<a href="https://apps.apple.com/app/id1452689527" target="_blank">
<img src="https://user-images.githubusercontent.com/26833433/98699617-a1595a00-2377-11eb-8145-fc674eb9b1a7.jpg" width="1000"></a>
 
<a href="https://github.com/ultralytics/yolov5/actions"><img src="https://github.com/ultralytics/yolov5/workflows/CI%20CPU%20testing/badge.svg" alt="CI CPU testing"></a>
This repository represents Ultralytics open-source research into future object detection methods, and incorporates lessons learned and best practices evolved over thousands of hours of training and evolution on anonymized client datasets. **All code and models are under active development, and are subject to modification or deletion without notice.** Use at your own risk.
<p align="center"><img width="800" src="https://user-images.githubusercontent.com/26833433/114313216-f0a5e100-9af5-11eb-8445-c682b60da2e3.png"></p>
<details>
<summary>YOLOv5-P5 640 Figure (click to expand)</summary>
<p align="center"><img width="800" src="https://user-images.githubusercontent.com/26833433/114313219-f1d70e00-9af5-11eb-9973-52b1f98d321a.png"></p>
</details>
<details>
<summary>Figure Notes (click to expand)</summary>
* GPU Speed measures end-to-end time per image averaged over 5000 COCO val2017 images using a V100 GPU with batch size 32, and includes image preprocessing, PyTorch FP16 inference, postprocessing and NMS.
* EfficientDet data from [google/automl](https://github.com/google/automl) at batch size 8.
* **Reproduce** by `python test.py --task study --data coco.yaml --iou 0.7 --weights yolov5s6.pt yolov5m6.pt yolov5l6.pt yolov5x6.pt`
</details>
- **April 11, 2021**: [v5.0 release](https://github.com/ultralytics/yolov5/releases/tag/v5.0): YOLOv5-P6 1280 models, [AWS](https://github.com/ultralytics/yolov5/wiki/AWS-Quickstart), [Supervise.ly](https://github.com/ultralytics/yolov5/issues/2518) and [YouTube](https://github.com/ultralytics/yolov5/pull/2752) integrations.
- **January 5, 2021**: [v4.0 release](https://github.com/ultralytics/yolov5/releases/tag/v4.0): nn.SiLU() activations, [Weights & Biases](https://wandb.ai/site?utm_campaign=repo_yolo_readme) logging, [PyTorch Hub](https://pytorch.org/hub/ultralytics_yolov5/) integration.
- **August 13, 2020**: [v3.0 release](https://github.com/ultralytics/yolov5/releases/tag/v3.0): nn.Hardswish() activations, data autodownload, native AMP.
- **July 23, 2020**: [v2.0 release](https://github.com/ultralytics/yolov5/releases/tag/v2.0): improved model definition, training and mAP.
## Pretrained Checkpoints
[assets]: https://github.com/ultralytics/yolov5/releases
Model |size<br><sup>(pixels) |mAP<sup>val<br>0.5:0.95 |mAP<sup>test<br>0.5:0.95 |mAP<sup>val<br>0.5 |Speed<br><sup>V100 (ms) | |params<br><sup>(M) |FLOPS<br><sup>640 (B)
--- |--- |--- |--- |--- |--- |---|--- |---
[YOLOv5s][assets] |640 |36.7 |36.7 |55.4 |**2.0** | |7.3 |17.0
[YOLOv5m][assets] |640 |44.5 |44.5 |63.3 |2.7 | |21.4 |51.3
[YOLOv5l][assets] |640 |48.2 |48.2 |66.9 |3.8 | |47.0 |115.4
[YOLOv5x][assets] |640 |**50.4** |**50.4** |**68.8** |6.1 | |87.7 |218.8
| | | | | | || |
[YOLOv5s6][assets] |1280 |43.3 |43.3 |61.9 |**4.3** | |12.7 |17.4
[YOLOv5m6][assets] |1280 |50.5 |50.5 |68.7 |8.4 | |35.9 |52.4
[YOLOv5l6][assets] |1280 |53.4 |53.4 |71.1 |12.3 | |77.2 |117.7
[YOLOv5x6][assets] |1280 |**54.4** |**54.4** |**72.0** |22.4 | |141.8 |222.9
| | | | | | || |
[YOLOv5x6][assets] TTA |1280 |**55.0** |**55.0** |**72.0** |70.8 | |- |-
<details>
<summary>Table Notes (click to expand)</summary>
* AP<sup>test</sup> denotes COCO [test-dev2017](http://cocodataset.org/#upload) server results, all other AP results denote val2017 accuracy.
