import pandas as pd
import numpy as np
import matplotlib.pyplot as plt
from statsmodels.tsa.arima.model import ARIMA
from sklearn.metrics import mean_squared_error
# 假设你有一个时间序列数据,存储在DataFrame的'value'列中
# 例如,这里我们生成一个示例时间序列数据
np.random.seed(0)
date_rng = pd.date_range(start='1/1/2020', end='1/01/2023', freq='D')
df = pd.DataFrame(date_rng, columns=['date'])
df['value'] = np.random.randn(len(df)).cumsum() # 累积和生成一个趋势时间序列
df.set_index('date', inplace=True)
# 拆分训练集和测试集
train_size = int(len(df) * 0.8)
train, test = df[:train_size], df[train_size:]
# 拟合ARIMA模型
# 这里我们假设p=1, d=1, q=1,但在实际应用中你应该根据ACF和PACF图来确定这些参数
model = ARIMA(train, order=(1, 1, 1))
model_fit = model.fit()
# 打印模型摘要
print(model_fit.summary())
# 预测测试集
start_index = test.index[0]
end_index = test.index[-1]
predictions = model_fit.predict(start=start_index, end=end_index, typ='levels')
# 计算均方误差(MSE)
mse = mean_squared_error(test['value'], predictions)
print(f'Mean Squared Error: {mse}')
# 可视化结果
plt.figure(figsize=(12, 6))
plt.plot(train.index, train['value'], label='Train')
plt.plot(test.index, test['value'], label='Test')
plt.plot(test.index, predictions, label='Predictions', color='red')
plt.legend(loc='best')
plt.show()

图表制作解说(目标1000个图表)
- 粉丝: 1359
- 资源: 431
最新资源
- GEE错误集-Cannot add an object of type <Element> to the map. Might be fixable with an explicit .pdf
- 矩阵与线程的对应关系图
- 人体人员检测46-YOLO(v5至v9)、COCO、Darknet、TFRecord数据集合集.rar
- GEMM优化代码实现1
- 资料阅读器(先下载解压) 5.0.zip
- 人、垃圾、非垃圾检测18-YOLO(v5至v11)、COCO、CreateML、Paligemma、TFRecord、VOC数据集合集.rar
- java实现的冒泡排序 含代码说明和示例.docx
- 440379878861684smart-parking.zip
- 金智维RPA server安装包
- 二维码图形检测6-YOLO(v5至v9)、COCO、CreateML、Darknet、Paligemma、TFRecord数据集合集.rar
资源上传下载、课程学习等过程中有任何疑问或建议,欢迎提出宝贵意见哦~我们会及时处理!
点击此处反馈


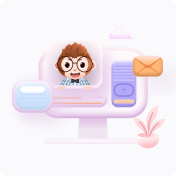