<a href="https://apps.apple.com/app/id1452689527" target="_blank">
<img src="https://user-images.githubusercontent.com/26833433/98699617-a1595a00-2377-11eb-8145-fc674eb9b1a7.jpg" width="1000"></a>
 

This repository represents Ultralytics open-source research into future object detection methods, and incorporates our lessons learned and best practices evolved over training thousands of models on custom client datasets with our previous YOLO repository https://github.com/ultralytics/yolov3. **All code and models are under active development, and are subject to modification or deletion without notice.** Use at your own risk.
<img src="https://user-images.githubusercontent.com/26833433/90187293-6773ba00-dd6e-11ea-8f90-cd94afc0427f.png" width="1000">** GPU Speed measures end-to-end time per image averaged over 5000 COCO val2017 images using a V100 GPU with batch size 32, and includes image preprocessing, PyTorch FP16 inference, postprocessing and NMS. EfficientDet data from [google/automl](https://github.com/google/automl) at batch size 8.
- **August 13, 2020**: [v3.0 release](https://github.com/ultralytics/yolov5/releases/tag/v3.0): nn.Hardswish() activations, data autodownload, native AMP.
- **July 23, 2020**: [v2.0 release](https://github.com/ultralytics/yolov5/releases/tag/v2.0): improved model definition, training and mAP.
- **June 22, 2020**: [PANet](https://arxiv.org/abs/1803.01534) updates: new heads, reduced parameters, improved speed and mAP [364fcfd](https://github.com/ultralytics/yolov5/commit/364fcfd7dba53f46edd4f04c037a039c0a287972).
- **June 19, 2020**: [FP16](https://pytorch.org/docs/stable/nn.html#torch.nn.Module.half) as new default for smaller checkpoints and faster inference [d4c6674](https://github.com/ultralytics/yolov5/commit/d4c6674c98e19df4c40e33a777610a18d1961145).
- **June 9, 2020**: [CSP](https://github.com/WongKinYiu/CrossStagePartialNetworks) updates: improved speed, size, and accuracy (credit to @WongKinYiu for CSP).
- **May 27, 2020**: Public release. YOLOv5 models are SOTA among all known YOLO implementations.
## Pretrained Checkpoints
| Model | AP<sup>val</sup> | AP<sup>test</sup> | AP<sub>50</sub> | Speed<sub>GPU</sub> | FPS<sub>GPU</sub> || params | GFLOPS |
|---------- |------ |------ |------ | -------- | ------| ------ |------ | :------: |
| [YOLOv5s](https://github.com/ultralytics/yolov5/releases) | 37.0 | 37.0 | 56.2 | **2.4ms** | **416** || 7.5M | 17.5
| [YOLOv5m](https://github.com/ultralytics/yolov5/releases) | 44.3 | 44.3 | 63.2 | 3.4ms | 294 || 21.8M | 52.3
| [YOLOv5l](https://github.com/ultralytics/yolov5/releases) | 47.7 | 47.7 | 66.5 | 4.4ms | 227 || 47.8M | 117.2
| [YOLOv5x](https://github.com/ultralytics/yolov5/releases) | **49.2** | **49.2** | **67.7** | 6.9ms | 145 || 89.0M | 221.5
| | | | | | || |
| [YOLOv5x](https://github.com/ultralytics/yolov5/releases) + TTA|**50.8**| **50.8** | **68.9** | 25.5ms | 39 || 89.0M | 801.0
** AP<sup>test</sup> denotes COCO [test-dev2017](http://cocodataset.org/#upload) server results, all other AP results denote val2017 accuracy.
