没有合适的资源?快使用搜索试试~ 我知道了~
温馨提示
CUBLAS函数库是CUDA专门用来解决线性代数运算的库,主要进行矩阵运算,分为三个级别:Lev1向量乘向量、Lev2矩阵乘向量、Lev3矩阵乘矩阵,并包含一些功能和状态结构函数。它能支持多种精度的运算,包括单精度和双精度等。 对于矩阵运算来说,CUBLAS库的效率比大部分人自己写核函数高很多。但是CUBLAS不同于C++,是列优先存储, 高效性能:CUBLAS库利用GPU进行加速,实现了高效的线性代数运算。相比传统的CPU运算,GPU并行处理的能力可以大大加快运算速度。 丰富的功能:CUBLAS库包含了完整的BLAS(Basic Linear Algebra Subroutines)函数集,可以进行各种线性代数运算,如矩阵乘法、向量运算等。 易于使用:CUBLAS库提供了友好的API接口,使得用户可以方便地调用库中的函数。同时,库中的函数都经过了高度优化,用户无需关心底层的实现细节,只需要关注自己的业务逻辑即可。 良好的兼容性:CUBLAS库与CUDA平台紧密集成,可以充分利用CUDA的特性,如流(stream)、事件(event)等,可以在CUDA程序中无缝地使用CUBLAS库
资源推荐
资源详情
资源评论
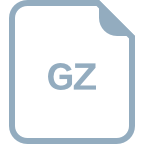
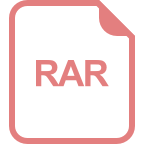
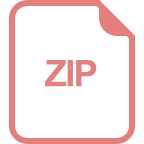
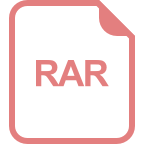
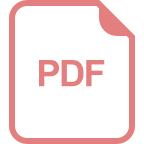
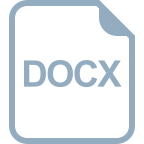
