没有合适的资源?快使用搜索试试~ 我知道了~
资源推荐
资源详情
资源评论
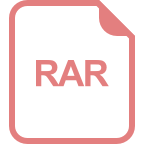
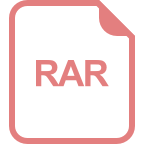
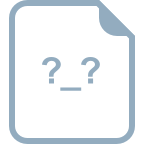
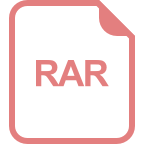
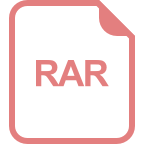
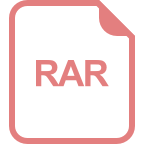
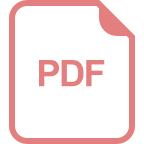
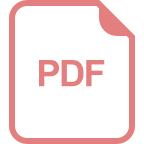
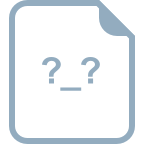
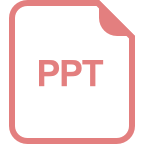
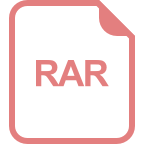
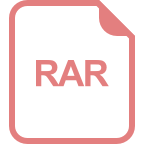
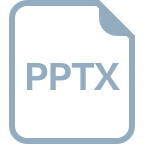
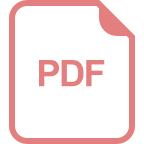
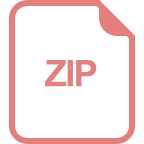
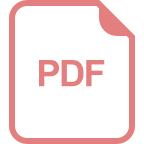
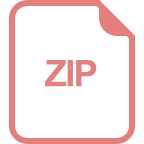
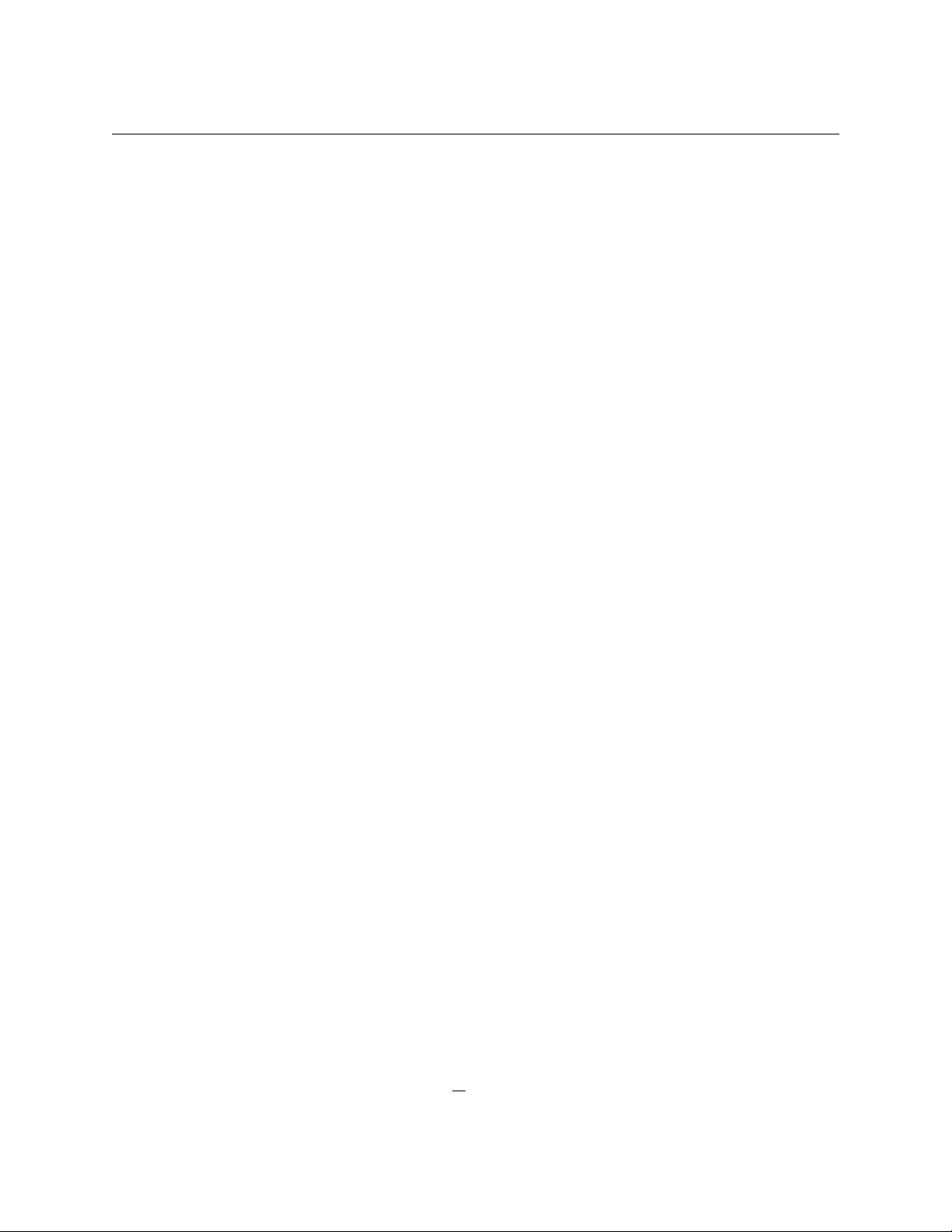
The Jackknife Estimation Method Avery I. McIntosh
1 Introduction
Statistical resampling methods have become feasible for parametric estimation, hypothesis testing,
and model validation now that the computer is a ubiquitous tool for statisticians. This essay focuses
on the resampling technique for parametric estimation known as the Jackknife procedure. To outline
the usefulness of the method and its place in the general class of statistical resampling techniques,
I will quickly delineate two similar resampling methods: the bootstrap and the permutation test.
1.1 Other Sampling Methods: The Bootstrap
The bootstrap is a broad class of usually non-parametric resampling methods for estimating the
sampling distribution of an estimator. The method was described in 1979 by Bradley Efron, and
was inspired by the previous success of the Jackknife procedure.
1
Imagine that a sample of n independent, identically distributed observations from an unknown
distribution have been gathered, and a mean of the sample,
¯