* AP values are for single-model single-scale unless otherwise noted. **Reproduce mAP** by `python test.py --data coco.yaml --img 640 --conf 0.001 --iou 0.65`
* Speed<sub>GPU</sub> averaged over 5000 COCO val2017 images using a GCP [n1-standard-16](https://cloud.google.com/compute/docs/machine-types#n1_standard_machine_types) V100 instance, and includes FP16 inference, postprocessing and NMS. **Reproduce speed** by `python test.py --data coco.yaml --img 640 --conf 0.25 --iou 0.45`
* All checkpoints are trained to 300 epochs with default settings and hyperparameters (no autoaugmentation).
* Test Time Augmentation ([TTA](https://github.com/ultralytics/yolov5/issues/303)) includes reflection and scale augmentation. **Reproduce TTA** by `python test.py --data coco.yaml --img 1536 --iou 0.7 --augment`
</details>
## Requirements
Python 3.8 or later with all [requirements.txt](https://github.com/ultralytics/yolov5/blob/master/requirements.txt) dependencies installed, including `torch>=1.7`. To install run:
```bash
$ pip install -r requirements.txt
```
## Tutorials
* [Train Custom Data](https://github.com/ultralytics/yolov5/wiki/Train-Custom-Data) ð RECOMMENDED
* [Tips for Best Training Results](https://github.com/ultralytics/yolov5/wiki/Tips-for-Best-Training-Results) âï¸ RECOMMENDED
* [Weights & Biases Logging](https://github.com/ultralytics/yolov5/issues/1289) ð NEW
* [Supervisely Ecosystem](https://github.com/ultralytics/yolov5/issues/2518) ð NEW
* [Multi-GPU Training](https://github.com/ultralytics/yolov5/issues/475)
* [PyTorch Hub](https://github.com/ultralytics/yolov5/issues/36) â NEW
* [ONNX and TorchScript Export](https://github.com/ultralytics/yolov5/issues/251)
* [Test-Time Augmentation (TTA)](https://github.com/ultralytics/yolov5/issues/303)
* [Model Ensembling](https://github.com/ultralytics/yolov5/issues/318)
* [Model Pruning/Sparsity](https://github.com/ultralytics/yolov5/issues/304)
* [Hyperparameter Evolution](https://github.com/ultralytics/yolov5/issues/607)
* [Transfer Learning with Frozen Layers](https://github.com/ultralytics/yolov5/issues/1314) â NEW
* [TensorRT Deployment](https://github.com/wang-xinyu/tensorrtx)
## Environments
YOLOv5 may be run in any of the following up-to-date verified environments (with all dependencies including [CUDA](https://developer.nvidia.com/cuda)/[CUDNN](https://developer.nvidia.com/cudnn), [Python](https://www.python.org/) and [PyTorch](https://pytorch.org/) preinstalled):
- **Google Colab and Kaggle** notebooks with free GPU: <a href="https://colab.research.google.com/github/ultralytics/yolov5/blob/master/tutorial.ipynb"><img src="https://colab.research.google.com/assets/colab-badge.svg" alt="Open In Colab"></a> <a href="https://www.kaggle.com/ultralytics/yolov5"><img src="https://kaggle.com/static/images/open-in-kaggle.svg" alt="Open In Kaggle"></a>
- **Google Cloud** Deep Learning VM. See [GCP Quickstart Guide](https://github.com/ultralytics/yolov5/wiki/GCP-Quickstart)
- **Amazon** Deep Learning AMI. See [AWS Quickstart Guide](https://github.com/ultralytics/yolov5/wiki/AWS-Quickstart)
- **Docker Image**. See [Docker Quickstart Guide](https://github.com/ultralytics/yolov5/wiki/Docker-Quickstart) <a href="https://hub.docker.com/r/ultralytics/yolov5"><img src="https://img.shields.io/docker/pulls/ultralytics/yolov5?logo=docker" alt="Docker Pulls"></a>
## Inference
`detect.py` runs inference on a variety of sources, downloading models automatically from the [latest YOLOv5 release](https://github.com/ultralytics/yolov5/releases) and saving results to `runs/detect`.