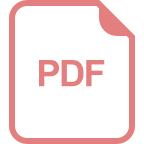
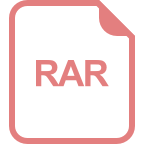
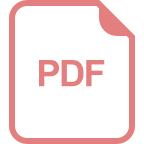
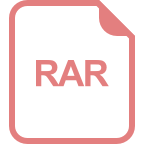
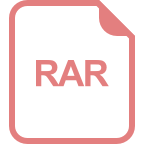
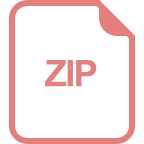
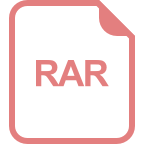
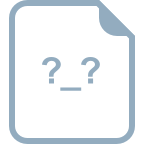
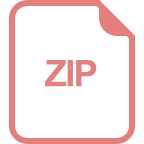
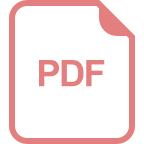
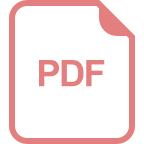
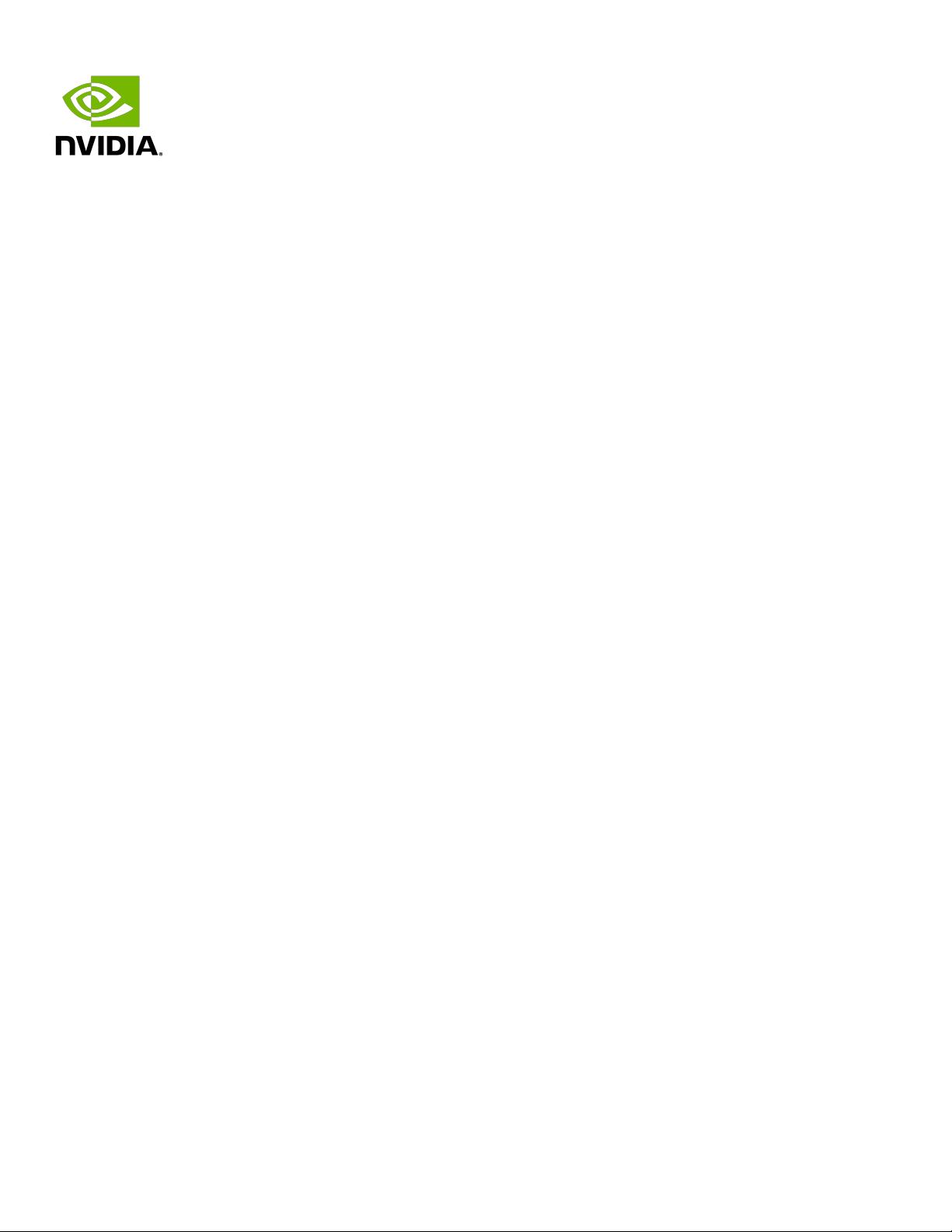
DU-06702-001_v11.8 | October2022
cuBLAS Library
User Guide
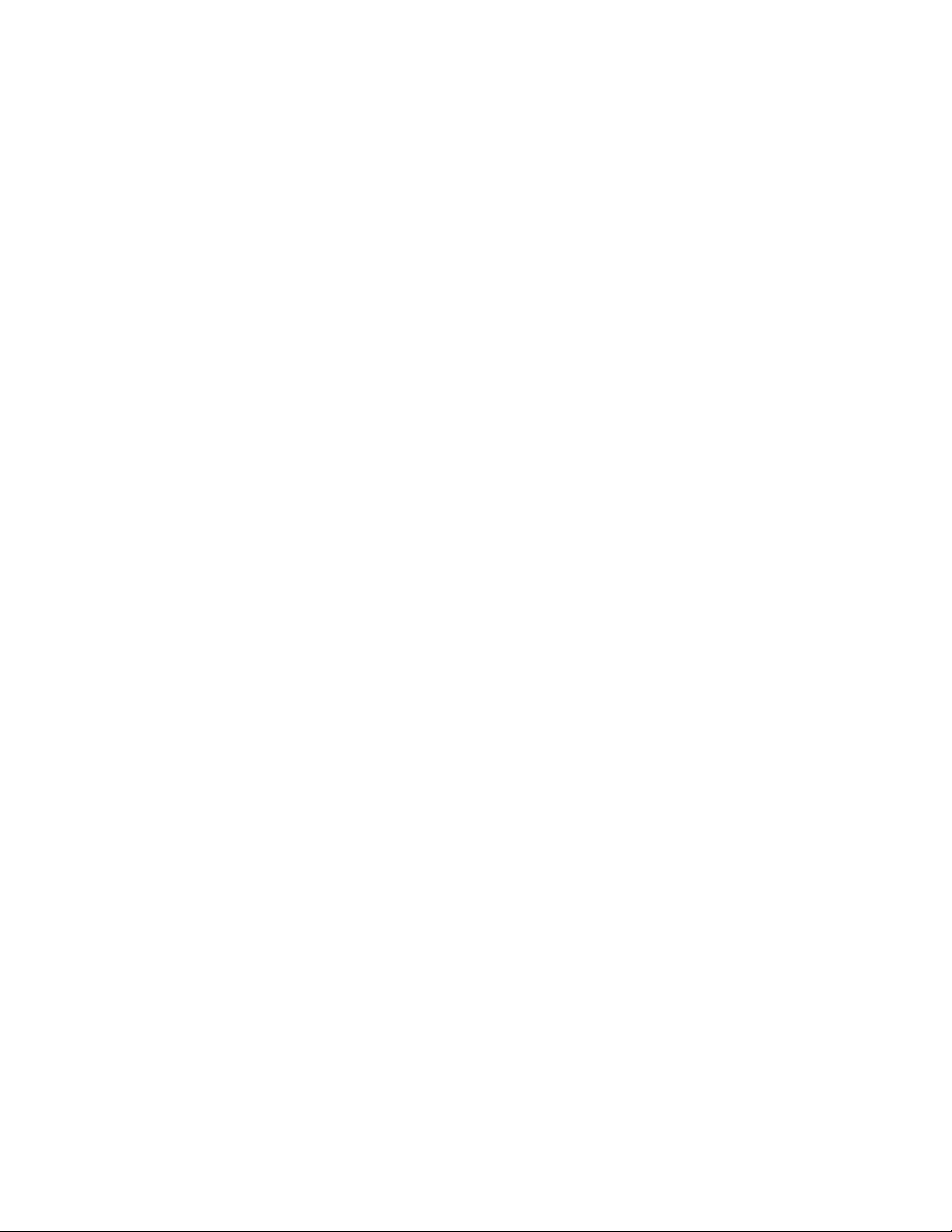
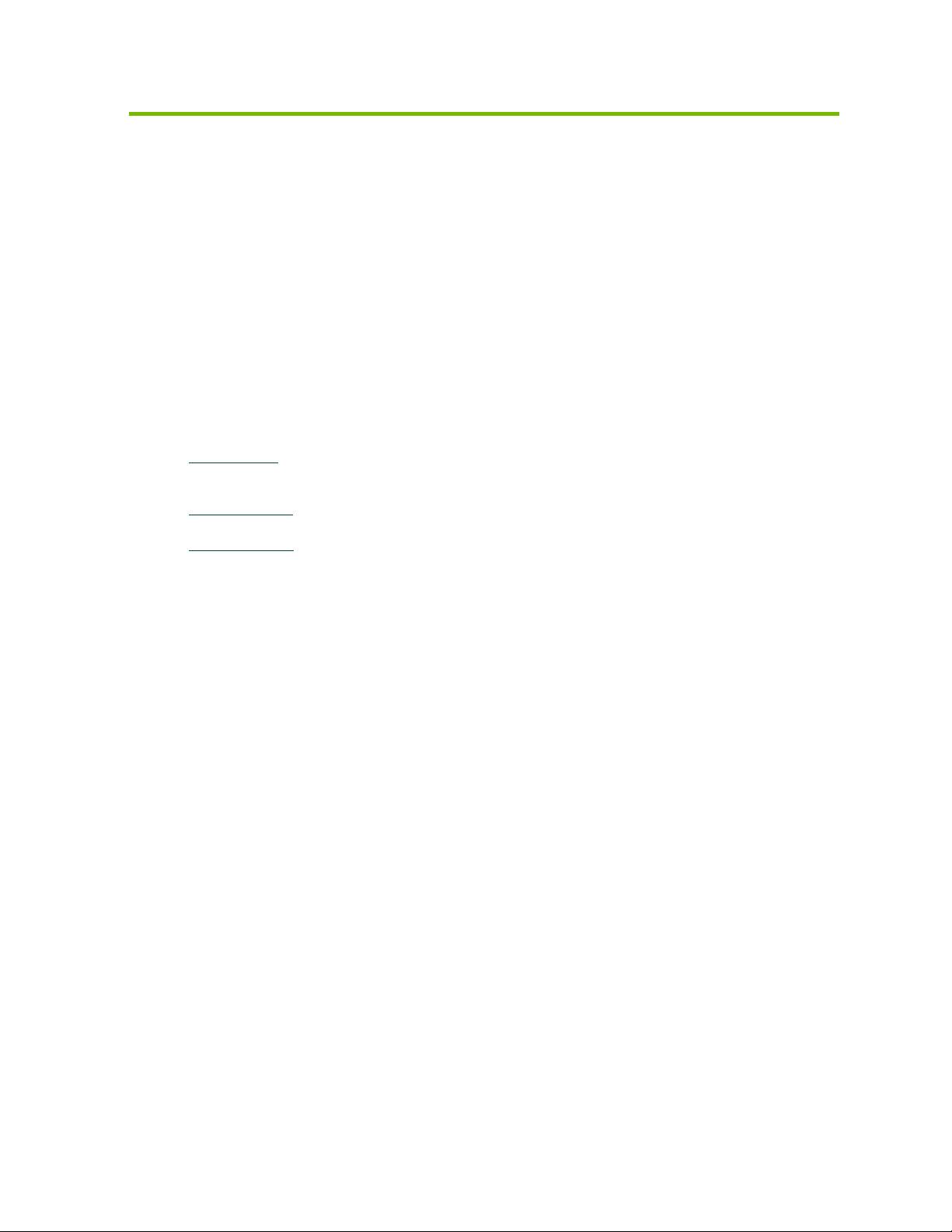
cuBLAS Library DU-06702-001_v11.8|1
Chapter1. Introduction
The cuBLAS library is an implementation of BLAS (Basic Linear Algebra Subprograms) on top
