没有合适的资源?快使用搜索试试~ 我知道了~
资源推荐
资源详情
资源评论
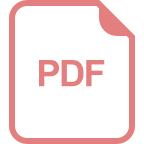
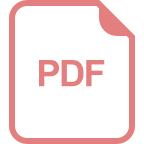
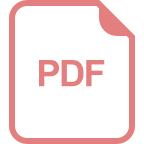
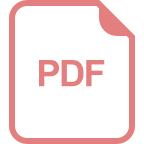
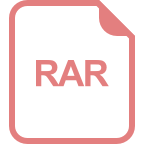
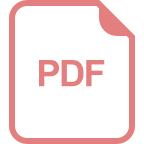
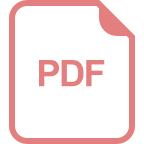
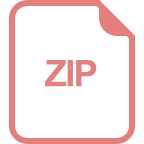
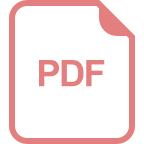
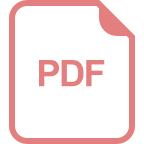
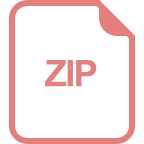
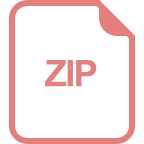
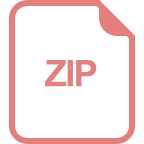
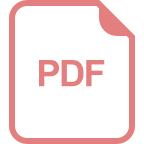
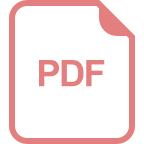
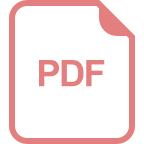
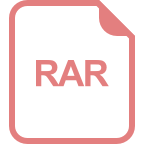
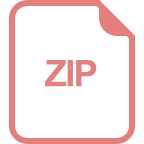
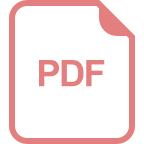
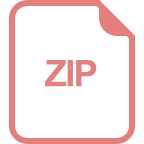
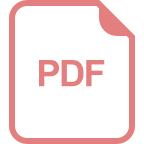
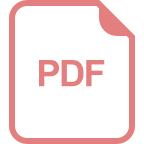
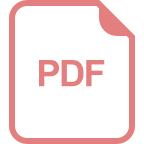
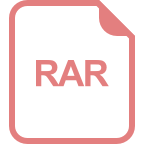
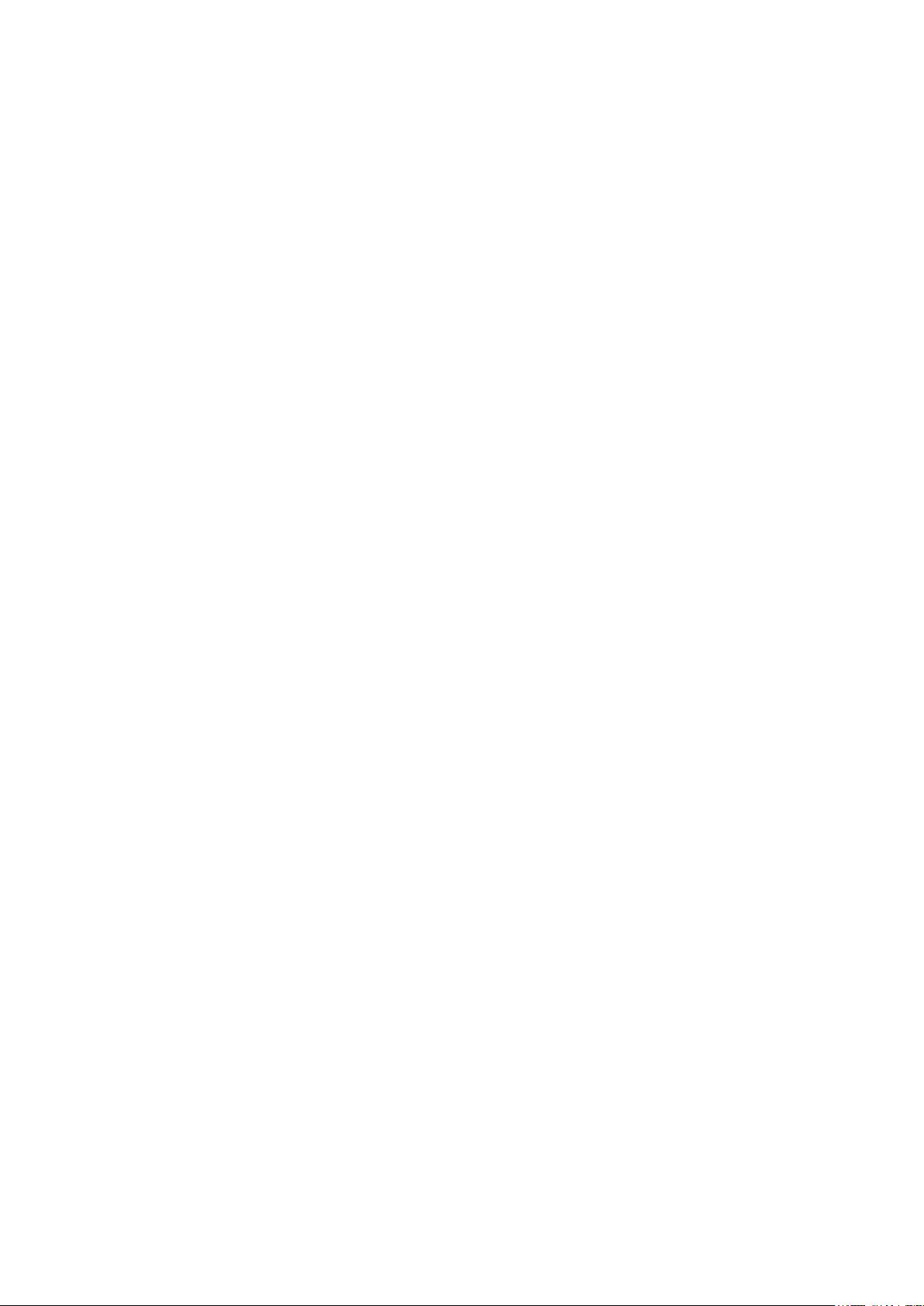
Optimal Trajectory Generation for Autonomous Vehicles Under
Centripetal Acceleration Constraints for In-lane Driving Scenarios
Yajia Zhang*, Hongyi Sun, Jinyun Zhou, Jiangtao Hu, Jinghao Miao
Abstract— This paper presents a noval method that gener-
ates optimal trajectories for autonomous vehicles for in-lane
driving scenarios. The method computes a trajectory using
a two-phase optimization procedure. In the first phase, the
optimization procedure generates a close-form driving guide
line with differetiable curvatures. In the second phase, the
procedure takes the driving guide line as input, and outputs
dynamically feasible, jerk and time optimal trajectories for
vehicles driving along the guide line. This method is especially
useful for generating trajectories at curvy road where the
vehicles need to apply frequent accelerations and decelerations
to accommodate centripetal acceleration limits.
I. INTRODUCTION
Trajectory planning is an important component in au-
tonomous driving systems (ADS). It plays a critical role
on safety and comfort. Safety is of top priority as any
