# Holt-Winters Forecasting
# Table of Contents
- [Holt-Winters Forecasting](#holt-winters-forecasting)
- [Table of Contents](#table-of-contents)
- [Introduction](#introduction)
- [Mathematical Overview](#mathematical-overview)
- [Time Series](#time-series)
- [Aspects of Time Series](#aspects-of-time-series)
- [Exponential Smoothing](#exponential-smoothing)
- [Why exponential smoothing?](#why-exponential-smoothing)
- [Formula](#formula)
- [Holt's Model](#holts-model)
- [Drawbacks of SES](#drawbacks-of-ses)
- [What is Holt's Model?](#what-is-holts-model)
- [Formula](#formula-1)
- [Comparison with Linear Regression](#comparison-with-linear-regression)
- [Holt-Winter Model](#holt-winter-model)
- [Additive Model](#additive-model)
- [Formula](#formula-2)
- [Multiplicative Model](#multiplicative-model)
- [Formula](#formula-3)
- [Anomaly Detection using Brutlag algorithm](#anomaly-detection-using-brutlag-algorithm)
- [What is Brutlag algorithm?](#what-is-brutlag-algorithm)
- [Formula](#formula-4)
- [References](#references)
- [Textbooks](#textbooks)
- [Other](#other)
- [Getting Started](#getting-started)
- [Installation guide](#installation-guide)
- [Jupyter Notebook](#jupyter-notebook)
- [Python Script](#python-script)
- [Generating the analysis](#generating-the-analysis)
- [Anomaly Detection](#anomaly-detection)
- [Author](#author)
# Introduction
Holt-Winters forecasting is a way to model and predict the behavior of a sequence of values over time—a time series.
# Mathematical Overview
Before getting into the analysis of the real-time series model let's understand a few basic concepts required to have a deeper understanding of the topic.
## Time Series
A time series is a sequence of numerical data points in successive and chronological order. Generally, the x-axis or index is taken as time and the y-axis or value represents the value for the corresponding x value.
### Aspects of Time Series
1. Level - the typical value or the average
2. Trend - the slope at that instance
3. Seasonality - cyclical repeating pattern
## Exponential Smoothing
Before knowing what is exponential smoothing lets understand why it's required.
### Why exponential smoothing?
The real-world datasets for time series are hard to forecast and generally, it's assumed that data of recent past have higher significance compared to old data, hence more weightage is given to recent data than older data.
The problem faced with [weighted moving average](https://www.fidelity.com/learning-center/trading-investing/technical-analysis/technical-indicator-guide/wma) is choosing the appropriate weights for each level.
Exponential smoothing uses EWMA (Exponential Weighted Moving Average), i.e older data have lesser contribution compared to newer data. In EWMA weights reduce exponentially overtime and provide reasonable weights for each level.
### Formula
If you have some time series xt, you can define a new time series st that is a smoothed version of xt.

The following graph with α=0.5 shows how weights of older data diminish over time. The black bars denote the actual value(xt) and colored bars denote the smoothened value(st).

[Image Source](https://orangematter.solarwinds.com/2014/11/25/how-exponentially-weighted-moving-averages-work/)
## Holt's Model
Holt's model or Double Exponential Smoothing is an extension to simple exponential smoothing.
### Drawbacks of SES
- SES assumes the time series to be [stationary](https://otexts.com/fpp2/stationarity.html),i.e it assumes the statistical properties such as the mean, variance and autocorrelation are all constant over time.
- But in general, a majority of time series have either trend or seasonality component, thus forecasts made by SES are unproductive.
### What is Holt's Model?
Holt's model overcomes the drawback of SES by considering both level and trend component. The term **double exponential smoothing** was coined because exponential smoothing is performed both on level and trend component.
### Formula

- **F** - the forecast at time **t**.
- **D** - the actual value at time **t**.
- **a** - level at time **t**.
- **b** - trend/slope component at time **t**.
- **α** - smoothing parameter for level.
- **β** - smoothing parameter for trend.
The following graph illustrates how holt's model is used to forecast time series having a trend component.