of the NVIDIA
®
CUDA
®
runtime. It allows the user to access the computational resources of
NVIDIA Graphics Processing Unit (GPU).
The cuBLAS Library exposes three sets of API:
‣
The cuBLAS API, which is simply called cuBLAS API in this document (starting with CUDA
6.0),
‣
The cuBLASXt API (starting with CUDA 6.0), and
‣
The cuBLASLt API (starting with CUDA 10.1)
To use the cuBLAS API, the application must allocate the required matrices and vectors in the
GPU memory space, fill them with data, call the sequence of desired cuBLAS functions, and
then upload the results from the GPU memory space back to the host. The cuBLAS API also
provides helper functions for writing and retrieving data from the GPU.
To use the cuBLASXt API, the application may have the data on the Host or any of the devices
involved in the computation, and the Library will take care of dispatching the operation to, and
transferring the data to, one or multiple GPUs present in the system, depending on the user
request.
The cuBLASLt is a lightweight library dedicated to GEneral Matrix-to-matrix Multiply (GEMM)
operations with a new flexible API. This library adds flexibility in matrix data layouts, input
types, compute types, and also in choosing the algorithmic implementations and heuristics
through parameter programmability. After a set of options for the intended GEMM operation
are identified by the user, these options can be used repeatedly for different inputs. This is
analogous to how cuFFT and FFTW first create a plan and reuse for same size and type FFTs
with different input data.
