# A C++ implementation of Yolov5 to detect head or helmet in the wild in Jetson Xavier nx and Jetson nano
This repository uses yolov5 to detect humnan heads and helmets which can run in Jetson Xavier nx and Jetson nano.
In Jetson Xavier Nx, it can achieve 33 FPS.
<img src="assets/helmet.gif" >
## Requirement
1. Jetson nano or Jetson Xavier nx
2. Jetpack 4.5.1
3. python3 with default(jetson nano or jetson xavier nx has default python3 with tensorrt 7.1.3.0 )
4. tensorrt 7.1.3.0
5. torch 1.8.0
6. torchvision 0.9.0
7. torch2trt 0.3.0
8. onnx 1.4.1
9. opencv-python 4.5.3.56
10. protobuf 3.17.3
11. scipy 1.5.4
## Achieve and Experiment
- Int8.
- yolov5-s
- yolov5-m
## Comming soon
- [ ] Faster and use less memory.
## Speed
Whole process time from read image to finish process (include every img preprocess and postprocess). And all results can get in Jetson Xavier nx.
| Backbone | before TensorRT |TensortRT(detection)| FPS(detection) |
| :--------------: | :--------------: | :--------------: |:--------------:|
| Yolov5s-640-float16 | 100ms |60-70ms | 14 ~ 18 |
| Yolov5m-640-float16 | 120ms |70-75ms | 13 ~ 14 |
| Yolov5s-640-int8 | |30-40ms | 25 ~ 33 |
| Yolov5m-640-int8 | |50-60ms | 16 ~ 20 |
------
## Build and Run
```shell
cd 项目
mkdir build
cmake ..
make
```
## Model
You need is yolov5 model, for detection, generating from [tensorrtx](https://github.com/wang-xinyu/tensorrtx). You should generate the model the same way.
### Generate yolov5 model
For yolov5 detection model, I choose yolov5s, and choose `yolov5s.pt->yolov5s.wts->yolov5s.engine`
Note that, used models can get from [yolov5](https://github.com/ultralytics/yolov5) and if you need to use your own model, you can follow the `Run Your Custom Model`.
You can also see [tensorrtx official readme](https://github.com/wang-xinyu/tensorrtx/tree/master/yolov5)
1. Get yolov5 repository
Note that, here uses the official pertained model.And I use yolov5-5, v5.0. So if you train your own model, please be sure your yolov5 code is v5.0.
```shell
git clone -b v5.0 https://github.com/ultralytics/yolov5.git
cd yolov5
mkdir weights
cd weights
// download https://github.com/ultralytics/yolov5/releases/download/v5.0/yolov5s.pt
wget https://github.com/ultralytics/yolov5/releases/download/v5.0/yolov5s.pt
```
2. Get tensorrtx.
```shell
git clone https://github.com/wang-xinyu/tensorrtx
```
3. Get xxx.wst model
```shell
cp tensorrtx/gen_wts.py yolov5/
cd yolov5
python3 gen_wts.py -w ./weights/yolov5s.pt -o ./weights/yolov5s.wts
// a file 'yolov5s.wts' will be generated.
```
You can get yolov5s.wts model in `yolov5/weights/`
4. Build tensorrtx/yolov5 and get tensorrt engine
```shell
cd tensorrtx/yolov5
// update CLASS_NUM in yololayer.h if your model is trained on custom dataset
mkdir build
cd build
cp {ultralytics}/yolov5/yolov5s.wts {tensorrtx}/yolov5/build
cmake ..
make
// yolov5s
sudo ./yolov5 -s yolov5s.wts yolov5s.engine s
// test your engine file
sudo ./yolov5 -d yolov5s.engine ../samples
```
Then you get the yolov5s.engine, and you can put `yolov5s.engine` in My project. For example
```shell
cd {yolov5-helmet-detection}
mkdir resources
cp {tensorrtx}/yolov5/build/yolov5s.engine {yolov5-helmet-detection}/resources
```
After all 4 step, you can get the yolov5s.engine .
You may face some problems in getting yolov5s.engine, you can upload your issue in github or [csdn artical](https://blog.csdn.net/weixin_42264234/article/details/121241573).
<details>
<summary>Different versions of yolov5</summary>
Currently, tensorrt support yolov5 v1.0(yolov5s only), v2.0, v3.0, v3.1, v4.0 and v5.0.
