, Wuhan 430074, People’s Republic of China
1. Introduction
The major histocompatibility complex (MHC) is a large genomic
region or gene family found in most vertebrates, which plays an
important role in the immune system. The proteins encoded by the
MHC are known as MHC proteins or MHC molecules. The antigen
peptides first bind with specific MHC molecules, and then are
displayed on the surfaces of one kind of blood cells, called T-cells,
for the recognition via the T-cell receptors (TCR), which
subsequently activates the T-cells cloning, and eventually leads
the cellular immune response to happen. These binding peptides
that can activate the T-cell immune response are well known as the
T-cell epitopes.
MHC molecules are grouped into two main types, classes I and
II. For MHC-I molecules, the binding grooves are closed at both
ends, so they usually bind peptides of a narrow range of lengths
(peptides with nine residues are usually observed). For MHC-II
molecules, the binding grooves are open at both ends, allowing for
various length peptides (9–25 residues). T-cell epitopes are
correspondingly classified into two categories: Tc epitopes
(binding to MHC-I) and Th epitopes (binding to MHC-II).
MHC–peptide binding is the prerequisite of immune response,
thus identifying which peptides binding to which kinds of MHC
molecules is fundamental to understanding the mechanism of T-
cell immunity, and it is helpful for the development of vaccines
against autoimmune diseases and cancers. However, traditional
wet methods for distinguishing MHC binding peptides are time-
consuming, labor-intensive, and expensive. Notice that MHC
Artificial Intelligence in Medicine 50 (2010) 127–132
ARTICLE INFO
Article history:
Received 21 April 2009
Received in revised form 31 March 2010
Accepted 12 May 2010
Keywords:
T-cell immunity
MHC-II quantitative prediction
Position-specific scoring matrix
Particle swarm optimization
ABSTRACT
Objective:
Helper T-cell epitopes (Th epitopes) are the basic units which activate helper T-cell’s immune
response, and they are helpful for understanding the immune mechanism and developing vaccines.
Peptide and major histocompatibility complex class II (MHC-II) binding is an importan t prerequisite
event for helper T-cell immune response, and the binding peptides are usually recognized as Th epitopes,
therefore we can identify Th epitopes by predicting MHC-II binding peptides. Recently, instead of
differentiating the peptides as binder or non-binder, researchers are more interested in predicting
binding affinities between MHC-II molecules and peptides.
Methodology: Motivated by the collective search strategy of the particle swarm optimization algorithm
(PSO), a method was developed to make the direct prediction of peptide binding affinity. In our paper,
PSO was utilized to search for the optimal position-specific scoring matrices (PSSM) from the
experimentally derived allele-related peptides, and then the prediction models were constructed based
on the matrices. Moreover, we evaluated several factors influencing the binding affinity, including
peptide length and flanking residue length, and incorporated them into our models.
Results: The performance of our models was evaluated on three MHC-II alleles from AntiJen database
and 14 MHC-II alleles from IEDB database. When compared to the existing popular quantitative methods
such as MHCPred, SVRMHC, ARB and SMM-align, our method can give out better performance in terms of
correlation coefficient (r) and area under ROC curve (AUC). In addition, the results demonstrated that the
performance of models was fur ther improv ed by incorporating the global length information, achieving
average AUC value of 0.7534 and average r value of 0.4707.
Conclusions: Quantitative prediction of MHC-II binding affinity can be modeled as an optimization
problem. Our PSO based method can find the optimal PSSM, which will then be used for identifying the
binding cores and scoring the binding affinities of the peptides. The experiment results show that our
method is promising for the prediction of MHC-II binding affinity.
ß 2010 Elsevier B.V. All rights reserved.
* Corresponding authors. Tel.: +86 27 6877 5711; fax: +86 27 6877 5711.
E-mail addresses: zw9977129@yahoo.com.cn (W. Zhang), liujuan@whu.edu.cn
(J. Liu), niuyanqing2005@hotmail.com (Y.Q. Niu).
Contents lists available at ScienceDirect
Artificial Intelligence in Medicine
journal homepage: www.elsevier.com/locate/aiim
0933-3657/$ – see front matter ß 2010 Elsevier B.V. All rights reserved.
doi:10.1016/j.artmed.2010.05.003