% @file mvrvm.m
% Author Arasanathan Thayananthan ( at315@cam.ac.uk)
% (c) Copyright University of Cambridge
%
% This library is free software; you can redistribute it and/or
% modify it under the terms of the GNU Lesser General Public
% License as published by the Free Software Foundation; either
% version 2 of the License, or (at your option) any later version.
%
% This library is distributed in the hope that it will be useful,
% but WITHOUT ANY WARRANTY; without even the implied warranty of
% MERCHANTABILITY or FITNESS FOR A PARTICULAR PURPOSE. See the GNU
% Lesser General Public License for more details.
%
% You should have received a copy of the GNU Lesser General Public
% License along with this library; if not, write to the Free Software
% Foundation, Inc., 59 Temple Place, Suite 330, Boston, MA 02111-1307 USA
%This m file implements the bottom-up relevance vector machine with multivariate outputs, proposed
%in
% Multivariate Relevance Vector Machines for Tracking
% Arasanathan Thayananthan et al. (University of Cambridge)
% in Proc. 9th European Conference on Computer Vision 2006.
%Detailed derivation can be found in
%Arasanathan Thayananthan. Template-based pose estimation and tracking of 3 hand motion
%PhD thesis, University of Cambridge, UK 2005.
% output
% weights trained weights
% used index into column of PHI of relevant basis
% alpha final set of hyperparamters
% beta final values of noise precisions.
% input
% PHI basis function/design matrix
% t targetmatrix (multivariate)
% N number of training examples
% M number of basis functions
% [ N M] =size(PHI)
% P the number of output dimensions
% [ N P]= size(t)
% maxIts number of iterations
function [weights, used, alpha, beta, SIGMA_nz, N] = mvrvm(PHI,t,maxIts)
ALPHA_MAX = 1e12;
%initialise beta
epsilon = std(t) * 10/100;
beta=1./(epsilon.^2);
[N,M] = size(PHI);
[N,P] = size(t);
w = zeros(M,P);
PHIt = PHI'*t;
alpha = ones(M,1);
nonZero = logical(ones(M,1));
alpha(:)=ALPHA_MAX;
%choosing the first basis function with non-zero weight
max_change=-inf;
max_k=-1;
max_alpha=-inf;
for k=1:M
phi=PHI(:,k);
for j=1:P
S(j)=beta(j)*phi'*phi;
Q(j)=beta(j)*phi'*t(:,j);
end
if(alpha(k)<ALPHA_MAX)
s=alpha(k)*S./(alpha(k)-S);
q=alpha(k)*Q./(alpha(k)-S);
else
s=S;
q=Q;
end
[new_alpha,l_inc]=SolveForAlpha(s',q',S',Q',alpha(k));
l_inc_vec(k)=l_inc;
alpha_vec(k)=new_alpha;
if(max_change<l_inc)
max_k=k;
max_s=s;
max_q=q;
max_alpha=new_alpha;
max_change=l_inc;
end
end
alpha(max_k)=max_alpha;
%start the iterations
for i=1:maxIts
i;
nonZero = (alpha<ALPHA_MAX);
alpha_nz = alpha(nonZero);
w(~nonZero) = 0;
nz=size(alpha_nz,1);
PHI_nz = PHI(:,nonZero);
SIGMA_nz=zeros(nz,nz,P);
for j=1:P
SIGMA_nz(:,:,j) = inv((PHI_nz'*PHI_nz)*beta(j) + diag(alpha_nz));
end
%choose the basis function which minimises the marginal likelihood
max_change=-inf;
max_k=-1;
max_alpha=-inf;
for k=1:M
phi=PHI(:,k);
for j=1:P
S(j)=beta(j)*phi'*phi-beta(j)*beta(j)*phi'*PHI_nz*SIGMA_nz(:,:,j)*PHI_nz'*phi;
Q(j)=beta(j)*phi'*t(:,j) -beta(j)*beta(j)*phi'*PHI_nz*SIGMA_nz(:,:,j)*PHI_nz'*t(:,j);
end
if(alpha(k)<ALPHA_MAX)
s=alpha(k)*S./(alpha(k)-S);
q=alpha(k)*Q./(alpha(k)-S);
else
s=S;
q=Q;
end
[new_alpha,l_inc]=SolveForAlpha(s',q',S',Q',alpha(k));
l_inc_vec(k)=l_inc;
alpha_vec(k)=new_alpha;
if(max_change<l_inc)
max_k=k;
max_s=s;
max_q=q;
max_alpha=new_alpha;
max_change=l_inc;
end
end
alpha(max_k)=max_alpha;
if(nz~=0)
d=zeros(nz,1);
for j=1:P
d=d+diag(SIGMA_nz(:,:,j));
end
gamma=P-alpha_nz.*d;
for j=1:P
gamma=1-alpha_nz.*diag(SIGMA_nz(:,:,j));
weights=beta(j)*SIGMA_nz(:,:,j)*PHI_nz'*t(:,j);
ED=sum((t(:,j)-PHI_nz*weights).^2);
beta(j)=(N-sum(gamma))/ED;
end
end
end
weights=zeros(nz,P);
for j=1:P
weights(:,j)=beta(j)*SIGMA_nz(:,:,j)*PHI_nz'*t(:,j);
end
used = find(nonZero);
function [new_alpha,l_inc]=SolveForAlpha(s,q,S,Q,old_alpha)
ALPHA_MAX = 1e12;
[n m]=size(s);
index=[1:n]';
C=zeros(n,1);
CC=zeros(2*n-1,1);
for i=1:n
SS=-s(find(index~=i));
P=poly(SS)';
PP=conv(P,P);
CC=CC+q(i)*q(i)*PP;
C=C+P;
end
P=poly(-s);
PP=n*conv(P,P)';
CC0=conv(P,C)';
CC=[0
CC];
CCC=[CC+CC0
0];
r=roots(PP-CCC);
r=r(find(abs(imag(r))==0));
r=r(find(r>0));
r=[r
ALPHA_MAX];
[nn mm]=size(r);
L=zeros(nn,1);
for i=1:nn
new_alpha=r(i);
if((old_alpha>=ALPHA_MAX)& (r(i)<ALPHA_MAX))
tt(i)=1;
for j=1:n
L(i)=L(i)+ log(new_alpha/(new_alpha+s(j)))+((q(j)*q(j)./(new_alpha+s(j))));
end
elseif((old_alpha<ALPHA_MAX)&(new_alpha>=ALPHA_MAX))
tt(i)=2;
for j=1:n
L(i)=L(i)+ (Q(j)*Q(j)/(S(j)-old_alpha))-log(1- (S(j)/old_alpha));
end
elseif((old_alpha<ALPHA_MAX)&(new_alpha<ALPHA_MAX))
tt(i)=3;
for j=1:n
ad=(1/new_alpha)-(1/old_alpha);
L(i)=L(i)+ (Q(j)*Q(j)/(S(j)+(1/ad))) -log(1+S(j)*ad);
end
else
tt(i)=4;
L(i)=0;
end
end
[m ind]=max(L);
new_alpha=r(ind);
l_inc=L(ind);
没有合适的资源?快使用搜索试试~ 我知道了~
mvrvm.rar_MVRVM_relevance
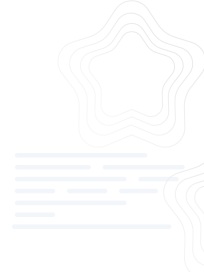
共1个文件
m:1个