```bash
$ python detect.py --source 0 # webcam
file.jpg # image
file.mp4 # video
path/ # directory
path/*.jpg # glob
'https://youtu.be/NUsoVlDFqZg' # YouTube video
'rtsp://example.com/media.mp4' # RTSP, RTMP, HTTP stream
```
To run inference on example images in `data/images`:
```bash
$ python detect.py
没有合适的资源?快使用搜索试试~ 我知道了~
YOLOv5算法dms驾驶员喝水-饮料检测权重+1000数据集
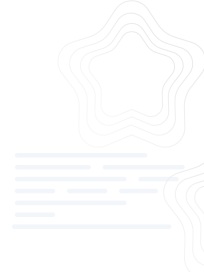
共2000个文件
txt:1003个
jpg:929个
yaml:29个

1.该资源内容由用户上传,如若侵权请联系客服进行举报
2.虚拟产品一经售出概不退款(资源遇到问题,请及时私信上传者)
2.虚拟产品一经售出概不退款(资源遇到问题,请及时私信上传者)
版权申诉
0 下载量 63 浏览量
2024-05-31
21:51:53
上传
评论
收藏 89.15MB ZIP 举报
温馨提示
YOLOv5DMS驾驶员喝水检测权重,1000多数据集,目录已经配置好,划分好 train,val, test,并附有data.yaml文件,yolov5、yolov7、yolov8,yolov9等算法可以直接进行训练模型,txt格式标签, 数据集和检测结果参考:https://blog.csdn.net/zhiqingAI/article/details/124230743 https://download.csdn.net/download/zhiqingAI/88935038 数据集配置目录结构data.yaml: nc: 1 names: - Drinking
资源推荐
资源详情
资源评论
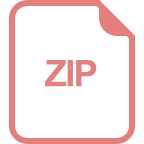
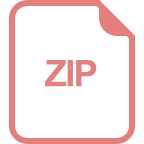
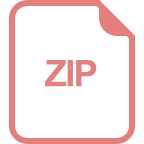
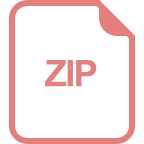
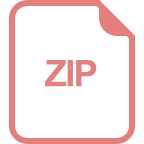
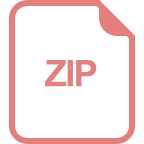