Y , has been calculated. To make
inferences about the population mean we need to know the variability of the sample mean, which
we know from basic statistical theory is V[
¯
Y ] = V[Y ]/n. Here, since the distribution is unknown,
we do not know the value of V[Y ] = σ
2
. The central limit theorem (CLT) states that the stan-
dardized sample mean converges in distribution to a standard normal Z as the sample size grows
large—and we can invoke Slutsky’s theorem to demonstrate that the sample standard deviation is
an adequate estimator for standard deviation σ when the distribution is unknown. However, for
other statistics of interest that do not admit the CLT, and for small sample sizes, the bootstrap is
a viable alternative.
Briefly, the bootstrap method specifies that B samples be generated from the data by sampling
with replacement from the original sample, with each sample set being of identical size as the
original sample (here, n). The larger B is, the closer the set of samples will be to the ideal exact
bootstrap sample, which is of the order of an n-dimensional simplex: |C
n
| = (2n − 1)C(n). The
computation of this number, never mind the actual sample, is generally unfeasible for all but the
smallest sample sizes (for example a sample size of 12 has about 1.3 million with-replacement
subsamples). Furthermore, the bootstrap follows a multinomial distribution, and the most likely
sample is in fact the original sample, hence it is almost certain that there will be random bootstrap
samples that are replicates of the original sample. This means that the computation of the exact
bootstrap is all but impossible in practice. However, Efron and Tibshirani have argued that in
some instances, as few as 25 bootstrap samples can be large enough to form a reliable estimate.