collision might lead to hazardous situations. Assuming pre-
dicted trajectories of surrounding obstacles are given from
upper stream module of ADS, path-time obstacle graph is
a commonly used tool for collision avoidance analysis if
future path of the autonomous driving vehicle (ADV) is
determined. This method projects the predicted trajectories of
surrounding obstacles onto the spatio-time plane and forms
path-time obstacles which specify at which time the further
path of the ADV would be on collision. The free area forms
the collision-free zone for trajectory planning. This method
is particularly useful for autonomous vehicles in structured
road scenarios. It fully utilizes the domain knowledge, as
most vehicles are driving along lanes. The method we pro-
pose adopts path-time-obstacle graph in collision avoidance
analysis and always plans a trajectory that lie within the
collision-free zone.
Comfort is another goal to achieve for ADS. Several
factors affect and are used to measure the comfort of one
trajectory. Acceleration and acceleration change rate (com-
monly known as jerk) are most commonly used metrics for
vehicle trajectories. Furthermore, depending on the direction,
human weight acceleration and jerk significant differently for
longitudinal and lateral movement. Acceleration and jerk in
lateral direction must be bounded and minimized. For driving
along a curvy road, the longitudinal speed must be adjusted
frequently according to the curve, i.e., the curvature of the
road. A driving guide line is an abstraction of the road
center line, which contains the geometrical information of
the road. We assume the target of the autonomous vehicle
in-lane driving is following the driving guide line. To achieve
Yajia Zhang, Hongyi Sun, Jinyun Zhou, Jiangtao Hu, Jinghao Miao are
with Baidu USA LLC, 250 Caribbean Drive Sunnyvale, USA *Correspond-
ing author: Yajia Zhang zhangyajia@baidu.com
comfortable riding experience, the vehicle needs to accelerate
and decelerate according to the curvature of the driving guide
line. In our proposed method, the algorithm can directly
consider the geometrical information of the driving guide
line.