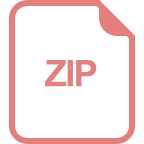
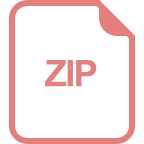
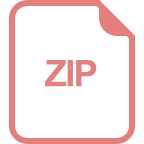
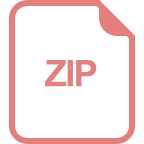
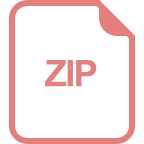
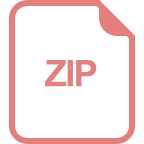
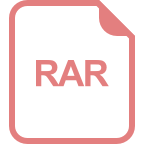
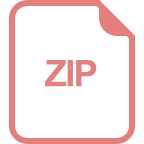
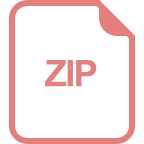
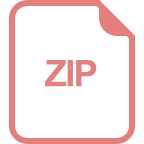
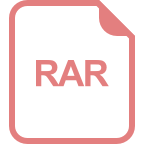
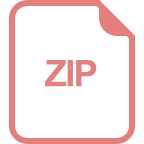
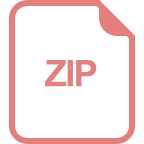
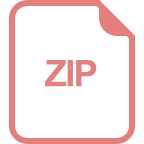
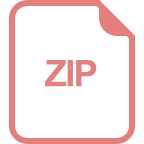
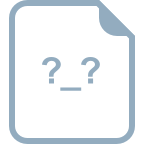
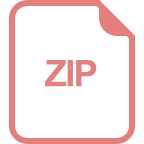
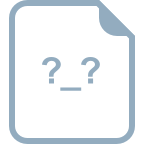
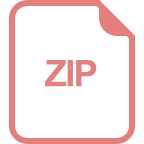
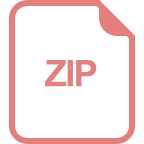
收起资源包目录

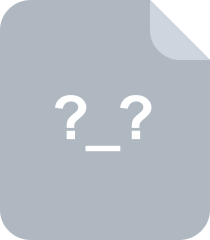
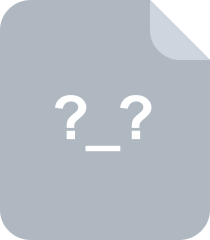
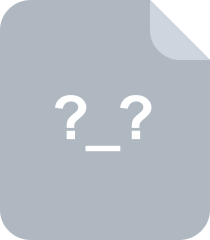
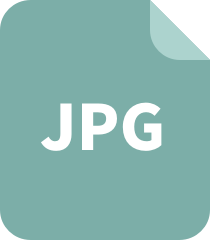
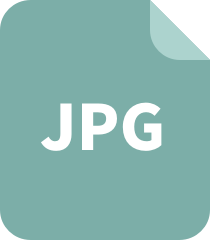
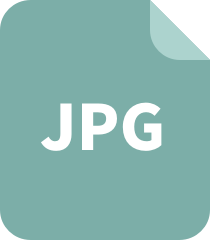
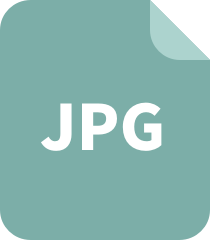