** All AP numbers are for single-model single-scale without ensemble or TTA. **Reproduce mAP** by `python test.py --data coco.yaml --img 640 --conf 0.001 --iou 0.65`
** Speed<sub>GPU</sub> averaged over 5000 COCO val2017 images using a GCP [n1-standard-16](https://cloud.google.com/compute/docs/machine-types#n1_standard_machine_types) V100 instance, and includes image preprocessing, FP16 inference, postprocessing and NMS. NMS is 1-2ms/img. **Reproduce speed** by `python test.py --data coco.yaml --img 640 --conf 0.25 --iou 0.45`
** All checkpoints are trained to 300 epochs with default settings and hyperparameters (no autoaugmentation).
** Test Time Augmentation ([TTA](https://github.com/ultralytics/yolov5/issues/303)) runs at 3 image sizes. **Reproduce TTA** by `python test.py --data coco.yaml --img 832 --iou 0.65 --augment`
## Requirements
Python 3.8 or later with all [requirements.txt](https://github.com/ultralytics/yolov5/blob/master/requirements.txt) dependencies installed, including `torch>=1.7`. To install run:
```bash
$ pip install -r requirements.txt
```
## Tutorials
* [Train Custom Data](https://github.com/ultralytics/yolov5/wiki/Train-Custom-Data) ð RECOMMENDED
* [Weights & Biases Logging](https://github.com/ultralytics/yolov5/issues/1289) ð NEW
* [Multi-GPU Training](https://github.com/ultralytics/yolov5/issues/475)
* [PyTorch Hub](https://github.com/ultralytics/yolov5/issues/36) â NEW
* [ONNX and TorchScript Export](https://github.com/ultralytics/yolov5/issues/251)
* [Test-Time Augmentation (TTA)](https://github.com/ultralytics/yolov5/issues/303)
* [Model Ensembling](https://github.com/ultralytics/yolov5/issues/318)
* [Model Pruning/Sparsity](https://github.com/ultralytics/yolov5/issues/304)
* [Hyperparameter Evolution](https://github.com/ultralytics/yolov5/issues/607)
* [Transfer Learning with Frozen Layers](https://github.com/ultralytics/yolov5/issues/1314) â NEW
* [TensorRT Deployment](https://github.com/wang-xinyu/tensorrtx)
## Environments
YOLOv5 may be run in any of the following up-to-date verified environments (with all dependencies including [CUDA](https://developer.nvidia.com/cuda)/[CUDNN](https://developer.nvidia.com/cudnn), [Python](https://www.python.org/) and [PyTorch](https://pytorch.org/) preinstalled):
- **Google Colab Notebook** with free GPU: <a href="https://colab.research.google.com/github/ultralytics/yolov5/blob/master/tutorial.ipynb"><img src="https://colab.research.google.com/assets/colab-badge.svg" alt="Open In Colab"></a>
- **Kaggle Notebook** with free GPU: [https://www.kaggle.com/ultralytics/yolov5](https://www.kaggle.com/ultralytics/yolov5)
- **Google Cloud** Deep Learning VM. See [GCP Quickstart Guide](https://github.com/ultralytics/yolov5/wiki/GCP-Quickstart)
- **Docker Image** https://hub.docker.com/r/ultralytics/yolov5. See [Docker Quickstart Guide](https://github.com/ultralytics/yolov5/wiki/Docker-Quickstart) 
## Inference
detect.py runs inference on a variety of sources, downloading models automatically from the [latest YOLOv5 release](https://github.com/ultralytics/yolov5/releases) and saving results to `runs/detect`.
```bash
$ python detect.py --source 0 # webcam
file.jpg # image
file.mp4 # video
path/ # directory
path/*.jpg # glob
rtsp://170.93.143.139/rtplive/470011e600ef003a004ee33696235daa # rtsp stream
rtmp://192.168.1.105/live/test # rtmp stream
http://112.50.243.8/PLTV/88888888/224/3221225900/1.m3u8 # http stream
```
To run inference on example images in `data/images`:
```bash
$ python detect.py --source data/images --weights yolov5s.pt --conf 0.25
Namespace(agnostic_nms=False, augment=False, classes=None, conf_thres=0.25, device='', img_size=640, iou_thres=0.45, save_conf=False, save_dir='runs/detect', save_txt=False, source='data/images/', update=False, view_img=False, weights=['yolov5s.pt'])
Using torch 1.7.0+cu101 CUDA:0 (Tesla V100-SXM2-16GB, 16130MB)
Downloading https://github.com/ultralytics/yolov5/releases/download/v3.1/yolov5s.pt to yolov5s.pt... 100%|ââââââââââââââ| 14.5M/14.5M [00:00<00:00, 21.3MB/s]
Fusing layers...
