function [net,tr,out3,out4,out5,out6]=train(net,varargin)
%TRAIN Train a neural network.
%
% [NET,TR] = <a href="matlab:doc train">train</a>(NET,X,T) takes a network NET, input data X
% and target data T and returns the network after training it, and a
% a training record TR.
%
% [NET,TR] = <a href="matlab:doc train">train</a>(NET,X) takes only input data, in cases where
% the network's training function is unsupervised (i.e. does not require
% target data).
%
% [NET,TR] = <a href="matlab:doc train">train</a>(NET,X,T,Xi,Ai,EW) takes additional optional
% arguments suitable for training dynamic networks and training with
% error weights. Xi and Ai are the initial input and layer delays states
% respectively and EW defines error weights used to indicate
% the relative importance of each target value.
%
% <a href="matlab:doc train">train</a> calls the network training function NET.<a href="matlab:doc nnproperty.net_trainFcn">trainFcn</a> with the
% parameters NET.<a href="matlab:doc nnproperty.net_trainParam">trainParam</a> to perform training. Training functions
% may also be called directly.
%
% <a href="matlab:doc train">train</a> arguments can have two formats: matrices, for static
% problems and networks with single inputs and outputs, and cell arrays
% for multiple timesteps and networks with multiple inputs and outputs.
%
% The matrix format is as follows:
% X - RxQ matrix
% Y - UxQ matrix.
% Where:
% Q = number of samples
% R = number of elements in the network's input
% U = number of elements in the network's output
%
% The cell array format is most general:
% X - NixTS cell array, each element X{i,ts} is an RixQ matrix.
% Xi - NixID cell array, each element Xi{i,k} is an RixQ matrix.
% Ai - NlxLD cell array, each element Ai{i,k} is an SixQ matrix.
% Y - NOxTS cell array, each element Y{i,ts} is a UixQ matrix.
% Xf - NixID cell array, each element Xf{i,k} is an RixQ matrix.
% Af - NlxLD cell array, each element Af{i,k} is an SixQ matrix.
% Where:
% TS = number of time steps
% Ni = NET.<a href="matlab:doc nnproperty.net_numInputs">numInputs</a>
% Nl = NET.<a href="matlab:doc nnproperty.net_numLayers">numLayers</a>,
% No = NET.<a href="matlab:doc nnproperty.net_numOutputs">numOutputs</a>
% ID = NET.<a href="matlab:doc nnproperty.net_numInputDelays">numInputDelays</a>
% LD = NET.<a href="matlab:doc nnproperty.net_numLayerDelays">numLayerDelays</a>
% Ri = NET.<a href="matlab:doc nnproperty.net_inputs">inputs</a>{i}.<a href="matlab:doc nnproperty.input_size">size</a>
% Si = NET.<a href="matlab:doc nnproperty.net_layers">layers</a>{i}.<a href="matlab:doc nnproperty.layer_size">size</a>
% Ui = NET.<a href="matlab:doc nnproperty.net_outputs">outputs</a>{i}.<a href="matlab:doc nnproperty.output_size">size</a>
%
% The error weights EW can be 1, indicating all targets are equally
% important. It can also be either a 1xQ vector defining relative sample
% importances, a 1xTS cell array of scalar values defining relative
% timestep importances, an Nox1 cell array of scalar values defining
% relative network output importances, or in general an NoxTS cell array
% of NixQ matrices (the same size as T) defining every target element's
% relative importance.
%
% The training record TR is a structure whose fields depend on the network
% training function (net.NET.<a href="matlab:doc nnproperty.net_trainFcn">trainFcn</a>). It may include fields such as:
% * Training, data division, and performance functions and parameters
% * Data division indices for training, validation and test sets
% * Data division masks for training validation and test sets
% * Number of epochs (num_epochs) and the best epoch (best_epoch).
% * A list of training state names (states).
% * Fields for each state name recording its value throughout training
% * Performances of the best network (best_perf, best_vperf, best_tperf)
%
% Here a static feedforward network is created, trained on some data, then
% simulated using SIM and network notation.
%
% [x,t] = <a href="matlab:doc simplefit_dataset">simplefit_dataset</a>;
% net = <a href="matlab:doc feedforwardnet">feedforwardnet</a>(10);
% net = <a href="matlab:doc train">train</a>(net,x,t);
% y1 = <a href="matlab:doc sim">sim</a>(net,x)
% y2 = net(x)
%
% Here a dynamic NARX network is created, trained, and simulated on
% time series data.
%
% [X,T] = <a href="matlab:doc simplenarx_dataset">simplenarx_dataset</a>;
% net = <a href="matlab:doc narxnet">narxnet</a>(1:2,1:2,10);
% <a href="matlab:doc view">view</a>(net)
% [Xs,Xi,Ai,Ts] = <a href="matlab:doc preparets">preparets</a>(net,X,{},T);
% net = <a href="matlab:doc train">train</a>(net,Xs,Ts,Xi,Ai);
% Y = net(Xs,Xi,Ai)
%
% <strong>Training with Parallel Computing</strong>
%
% Parallel Computing Toolbox allows Neural Network Toolbox to train
% networks faster and on larger datasets than can fit on one PC.
