# Fine-tune Convolutional Neural Network in Keras with ImageNet Pretrained Models
The reason to create this repo is that there are not many online resources that provide sample codes for performing fine-tuning, and that there is not a centralized place where we can easily download ImageNet pretrained models for common ConvNet architectures such as VGG, Inception, ResNet, and DenseNet. This repo serves to fill this gap by providing working examples of fine-tuning on Cifar10 dataset with ImageNet pretrained models on popular ConvNet implementations.
See [this](https://flyyufelix.github.io/2016/10/03/fine-tuning-in-keras-part1.html) for a comprehensive treatment of fine-tuning Deep Learning Models in Keras
## Usage
For illustration purpose, let's say you want to perform fine-tuning with VGG-16. First, download the ImageNet pretrained weights for VGG-16 to the `imagenet_models` directory. The schema and sample code for fine-tuning on Cifar10 can be found in `vgg16.py`. Run the file:
```
python vgg16.py
```
The code will automatically download Cifar10 dataset and performs fine-tuning with VGG-16. Please be aware that it might take some time (up to minutes) for the model to compile and load the ImageNet weights.
## Finetune with your own dataset
If you wish to perform fine-tuning on your own dataset, you have to replace the module which loads the Cifar10 dataset with your own load_data() module to load your own dataset.
```
X_train, Y_train, X_valid, Y_valid = load_data()
```
In particular, the following image preprocessing step have to be performed to get the format of the dataset compatible with the pretrained models:
```
# For Tensorflow
# Switch RGB to BGR order
x = x[:, :, :, ::-1]
# Subtract ImageNet mean pixel
x[:, :, :, 0] -= 103.939
x[:, :, :, 1] -= 116.779
x[:, :, :, 2] -= 123.68
# For Theano
# Switch RGB to BGR order
x = x[:, ::-1, :, :]
# Subtract ImageNet mean pixel
x[:, 0, :, :] -= 103.939
x[:, 1, :, :] -= 116.779
x[:, 2, :, :] -= 123.68
```
## ImageNet Pretrained Models
Network|Theano|Tensorflow
:---:|:---:|:---:
VGG-16 | [model (553 MB)](https://drive.google.com/file/d/0Bz7KyqmuGsilT0J5dmRCM0ROVHc/view?usp=sharing)| -
VGG-19 | [model (575 MB)](https://drive.google.com/file/d/0Bz7KyqmuGsilZ2RVeVhKY0FyRmc/view?usp=sharing)| -
GoogLeNet (Inception-V1) | [model (54 MB)](https://drive.google.com/open?id=0B319laiAPjU3RE1maU9MMlh2dnc)| -
Inception-V3 | [model (95 MB)](https://github.com/fchollet/deep-learning-models/releases/download/v0.2/inception_v3_weights_th_dim_ordering_th_kernels.h5)| -
Inception-V4 | [model (172 MB)](https://github.com/kentsommer/keras-inceptionV4/releases/download/2.0/inception-v4_weights_th_dim_ordering_th_kernels.h5)| [model (172 MB)](https://github.com/kentsommer/keras-inceptionV4/releases/download/2.0/inception-v4_weights_tf_dim_ordering_tf_kernels.h5)
ResNet-50 | [model (103 MB)](https://github.com/fchollet/deep-learning-models/releases/download/v0.2/resnet50_weights_th_dim_ordering_th_kernels.h5)| [model (103 MB)](https://github.com/fchollet/deep-learning-models/releases/download/v0.2/resnet50_weights_tf_dim_ordering_tf_kernels.h5)
ResNet-101 | [model (179 MB)](https://drive.google.com/file/d/0Byy2AcGyEVxfdUV1MHJhelpnSG8/view?usp=sharing)| [model (179 MB)](https://drive.google.com/file/d/0Byy2AcGyEVxfTmRRVmpGWDczaXM/view?usp=sharing)
ResNet-152 | [model (243 MB)](https://drive.google.com/file/d/0Byy2AcGyEVxfZHhUT3lWVWxRN28/view?usp=sharing)| [model (243 MB)](https://drive.google.com/file/d/0Byy2AcGyEVxfeXExMzNNOHpEODg/view?usp=sharing)
DenseNet-121 | [model (32 MB)](https://drive.google.com/open?id=0Byy2AcGyEVxfMlRYb3YzV210VzQ)| [model (32 MB)](https://drive.google.com/open?id=0Byy2AcGyEVxfSTA4SHJVOHNuTXc)
DenseNet-169 | [model (56 MB)](https://drive.google.com/open?id=0Byy2AcGyEVxfN0d3T1F1MXg0NlU)| [model (56 MB)](https://drive.google.com/open?id=0Byy2AcGyEVxfSEc5UC1ROUFJdmM)
DenseNet-161 | [model (112 MB)](https://drive.google.com/open?id=0Byy2AcGyEVxfVnlCMlBGTDR3RGs)| [model (112 MB)](https://drive.google.com/open?id=0Byy2AcGyEVxfUDZwVjU2cFNidTA)
## Requirements
* Keras ~~1.2.2~~ 2.0.5
* Theano 0.8.2 or TensorFlow ~~0.12.0~~ 1.2.1
## Updates
* Keras 2.0.5 and TensorFlow 1.2.1 are supported
没有合适的资源?快使用搜索试试~ 我知道了~
KonIQ-10k based image assessment
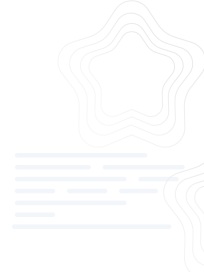
共29个文件
py:19个
ipynb:6个
md:2个