1.1. Data Layout
For maximum compatibility with existing Fortran environments, the cuBLAS library uses
column-major storage, and 1-based indexing. Since C and C++ use row-major storage,
applications written in these languages can not use the native array semantics for two-
dimensional arrays. Instead, macros or inline functions should be defined to implement
matrices on top of one-dimensional arrays. For Fortran code ported to C in mechanical
fashion, one may chose to retain 1-based indexing to avoid the need to transform loops. In this
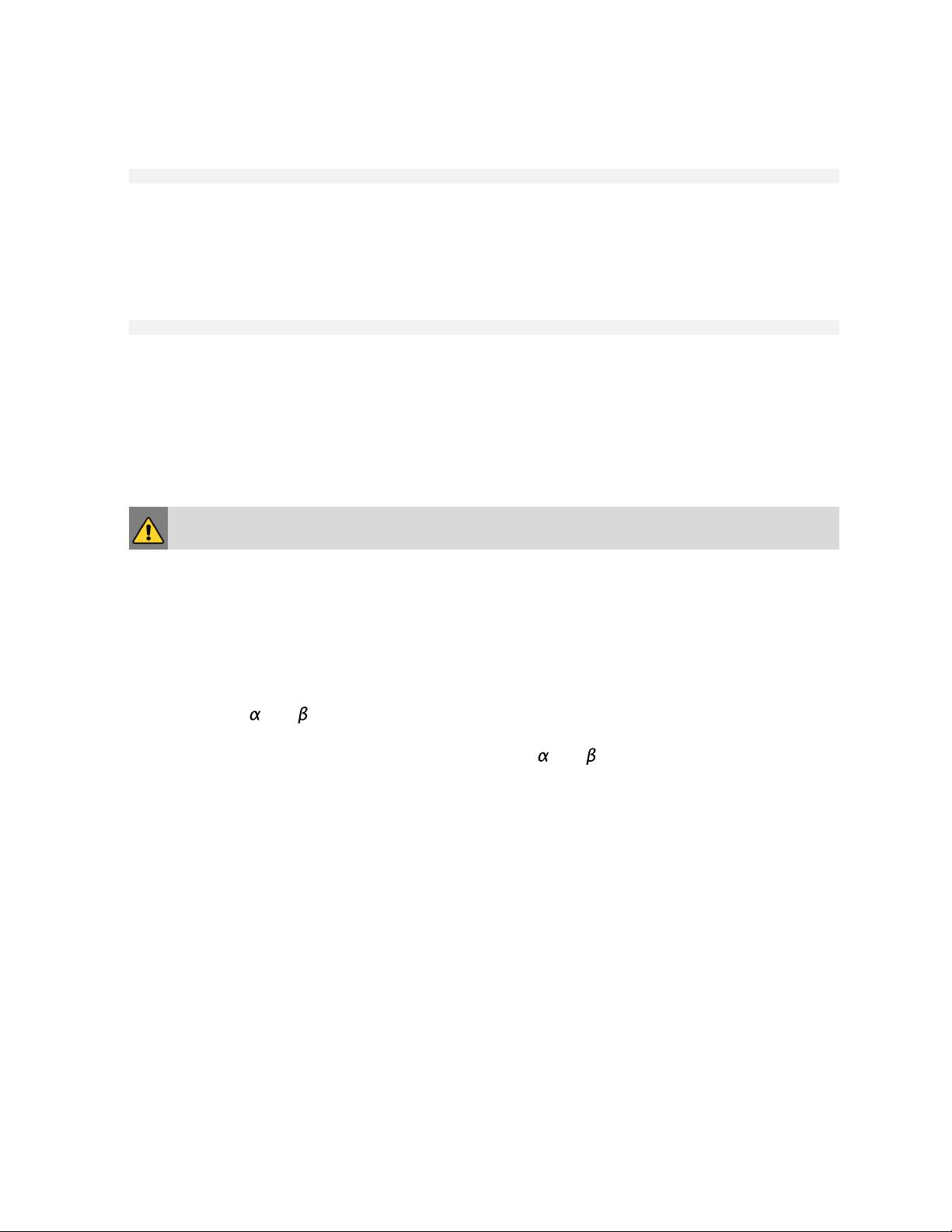
Introduction
cuBLAS Library DU-06702-001_v11.8|2
case, the array index of a matrix element in row “i” and column “j” can be computed via the
following macro
#define IDX2F(i,j,ld) ((((j)-1)*(ld))+((i)-1))
Here, ld refers to the leading dimension of the matrix, which in the case of column-major
storage is the number of rows of the allocated matrix (even if only a submatrix of it is being
used). For natively written C and C++ code, one would most likely choose 0-based indexing, in
which case the array index of a matrix element in row “i” and column “j” can be computed via
the following macro
#define IDX2C(i,j,ld) (((j)*(ld))+(i))
1.2. New and Legacy cuBLAS API
Starting with version 4.0, the cuBLAS Library provides a new API, in addition to the existing
legacy API. This section discusses why a new API is provided, the advantages of using it, and
the differences with the existing legacy API.
WARNING: The legacy cuBLAS API is deprecated and will be removed in a future release.