Optimization is a common approach in trajectory gen-
eration as it takes the objective or cost function and con-
straints directly into trajectory generation. For high degrees
of freedom (DOFs) configuration space, optimization for
trajectory generation is generally slow and prone to local
minima, it is generally suitable for lower dimensional vehicle
configuration space. In our method, we use a two-phase
optimization procedure. Each one intends to solve a subset of
trajectory generation problem. In this way, it greatly reduces
the overall complexity of optimization. For the first phase,
our method generates a smooth driving guide line for ADV
to follow; in the second phase, the optimization procedure
takes the collision-free zone resulted from path-time obstacle
graph analysis and the close-formed driving guide line as
input, and generates a collision-free and comfort trajectory
that minimizes longitudinal acceleration, and centripetal ac-
celeration and jerk.
II. RELATED WORK
Trajectory planning is a critical component in autonomous
driving systems. Recently, a number of algorithms [7], [8],
[10] have been developed since DARPA Grand Challenge
(2004, 2005) and Urban Challenge (2007).
Randomized planners such as Rapidly Exploring Random
Tree (RRT)[6] are intended to solve high-DOF robot motion
planning with differential constraints. However, it is difficult
for randomized planners to utilize the domain knowledge
from the structured environment for quickly convergence.
Nevertheless, the computed trajectory is generally low qual-
ity and thus cannot be used directly without a post-processing
step. Recent research on optimal randomized planner, such as
[3], can produce high-quality trajectories given enough plan-
ning time. But the convergence to optimal trajectory takes
rather long time thus it cannot be used in the dynamically
changing environment.
Discrete search method [5] computes a trajectory by
concatenating a sequence of pre-computed maneuvers. The
contatenation is done by checking whether the ending state
of a maneuver is sufficiently close to the starting state of
the target maneuver. This method generally works well for
simple environment such as highway scenarios. However, the
number of required maneuvers needs to grow exponentially
in order to solve complex urban driving cases.
2019 IEEE Intelligent Transportation Systems Conference (ITSC)
Auckland, NZ, October 27-30, 2019
978-1-5386-7024-8/19/$31.00 ©2019 IEEE 3619
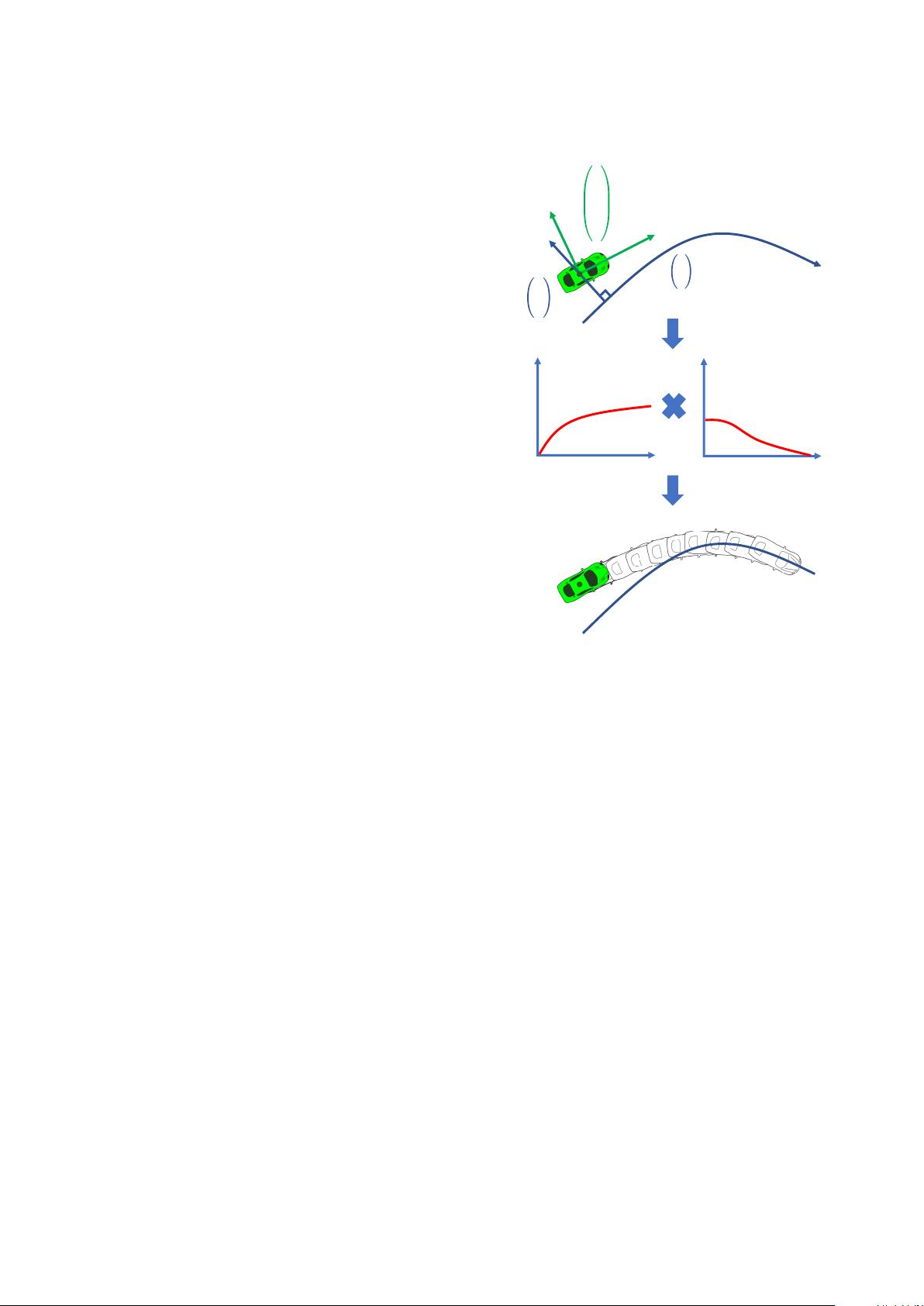
The work in [13] runs an quadratic programming pro-
cedure in global/map frame. The trajectory is finely dis-
cretized in Cartesian space. The positional attributions of the
trajectory are directly used as optimization variables, and
outputs a trajectory that minimizes the objective function
which combines the measurement of safety and comfort.