Model Summary: 232 layers, 7459581 parameters, 0 gradients
image 1/2 data/images/bus.jpg: 640x480 4 persons, 1 buss, 1 skateboards, Done. (0.012s)
image 2/2 data/images/zidane.jpg: 384x640 2 persons, 2 ties, Done. (0.012s)
Results saved to runs/detect/exp
Done. (0.113s)
```
<img src="https://user-images.g
没有合适的资源?快使用搜索试试~ 我知道了~
资源推荐
资源详情
资源评论
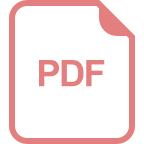
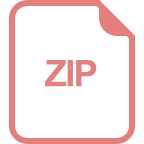
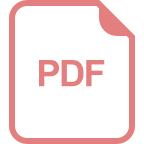
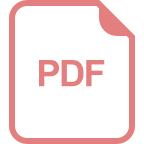
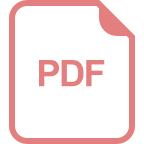
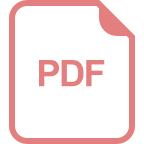
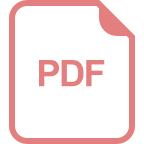
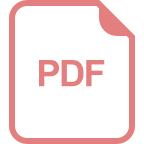
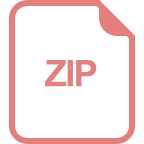
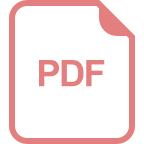
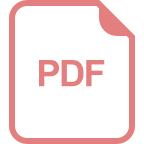
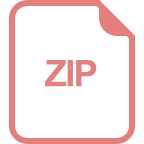
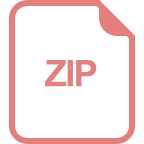
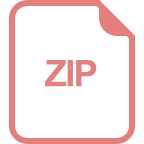
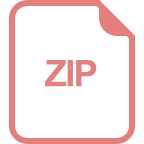
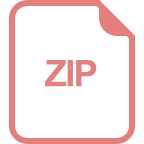
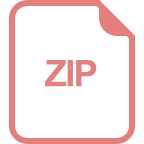