The new cuBLAS library API can be used by including the header file “cublas_v2.h”. It has
the following features that the legacy cuBLAS API does not have:
‣
The handle to the cuBLAS library context is initialized using the function and is explicitly
passed to every subsequent library function call. This allows the user to have more control
over the library setup when using multiple host threads and multiple GPUs. This also
allows the cuBLAS APIs to be reentrant.
‣
The scalars and can be passed by reference on the host or the device, instead of only
being allowed to be passed by value on the host. This change allows library functions to
execute asynchronously using streams even when and are generated by a previous
kernel.
‣
When a library routine returns a scalar result, it can be returned by reference on the
host or the device, instead of only being allowed to be returned by value only on the host.
This change allows library routines to be called asynchronously when the scalar result is
generated and returned by reference on the device resulting in maximum parallelism.
‣
The error status cublasStatus_t is returned by all cuBLAS library function calls.
This change facilitates debugging and simplifies software development. Note that
cublasStatus was renamed cublasStatus_t to be more consistent with other types in
the cuBLAS library.
‣
The cublasAlloc() and cublasFree() functions have been deprecated. This change
removes these unnecessary wrappers around cudaMalloc() and cudaFree(),
respectively.
‣
The function cublasSetKernelStream() was renamed cublasSetStream() to be more
consistent with the other CUDA libraries.
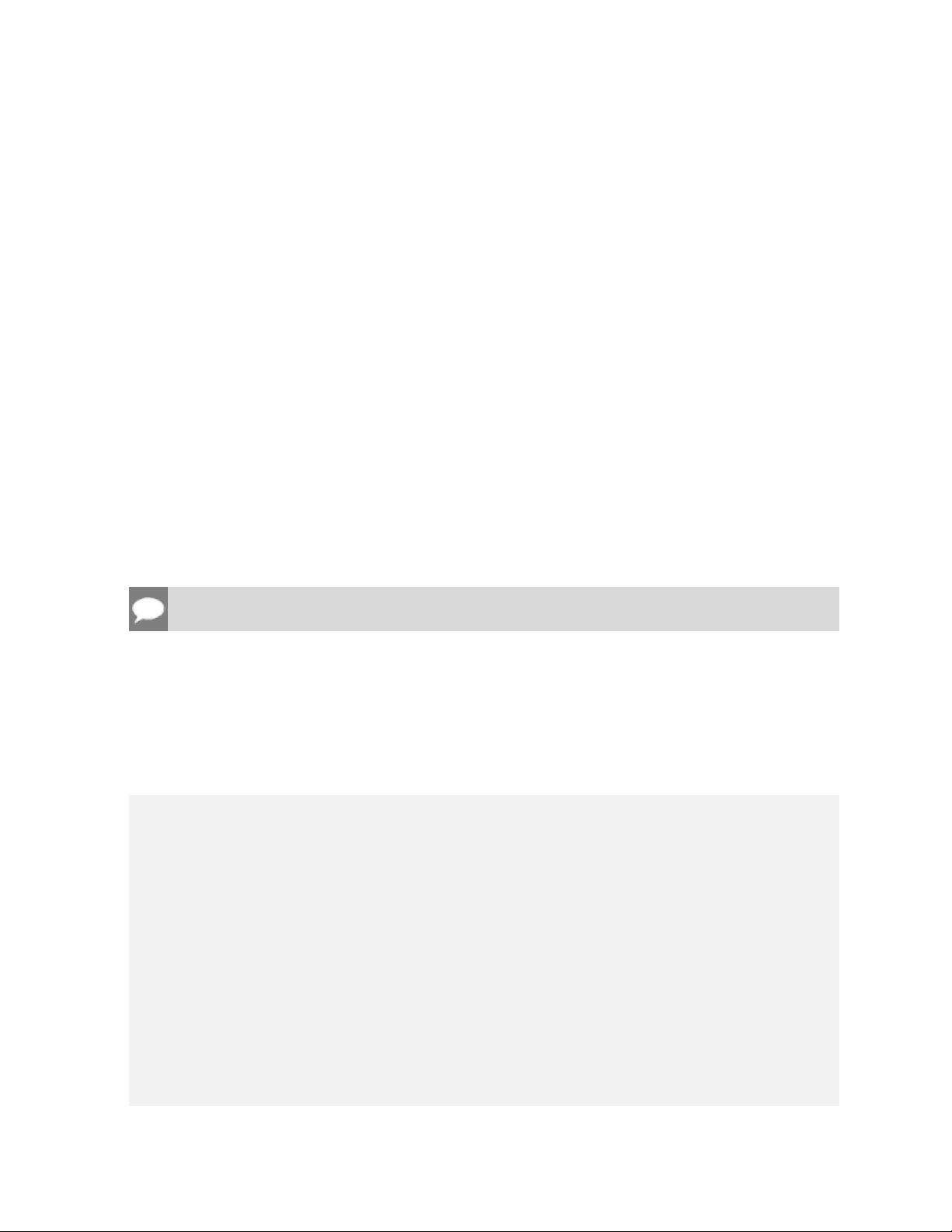
Introduction
cuBLAS Library DU-06702-001_v11.8|3
The legacy cuBLAS API, explained in more detail in the Appendix A, can be used by including
the header file “cublas.h”. Since the legacy API is identical to the previously released cuBLAS
library API, existing applications will work out of the box and automatically use this legacy API
without any source code changes.