2
The next step in the process is to perform the action that derived the initial statistic—here the
mean: so we sum each bootstrap sample and divide the total by n, and use those quantities to
generate an estimate of the variance of
¯
Y as follows:
SE(
¯
Y )
B
=
n
1
B
B
X
b=1
(
¯
Y
b
−
¯
Y )
2
o
1/2
1
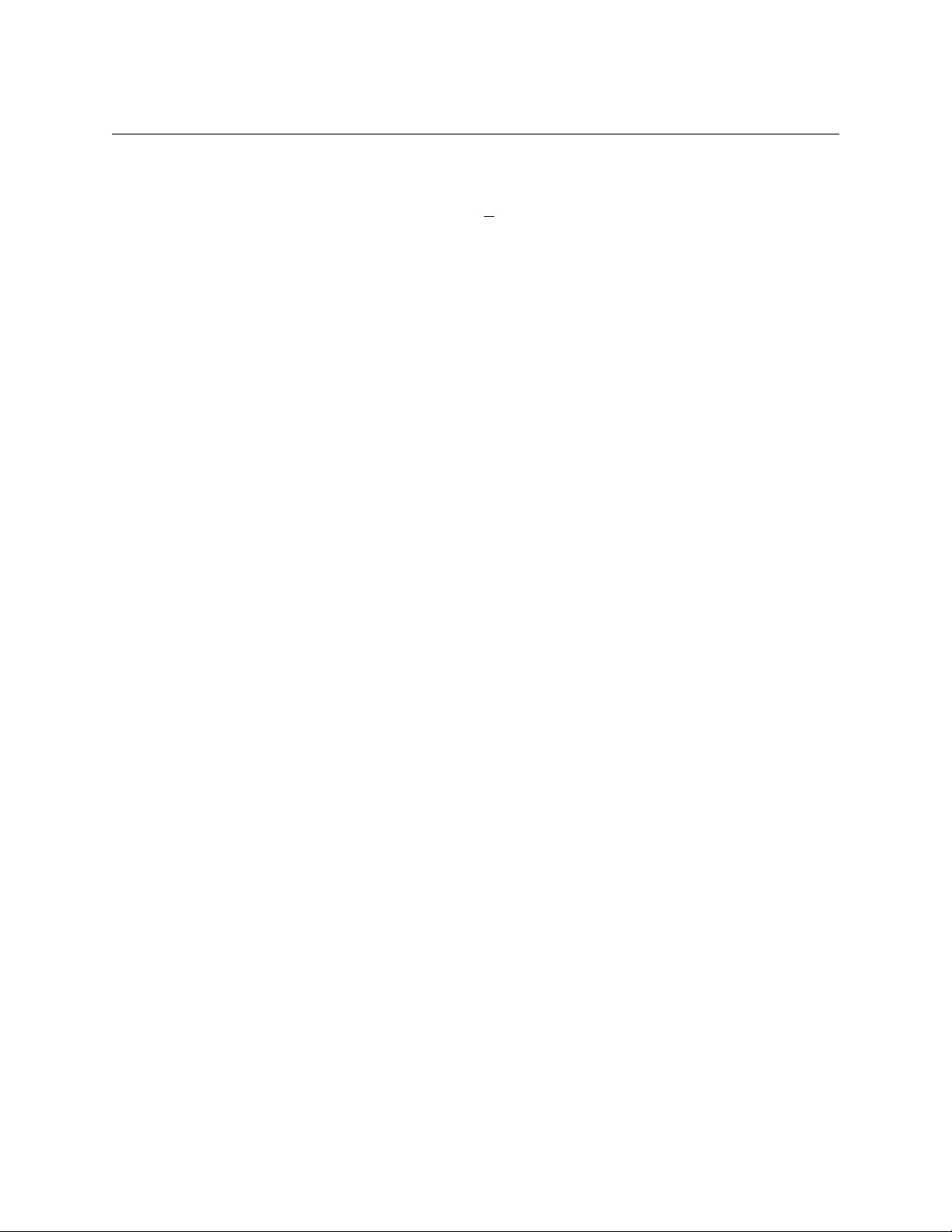
The Jackknife Estimation Method Avery I. McIntosh
The empirical distribution function (EDF) used to generate the bootstrap samples can be shown
to be a consistent, unbiased estimator for the actual cumulative distribution function (CDF) from
which the samples were drawn, F. In fact, the bootstrap performs well because it has a faster rate
of convergence than the CLT: O(1/n) vs. O(1/
√
n), as the bootstrap relies on the strong law of
large numbers (SLLN), a more robust condition than the CLT.