[Image Source](https://orangematter.solarwinds.com/2017/06/22/exponential-smoothing-for-time-series-forecasting/)
### Comparison with Linear Regression
- Linear Regression is an effective method used to predict data points. The downside is that it considers all data with the same weightage.
- This property of LR isn't suitable for time series data, hence holt's is preferred over LR for time series analysis as the model uses the exponential smoothing.
## Holt-Winter Model
Holt-Winter model is an extension of Holt's model. This takes into account all the 3 time-series components (level, trend and seasonality). The seasonality component of time series is an important component since most real-world data have a seasonal period.
### Additive Model
Holt-Winter additive model is preferred when the time series shows constant or roughly constant seasonality over time.
#### Formula

- **F** - the forecast at time **t**.
- **D** - the actual value at time **t**.
- **a** - level at time **t**.
- **b** - trend/slope component at time **t**.
- **c** - seasonal component at time **t**.
- **α** - smoothing parameter for level.
- **β** - smoothing parameter for trend.
- **γ** - smoothing parameter for seasonality.
- **p** - period for the time series.
The following graph illustrates how the Holt-Winter additive model is used to predict seasonal data having a constant seasonality of period=12 or 1 year.

[Image Source](https://orangematter.solarwinds.com/2017/06/22/exponential-smoothing-for-time-series-forecasting/)
### Multiplicative Model
Holt-Winter multiplicative is useful in cases when the time series has the seasonality component changing over time.
#### Formula

The following graph illustrates how the multiplicative model is used to generate forecasts to predict seasonal data having the seasonal component changes over time.
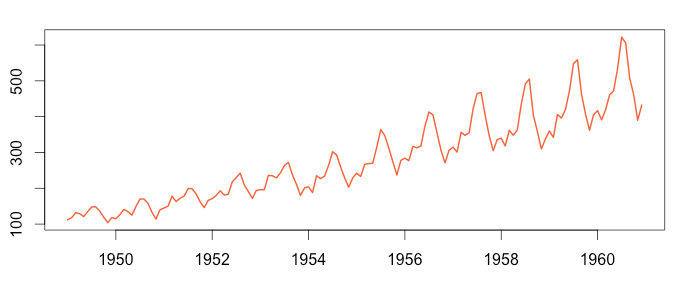
[Image So

茶了不几
- 粉丝: 36
- 资源: 4772
最新资源
- 聋哑人手语词汇图像分类数据集【已标注,约1,100张数据】
- 基于Pygame库实现新年烟花效果的Python代码
- 必应图片壁纸Python爬虫代码bing-img.zip
- 购物返利源码/代购网站源码/每日分打包完整版源码下载
- Java外卖项目(瑞吉外卖项目的扩展)
- 使用Python和matplotlib库绘制爱心图形的技术教程
- 国际象棋检测11-YOLO(v7至v9)、COCO、Darknet、Paligemma、VOC数据集合集.rar
- Python与Pygame实现带特效的圣诞节场景模拟程序
- R语言实战机器学习实战教程
- 常用算法介绍与学习资源汇总
- ssd5课件图片记录保存
- 国际象棋检测2-YOLO(v5至v11)、COCO、CreateML、Paligemma、TFRecord、VOC数据集合集.rar
- Offer资讯交流Web系统(编号:0889870).zip
- 高考志愿智能推荐系统_2a1qfv22.zip
- 个性化推荐影院(编号:03132141).zip
- 高校学生求职就业平台(编号:24440246).zip
资源上传下载、课程学习等过程中有任何疑问或建议,欢迎提出宝贵意见哦~我们会及时处理!
点击此处反馈


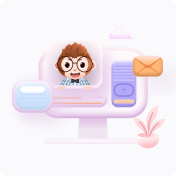
评论0