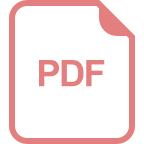
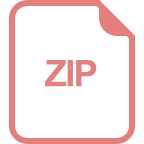
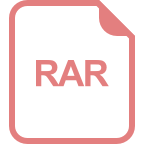
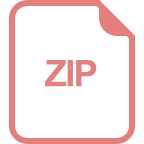
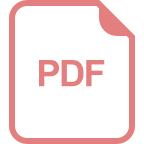
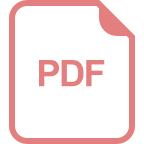
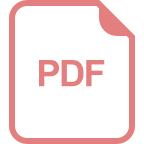
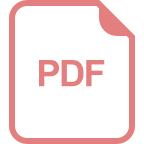
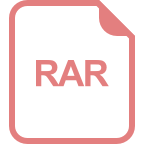
收起资源包目录

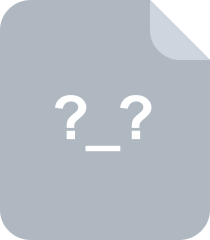
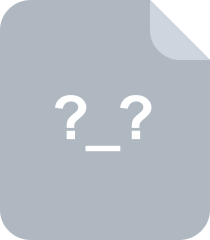
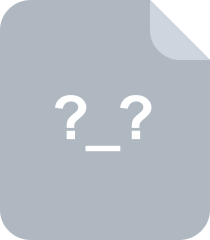
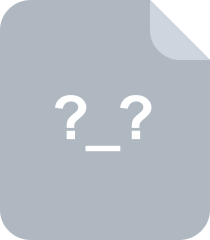
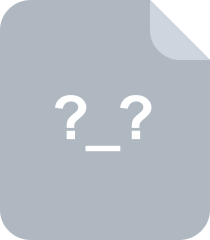
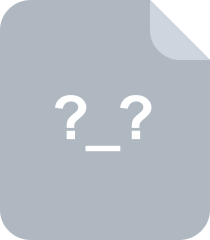
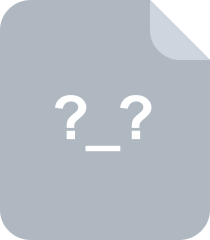
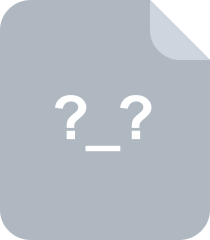
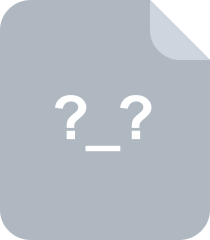
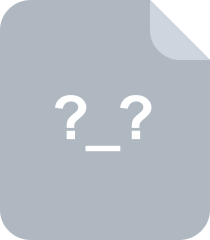
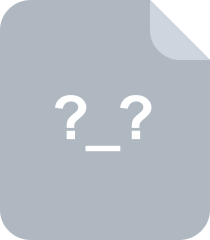
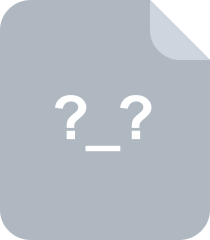
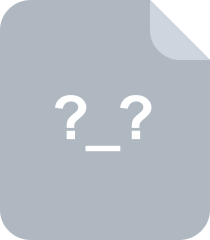
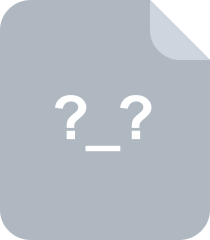
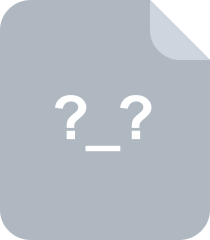
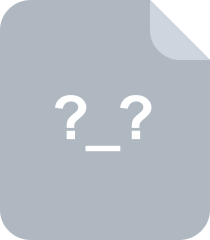