- For yolov5 v5.0, download .pt from [yolov5 release v5.0](https://github.com/ultralytics/yolov5/releases/tag/v5.0), `git clone -b v5.0 https://github.com/ultralytics/yolov5.git` and `git clone https://github.com/wang-xinyu/tensorrtx.git`, then follow how-to-run in current page.
- For yolov5 v4.0, download .pt from [yolov5 release v4.0](https://github.com/ultralytics/yolov5/releases/tag/v4.0), `git clone -b v4.0 https://github.com/ultralytics/yolov5.git` and `git clone -b yolov5-v4.0 https://github.com/wang-xinyu/tensorrtx.git`, then follow how-to-run in [tensorrtx/yolov5-v4.0](https://github.com/wang-xinyu/tensorrtx/tree/yolov5-v4.0/yolov5).
- For yolov5 v3.1, download .pt from [yolov5 release v3.1](https://github.com/ultralytics/yolov5/releases/tag/v3.1), `git clone -b v3.1 https://github.com/ultralytics/yolov5.git` and `git clone -b yolov5-v3.1 https://github.com/wang-xinyu/tensorrtx.git`, then follow how-to-run in [tensorrtx/yolov5-v3.1](https://github.com/wang-xinyu/tensorrtx/tree/yolov5-v3.1/yolov5).
- For yolov5 v3.0, download .pt from [yolov5 release v3.0](https://github.com/ultralytics/yolov5/releases/tag/v3.0), `git clone -b v3.0 https://github.com/ultralytics/yolov5.git` and `git clone -b yolov5-v3.0 https://github.com/wang-xinyu/tensorrtx.git`, then follow how-to-run in [tensorrtx/yolov5-v3.0](https://github.com/wang-xinyu/tensorrtx/tree/yolov5-v3.0/yolov5).
- For yolov5 v2.0, download .pt from [yolov5 release v2.0](https://github.com/ultralytics/yolov5/releases/tag/v2.0), `git clone -b v2.0 https://github.com/ultralytics/yolov5.git` and `git clone -b yolov5-v2.0 https://github.com/wang-xinyu/tensorrtx.git`, then follow how-to-run in [tensorrtx/yolov5-v2.0](https://github.com/wang-xinyu/tensorrtx/tree/yolov5-v2.0/yolov5).
- For yolov5 v1.0, download .pt from [yolov5 release v1.0](https://github.com/ultralytics/yolov5/releases/tag/v1.0), `git clone -b v1.0 https://github.com/ultralytics/yolov5.git` and `git clone -b yolov5-v1.0 https://github.com/wang-xinyu/tensorrtx.git`, then follow how-to-run in [tensorrtx/yolov5-v1.0](https://github.com/wang-xinyu/tensorrtx/tree/yolov5-v1.0/yolov5).
</details>
<details>
<summary>Config</summary>
- Choose the model s/m/l/x/s6/m6/l6/x6 from command line arguments.
- Input shape defined in yololayer.h
- Number of classes defined in yololayer.h, **DO NOT FORGET TO ADAPT THIS, If using your own model**
- INT8/FP16/FP32 can be selected by the macro in yolov5.cpp, **INT8 need more steps, pls follow `How to Run` first and then go the `INT8 Quantization` below**
- GPU id can be selected by the macro in yolov5.cpp
- NMS thresh in yolov5.cpp
- BBox confidence thresh in yolov5.cpp
- Batch size in yolov5.cpp
</details>
## Run Your Custom Model
You may need train your own model and transfer your trained-model to tensorRT. So you can follow the following steps.
1. Train Custom Model
You can follow the [official wiki](https://github.com/ultralytics/yolov5/wiki/Train-Custom-Data
) to train your own model on your dataset. For example, I choose yolov5-s to train my model.
2. Transfer Custom Model
Just like [tensorRT official guideline](https://github.com/wang-xinyu/tensorrtx/edit/master/yolov5/).When your follow ` Generate yolov5 model` to get yolov5 and tensorrt rep, next step is to transfer your pytorch model to tensorrt.
Before this, you need to change yololayer.h file 20,21 and 22 line(CLASS_NUM,INPUT_H,INPUT_W) to your own parameters.