The current and the legacy cuBLAS APIs cannot be used simultaneously in a single translation
unit: including both “cublas.h” and “cublas_v2.h” header files will lead to compilation
errors due to incompatible symbol redeclarations.
In general, new applications should not use the legacy cuBLAS API, and existing applications
should convert to using the new API if it requires sophisticated and optimal stream
parallelism, or if it calls cuBLAS routines concurrently from multiple threads.
For the rest of the document, the new cuBLAS Library API will simply be referred to as the
cuBLAS Library API.
As mentioned earlier the interfaces to the legacy and the cuBLAS library APIs are the header
file “cublas.h” and “cublas_v2.h”, respectively. In addition, applications using the cuBLAS
library need to link against:
‣
The DSO cublas.so for Linux,
‣
The DLL cublas.dll for Windows, or
‣
The dynamic library cublas.dylib for Mac OS X.
Note: The same dynamic library implements both the new and legacy cuBLAS APIs.
1.3. Example Code
The following code examples show an application written in C using the cuBLAS library API
with two indexing styles. Example 1 shows 1-based indexing and Example 2 shows 0-based
indexing.
//Example 1. Application Using C and cuBLAS: 1-based indexing
//-----------------------------------------------------------
#include <stdio.h>
#include <stdlib.h>
#include <math.h>
#include <cuda_runtime.h>
#include "cublas_v2.h"
#define M 6
#define N 5
#define IDX2F(i,j,ld) ((((j)-1)*(ld))+((i)-1))
static __inline__ void modify (cublasHandle_t handle, float *m, int ldm, int n, int
p, int q, float alpha, float beta){
cublasSscal (handle, n-q+1, &alpha, &m[IDX2F(p,q,ldm)], ldm);
cublasSscal (handle, ldm-p+1, &beta, &m[IDX2F(p,q,ldm)], 1);
}
int main (void){
cudaError_t cudaStat;
cublasStatus_t stat;
cublasHandle_t handle;
剩余274页未读,继续阅读
资源评论
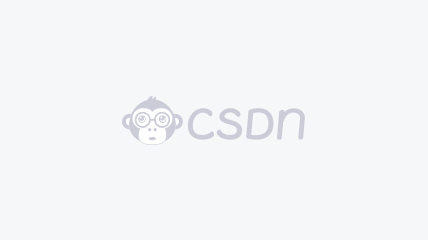

杨咩咩ing
- 粉丝: 395
- 资源: 2
上传资源 快速赚钱
我的内容管理 展开
我的资源 快来上传第一个资源
我的收益
登录查看自己的收益我的积分 登录查看自己的积分
我的C币 登录后查看C币余额
我的收藏
我的下载
下载帮助

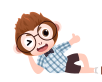
最新资源
资源上传下载、课程学习等过程中有任何疑问或建议,欢迎提出宝贵意见哦~我们会及时处理!
点击此处反馈


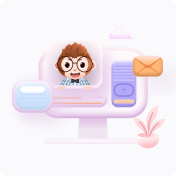
安全验证
文档复制为VIP权益,开通VIP直接复制