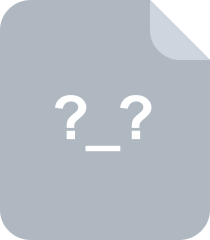
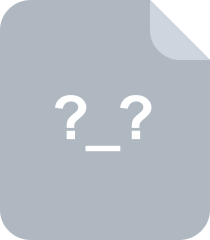
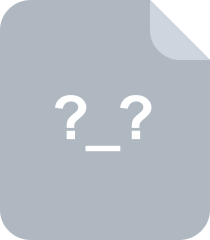
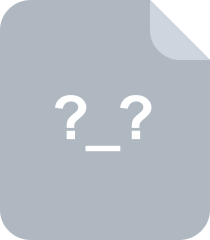
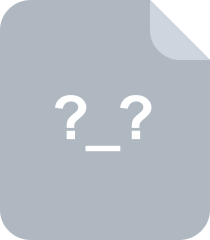
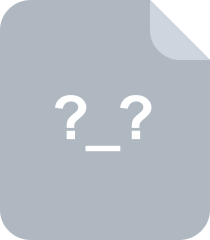
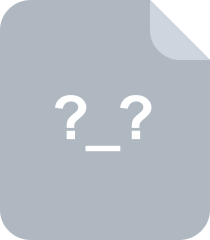
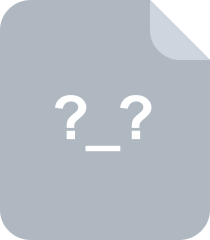
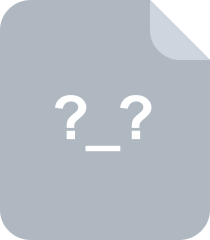
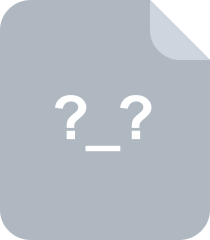
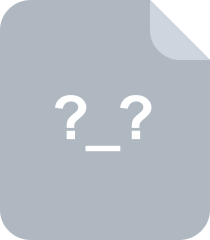
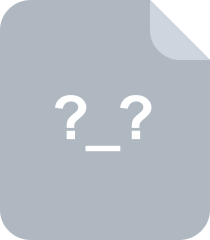
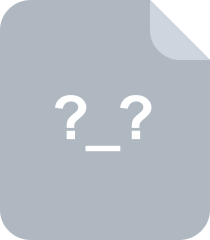
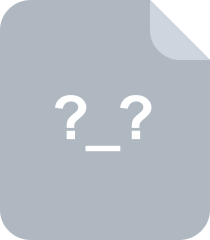
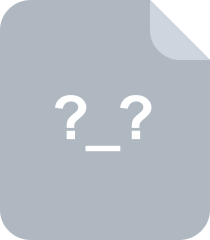
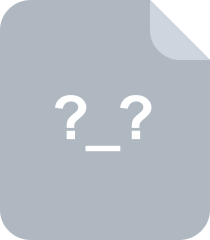
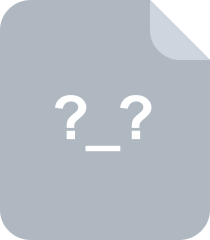
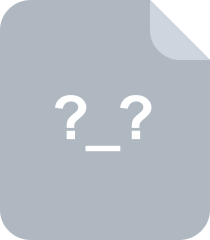
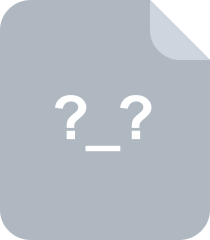
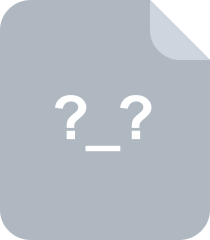
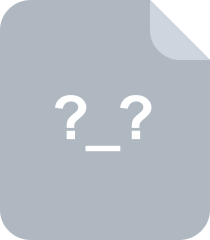
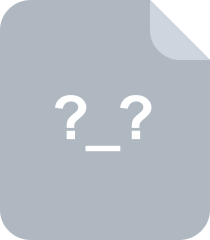
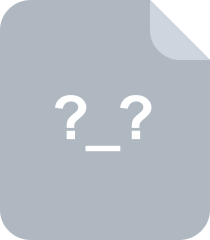
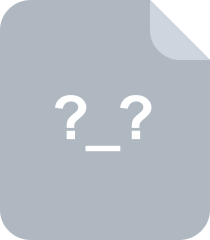
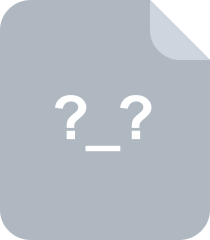