1.2 Other Sampling Methods: Permutation
Permutation testing is done in many arenas, and a classical example is that of permuted y’s in
a pair of random vectors (X, Y) to get a correlation coefficient p-value. For an observed sample
z = {(X
1
, . . . , X
n
), (Y
1
, . . . , Y
n
)}, the elements of (only) the Y vector are permuted B times. Then
for permutation function π(·), we have that an individual permutation sample z
b
is:
z
b
= {(X
1
, . . . , X
n
), (Y
π(1)
, . . . , Y
π(n)
)}
The next step is to compute the number of times that the original correlation statistic is in absolute
value greater than the chosen percentile threshold (say, 0.025 and 0.975 for an empirical α level of
0.05), divided by B. This value is the empirical p-value. If B = n! then the test is called exact; if
all of the permutations are not performed, then there is an inflated Type I error rate, as we are less
likely to sample those values in the tails of the null distribution, and hence we are less likely to say
that there are values greater in absolute value than our original statistic. This method is entirely
non-parametric, and is usually approximated by Monte Carlo methods for large sample sizes where
the exact permutation generation is computationally impractical.
2 The Jackknife: Introduction and Basic Properties
The Jackknife was proposed by M.H. Quenouille in 1949 and later refined and given its current
name by John Tukey in 1956. Quenouille originally developed the method as a procedure for
correcting bias. Later, Tukey described its use in constructing confidence limits for a large class
of estimators. It is similar to the bootstrap in that it involves resampling, but instead of sampling
with replacement, the method samples without replacement.
Many situations arise where it is impractical or even impossible to calculate good estimators or