```shell
// before
static constexpr int CLASS_NUM = 80; // 20
static constexpr int INPUT_H = 640; // 21 yolov5's input height and width must be divisible by 32.
static constexpr int INPUT_W = 640; // 22
// after
// if your model is 2 classfication and image size is 416*416
static constexpr int CLASS_NUM = 2; // 20
static constexpr int INPUT_H = 416; // 21 yolov5's input height and width must be divisible by 32.
static constexpr int INPUT_W = 416; // 22
```
```shell
cd {tensorrtx}/yolov5/
// update CLASS_NUM in yololayer.h if your model is trained on custom dataset
mkdir build
cd build
cp {ultralytics}/yolov5/yolov5s.wts {tensorrtx}/yolov5/build
cmake ..
make
sudo ./yolov5 -s [.wts] [.engine] [s/m/l/
没有合适的资源?快使用搜索试试~ 我知道了~
资源推荐
资源详情
资源评论
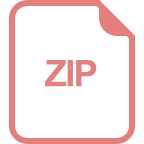
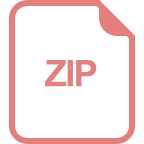
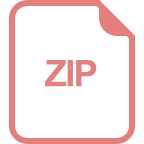
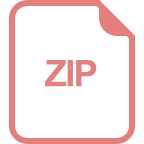
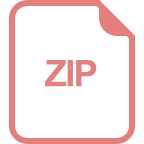
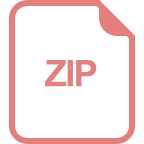
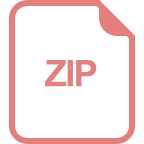
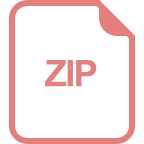
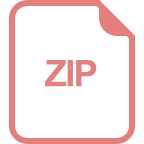
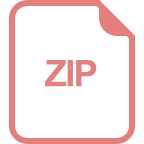
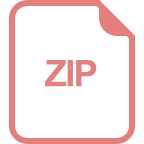
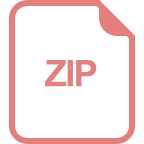
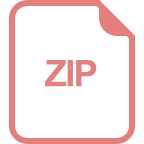
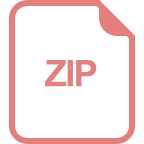
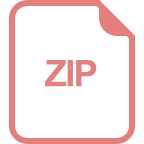
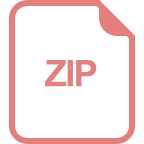
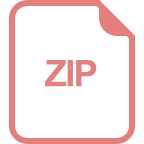
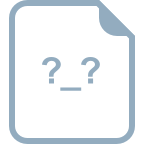
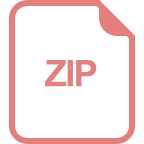
