import numpy as np
from tensorflow.keras.models import Sequential
from tensorflow.keras.layers import LSTM
from tensorflow.keras.layers import Dense
from tensorflow.keras.layers import Bidirectional
import pandas as pd
import matplotlib.pyplot as plt
import time
from tqdm import tqdm
#The program is designed to restore missing value, which is -1, in CSV file thatderives from time series remote sensing images. At first, the CSV file has many data type. Thus, we need to clean up the data. Then using KNN classifier to find out k nearest data arrays for target array. Finally, the Bi-LSTM module restorethe data.
#CSV reader
def csv_reader(file,chunkSize=1000,patitions=10**2):
reader=pd.read_csv(file,iterator=True,header=None)
chunks=[]
with tqdm(range(patitions),'Reading...') as t:
for _ in t:
try:
chunk=reader.get_chunk(chunkSize)
chunks.append(chunk)
except StopIteration:
break
return pd.concat(chunks,ignore_index=True)
#Cleaning data to convert them into float.
def data_clean(seq):
index=[]
for i in range(0,len(seq)):
if(isinstance(seq[i],str)):
if not('#IO' in seq[i]):
ex=seq[i]
seq=np.delete(seq,i,axis=0)
seq=np.insert(seq,i,float(ex))
else:
seq=np.delete(seq,i,axis=0)
seq=np.insert(seq,i,-999)
index.append(i)
return seq,index
#The header of CSV is the date, however, we transform the date into a list of number which is easier to be handled.
def date_calculate(seq):
date=[5]
count=5
for i in range(0,len(seq)-1):
day=float(seq[i+1])-float(seq[i])
count=count+day
date.append(count)
return date
#Before further operation, data arrays with missing value require preliminary restoration.
def linearInsert(X,Y):
# indexes=[]
for i in range(0,len(Y)):
if(Y[i]==-999):
# indexes.append(i)
Y=np.delete(Y,i,axis=0)
coordination=X[i]
X=np.delete(X,i,axis=0)
newElement=np.interp(coordination,X,Y)
Y=np.insert(Y,i,newElement)
X=np.insert(X,i,coordination)
return Y
def split_sequence(sequence, n_steps):
X, y = list(), list()
for i in range(len(sequence)):
# find the end of this pattern
end_ix = i + n_steps
# check if we are beyond the sequence
if end_ix > len(sequence)-1:
break
# gather input and output parts of the pattern
seq_x, seq_y = sequence[i:end_ix], sequence[end_ix]
X.append(seq_x)
y.append(seq_y)
return np.array(X), np.array(y)
#Knn designed to search k nearest arrays for input
def classify(input,dataSet,k):
dataSize=dataSet.shape[0] #给出训练数据的个数group.shape=(4,2)
#print('1.Calculate dataSize:',dataSize)
##计算欧式距离
diff=np.tile(input,(dataSize,1))-dataSet
#print('2.Calculate Distance,diff:',diff)
squarediff=diff**2
squareDist=np.sum(squarediff,axis=1)
#print('3.Sum of square of diff:',squareDist,'Distance done')
dist=squareDist**0.5
#print('dist:',dist)
#对距离进行排序
sortedDistIndex=np.argsort(dist) #argsort()根据元素的值从小到大进行排序,返回索引
#print('4.Sort distance,sorteedDistIndex:',sortedDistIndex)
resembleArray=input
for i in range(k):
if(sortedDistIndex[i]!=0):
#resembleArray=np.insert(resembleArray,i,dataSet[sortedDistIndex[i]])
resembleArray=np.vstack((resembleArray,dataSet[sortedDistIndex[i]]))
print('The ',i,'th data is ',dataSet[sortedDistIndex[i]])
print('Distance is ',dist[sortedDistIndex[i]])
#axis=1 is delete column, while axis=0 is delete row
resembleArray=np.delete(resembleArray,0,axis=0)
return resembleArray
#a=[30,31,32,33,34,35,36,37,38]
#raw_seq=pd.read_csv('data/test.csv').iloc[1,:].values
#raw_dataset=pd.read_csv('data/test.csv').iloc[1:,:].values
#raw_seq=np.delete(raw_seq,a,axis=0)
#raw_seq=raw_seq.tolist()
#raw_dataset=np.delete(raw_dataset,a,axis=1)
#reader=raw_dataset
#date=pd.read_csv('data/test.csv').iloc[0,:].values
reader=np.array(csv_reader('data/2018NDVI.csv'))
reader=np.delete(reader,[0],axis=1)
reader=np.delete(reader,[51],axis=1)
date=reader[0].tolist()
date=date_calculate(date)
raw_seq_dirty=reader[1]
#raw_seq=data_clean(raw_seq).tolist()
raw_seq,seq_index=data_clean(raw_seq_dirty)
raw_seq=raw_seq.tolist()
raw_dataset_dirty=np.delete(reader,[0,1],axis=0)
#raw_dataset=data_clean(raw_dataset).tolist()
print('It is under cleaning process.......................')
raw_dataset=[]
indexes=[seq_index]
for data in raw_dataset_dirty:
data,data_index=data_clean(data)
data=data.tolist()
raw_dataset.append(data)
indexes.append(data_index)
print('Cleaning process is done!')
