# CUDA-FastBEV
This repository contains sources and model for [Fast-BEV](https://github.com/Sense-GVT/Fast-BEV) inference using CUDA & TensorRT. And provide PTQ and QAT int8 quantization code.
## 3D Object Detection(on nuScenes validation set)
- For all models, I used the configs/fastbev_m0_r18_s256x704_v200x200x4_c192_d2_f1.py configuration, delete time sequence.
- The camera resolution is 256x704
- Backbone is ResNet18, without DCN
| **Model** | **Framework** | **Precision** | **mAP** | **FPS** |
|:------------------------:|:-------------:|:-------------:|:-------:|:-------:|
| ResNet18 | TensorRT | FP16 | 24.3 | 113.6(on RTX2080Ti) |
| ResNet18-PTQ | TensorRT | FP16+INT8 | 23.89 | 143.8 |
| ResNet18-head-PTQ | TensorRT | FP16 + INT8 | 23.83 | 144.9 |
## Model and Data
- For quick practice, I provide an example data of nuScenes.You can download from [Google Drive](https://drive.google.com/file/d/14-fqrtbC5ZOWgkNA0m7jA7bQAySrzLrm/view?usp=sharing) It contains the following:
1. Camera images in 6 directions.
2. Transformation matrix of camera/lidar and vtrans need data.
- All models (model.zip) can be downloaded from [Google Drive](https://drive.google.com/file/d/1wwwckM0vux5ub3U4R_zS9pm01QFmMPru/view?usp=sharing) .It contains the following:
1. fastbev onnx models and pytorch models.
2. resnet18 int8 onnx and PTQ models.
## Prerequisites
To build fastbev, we need to depend on the following libraries:
- CUDA >= 11.0
- CUDNN >= 8.2
- TensorRT >= 8.5.0
- libprotobuf-dev == 3.6.1
- [Compute Capability](https://developer.nvidia.com/cuda-gpus#compute) >= sm_80
- Python >= 3.6
The data in the performance table was obtained by us on the Nvidia Orin platform, using TensorRT-8.6, cuda-11.4 and cudnn8.6 statistics.
## Quick Start for Inference
### 1. Download models and datas to CUDA-FastBEV directory
- download model.zip
- download nuScenes-example-data.zip
```bash
# download models and datas to CUDA-FastBEV
cd CUDA-FastBEV
# unzip models and datas
unzip model.zip
unzip nuScenes-example-data.zip
# here is the directory structure after unzipping
CUDA-FastBEV
|-- example-data
|-- 0-FRONT.jpg
|-- 1-FRONT_RIGHT.jpg
|-- ...
|-- example-data.pth
|-- x.tensor
|-- y.tensor
`-- valid_c_idx.tensor
|-- src
|-- ptq
|-- model
|-- resnet18int8
| |-- fastbev_pre_trt.onnx
| |-- fastbev_post_trt_decode.onnx
| |-- fastbev_ptq.pth
|-- resnet18
`-- resnet18int8head
`-- tool
```
### 2. Configure the environment.sh
- Install python dependency libraries
```bash
apt install libprotobuf-dev
pip install onnx
```
- Modify the TensorRT/CUDA/CUDNN/fastbev variable values in the tool/environment.sh file.
```bash
# change the path to the directory you are currently using
export TensorRT_Lib=/path/to/TensorRT/lib
export TensorRT_Inc=/path/to/TensorRT/include
export TensorRT_Bin=/path/to/TensorRT/bin
export CUDA_Lib=/path/to/cuda/lib64
export CUDA_Inc=/path/to/cuda/include
export CUDA_Bin=/path/to/cuda/bin
export CUDA_HOME=/path/to/cuda
export CUDNN_Lib=/path/to/cudnn/lib
# resnet18/resnet18int8/resnet18int8head
export DEBUG_MODEL=resnet18int8
# fp16/int8
export DEBUG_PRECISION=int8
export DEBUG_DATA=example-data
export USE_Python=OFF
```
- Apply the environment to the current terminal.
```bash
. tool/environment.sh
```
### 3. Compile and run
1. Building the models for tensorRT
```bash
bash tool/build_trt_engine.sh
```
2. Compile and run the program
```bash
bash tool/run.sh
```
## PTQ and export ONNX
- PTQ
```bash
python ptq_bev.py
```
- QAT
```bash
python qat_bev.py
```
- export ONNX
```bash
python export_onnx.py
```
## DEMO show



__AtYou__
- 粉丝: 3277
- 资源: 1555
最新资源
- 2005-2021年国自然管理学部标书80+份及历年立项清单.zip
- 基于ESP32实现的贪吃蛇游戏
- 细胞分割-基于UNet-2D实现的医疗细胞分割算法-附模型下载+项目源码-优质项目分享.zip
- 无人机自主导航-基于强化学习实现的无人机自主导航-附项目源码-优质项目实战.zip
- Java项目-基于springboot框架的企业客户信息反馈系统项目实战(附源码+文档)
- 无人机着陆-无人机自主着陆系统ROS包实现-附项目源码+动画演示-优质项目实战.zip
- C语言入门:基础知识解析与实战案例
- 点云技术在多个领域的应用案例解析
- 无人机三维轨迹预测-基于Matlab实现的改进粒子滤波的无人机三维航迹预测算法-附项目源码-优质项目实战.zip
- 嵌入式Linux C应用编程指南V1.4
资源上传下载、课程学习等过程中有任何疑问或建议,欢迎提出宝贵意见哦~我们会及时处理!
点击此处反馈


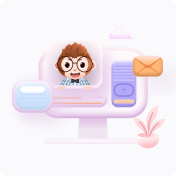