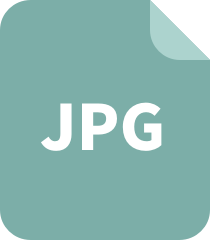
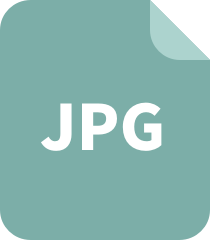
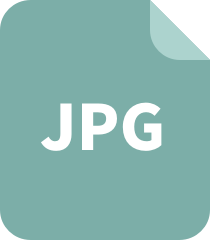
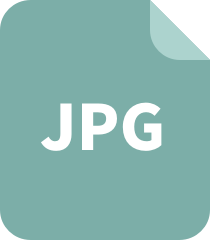
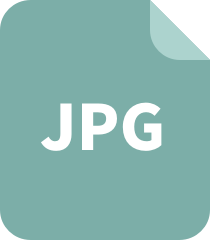
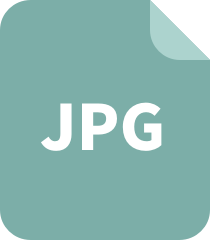
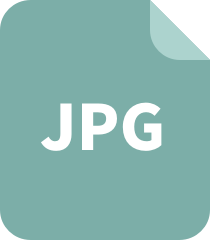
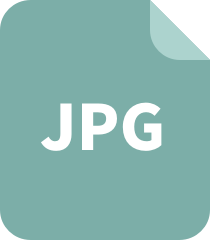
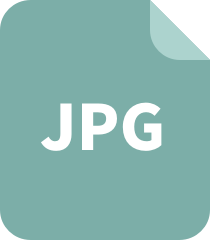
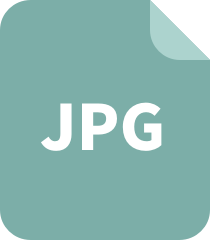
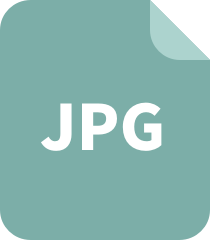
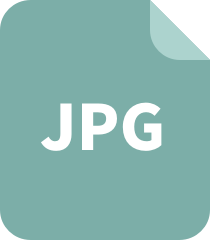
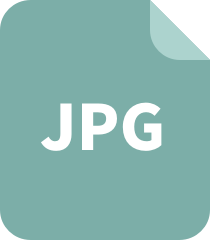
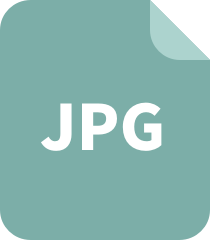
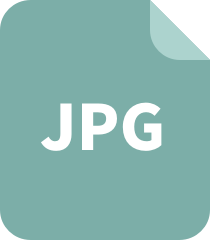
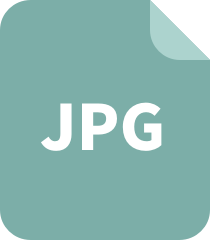
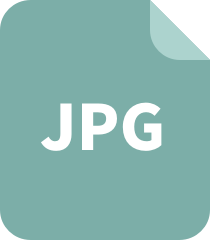
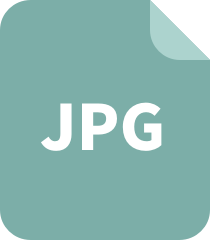
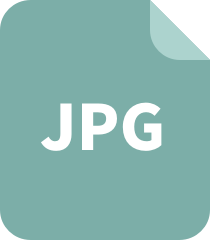
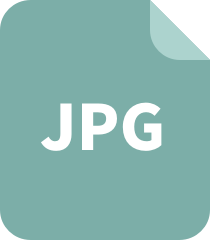
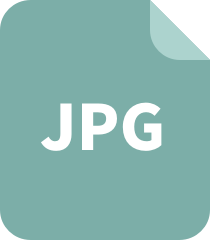
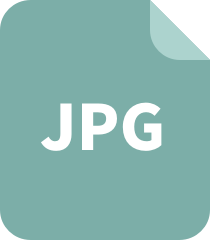
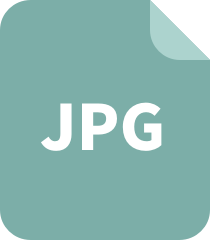
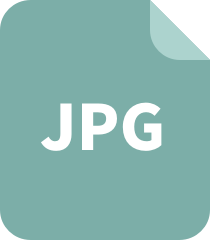
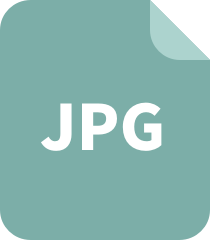
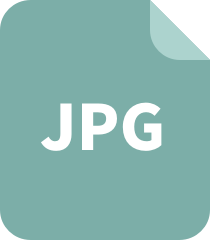
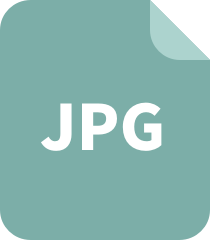
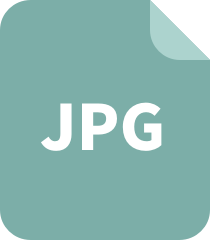
