Introduction to Statistical Learning Theory
需积分: 10 8 浏览量
2017-05-27
16:49:02
上传
评论 1
收藏 265KB PDF 举报

边沿漫游者
- 粉丝: 11
- 资源: 49
最新资源
- 基于python实现的基于PyQt5和爬虫的小说阅读系统.zip
- 机械设计整经机上纱自动化sw20非常好的设计图纸100%好用.zip
- Screenshot_20240427_031602.jpg
- 网页PDF_2024年04月26日 23-46-14_QQ浏览器网页保存_QQ浏览器转格式(6).docx
- 直接插入排序,冒泡排序,直接选择排序.zip
- 在排序2的基础上,再次对快排进行优化,其次增加快排非递归,归并排序,归并排序非递归版.zip
- 实现了7种排序算法.三种复杂度排序.三种nlogn复杂度排序(堆排序,归并排序,快速排序)一种线性复杂度的排序.zip
- 冒泡排序 直接选择排序 直接插入排序 随机快速排序 归并排序 堆排序.zip
- 课设-内部排序算法比较 包括冒泡排序、直接插入排序、简单选择排序、快速排序、希尔排序、归并排序和堆排序.zip
- Python排序算法.zip
资源上传下载、课程学习等过程中有任何疑问或建议,欢迎提出宝贵意见哦~我们会及时处理!
点击此处反馈


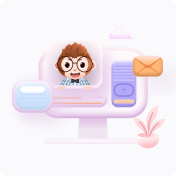