# Predicting Depression Using Health Care Data
**Author**: Vivienne DiFrancesco
<b> A companion dashboard for exploring the data used in this project can be found [here](https://share.streamlit.io/heythatsviv/predicting-depression/main/Dashboard/depression_app.py)</b>
The contents of this repository is an analysis of using machine learning models to predict depression in people using health care data. This analysis is detailed in hopes of making the work accessible and replicable.
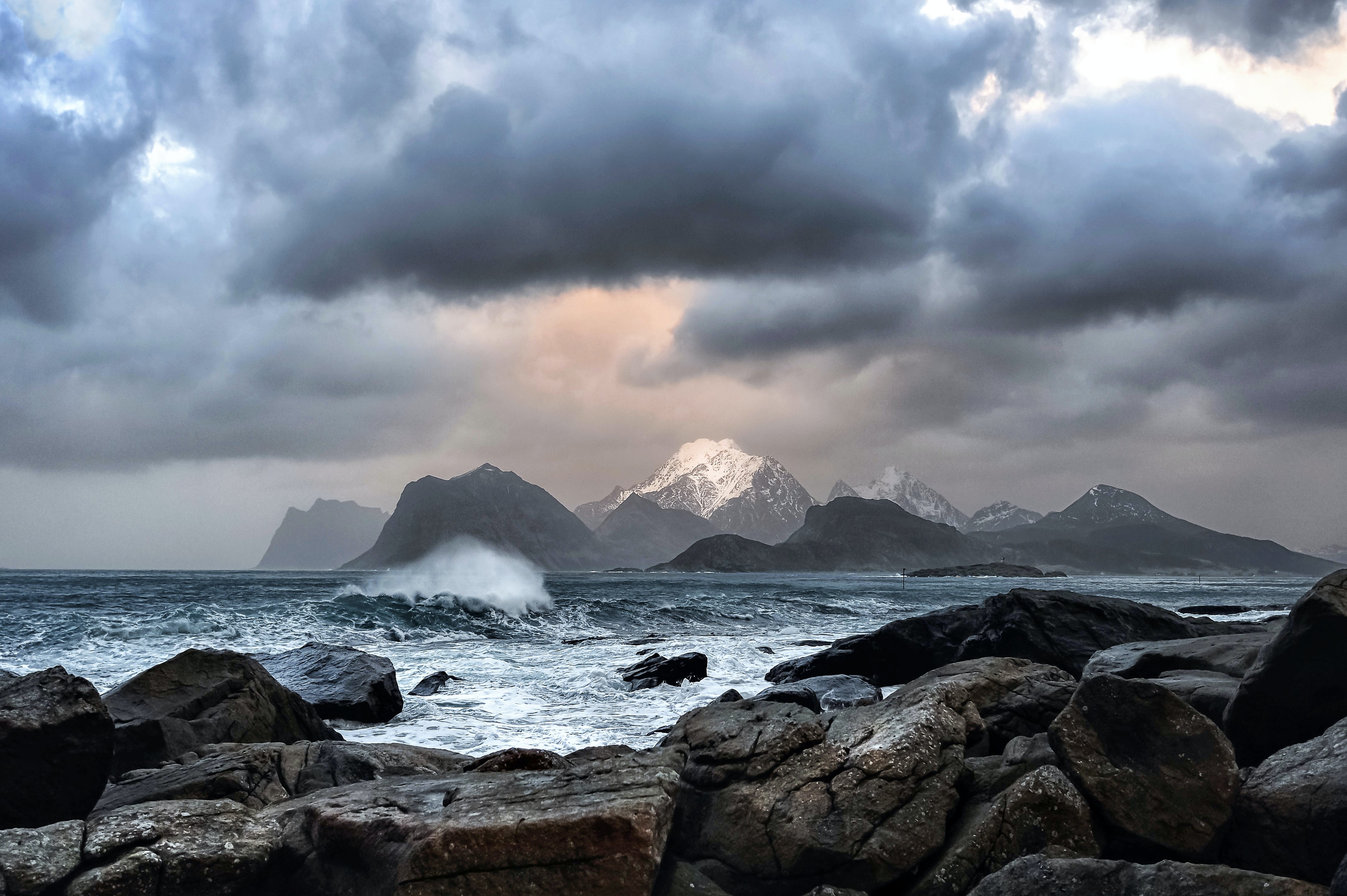
## Repository Structure
- <b>README.md:</b> The top level README for reviewers of this project
- <b>first_notebook.ipynb:</b> Beginning narrative documentation of analysis in jupyter notebook up through the data cleaning stages
- <b>second_notebook.ipynb:</b> Continuation of the narriative documentation that begins after data cleaning at the explore stage of the project
- <b>PredictingDepressionSlides.pdf:</b> PDF version of project presentation slides
- <b>project_functions folder:</b> Contains the custom functions written for use in the first_notebook and second_notebook
- <b>Dashboard folder:</b> Folder containing files for creating the companion dashboard for this project
## Abstract
Millions of people globally suffer from depression and it is a debilitating condition. At best it can be difficult for people to live their lives normally and happily, and at worst it leads to death by suicide. Primary care doctors are overwhelmingly finding that they are faced with the need to treat mental health conditions such as depression without any particular training of how to handle such cases.
There is evidence that an integrated approach where physicians regularly screen patients for mental health disorders and work together with psychologists and other mental health professionals to treat patients leads to reduced costs and better patient outcomes. However, this approach can require a lot of buy-in from many individuals, require extra training, and is often not logistically feasible.
Using data from the CDC National Health and Examination Survey, machine learning was applied to predict patients who may have depression based on information that could typically be found in a medical file. These predictions could be used to put patients in touch with experienced mental health professionals sooner and easier.