#Now parameters: raw_dataset, indexes
K=5
n_steps = 3
n_features = 1
#Process below can be written as a loop. I didn't because my PC sucks.
#for i in range(0,len(dataset)):
i=1
input=raw_dataset[i]
input_index=indexes[i]
unsatisfiedArray=[]
for j in range(0,len(indexes)):
for b in indexes[j]:
if(b in input_index):
unsatisfiedArray.append(j)
break
#training_samples=np.delete(training_samples,unsatisfiedArray,axis=0)
raw_dataset=np.delete(raw_dataset,unsatisfiedArray,axis=0)
#Now parameters:raw_dataset, input,input_index
#Linear interpolate input sequence
#The reason why read the first array and the rest seperately is to reform a intact dataset in linearInterpolation step.
#After this step, we get a interpolated dataset that fits CSV file
print('Then it is linear interpolation process..............')
#raw_seq is the first array in the dataset, acting as a corrdination.
dataset=[linearInsert(date,raw_seq)]
input=linearInsert(date,input)
#indexset=[index]
for i in raw_dataset:
#data,index=linearInsert(date,i)
data=linearInsert(date,i)
#Mention that dataset is a numpy array, when indexset is not, because indexset cannot be a matrix or there will be an error
dataset=np.vstack((dataset,data))
#indexset.append(index)
dataset=np.delete(dataset,0,axis=0)
print('All data have been interpolated!')
#Reconstruction of each data array in CSV
# missingValue_indexes=indexset[i]
#samplesNoInput=np.delete(dataset,i,axis=0)
training_samples=dataset
#indexesNoInput=np.delete(indexset,i,axis=0)
training_indexes=indexes
#print('Unprocess indexset: ',indexset)
#Remove arrays that have missing values in the same timesteps with input
#But we cannot remove them in loop, instead we should record it.
print('The input data that will be reconstructed is: ',input)
print('KNN classifier is searching k nearest data for input')
#for j in range(0,len(indexset)):
# for b in indexset[j]:
# if(b in indexset[i]):
# unsatisfiedArray.append(j)
# break
#training_samples=np.delete(training_samples,unsatisfiedArray,axis=0)
print('The k training sampels is:',training_samples)
input_X,input_y=split_sequence(input,n_steps)
# reshape from [samples, timesteps] into [samples, timesteps, features]
input_X = input_X.reshape((input_X.shape[0], input_X.shape[1], n_features))
print('Bidirectional')
# define model
model = Sequential()
model.add(Bidirectional(LSTM(50, activation='relu'), input_shape=(n_steps, n_features)))
model.add(Dense(1))
model.compile(optimizer='adam', loss='mse')
# fit model
model.fit(input_X, input_y, epochs=200, verbose=0)
#Search k nearest data array the normalize for training
if(len(training_samples)!=0):
k_nearest_samples=classify(input,training_samples,K)
print('The k training sampels is:',k_nearest_samples)
#Normalize input and training samples
for k in k_nearest_samples:
training_X,training_y=split_sequence(k,n_steps)
training_X=training_X.reshape((training_X.shape[0],training_X.shape[1],n_features))
model.fit(tr
没有合适的资源?快使用搜索试试~ 我知道了~
温馨提示
毕业设计 基于KNN分类器和双向LSTM实现遥感图像中的缺失值恢复python源码.zip 【项目介绍】 毕业设计,旨在恢复遥感图像中的缺失值。该项目主要分为三个部分:数据清洗、KNN分类器和双向LSTM。 主要功能点 将CSV文件中的不同数据类型转换为浮点数,并将缺失元素赋值为-999。 使用K个最近的数据数组来训练双向LSTM模型。 将输入数组输入到训练好的模型中获得预测结果,并不断更新和优化预测。 技术栈 Python 【备注】 1、该资源内项目代码百分百可运行,请放心下载使用!有问题请及时沟通交流。 2、适用人群:计算机相关专业(如计科、信息安全、数据科学与大数据技术、人工智能、通信、物联网、自动化、电子信息等)在校学生、专业老师或者企业员工下载使用。 3、用途:项目具有较高的学习借鉴价值,不仅适用于小白学习入门进阶。也可作为毕设项目、课程设计、大作业、初期项目立项演示等。 4、如果基础还行,或热爱钻研,亦可在此项目代码基础上进行修改添加,实现其他不同功能。 欢迎下载!欢迎交流学习!不清楚的可以私信问我!