需积分: 5 0 下载量 176 浏览量
2024-11-22
13:30:54
上传
评论
收藏 449KB ZIP 举报
温馨提示
深度学习方法在图像质量评估(IQA)方面的应用受到现有数据集规模小的限制。构建大规模数据集需要大量的资源,既要生成可发布的内容丰富,又要进行准确的标注。一种系统化和可扩展的方法来创建迄今为止最大的IQA数据集KonIQ-10k,包含10,073张质量评分的图像。这是第一个面向野外环境的数据集,旨在实现生态有效性,关注失真的真实性、内容的多样性以及与质量相关的指标。通过众包的方式,从1,459名众包工作者那里获得了120万个可靠的质量评分,为更通用的IQA模型铺平了道路。一个新颖的深度学习模型(KonCept512),在测试集(0.921 SROCC)以及当前最先进的数据库LIVE-in-the-Wild(0.825 SROCC)上显示出了出色的泛化能力。该模型的核心性能来自于InceptionResNet架构,比以往的模型以更高的分辨率(512x384)进行训练。相关性分析显示,KonCept512的性能类似于每个测试图像拥有9个主观评分
资源推荐
资源详情
资源评论
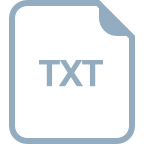
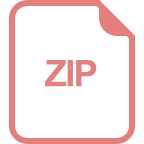
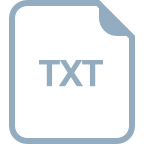
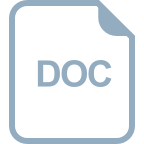
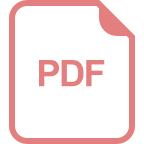
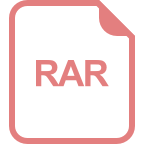
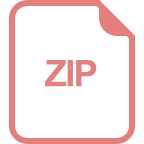
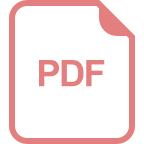
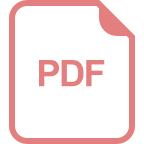
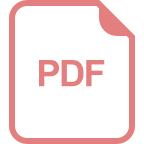
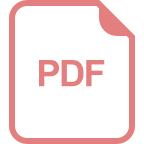
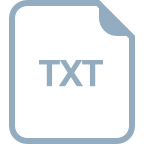
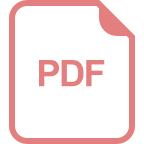
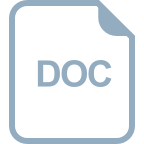
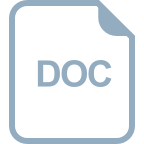
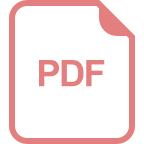
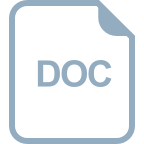
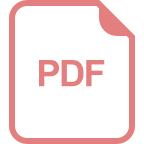
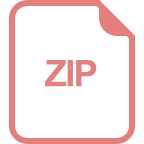
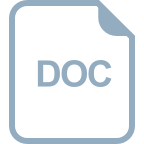
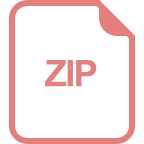
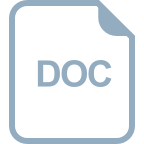
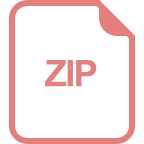
收起资源包目录