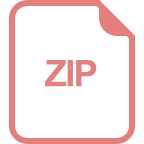
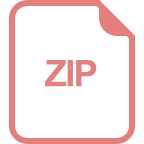
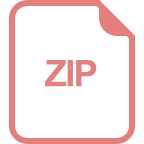
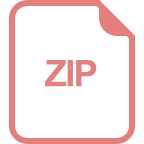
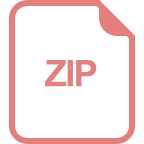
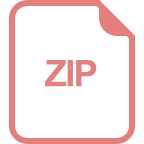
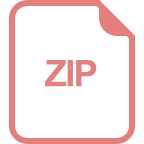
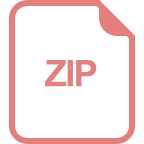
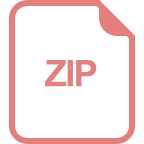
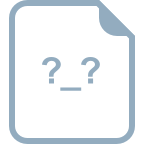
收起资源包目录



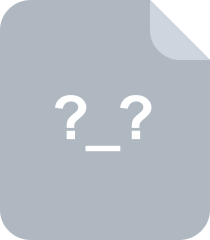
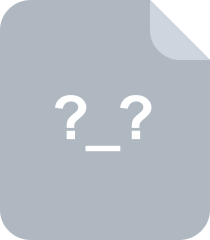
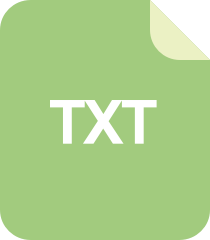

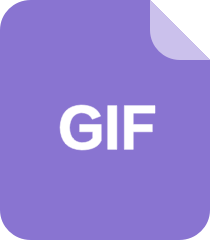

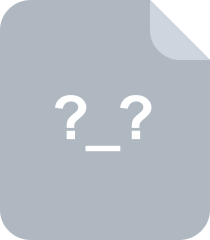

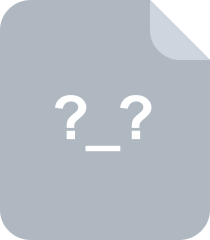
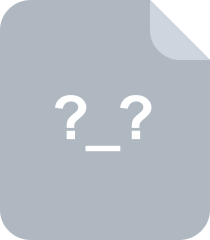
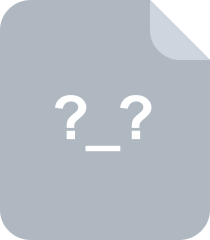


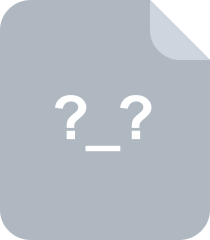
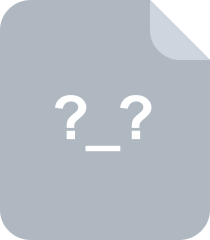
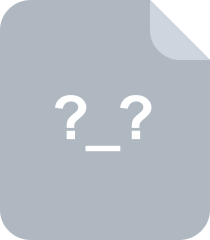
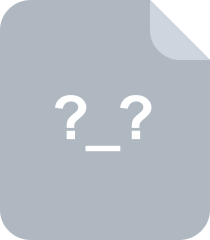
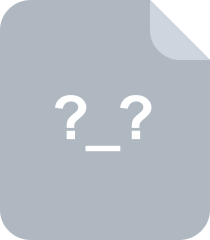
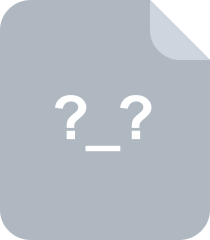
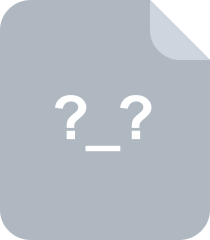
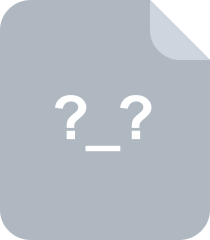
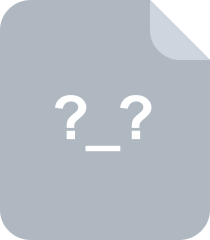

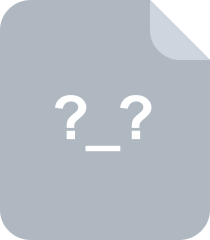
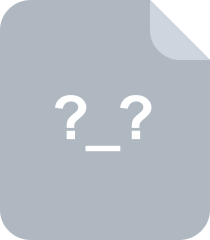
共 19 条
- 1
资源评论
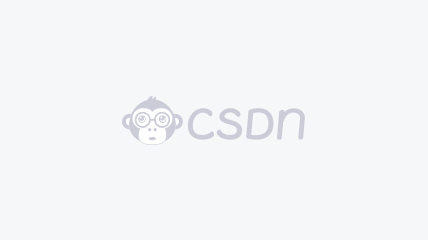

__AtYou__
- 粉丝: 3276
- 资源: 1555
上传资源 快速赚钱
我的内容管理 展开
我的资源 快来上传第一个资源
我的收益
登录查看自己的收益我的积分 登录查看自己的积分
我的C币 登录后查看C币余额
我的收藏
我的下载
下载帮助

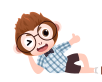
最新资源
资源上传下载、课程学习等过程中有任何疑问或建议,欢迎提出宝贵意见哦~我们会及时处理!
点击此处反馈


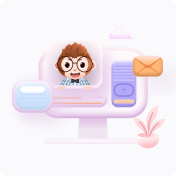
安全验证
文档复制为VIP权益,开通VIP直接复制