The results show that 71% of those who have depression and 80% of those who don't have depression can be correctly identified. Though more work needs to be done to create a more accurate model, this shows proof of concept that this is a realistic prediction task. Better results could be yielded by adding more patient information to the data or testing more types of models.
## Introduction
According to the World Health Organization, more that 264 million people globally have depression. Many suicides each year are caused by depression with suicide being among the leading causes of death for young people especially.[1](https://www.who.int/news-room/fact-sheets/detail/depression) The National Institute of Mental Health found that the prevalence of a major depressive episode among U.S. adults in 2017 was 7.1% of people with young adults being the most affected.[2](https://www.nimh.nih.gov/health/statistics/major-depression.shtml)
The American Psychological Association identified that primary care physicians are often being asked to diagnose mental disorders such as depression without adequate training on how to handle such treatments. According to their numbers, 70% of primary care visits are because of patients’ psychological problems, more than 80% of patients who have symptoms with no diagnosis receive psychological treatment by a physician, and only 10% follow up to a mental health professional. Patients are not getting the care they desperately need as 70% of individuals with depression go undiagnosed. Among people who commit suicide, 90% of people had a mental disorder and 40% of people had visited their doctor within the last month.[3](https://www.apa.org/health/briefs/primary-care.pdf)
In a study published in JAMA, doctors looked at patient outcomes, cost of care, and other factors between patients that were provided more overt diagnosing and treatment for mental health at standard doctor appointments versus patients that were not. They found that for patients that receive mental health intervention, costs went down, health care services were better utilized, patient outcomes improved, primary care doctor visits declined, treatment interventions were started earlier, and hospital and emergency care visits declined.[4](https://jamanetwork.com/journals/jama/fullarticle/2545685)
## Goal
The goal of this project is to gather data about people that would typically be in a patient’s medical record to predict depression.