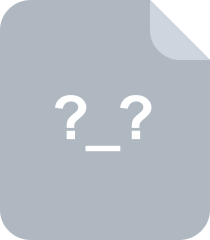
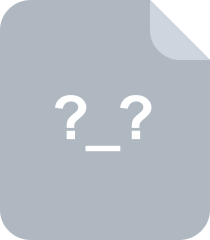
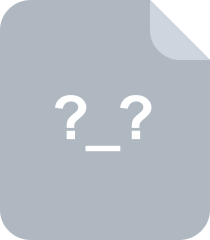
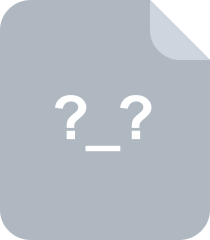
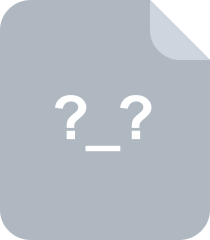
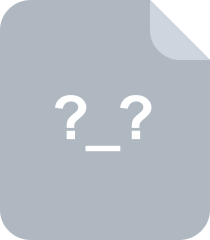
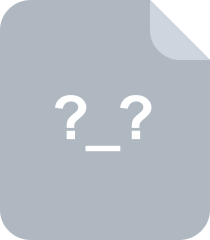
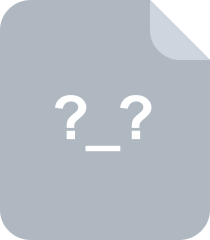
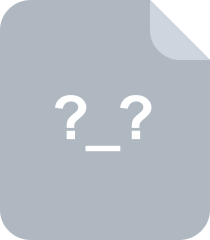
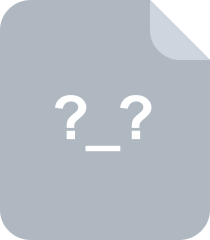
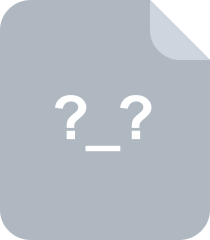
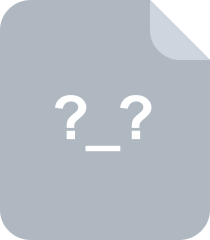
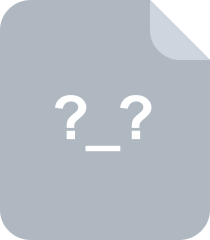
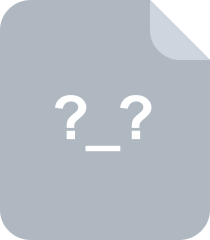
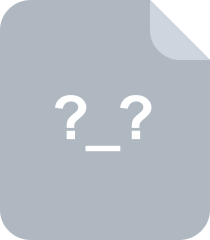
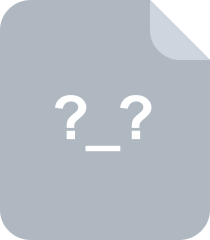
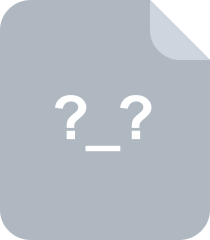
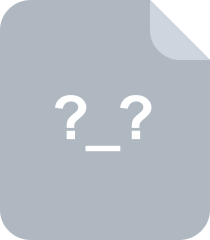
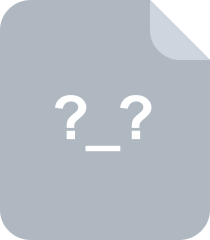
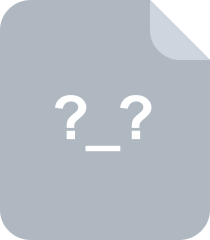
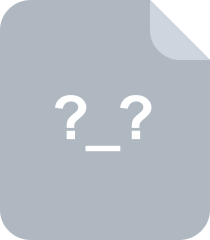
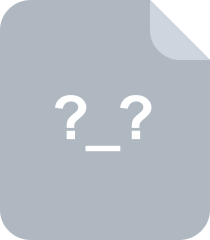
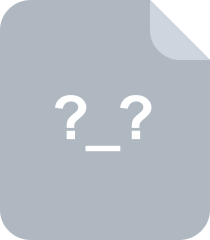
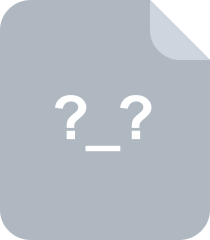
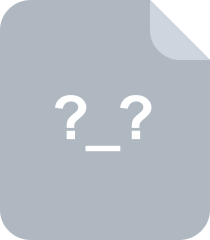
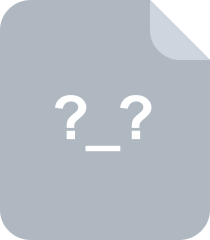