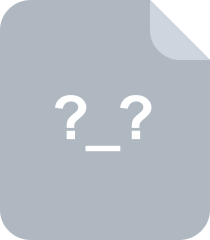
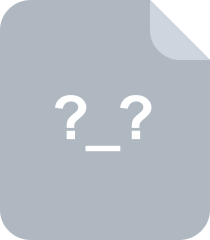
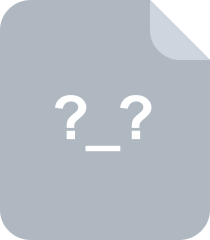
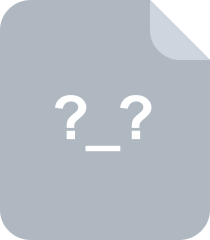
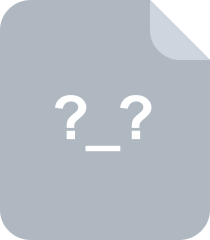

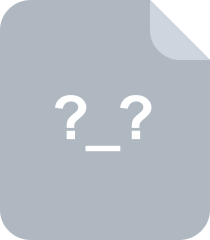

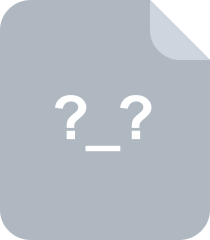
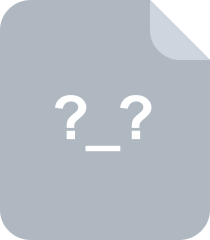