Many clinics or doctors may find it impossible to have such integrated mental health services as cited in the previously mentioned study. Having standard services where patients are constantly screened for mental health disorders and treatment is tightly integrated with teams of physicians and psychological professionals can be expensive, requires a lot of training, requires participation from many individual doctors that may feel too overwhelmed, and may also not be possible in certain areas due to various logistical factors. Using machine learning and data that may otherwise be in a patient’s medical file, the goal is to predict who may have depression in a way that requires very little human participation from doctors and has lower time and money costs associated. The patients who are predicted to have depression could potentially be referred straight to mental health professionals in their area or who accept their health care coverage. The patient’s file could also be flagged to alert the medical staff the next time they have any kind of physician appointment to prompt doctors to start the conversation with patients. At the very least information and resources could be sent to patients directly to encourage them to take action on their own behalf.
## Data
The data for this project is from the Centers for Disease Control and Prevention National health and Nutrition Examination Survey. This data includes a vast array of health data done on a sample of the American population each year and is released every two years. The data can be found at this website: https://wwwn.cdc.gov/nchs/nhanes/default.aspx.
For this project, data was taken from the years between 2005 and 2018 and comprised of 36259 entries total of U.S. adults. Only data that was consistent across years was used and there was effort to only include data that would be reasonably found in a patient's medical file. Using as little data as possible while still being able to have accurate predictions is desirable as it would catch more people who may not have a very deep medical history and also puts less burden on providers to have to capture so much information.
## Approach
The target was calculated using the PHQ-9 depression screening tool that was asked of all participants in the NHANES data. A study showed that this screening tool has a specificity and sensitivity of 88% for major depression at a threshold score of 10 or more.[5](https://pubmed.ncbi.nlm.nih.gov/11556941/) People were divided into “depressed” and “not depressed” categories based on the score for their answers in the screening tool with a score of 10 or more being “depressed”.
The approach for this project was to create many different model types to see what performs the best and to compare and contrast the different types of models. The modeling effort was done starting with simpler models and moving to more complex models. The OSEMiN process is the overarching structure of this project.
## Methods
The way the data was preprocessed with feature engi

活宝spring
- 粉丝: 32
- 资源: 4686
最新资源
- 【中泰证券-2024研报-】银行戴志锋:重庆区域专题 经济景气度提升,个股基本面向好.pdf
- 【国海证券-2024研报-瑞鹄模具】2024Q3业绩点评:2024Q3营收利润同比增长,盈利能力提升.pdf
- 【格林期货-2024研报-】格林大华期货有色贵金属.pdf
- 【国海证券-2024研报-福耀玻璃】福耀玻璃(600660):2024Q3业绩符合预期,单季收入创历史新高.pdf
- 【国海证券-2024研报-福耀玻璃】2023年三季报点评:2024Q3业绩符合预期,单季收入创历史新高.pdf
- 【格林期货-2024研报-】一周简评:板块及品种.pdf
- 【格林期货-2024研报-】格林大华期货一周期市简评.pdf
- 【国海证券-2024研报-京東集團-SW】京东集团-SW(09618):2024Q3业绩前瞻:以旧换新推动收入增长回暖,利润稳健释放.pdf
- 【格林期货-2024研报-】格林大华期货一周简评.pdf
- 【格林期货-2024研报-】研究院专题报告:9月M1增速续创新低.pdf
- 【国海证券-2024研报-百亚股份】百亚股份(003006):2024年三季报点评:电商渠道持续高增,产品升级带动盈利稳步提升.pdf
- 【国海证券-2024研报-海光信息】海光信息(688041):科创板公司动态研究:Q3业绩倍数增长,存货+预付款展现成长信心.pdf
- 毕设&课程作业_基于C#的汽车衡智能称重系统.zip
- 毕设&课程作业_基于C#的课程管理系统基于vs2017+Sql Server环境.zip
- 毕设&课程作业_基于C#的股票分析系统客户端.zip
- 毕设&课程作业_基于C#的winform酒店管理系统,功能基本完善.zip
资源上传下载、课程学习等过程中有任何疑问或建议,欢迎提出宝贵意见哦~我们会及时处理!
点击此处反馈


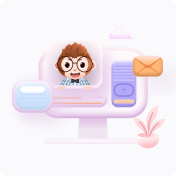