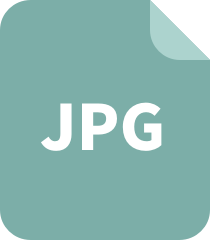
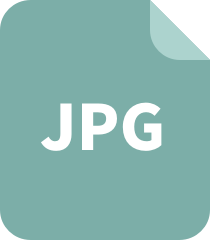
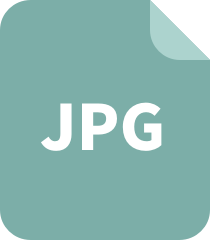
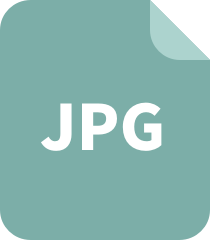
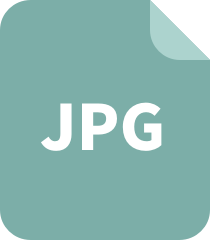
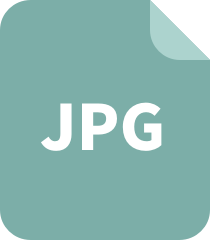
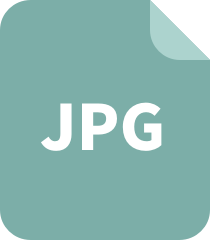
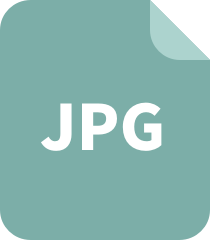
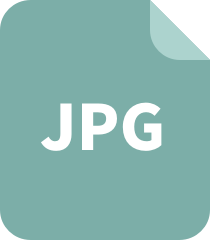
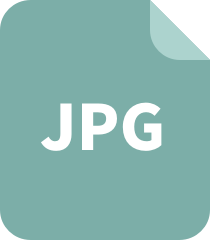
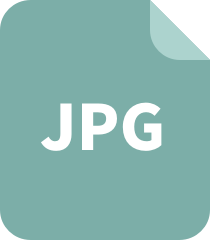
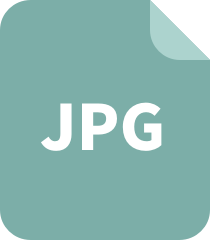
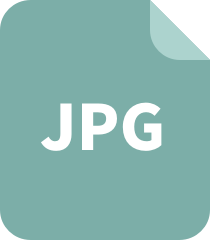
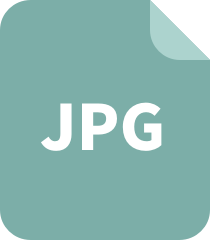
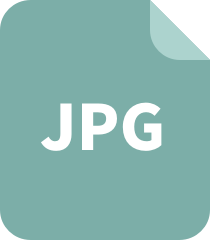
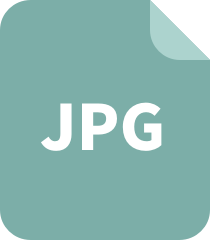
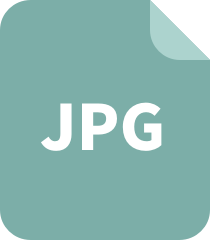
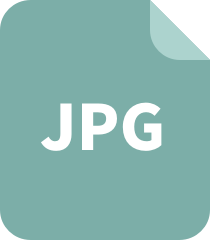
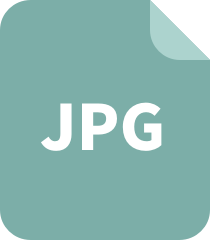
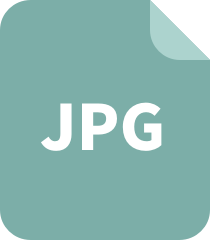
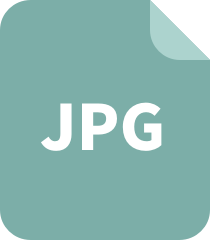
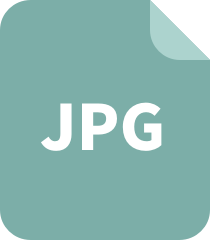
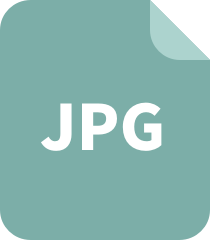
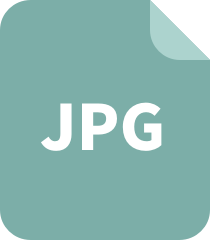
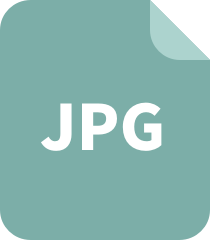
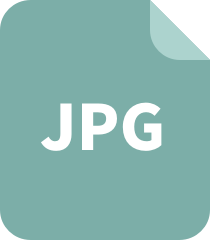
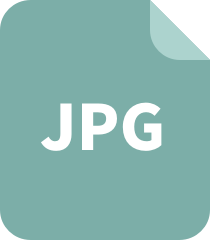
