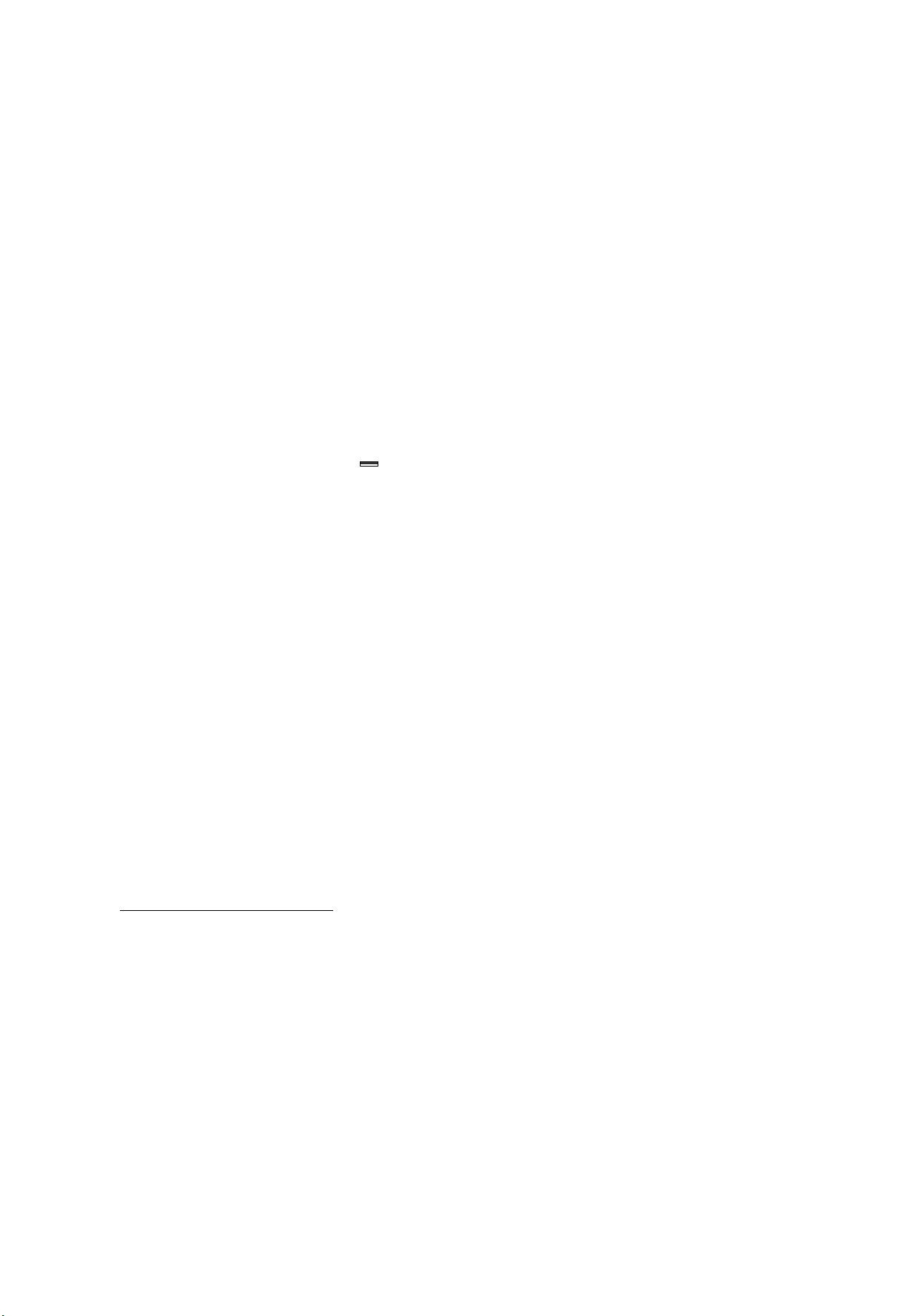
Problem Chosen 2020 Control Team Number
C
MCM/ICM
2015586
Summary
The Impact and Forecast of Reviews and Star Ratings
on Sales in Online Sales–Based on Markov Forecasting
Model and Text Emotional Analysis
This article mainly analyzes the impact of user reviews and star ratings on product sales in online
sales. Firstly, the correlation analysis model is used to analyze the quantified reviews with high
correlation with indicators such as stars. Furthermore, the review index was split and a stepwise
regression analysis model was used to analyze that the sentiment orientation index of the review
has the highest correlation with sales. Then we use this indicator to build the three sales conditions
required by the Markov forecasting model. After solving the model, we predict the change of sales
status in the next 5 years, and get the reviews and star atmosphere needed to achieve the best-selling
status. Finally, based on a known actual phenomenon, we use the data in the Markov model to
establish a low star-high sales reverse inference model. And the phenomenon is reversely derived to
be true, thereby increasing the accuracy of the method and making the model more Persuasive.
For question one, we conducted a more comprehensive analysis of star ratings and reviews, qual-
itatively and quantitatively explored these indicators, and analyzed the internal connections between
these indicators. First, we define two major indicators: star rating and review. The review indicator
is divided into three different indicators: the number of reviews, the hot words of the review, and
the importance of the review. The importance of the review is composed of the reviewer’s prestige,
the time span of the review, and the number of people who think the review is useful. Quantitative
calculations of stars and reviews are given by people giving weight to them. Then, using the obtained
data and bringing it into the univariate linear regression model, it is calculated that the correlation co-
efficient between the two indicators of reviews and star ratings is greater than 0.92 in the three types
of products. So we draw the conclusion that there is a high correlation between star indicators and
reviews
In response to question two, our overall goal is to analyze the atmosphere of star ratings and
reviews for three unlisted products based on available data, and to be able to obtain better sales strate-
gies. First, for the six indicators defined in the first question, such as the star rating, the importance
of the review, the number of reviews, and the hot words of the review, the time is introduced to estab-
lish time-related functions. At the same time, the hot words was renamed to comment emotionality.
Meanwhile, the negative vocabulary, positive vocabulary and functional vocabulary were assigned
respectively: -1, +1, +1 (see Appendix 1 for the dictionary), and we recalculate the emotional ten-
dencies of all reviews . Secondly, analyze the relationship between the above six important indicators
and sales volume, and use the stepwise regression analysis model to find out the factors that can most
affect sales volume. After analysis, we get that the factor is emotional. Then, we use the indicator
sentiment to define the sales status. Applying the Markov forecasting model, we predict the changes
in the sales status of each category of goods in the next 5 years. And we find the review atmosphere
needed to achieve the best-selling status, thereby achieving our goal.
In terms of model testing, we found a phenomenon based on common sense: a product can raise
disputes, increase attention, increase the number of reviews, and then achieve a significant increase
in sales. In order to verify this phenomenon, we set up a low star-high sales inverse inference model,
using the data in the Markov prediction model, to infer that the phenomenon is true. Thereby, it
further confirms the rationality and accuracy of the model.
For the model established in this question, we analyze its advantages and disadvantages. The
advantages are: the model is progressively layered and closely linked. It analyzes the internal rela-
tionship between reviews, star ratings and sales, and provides the predicted results. The indicators are
quantified and universal. With a validation model, the results are highly credible. The disadvantage is
that the data processing is more complicated, and there are more types of software used, which raises
the threshold for use.
Key Words: Markov Prediction Model, Stepwise Regression Analysis Model, Correlation anal-
ysis, Review sentiment analysis