find those estimators’ standard errors. The situation may be one where there is no theoretical basis
to fall back on, or it may be that in estimating the variance of a difficult function of a statistic,
say g(
¯
X) for some function with no closed-form integral, making use of the usual route of esti-
mation—the delta method theorem—is impossible. In these situations the Jackknife method can
be used to derive an estimate of bias and standard error. Keith Knight has noted, in his book
Mathematical Statistics, that the Jackknife estimate of the standard error is roughly equivalent
2
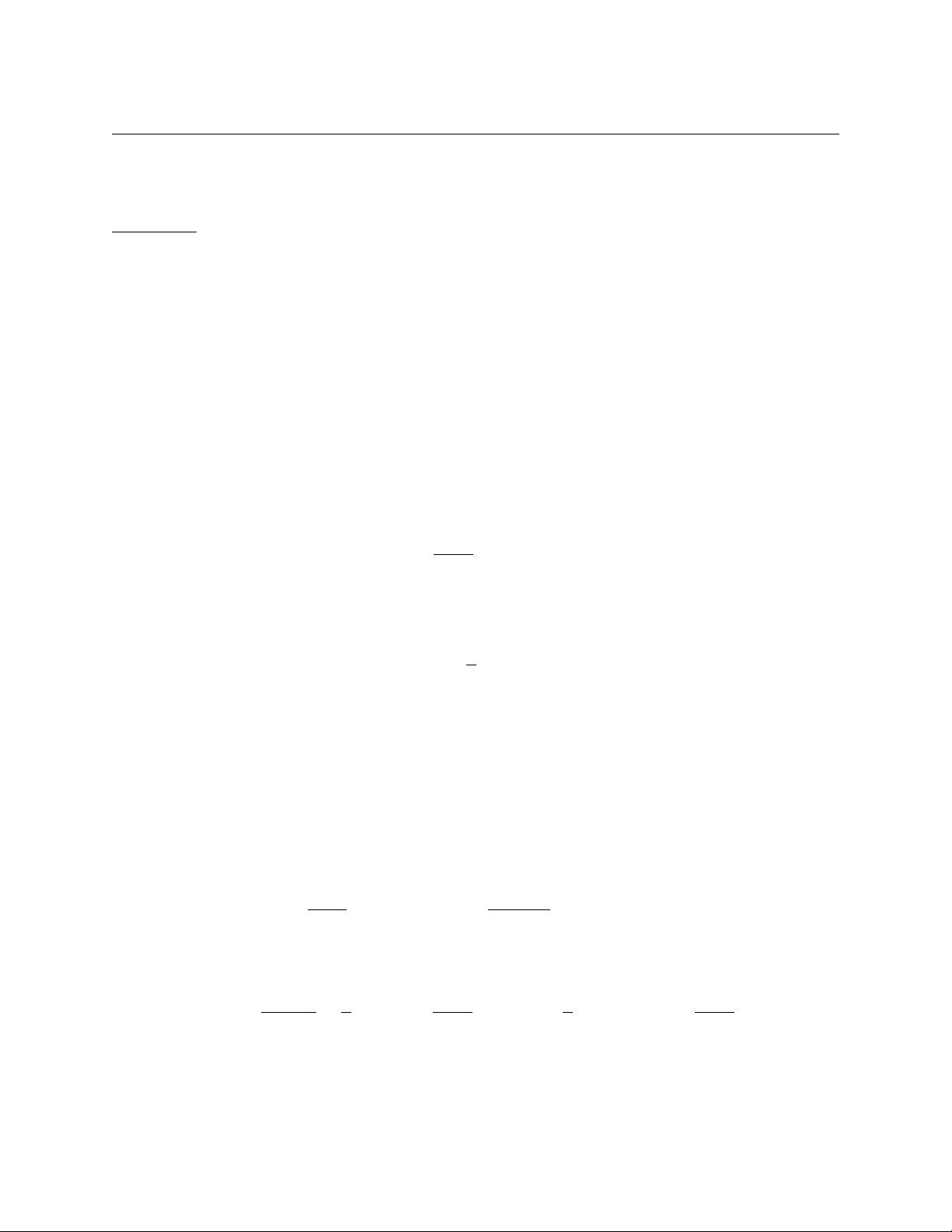
The Jackknife Estimation Method Avery I. McIntosh
to the delta method for large samples.
3
Definition: The delete-1 Jackknife Samples are selected by taking the original data vector and
deleting one observation from the set. Thus, there are n unique Jackknife samples, and the ith
Jackknife sample vector is defined as:
X
[i]
= {X
1
, X
2
, . . . , X
i−1
, X
i+1
, . . . , X
n−1
, X
n
}
This procedure is generalizable to k deletions, which is discussed further below.
The ith Jackknife Replicate is defined as the value of the estimator s(·) evaluated at the ith
Jackknife sample.
ˆ
θ
(i)
:= s(X
[i]
)
The Jackknife Standard Error is defined
SE(
ˆ
θ)
jack
=
n
n − 1
n
n
X
i=1
(
ˆ
θ
(i)
−
ˆ
θ
(·)
)
2
o
1/2
,
where
ˆ
θ
(·)
is the empirical average of the Jackknife replicates:
ˆ
θ
(·)
=
1
n
n
X
i=1
ˆ
θ
(i)
The (n − 1)/n factor in the formula above looks similar to the formula for the standard error of
the sample mean, except that there is a quantity (n −1) included in the numerator. As motivation
for this estimator, I consider the case that does not actually need any resampling methods: that of
the sample mean. Here, the Jackknife estimator above is an unbiased estimator of the variance of
the sample mean.