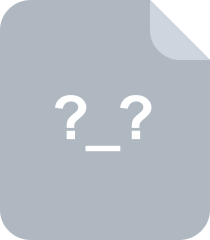
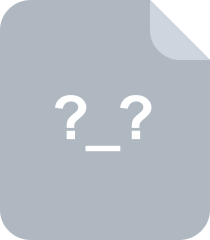
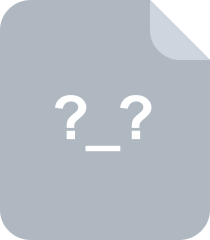
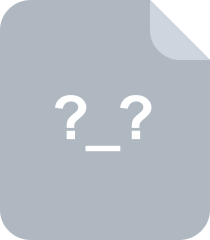
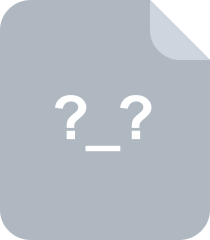
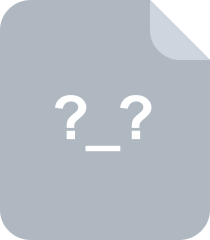
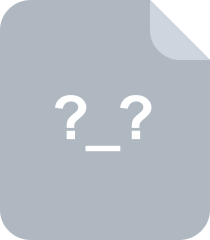
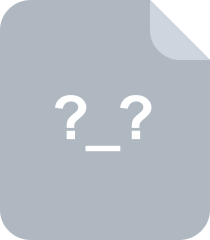
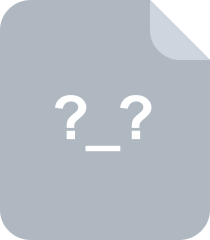
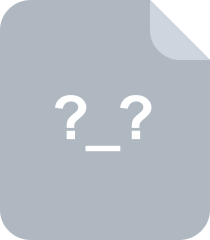
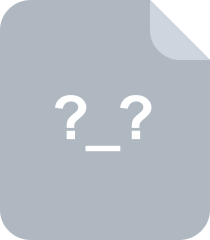
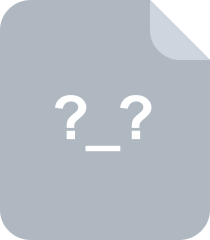
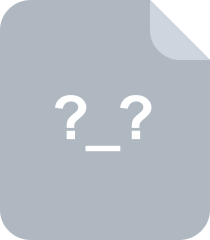
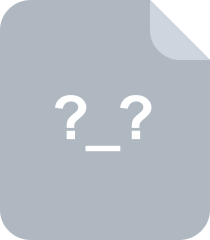
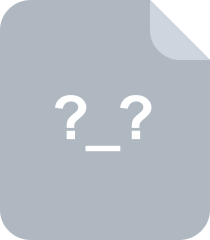
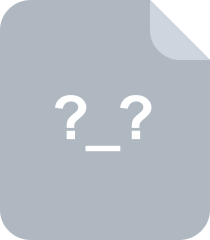
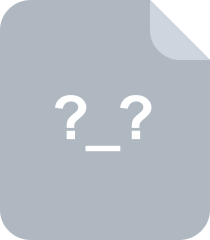
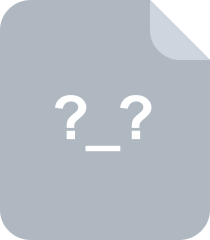
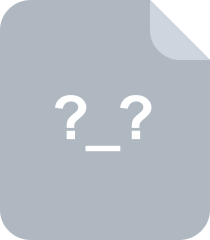
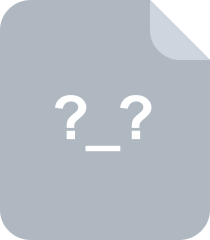
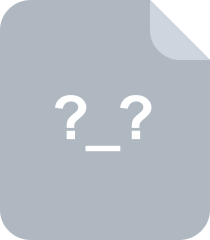
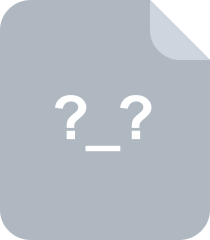
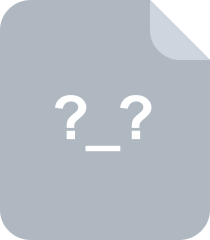
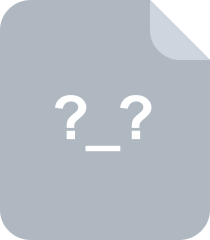
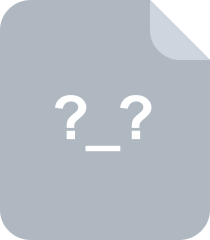