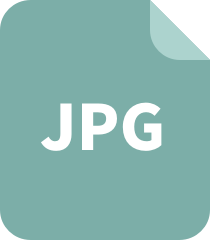
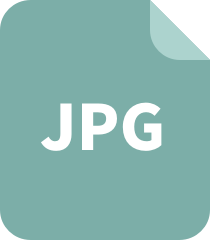
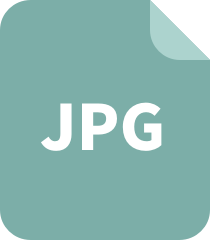
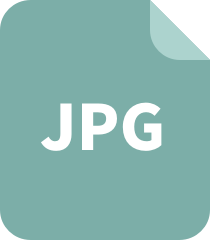
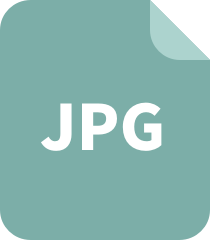
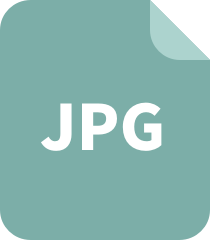
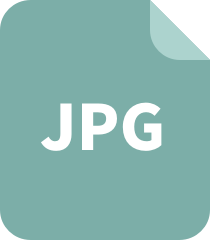
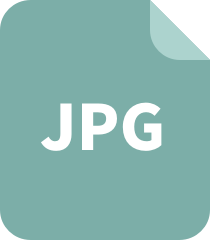
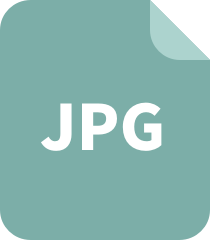
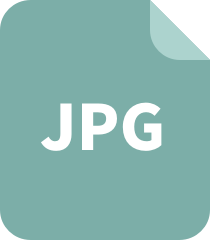
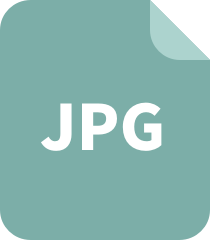
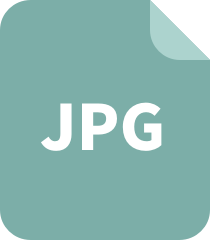
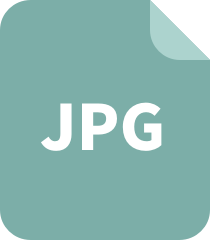
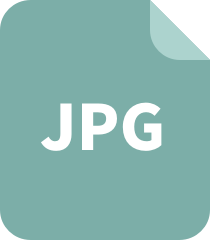
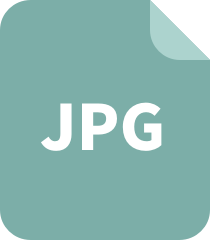
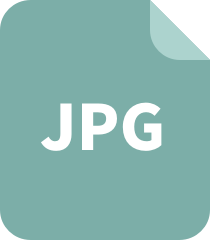
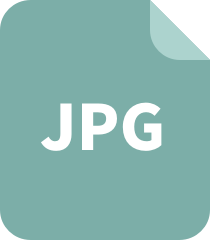
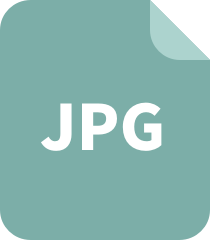
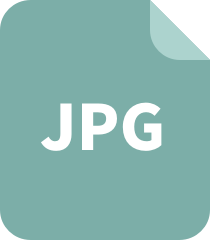
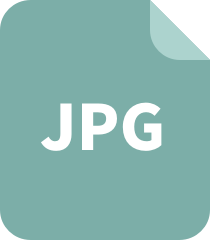
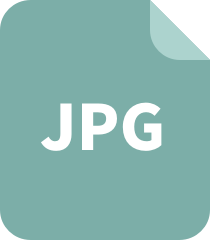
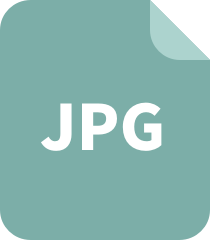
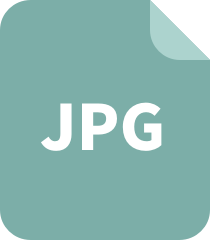
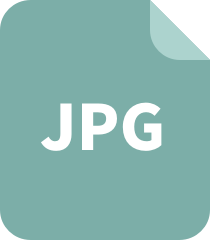
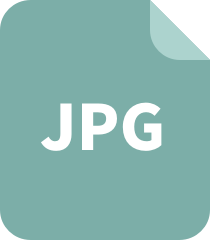
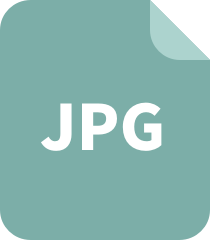
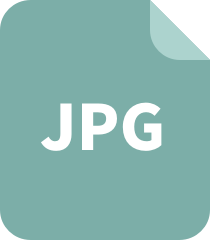
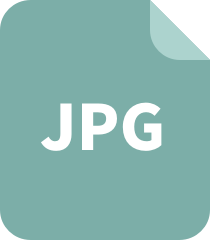
