%% 清空环境变量
warning off % 关闭报警信息
close all % 关闭开启的图窗
clear % 清空变量
clc % 清空命令行
%% 导入数据
res = xlsread('数据集.xlsx');
%% 划分训练集和测试集
temp = randperm(103);
P_train = res(temp(1: 80), 1: 7)';
T_train = res(temp(1: 80), 8)';
M = size(P_train, 2);
P_test = res(temp(81: end), 1: 7)';
T_test = res(temp(81: end), 8)';
N = size(P_test, 2);
%% 数据归一化
[p_train, ps_input] = mapminmax(P_train, 0, 1);
p_test = mapminmax('apply', P_test, ps_input);
[t_train, ps_output] = mapminmax(T_train, 0, 1);
t_test = mapminmax('apply', T_test, ps_output);
%% 转置以适应模型
p_train = p_train'; p_test = p_test';
t_train = t_train'; t_test = t_test';
%% 训练模型
trees = 100; % 决策树数目
leaf = 5; % 最小叶子数
OOBPrediction = 'on'; % 打开误差图
OOBPredictorImportance = 'on'; % 计算特征重要性
Method = 'regression'; % 分类还是回归
net = TreeBagger(trees, p_train, t_train, 'OOBPredictorImportance', OOBPredictorImportance,...
'Method', Method, 'OOBPrediction', OOBPrediction, 'minleaf', leaf);
importance = net.OOBPermutedPredictorDeltaError; % 重要性
%% 仿真测试
t_sim1 = predict(net, p_train);
t_sim2 = predict(net, p_test );
%% 数据反归一化
T_sim1 = mapminmax('reverse', t_sim1, ps_output);
T_sim2 = mapminmax('reverse', t_sim2, ps_output);
%% 均方根误差
error1 = sqrt(sum((T_sim1' - T_train).^2) ./ M);
error2 = sqrt(sum((T_sim2' - T_test ).^2) ./ N);
%% 绘图
figure
plot(1: M, T_train, 'r-*', 1: M, T_sim1, 'b-o', 'LineWidth', 1)
legend('真实值', '预测值')
xlabel('预测样本')
ylabel('预测结果')
string = {'训练集预测结果对比'; ['RMSE=' num2str(error1)]};
title(string)
xlim([1, M])
grid
figure
plot(1: N, T_test, 'r-*', 1: N, T_sim2, 'b-o', 'LineWidth', 1)
legend('真实值', '预测值')
xlabel('预测样本')
ylabel('预测结果')
string = {'测试集预测结果对比'; ['RMSE=' num2str(error2)]};
title(string)
xlim([1, N])
grid
%% 绘制误差曲线
figure
plot(1: trees, oobError(net), 'b-', 'LineWidth', 1)
legend('误差曲线')
xlabel('决策树数目')
ylabel('误差')
xlim([1, trees])
grid
%% 绘制特征重要性
figure
bar(importance)
legend('重要性')
xlabel('特征')
ylabel('重要性')
%% 相关指标计算
% R2
R1 = 1 - norm(T_train - T_sim1')^2 / norm(T_train - mean(T_train))^2;
R2 = 1 - norm(T_test - T_sim2')^2 / norm(T_test - mean(T_test ))^2;
disp(['训练集数据的R2为:', num2str(R1)])
disp(['测试集数据的R2为:', num2str(R2)])
% MAE
mae1 = sum(abs(T_sim1' - T_train)) ./ M;
mae2 = sum(abs(T_sim2' - T_test )) ./ N;
disp(['训练集数据的MAE为:', num2str(mae1)])
disp(['测试集数据的MAE为:', num2str(mae2)])
% MBE
mbe1 = sum(T_sim1' - T_train) ./ M ;
mbe2 = sum(T_sim2' - T_test ) ./ N ;
disp(['训练集数据的MBE为:', num2str(mbe1)])
disp(['测试集数据的MBE为:', num2str(mbe2)])
%% 绘制散点图
sz = 25;
c = 'b';
figure
scatter(T_train, T_sim1, sz, c)
hold on
plot(xlim, ylim, '--k')
xlabel('训练集真实值');
ylabel('训练集预测值');
xlim([min(T_train) max(T_train)])
ylim([min(T_sim1) max(T_sim1)])
title('训练集预测值 vs. 训练集真实值')
figure
scatter(T_test, T_sim2, sz, c)
hold on
plot(xlim, ylim, '--k')
xlabel('测试集真实值');
ylabel('测试集预测值');
xlim([min(T_test) max(T_test)])
ylim([min(T_sim2) max(T_sim2)])
title('测试集预测值 vs. 测试集真实值')

GZM888888
- 粉丝: 515
- 资源: 3067
最新资源
- 适用于 Python 的 LINE 消息 API SDK.zip
- 适用于 Python 的 AWS 开发工具包.zip
- 适用于 Python 3 的 Django LDAP 用户身份验证后端 .zip
- 基于PBL-CDIO的材料成型及控制工程课程设计实践与改革
- JQuerymobilea4中文手册CHM版最新版本
- 适用于 Python 2 和 3 以及 PyPy (ws4py 0.5.1) 的 WebSocket 客户端和服务器库.zip
- 适用于 AWS 的 Python 无服务器微框架.zip
- 适用于 Apache Cassandra 的 DataStax Python 驱动程序.zip
- WebAPI-案例-年会抽奖.html
- 这里有一些基础问题和一些棘手问题的解答 还有hackerrank,hackerearth,codechef问题的解答 .zip
资源上传下载、课程学习等过程中有任何疑问或建议,欢迎提出宝贵意见哦~我们会及时处理!
点击此处反馈


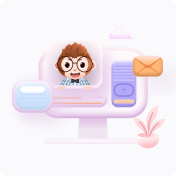
- 1
- 2
- 3
- 4
前往页