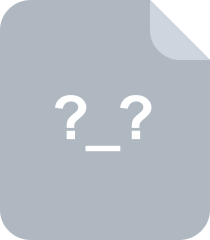
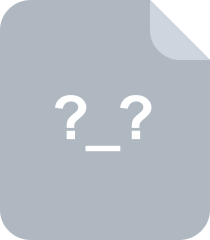
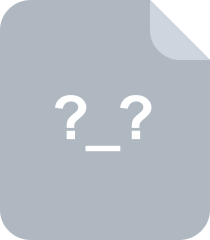
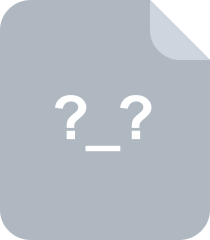
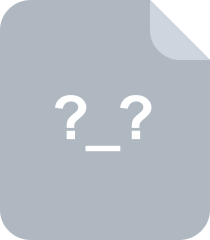
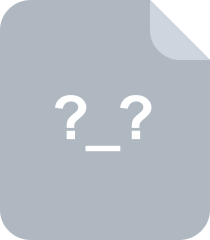
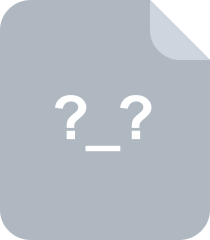
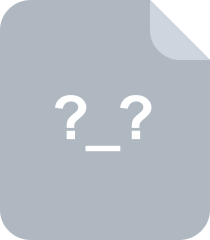
共 2000 条
- 1
- 2
- 3
- 4
- 5
- 6
- 20
资源评论
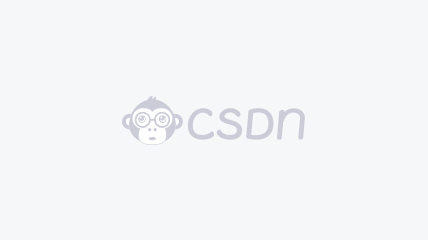

__AtYou__
- 粉丝: 3475
- 资源: 2146
上传资源 快速赚钱
我的内容管理 展开
我的资源 快来上传第一个资源
我的收益
登录查看自己的收益我的积分 登录查看自己的积分
我的C币 登录后查看C币余额
我的收藏
我的下载
下载帮助

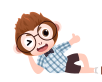
最新资源
- C183579-123578-c1235789.jpg
- Qt5.14 绘画板 Qt Creator C++项目
- python实现Excel表格合并
- Java实现读取Excel批量发送邮件.zip
- 【java毕业设计】商城后台管理系统源码(springboot+vue+mysql+说明文档).zip
- 【java毕业设计】开发停车位管理系统(调用百度地图API)源码(springboot+vue+mysql+说明文档).zip
- 星耀软件库(升级版).apk.1
- 基于Django后端和Vue前端的多语言购物车项目设计源码
- 基于Python与Vue的浮光在线教育平台源码设计
- 31129647070291Eclipson MXS R.zip
资源上传下载、课程学习等过程中有任何疑问或建议,欢迎提出宝贵意见哦~我们会及时处理!
点击此处反馈


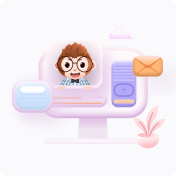
安全验证
文档复制为VIP权益,开通VIP直接复制