1.该资源内容由用户上传,如若侵权请联系客服进行举报
2.虚拟产品一经售出概不退款(资源遇到问题,请及时私信上传者)
2.虚拟产品一经售出概不退款(资源遇到问题,请及时私信上传者)
版权申诉
0 下载量 156 浏览量
2022-07-15
03:57:56
上传
评论
收藏 2KB RAR 举报
温馨提示
This m file implements the bottom-up relevance vector machine with multivariate outputs, proposed in.
资源推荐
资源详情
资源评论
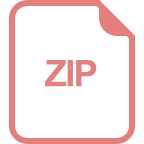
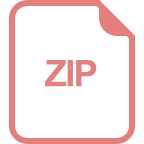
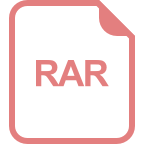
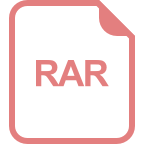
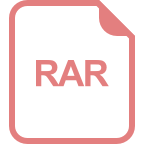
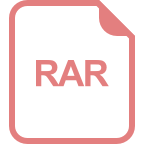
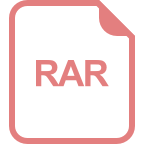
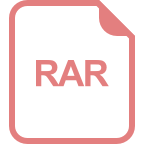
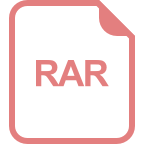
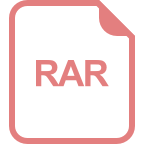
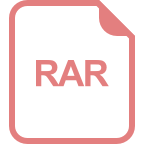
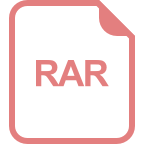
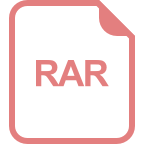
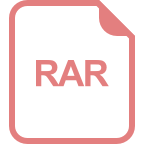
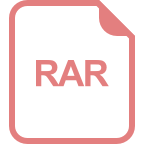
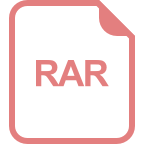
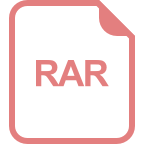
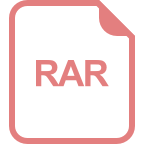
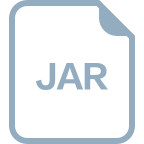
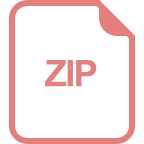
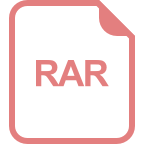
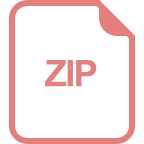
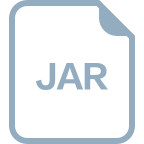
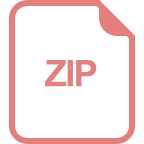
收起资源包目录