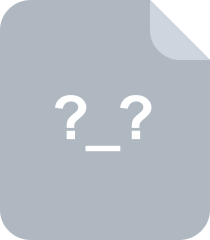
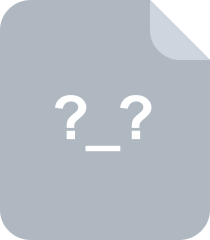
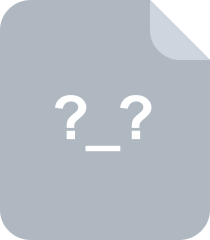
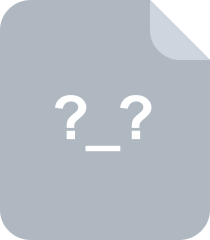
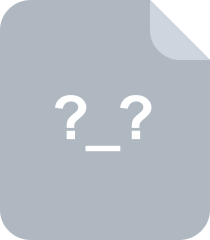
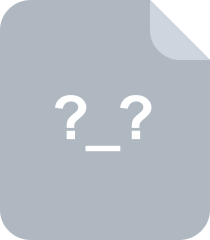
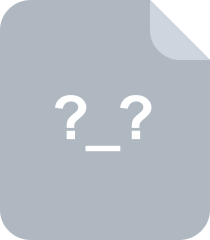
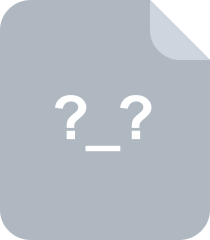
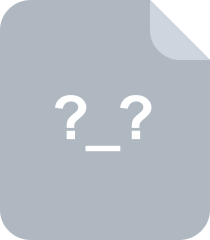
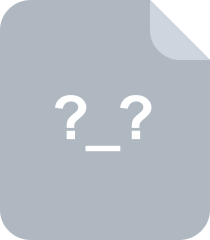
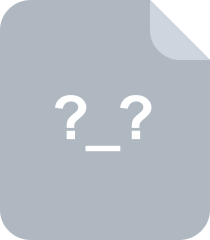
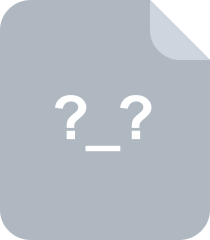
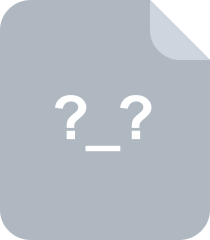

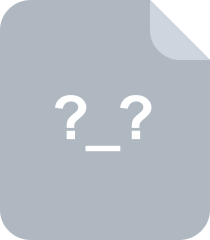
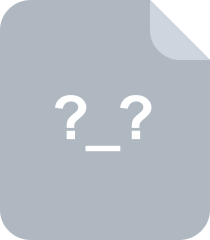
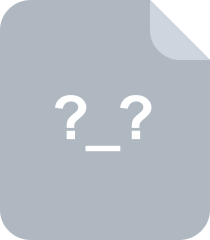
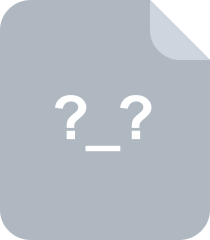
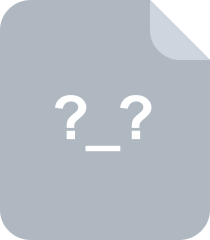
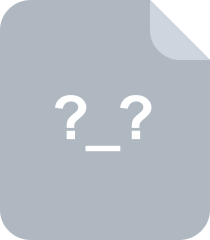
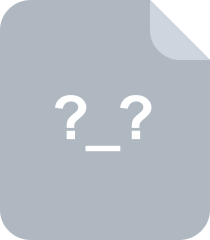
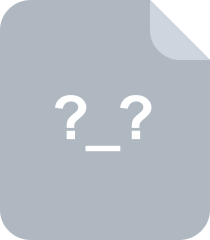
共 29 条
- 1
资源评论
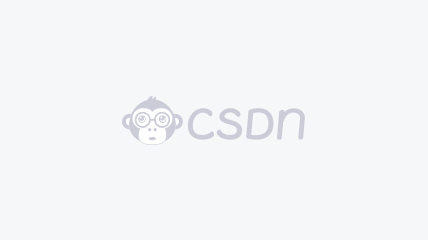

leaf_csdn
- 粉丝: 2108
- 资源: 13
上传资源 快速赚钱
我的内容管理 展开
我的资源 快来上传第一个资源
我的收益
登录查看自己的收益我的积分 登录查看自己的积分
我的C币 登录后查看C币余额
我的收藏
我的下载
下载帮助

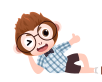
最新资源
资源上传下载、课程学习等过程中有任何疑问或建议,欢迎提出宝贵意见哦~我们会及时处理!
点击此处反馈


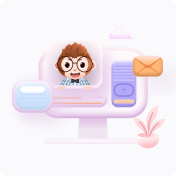
安全验证
文档复制为VIP权益,开通VIP直接复制