%
% (Parallel and GPU training are currently supported for backpropagation
% training only, i.e. not Self-Organizing Maps.
%
% Here training automatically happens across MATLAB parallel workers.
%
% parpool
% [X,T] = vinyl_dataset;
% net = feedforwardnet(140,'trainscg');
% net = <a href="matlab:doc train">train</a>(net,X,T,'UseParallel','yes');
% Y = net(X,'UseParallel','yes');
%
% Use Composite values to distribute the data manually, and get back
% the results as a Composite value. If the data is loaded as it is
% distributed then while each piece of the dataset must fit in RAM, the
% entire dataset is only limited by the number of workers RAM. Use
% the function <a href="matlab:doc configure">configure</a> to prepare a network for training
% with parallel data.
%
% net = feedforwardnet(140,'trainscg');
% net = configure(net,X,T);
% Xc = Composite;
% Tc = Composite;
% for i=1:numel(Xc)
% Xc{i} = X+rand(size(X))*0.1; % (Use real data instead
% Tc{i} = T+rand(size(T))*0.1; % instead of random data)
% end
% net = <a href="matlab:doc train">train</a>(net,Xc,Tc);
% Yc = net(Xc);
% Y = cat(2,Yc{:});
%
% Networks can be trained using the current GPU device, if it is
% supported by the Parallel Computing Toolbox. This is efficient for
% large static problems or dynamic problems with many series.
%
% net = feedforwardnet(140,'trainscg');
% net = <a href="matlab:doc train">train</a>(net,X,T,'UseGPU','yes');
% Y = net(X,'UseGPU','yes');
%
% If a network is static (no delays) and has a single input and output,
% then training can be done with data already converted to gpuArray form,
% if the network is configured with MATLAB data first.
%
% net = feedforwardnet(140,'trainscg');
% net = configure(net,X,T);
% Xgpu = gpuArray(X);
% Tgpu = gpuArray(T);
% net = <a href="matlab:doc train">train</a>(net,Xgpu,Tgpu);
% Ygpu = net(Xgpu);
% Y = gather(Ygpu);
%
% To run in parallel, with workers associated with unique GPUs taking
% advantage of that hardware, while the rest of the workers use CPUs:
%
% net = feedforwardnet(140,'trainscg');
% net = <a href="matlab:doc train">train</a>(net,X,T,'UseParallel','yes','UseGPU','yes');
% Y = net(X,'UseParallel','yes','UseGPU','yes');
%
% Only using workers with unique GPUs may result in higher speed, as CPU
% workers may not keep up.
%
% net = feedforwardnet(140,'trainscg');
% net = <a href="matlab:doc train">train</a>(net,X,T,'UseParallel','yes','UseGPU','only');
% Y = net(X,'UseParallel','yes','UseGPU','only');
%
% Use the 'ShowResources' option to verify the computing resources used.
%
% net = train(...,'ShowResources','yes');
%
% <strong>Training Safely with Checkpoint Files</strong>
%
% The optional parameter CheckpointFile allows

GZM888888
- 粉丝: 577
- 资源: 3066
最新资源
- 基于Springboot+Vue疫情打卡健康评测系统-毕业源码案例设计(源码+项目说明+演示视频).zip
- 基于Springboot+Vue校园失物招领系统-毕业源码案例设计(高分毕业设计).zip
- 北京市各项指标.xlsx
- 基于Springboot+Vue新冠病毒密接者跟踪系统-毕业源码案例设计(高分项目).zip
- 机械设计电池焊脚检测设备sw18可编辑非常好的设计图纸100%好用.zip
- 基于Springboot+Vue校园疫情防控系统-毕业源码案例设计(源码+论文).zip
- 基于Springboot+Vue新闻稿件管理系统-毕业源码案例设计(高分毕业设计).zip
- 基于Springboot+Vue新闻资讯系统-毕业源码案例设计(95分以上).zip
- 基于Springboot+Vue学科竞赛报名管理系统毕业源码案例设计(源码+数据库).zip
- 基于Springboot+Vue学生评奖评优管理系统-毕业源码案例设计(高分毕业设计).zip
- Comsol冻土路基水热力源文件 该文件建立了路基水热耦合计算控制方程, 利用COMSOL 软件二次开发实现了路基冻胀融沉问题的水热耦合计算 本案例建立成二维模型,物理场采用两个PDE模块和固体力学
- 基于Springboot+Vue学生宿舍管理系统毕业源码案例设计(源码+论文).zip
- 基于Springboot+Vue学生网上请假系统设计与实现-毕业源码案例设计(源码+项目说明+演示视频).zip
- 基于Springboot+Vue学生宿舍信息系统-毕业源码案例设计(高分毕业设计).zip
- 基于Springboot+Vue学生选课系统-毕业源码案例设计(95分以上).zip
- GPT-SoVITS-WebUI
资源上传下载、课程学习等过程中有任何疑问或建议,欢迎提出宝贵意见哦~我们会及时处理!
点击此处反馈


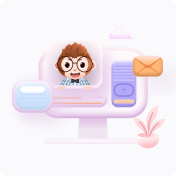