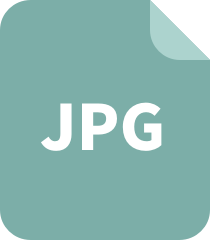
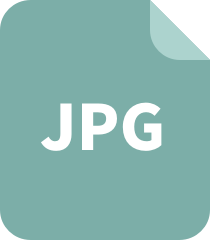
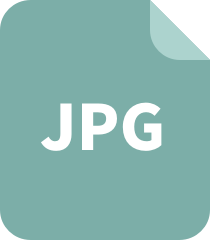
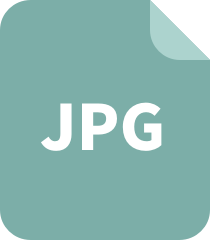
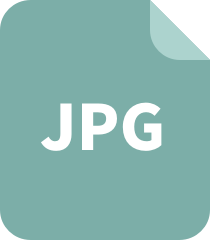
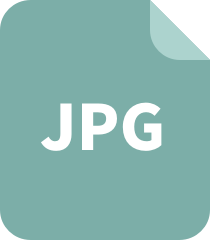
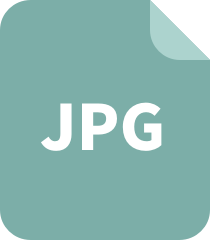
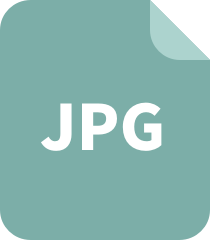
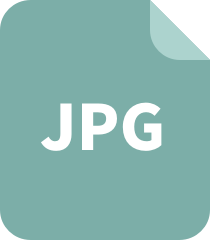
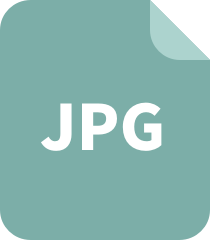
共 2000 条
- 1
- 2
- 3
- 4
- 5
- 6
- 20
资源评论
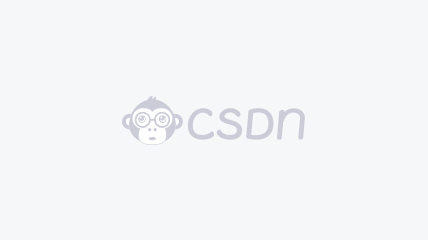

stsdddd
- 粉丝: 3w+
- 资源: 929
上传资源 快速赚钱
我的内容管理 展开
我的资源 快来上传第一个资源
我的收益
登录查看自己的收益我的积分 登录查看自己的积分
我的C币 登录后查看C币余额
我的收藏
我的下载
下载帮助

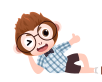
最新资源
- apache-maven-3.6.1-bin.zip
- c593f5fc-d4a7-4b43-8ab2-51afc90f3f62
- IIR滤波器参数计算函数
- WPF树菜单拖拽功能,下级目录拖到上级目录,上级目录拖到下级目录.zip
- CDH6.3.2版本hive2.1.1修复HIVE-14706后的jar包
- 鸿蒙项目实战-天气项目(当前城市天气、温度、湿度,24h天气,未来七天天气预报,生活指数,城市选择等)
- Linux环境下oracle数据库服务器配置中文最新版本
- Linux操作系统中Oracle11g数据库安装步骤详细图解中文最新版本
- SMA中心接触件插合力量(插入力及分离力)仿真
- 变色龙记事本,有NPP功能,JSONview功能
资源上传下载、课程学习等过程中有任何疑问或建议,欢迎提出宝贵意见哦~我们会及时处理!
点击此处反馈


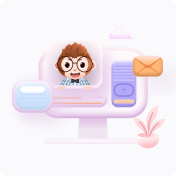
安全验证
文档复制为VIP权益,开通VIP直接复制