资源推荐
资源详情
资源评论
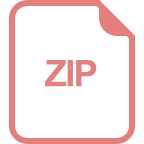
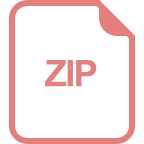
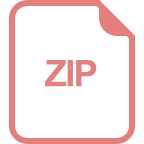
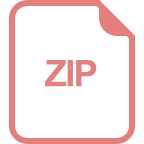
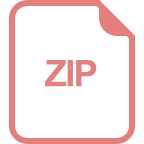
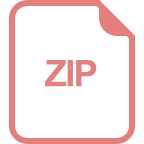
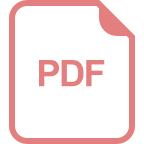
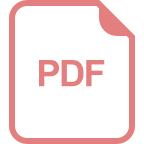
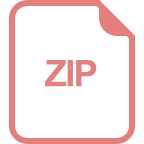
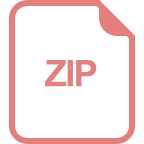
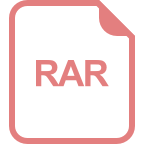
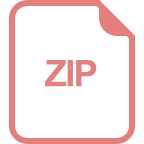
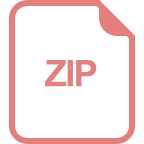
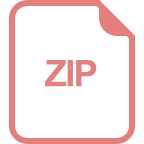
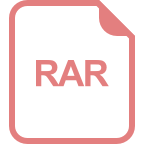
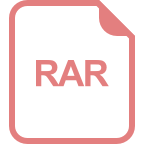
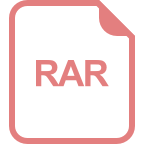
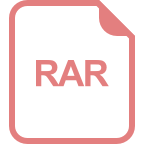
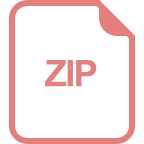
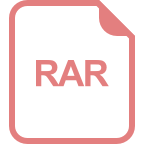
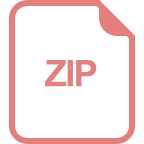
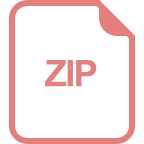
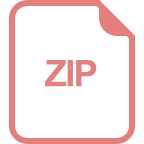
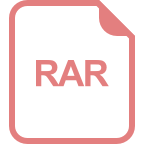
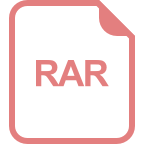
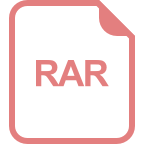
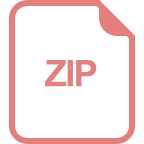
收起资源包目录

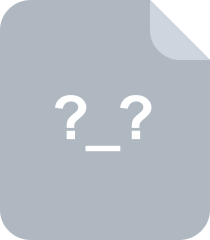
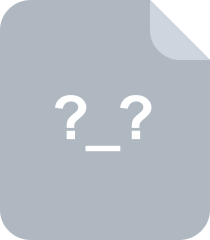
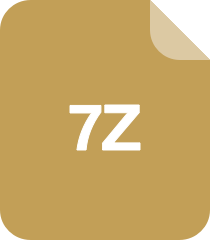
共 3 条
- 1
资源评论
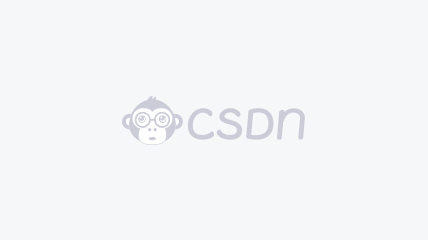

onnx
- 粉丝: 9973
- 资源: 5626
上传资源 快速赚钱
我的内容管理 展开
我的资源 快来上传第一个资源
我的收益
登录查看自己的收益我的积分 登录查看自己的积分
我的C币 登录后查看C币余额
我的收藏
我的下载
下载帮助

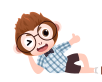
最新资源
资源上传下载、课程学习等过程中有任何疑问或建议,欢迎提出宝贵意见哦~我们会及时处理!
点击此处反馈


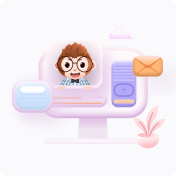
安全验证
文档复制为VIP权益,开通VIP直接复制