The advantage of using optimization is it provides direct
enforcement of optimality modeling. The dense discretization
approach provides maximal control of trajectory to tackle
complex scenarios.
In [12], trajectory planning is performed in Frenet frame.
Given a smooth driving guide line, this method decouples
the movement of vehicle in map frame into two orthogonal
movements, one longitudinal movement that along the driv-
ing guide line and one lateral movement that perpendicular
to the guide line. For both movements, the trajectories are
generated using random samples, i.e., the end conditions
and parameter are discretized into certain resolution. The
sampled end conditions are directly connected with the
initial condition using quintic or quartic polynomials. Then
these longitudinal and lateral trajectories are combined and
selected according to a predefined cost function.
The major drawback with the method is it lacks control of
the trajectory. For complex driving scenarios, it is difficult for
this method to generate feasible trajectories. Nevertheless,
some caveats of using polynomial include problems with
stopping, unexpected acceleration and deceleration. To tackle
complex problems in real world, we need to maximize our
ability of controlling the trajectory. Thus, optimization is
a promising direction as constraints in the task domain
can be directly considered in trajectory generation. Exist-
ing optimization-based methods such as [13] [9] runs the
optimization in map frame.
The overall algorithm framework we proposed is similar
to the one in [12], however, we use optimization to solve the
1d planning problems, which greatly enhances the flexibility
of the trajectory.
The method we present hybrids the Frenet frame trajectory
planning framework and optimization-based trajectory gener-
ation. Also, the trajectory optimization is performed in two-
phases, where each phase is a lower dimensional problem. In
this proposed method, the difficulty of optimization is greatly
reduced.
III. PROBLEM DEFINITION
The configuration for a vehicle with differential constraints
in Cartesian space can be represented using three variables,
(x, y, θ), where x, y specify the coordinate of some reference
point for the vehicle and θ specifies the vehicle’s heading
angle in the Cartesian space. In our work, we incorporate
one more dimension κ, which is the instant curvature resulted
from vehicle’s steering, into the configuration space for more
accurate configuration modeling, and hence the computed
trajectory provides additional information that can be used
for better designing the feedback controller. Trajectory plan-
ning for non-holonomic vehicles is essentially finding a
!
"
"
!
#
$
%
&
'
&
(
&
)
'
)
()
)
&
)
*
&
*
Fig. 1. Illustration of vehicle trajectory planning in assist of Frenet frame.