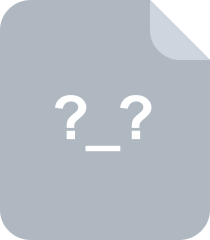
共 1 条
- 1
资源评论
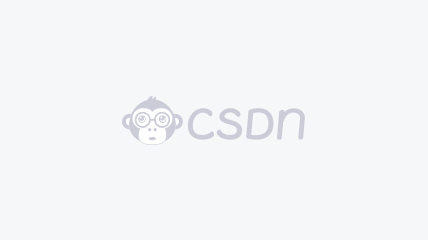

钱亚锋
- 粉丝: 106
- 资源: 1万+
上传资源 快速赚钱
我的内容管理 展开
我的资源 快来上传第一个资源
我的收益
登录查看自己的收益我的积分 登录查看自己的积分
我的C币 登录后查看C币余额
我的收藏
我的下载
下载帮助

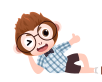
最新资源
资源上传下载、课程学习等过程中有任何疑问或建议,欢迎提出宝贵意见哦~我们会及时处理!
点击此处反馈


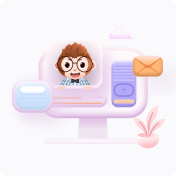
安全验证
文档复制为VIP权益,开通VIP直接复制