To demonstrate this claim, I need to show that
n − 1
n
n
X
i=1
(
ˆ
θ
(i)
−
ˆ
θ
(·)
)
2
=
1
n(n − 1)
n
X
i=1
(x
i
− ¯x)
2
I note that here the Jackknife replicates in the inner squared term on the left simplify as follows:
(
ˆ
θ
(i)
−
ˆ
θ
(·)
) =
n¯x − x
i
n − 1
−
1
n
n
X
i=1
¯x
(i)
=
1
n − 1
n¯x − x
i
−
1
n
n
X
i=1
n¯x − x
i
=
1
n − 1
(¯x − x
i
)
Once the term is squared, the equation is complete, and is identically equal to the right hand term
above. Thus, in the case of the sample mean, the Jackknife estimate of the standard error reduces
3
剩余10页未读,继续阅读
资源评论
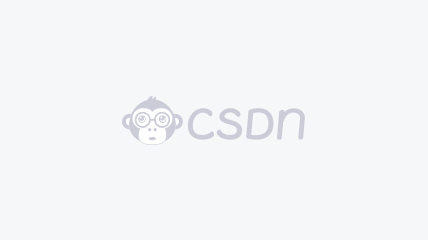

Lee_gaeul
- 粉丝: 27
- 资源: 2
上传资源 快速赚钱
我的内容管理 展开
我的资源 快来上传第一个资源
我的收益
登录查看自己的收益我的积分 登录查看自己的积分
我的C币 登录后查看C币余额
我的收藏
我的下载
下载帮助

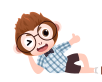
最新资源
- 基于matlab的传统滤波、Butterworth滤波、FIR、移动平均滤波、中值滤波、现代滤波、维纳滤波、自适应滤波、小波变,七种滤波方法,可替自己的数据进行滤波,程序已调通,可直接运行
- 基于Java语言开发的ASR+TTS+声纹识别功能的智能聊天小程序设计源码
- 含风电-光伏-光热电站电力系统N-k安全优化调度模型 关键词:N-K安全约束 光热电站 优化调度 参考文档:参考《光热电站促进风电消纳的电力系统优化调度》光热电站模型; 仿真软件: matlab+y
- 基于TypeScript和JavaScript的每日饮食与运动记录工具设计源码
- 基于JavaScript的仪器预约系统设计源码
- 基于Vue的依沫一站式内容资源变现博客设计源码
- 基于SSM框架与微信小程序的宠物管理系统源码设计
- 基于宝塔Linux面板7.9.0免费版的7.9.2兼容CSS美化设计源码
- 基于ActiveReports的C#报表控件设计源码
- 基于C#与Shell语言的SangServerTool服务器DDNS与SSL证书申请工具设计源码
- 基于SpringBoot+Vue的智能停车场管理系统设计源码
- 基于Shell、Python、PHP、HTML的zzxia-op-super-invincible-lollipop代码构建部署运维工具箱设计源码
- 华为FusionCompute 8.0.1 集成设计指导书
- 基于C语言实现的新型疫苗接种管理系统设计源码
- 基于JavaScript和微信小程序的抖音本地生活团购系统源码搭建与部署方案
- 电力电子boost升压电路MATLAB仿真,pi控制闭环(15r)滑模控制改进版(29r)24升48V,电压可修改 基于反激变器的升压电路,降压电路boost buck的MATLAB仿真,PLECS也
资源上传下载、课程学习等过程中有任何疑问或建议,欢迎提出宝贵意见哦~我们会及时处理!
点击此处反馈


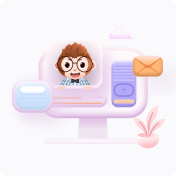
安全验证
文档复制为VIP权益,开通VIP直接复制