First, the vehicle dynamic state (x, y, θ, κ, v, a), which represents vehicles
position, heading, steering angle, velocity and acceleration, respective,
is projected on to a given driving guide line to obtain its decoupled
states in Frenet frame. (s, ˙s, ¨s) represents the vehicle state, i.e., position,
velocity and acceleration, along the guide line (i.e., longitudinal state) and
(d,
˙
d,
¨
d) represents the vehicle state, i.e., position, velocity and acceleration,
perpendicular to the guild line (i.e., lateral state). Then, plan longitudinal
and lateral motions independently. Finally, longitudinal and lateral motions
in Frenet frame are combined and transformed to a trajectory in Cartesian
space.
function τ (t) that maps a time t to a specific configuration
(x, y, θ, κ).
Trajectory Planning in Frenet frame
Our method adopts a similar framework as in [12], which
utilizes the concept of Frenet frame for trajectory planning
(see Fig. 1). Given a smooth driving guide line, a Frenet
frame decouples the vehicle motions in Cartesian space into
two independent 1D movements, longitudinal movement that
moves along the guide line and lateral movement that moves
orthogonally to the guide line. Thus, a trajectory planning
problem in Cartesian space is transformed to two lower
dimensional and independent planning problems in Frenet
frame. This framework exploits the task domain that most
vehicles are moving along the lane, and it is particularly
advantageous as it greatly simplifies problem by reducing
the dimensionality of planning.
We assume that the autonomous vehicle is roughly driving
around the guild line. The main task for in lane driving is
to generate a trajectory for the autonomous vehicle driving
3620
剩余7页未读,继续阅读
资源评论
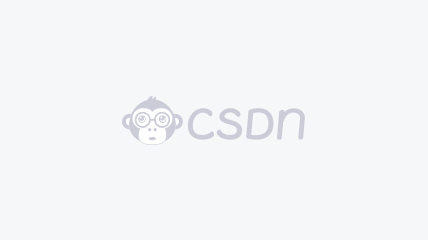

zhaoqi暮宿
- 粉丝: 0
- 资源: 5
上传资源 快速赚钱
我的内容管理 展开
我的资源 快来上传第一个资源
我的收益
登录查看自己的收益我的积分 登录查看自己的积分
我的C币 登录后查看C币余额
我的收藏
我的下载
下载帮助

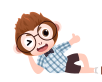
最新资源
- 基于Flask框架的Web外卖程序设计(python)
- 永磁同步电机PMSM仿真计算,本项目使用直接转矩控制和磁场定向控制FOC来测试模型的准确性和可行性,使用扩展卡尔曼滤波器来估计转子的速度和位置,以便减少在闭环中驱动电机所需的传感器的数量 仿真效果良
- comsol不同电压等级盆氏绝缘子电场分布和温度场分布,330kv、550kv绝缘子电热耦合,与文献内容对应,comsol电热耦合仿真
- 同步机(VSG)三相并网仿真模型 包括VSG有功无功环,电压电流双闭环,阻抗部分 仿真结果波形完美,该仿真主要用来基础原理的学习
- 机械设计液晶屏点胶后检测固化一体机sw20可编辑全套技术资料100%好用.zip
- 基于A*算法的路径规划 鼠标自由选择起始点终点 五种地图随意切, 附涵的代码注释
- Simulink仿真:三相光伏MPPT并网谐振 关键词:光伏电池 Matlab MPPT 并网 离网 参考文献:提前录制的详细讲解视频 仿真平台:MATLAB Simulink
- 基于FPGA的硬件电子琴设计(文档+程序)
- 三机九节点,含火力,水力,风机发电机,风机采用惯性控制 渗透率可调,可用于基础研究
- 西门子S7-200PLC程序和组态王4层电梯四层电梯带组态仿真组态设计PLC设计
- EDA技术中基于Quartus II的8位电子密码锁设计与仿真实践
- 多孔介质(随机生成),应力分析,孔隙渗流 1.孔隙率、孔径大小可调 2.并行重构,效率高 3.可导入ansys,comsol,abaqus等软件 4.固相和孔隙可导出数字模型、stl、stp等格式
- Python Pygame模块实现贪吃蛇游戏
- 三相VIENNA整流,维也纳整流器simulink仿真 输入电压220v有效值 输出电压800v纹波在1%以内 0.1s后系统稳定 功率因数>0.95 电流THD<5% 开关频率20k 图一为拓扑,可
- 机械设计在线自动扫码测试机sw21可编辑全套技术资料100%好用.zip
- All电视节目列表saving.txt
资源上传下载、课程学习等过程中有任何疑问或建议,欢迎提出宝贵意见哦~我们会及时处理!
点击此处反馈


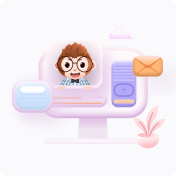
安全验证
文档复制为VIP权益,开通VIP直接复制
